Thank you for visiting nature.com. You are using a browser version with limited support for CSS. To obtain the best experience, we recommend you use a more up to date browser (or turn off compatibility mode in Internet Explorer). In the meantime, to ensure continued support, we are displaying the site without styles and JavaScript.
- View all journals
- Explore content
- About the journal
- Publish with us
- Sign up for alerts

Image processing articles within Scientific Reports
Article 30 November 2024 | Open Access
Bilateral enhancement network with signal-to-noise ratio fusion for lightweight generalizable low-light image enhancement
- Junfeng Wang
- , Shenghui Huang
- & Yingxu Qiao
Article 29 November 2024 | Open Access
U-shape-based network for left ventricular segmentation in echocardiograms with contrastive pretraining
- Zhengkun Qian
- & Zizhong Yang
Face mask identification with enhanced cuckoo optimization and deep learning-based faster regional neural network
- Binay Kumar Pandey
- , Digvijay Pandey
- & Mesfin Esayas Lelisho
Article 28 November 2024 | Open Access
Innovative multi-class segmentation for brain tumor MRI using noise diffusion probability models and enhancing tumor boundary recognition
- Zengxin Liu
- , Caiwen Ma
- & Meilin Xie
A hypergraph cell membrane computing network model for soybean disease identification
- Yourui Huang
- , Hongping Song
- & Xiaoqiao Wang
Article 26 November 2024 | Open Access
Vascular insult in neonatal retinal hemorrhage: computational analysis of a fundus-segmented blood vessel network
- Matthew R. Lam
- , Jose A. Colmenarez
- & Donny W. Suh
Extraction and evaluation of features of preterm patent ductus arteriosus in chest X-ray images using deep learning
- Phillip Chang
- , Hyeon Sung Choi
- & Hyun Ho Kim
Article 25 November 2024 | Open Access
Bladder image stitching algorithm for navigation and referencing using a standard cystoscope
- , Nicole A. Varble
- & Bradford J. Wood
Article 22 November 2024 | Open Access
Scan speed affects quantitative optical coherence tomography angiography vascular metrics
- Lourdes Vidal-Oliver
- , Elisa Herzig-de Almeida
- & Robert P. Finger
Article 18 November 2024 | Open Access
Automated measurement and correlation analysis of fundus tessellation and optic disc characteristics in myopia
- , Lingzhi Chen
- & Jun Li
Generating and evaluating synthetic data in digital pathology through diffusion models
- Matteo Pozzi
- , Shahryar Noei
- & Giuseppe Jurman
Article 17 November 2024 | Open Access
Edge-guided multi-scale adaptive feature fusion network for liver tumor segmentation
- Tiange Zhang
- , Yuefeng Liu
- & Hongyu Shen
DTASUnet: a local and global dual transformer with the attention supervision U-network for brain tumor segmentation
- & Gang Yu
Article 13 November 2024 | Open Access
Isovolumic relaxation intraventricular pressure difference predicts elevated left ventricular end-diastolic pressure in patients with coronary artery disease
- Hongquan Lu
- , Fujian Duan
- & Hui Li
Article 12 November 2024 | Open Access
Detection and identification of centipedes based on deep learning
- Weitao Chen
- , Zhaoli Yao
- & Liangquan Jia
Article 11 November 2024 | Open Access
Continuous sign language recognition algorithm based on object detection and variable-length coding sequence
- & Changzhi Lv
Data free knowledge distillation with feature synthesis and spatial consistency for image analysis
- Pengchen Liang
- , Jianguo Chen
- & Guo Ran
Article 08 November 2024 | Open Access
Deep learning method for detecting fluorescence spots in cancer diagnostics via fluorescence in situ hybridization
- , Tianxiang Song
- & Kan Liu
Article 07 November 2024 | Open Access
Quantifying the recovery process of skeletal muscle on hematoxylin and eosin stained images via learning from label proportion
- , Weng Ian Chan
- & Hideo Matsuda
Article 06 November 2024 | Open Access
Deep learning hybrid model ECG classification using AlexNet and parallel dual branch fusion network model
- Manjur Kolhar
- & Ahmed M. Al Rajeh
Article 04 November 2024 | Open Access
Explainable fully automated CT scoring of interstitial lung disease for patients suspected of systemic sclerosis by cascaded regression neural networks and its comparison with experts
- Jingnan Jia
- , Irene Hernández-Girón
- & Berend C. Stoel
Cattle identification based on multiple feature decision layer fusion
- , Baoshan Li
- & Mingshuo Han
Article 03 November 2024 | Open Access
Learning super-resolution and pyramidal convolution residual network for vehicle re-identification
- Mengxue Liu
- , Weidong Min
- & Meng Zhu
Novel large empirical study of deep transfer learning for COVID-19 classification based on CT and X-ray images
- Mansour Almutaani
- , Turki Turki
- & Y.-H. Taguchi
Article 31 October 2024 | Open Access
Evaluating neonatal pain via fusing vision transformer and concept-cognitive computing
- , Liang Zhang
- & Yuping Zhang
Article 30 October 2024 | Open Access
AI predictive modeling of survival outcomes for renal cancer patients undergoing targeted therapy
- , Jirui Niu
- & Shiheng Sun
Article 29 October 2024 | Open Access
TCDDU-Net: combining transformer and convolutional dual-path decoding U-Net for retinal vessel segmentation
- & Shuping Zhang
Article 28 October 2024 | Open Access
Diagnostic precision in thyroid-associated ophthalmopathy using multi-center radiomics with 99m Tc-DTPA SPECT/CT
- , Mengting Li
- & Xiaotian Xia
Article 27 October 2024 | Open Access
Bone scintigraphy based on deep learning model and modified growth optimizer
- Omnia Magdy
- , Mohamed Abd Elaziz
- & Mohammed Sallah
Article 25 October 2024 | Open Access
GLE-net: global-local information enhancement for semantic segmentation of remote sensing images
- Junliang Yang
- , Guorong Chen
- & Huazheng Zhu
The two-stage detection-after-segmentation model improves the accuracy of identifying subdiaphragmatic lesions
- Chih-Hsiung Chen
- , Steven H. Hsu
- & Hsien-Yung Lai
Article 23 October 2024 | Open Access
Integrated ensemble CNN and explainable AI for COVID-19 diagnosis from CT scan and X-ray images
- Reenu Rajpoot
- , Mahesh Gour
- & Vijay Bhaskar Semwal
Article 22 October 2024 | Open Access
Automated volumetric analysis of the inner ear fluid space from hydrops magnetic resonance imaging using 3D neural networks
- Tae-Woong Yoo
- , Cha Dong Yeo
- & Eun Jung Lee
Article 21 October 2024 | Open Access
A deep learning based assisted analysis approach for Sjogren’s syndrome pathology images
- Peihe Jiang
- & Ning Lu
Article 19 October 2024 | Open Access
Enhanced climate change resilience on wheat anther morphology using optimized deep learning techniques
- Arifa Zahir
- , Zulfiqar Ali
- & Adnan Akhunzada
Article 15 October 2024 | Open Access
An automatic tracking method to measure the mandibula movement during real time MRI
- Jérémy Mouchoux
- , Florian Sojka
- & Anja Quast
Concordance in the estimation of tumor percentage in non-small cell lung cancer using digital pathology
- Irene Carretero-Barrio
- , Lara Pijuan
- & José Palacios
Assessment of NK cytotoxicity and interactions with porcine endothelial cells by live-cell imaging in 2D static and 3D microfluidic systems
- , Viktoriia Galdina
- & Jörg D. Seebach
Evaluation of particle tracking codes for dispersing particles in porous media
- Marc Berghouse
- , Filippo Miele
- & Rishi Parashar
Article 11 October 2024 | Open Access
The potential of federated learning for self-configuring medical object detection in heterogeneous data distributions
- Gabriel Rashidi
- , Dimitrios Bounias
- & Klaus H. Maier-Hein
Article 10 October 2024 | Open Access
Multi-scale input layers and dense decoder aggregation network for COVID-19 lesion segmentation from CT scans
- & Wenbing Jin
Efficient urinary stone type prediction: a novel approach based on self-distillation
- , Xuanqi Zhang
- & Kun Yang
Article 09 October 2024 | Open Access
Identification of texture MRI brain abnormalities on Fibromyalgia syndrome using interpretable machine learning models
- Hongyang Jiang
- , Aihui Liu
- & Zhenhua Ying
Deep learning pipeline for automated cell profiling from cyclic imaging
- Christian Landeros
- , Juhyun Oh
- & Hakho Lee
Integrating Kalman filter noise residue into U-Net for robust image denoising: the KU-Net model
- & K. C. Sriharipriya
Article 05 October 2024 | Open Access
A full-scale lung image segmentation algorithm based on hybrid skip connection and attention mechanism
- Qiong Zhang
- , Byungwon Min
- & Jianlin Qiu

The utility of MRI radiological biomarkers in determining intracranial pressure
- Anand S. Pandit
- , Musa China
- & Ahmed K. Toma
Article 01 October 2024 | Open Access
Retinal fundus image super-resolution based on generative adversarial network guided with vascular structure prior
- , Guangda Chen
- & Haotian Chi
Article 30 September 2024 | Open Access
Predicting coronary artery occlusion risk from noninvasive images by combining CFD-FSI, cGAN and CNN
- Mozhdeh Nikpour
- & Ali Mohebbi
Article 27 September 2024 | Open Access
Deep learning domain adaptation to understand physico-chemical processes from fluorescence spectroscopy small datasets and application to the oxidation of olive oil
- Umberto Michelucci
- & Francesca Venturini
Browse broader subjects
- Computational biology and bioinformatics
Quick links
- Explore articles by subject
- Guide to authors
- Editorial policies

Advertisement
Deep learning models for digital image processing: a review
- Published: 07 January 2024
- Volume 57 , article number 11 , ( 2024 )
Cite this article
- R. Archana 1 &
- P. S. Eliahim Jeevaraj 1
23k Accesses
41 Citations
Explore all metrics
Within the domain of image processing, a wide array of methodologies is dedicated to tasks including denoising, enhancement, segmentation, feature extraction, and classification. These techniques collectively address the challenges and opportunities posed by different aspects of image analysis and manipulation, enabling applications across various fields. Each of these methodologies contributes to refining our understanding of images, extracting essential information, and making informed decisions based on visual data. Traditional image processing methods and Deep Learning (DL) models represent two distinct approaches to tackling image analysis tasks. Traditional methods often rely on handcrafted algorithms and heuristics, involving a series of predefined steps to process images. DL models learn feature representations directly from data, allowing them to automatically extract intricate features that traditional methods might miss. In denoising, techniques like Self2Self NN, Denoising CNNs, DFT-Net, and MPR-CNN stand out, offering reduced noise while grappling with challenges of data augmentation and parameter tuning. Image enhancement, facilitated by approaches such as R2R and LE-net, showcases potential for refining visual quality, though complexities in real-world scenes and authenticity persist. Segmentation techniques, including PSPNet and Mask-RCNN, exhibit precision in object isolation, while handling complexities like overlapping objects and robustness concerns. For feature extraction, methods like CNN and HLF-DIP showcase the role of automated recognition in uncovering image attributes, with trade-offs in interpretability and complexity. Classification techniques span from Residual Networks to CNN-LSTM, spotlighting their potential in precise categorization despite challenges in computational demands and interpretability. This review offers a comprehensive understanding of the strengths and limitations across methodologies, paving the way for informed decisions in practical applications. As the field evolves, addressing challenges like computational resources and robustness remains pivotal in maximizing the potential of image processing techniques.
Similar content being viewed by others
A Comprehensive Analysis for Advancements and Challenges in Deep Learning Models for Image Processing
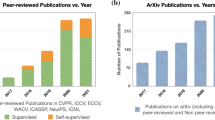
Image denoising in the deep learning era
Impact of Deep Learning in Image Processing and Computer Vision
Explore related subjects.
- Artificial Intelligence
Avoid common mistakes on your manuscript.
1 Introduction
Image Processing (IP) stands as a multifaceted field encompassing a range of methodologies dedicated to gleaning valuable insights from images. Concurrently, the landscape of Artificial Intelligence (AI) has burgeoned into an expansive realm of exploration, serving as the conduit through which intelligent machines strive to replicate human cognitive capacities. Within the expansive domain of AI, Machine Learning (ML) emerges as a pivotal subset, empowering models to autonomously extrapolate outcomes from structured datasets, effectively diminishing the need for explicit human intervention in the decision-making process. At the heart of ML lies Deep Learning (DL), a subset that transcends conventional techniques, particularly in handling unstructured data. DL boasts an unparalleled potential for achieving remarkable accuracy, at times even exceeding human-level performance. This prowess, however, hinges on the availability of copious data to train intricate neural network architectures, characterized by their multilayered composition. Unlike their traditional counterparts, DL models exhibit an innate aptitude for feature extraction, a task that historically posed challenges. This proficiency can be attributed to the architecture's capacity to inherently discern pertinent features, bypassing the need for explicit feature engineering. Rooted in the aspiration to emulate cognitive processes, DL strives to engineer learning algorithms that faithfully mirror the intricacies of the human brain. In this paper, a diverse range of deep learning methodologies, contributed by various researchers, is elucidated within the context of Image Processing (IP) techniques.
This comprehensive compendium delves into the diverse and intricate landscape of Image Processing (IP) techniques, encapsulating the domains of image restoration, enhancement, segmentation, feature extraction, and classification. Each domain serves as a cornerstone in the realm of visual data manipulation, contributing to the refinement, understanding, and utilization of images across a plethora of applications.
Image restoration techniques constitute a critical first step in rectifying image degradation and distortion. These methods, encompassing denoising, deblurring, and inpainting, work tirelessly to reverse the effects of blurring, noise, and other forms of corruption. By restoring clarity and accuracy, these techniques lay the groundwork for subsequent analyses and interpretations, essential in fields like medical imaging, surveillance, and more.
The purview extends to image enhancement, where the focus shifts to elevating image quality through an assortment of adjustments. Techniques that manipulate contrast, brightness, sharpness, and other attributes enhance visual interpretability. This enhancement process, applied across diverse domains, empowers professionals to glean finer details, facilitating informed decision-making and improved analysis.
The exploration further extends to image segmentation, a pivotal process for breaking down images into meaningful regions. Techniques such as clustering and semantic segmentation aid in the discernment of distinct entities within images. The significance of image segmentation is particularly pronounced in applications like object detection, tracking, and scene understanding, where it serves as the backbone of accurate identification and analysis.
Feature extraction emerges as a fundamental aspect of image analysis, entailing the identification of crucial attributes that pave the way for subsequent investigations. While traditional methods often struggle to encapsulate intricate attributes, deep learning techniques excel in autonomously recognizing complex features, contributing to a deeper understanding of images and enhancing subsequent analysis.
Image classification, a quintessential task in the realm of visual data analysis, holds prominence. This process involves assigning labels to images based on their content, playing a pivotal role in areas such as object recognition and medical diagnosis. Both machine learning and deep learning techniques are harnessed to automate the accurate categorization of images, enabling efficient and effective decision-making.
The Sect. 1 elaborates the insights of the image processing operations. In Sect. 2 of this paper, a comprehensive overview of the evaluation metrics employed for various image processing operations is provided. Moving to Sect. 3 , an in-depth exploration unfolds concerning the diverse range of Deep Learning (DL) models specifically tailored for image preprocessing tasks. Within Sect. 4 , a thorough examination ensues, outlining the array of DL methods harnessed for image segmentation tasks, unraveling their techniques and applications.
Venturing into Sect. 5 , a meticulous dissection is conducted, illuminating DL strategies for feature extraction, elucidating their significance and effectiveness. In Sect. 6 , the spotlight shifts to DL models designed for the intricate task of image classification, delving into their architecture and performance characteristics. The significance of each models are discussed in Sect. 7 . Concluding this comprehensive analysis, Sect. 8 encapsulates the synthesized findings and key takeaways, consolidating the insights gleaned from the study.
The array of papers discussed in this paper collectively present a panorama of DL methodologies spanning various application domains. Notably, these domains encompass medical imagery, satellite imagery, botanical studies involving flower images, as well as fruit images, and even real-time image scenarios. Each domain's unique challenges and intricacies are met with tailored DL approaches, underscoring the adaptability and potency of these methods across diverse real-world contexts.
2 Metrics for image processing operations
Evaluation metrics serve as pivotal tools in the assessment of the efficacy and impact of diverse image processing techniques. These metrics serve the essential purpose of furnishing quantitative measurements that empower researchers and practitioners to undertake an unbiased analysis and facilitate meaningful comparisons among the outcomes yielded by distinct methods. By employing these metrics, the intricate and often subjective realm of image processing can be rendered more objective, leading to informed decisions and advancements in the field.
2.1 Metrics for image preprocessing
2.1.1 mean squared error (mse).
The average of the squared differences between predicted and actual values. It penalizes larger errors more heavily.
where, M and N are the dimensions of the image. \({Original}_{(i,j)}\,and\, {Denoised}_{(i,j)}\) are the pixel values at position (i, j) in the original and denoised images respectively.
2.1.2 Peak signal-to-noise ratio (PSNR)
PSNR is commonly used to measure the quality of restored images. It compares the original and restored images by considering the mean squared error between their pixel values.
where, MAX is the maximum possible pixel value (255 for 8-bit images), MSE is the mean squared error between the original and denoised images.
2.1.3 Structural similarity index (SSIM)
SSIM is applicable to image restoration as well. It assesses the similarity between the original and restored images in terms of luminance, contrast, and structure. Higher SSIM values indicate better restoration quality.
\({SSIM}_{\left(x,y\right)}=\left(2*{\mu }_{x }*{\mu }_{y }+{c}_{1}\right)*(2*{\sigma }_{xy }+{c}_{2})/({\mu }_{x}^{2}+{\mu }_{y}^{2}+{c}_{1})*({\sigma }_{x}^{2}+{\sigma }_{y}^{2}+{c}_{2}\) ).where, \({\mu }_{x }and {\mu }_{y}\) are the mean values of the original and denoised images. \({\sigma }_{x}^{2} and {\sigma }_{y}^{2}\) are the variances of the original and denoised images. \({\sigma }_{xy}\) is the covariance between the original and denoised images. \({c}_{1}{ and c}_{2}\) are constants to avoid division by zero.
2.1.4 Mean structural similarity index (MSSIM)
MSSIM extends SSIM to multiple patches of the image and calculates the mean SSIM value over those patches.
where x i and y i are the patches of the original and enhanced images.
2.1.5 Mean absolute error (MAE)
The average of the absolute differences between predicted and actual values. It provides a more robust measure against outliers.
where n is the number of samples.
2.1.6 NIQE (Naturalness image quality evaluator)
NIQE quantifies the naturalness of an image by measuring the deviation of local statistics from natural images. It calculates the mean of the local differences in luminance and contrast.
2.1.7 FID (Fréchet inception distance)
FID measures the distance between two distributions (real and generated images) using the Fréchet distance between their feature representations calculated by a pre-trained neural network.
2.2 Metrics for image segmentation
2.2.1 intersection over union (iou).
IoU measures the overlap between the predicted bounding box and the ground truth bounding box. Commonly used to evaluate object detection models.
2.2.2 Average precision (AP)
AP measures the precision at different recall levels and computes the area under the precision-recall curve. Used to assess object detection and instance segmentation models.
2.2.3 Dice similarity coefficient
The Dice similarity coefficient is another measure of similarity between the predicted segmentation and ground truth. It considers both false positives and false negatives.
The Dice Similarity Coefficient, also known as the Sørensen-Dice coefficient, is a common metric for evaluating the similarity between two sets. In the context of image segmentation, it quantifies the overlap between the predicted segmentation and the ground truth, taking into account both true positives and false positives. DSC ranges from 0 to 1, where higher values indicate better overlap between the predicted and ground truth segmentations. A DSC of 1 corresponds to a perfect match.
2.2.4 Average accuracy (AA)
Average Accuracy measures the overall accuracy of the segmentation by calculating the percentage of correctly classified pixels across all classes.
where, N is the number of classes. True Positives i and True Negativesi are the true positives and true negatives for class ii. Total Pixels i is the total number of pixels in class.
2.3 Metrics for feature extraction and classification
2.3.1 accuracy.
The ratio of correctly predicted instances to the total number of instances. It's commonly used for balanced datasets but can be misleading for imbalanced datasets.
2.3.2 Precision
The ratio of true positive predictions to the total number of positive predictions. It measures the model’s ability to avoid false positives.
2.3.3 Recall (Sensitivity or true positive rate)
The ratio of true positive predictions to the total number of actual positive instances. It measures the model’s ability to correctly identify positive instances.
2.3.4 F1-Score
The harmonic mean of precision and recall. It provides a balanced measure between precision and recall.
2.3.5 Specificity (True negative rate)
The ratio of true negative predictions to the total number of actual negative instances.
2.3.6 ROC curve (Receiver operating characteristic curve )
A graphical representation of the trade-off between true positive rate and false positive rate as the classification threshold varies. These metrics are commonly used in binary classification. The ROC curve plots this trade-off, and AUC summarizes the curve's performance.
3 Image preprocessing
Image preprocessing is a fundamental step in the field of image processing that involves a series of operations aimed at preparing raw or unprocessed images for further analysis, interpretation, or manipulation. This crucial phase helps enhance the quality of images, mitigate noise, correct anomalies, and extract relevant information, ultimately leading to more accurate and reliable results in subsequent tasks such as image analysis, recognition, and classification.
Image preprocessing is broadly categorized into image restoration which removes the noises and blurring in the images and image enhancement which improves the contrast, brightness and details of the images.
3.1 Image restoration
Image restoration serves as a pivotal process aimed at reclaiming the integrity and visual quality of images that have undergone degradation or distortion. Its objective is to transform a degraded image into a cleaner, more accurate representation, thereby revealing concealed details that may have been obscured. This process is particularly vital in scenarios where images have been compromised due to factors like digital image acquisition issues or post-processing procedures such as compression and transmission. By rectifying these issues, image restoration contributes to enhancing the interpretability and utility of visual data.
A notable adversary in the pursuit of pristine images is noise, an unintended variation in pixel values that introduces unwanted artifacts and can lead to the loss of important information. Different types of noise, such as Gaussian noise characterized by its random distribution, salt and pepper noise causing sporadic bright and dark pixels, and speckle noise resulting from interference, can mar the quality of images. These disturbances often originate from the acquisition process or subsequent manipulations of the image data.
Historically, traditional image restoration techniques have included an array of methods to mitigate the effects of degradation and noise. These techniques encompass constrained least square filters, blind deconvolution methods that aim to reverse the blurring effects, Weiner and inverse filters for enhancing signal-to-noise ratios, as well as Adaptive Mean, Order Static, and Alpha-trimmed mean filters that tailor filtering strategies based on the local pixel distribution. Additionally, algorithms dedicated to deblurring counteract motion or optical-induced blurriness, restoring sharpness. Denoising techniques (Tian et al. 2018 ; Peng et al. March 2020 ; Tian and Fei 2020 ) such as Total Variation Denoising (TVD) and Non-Local Means (NLM) further contribute by effectively reducing random noise while preserving essential image details, collectively advancing the field's capacity to improve image integrity and visual clarity. In Table 1 , a summary of deep learning models for image restoration is provided, including their respective advantages and disadvantages.
Recent advancements in deep learning, particularly through Convolutional Neural Networks (CNN), have revolutionized the field of image restoration. CNNs are adept at learning and extracting complex features from images, allowing them to recognize patterns and nuances that may be challenging for traditional methods to discern. Through extensive training on large datasets, these networks can significantly enhance the quality of restored images, often surpassing the capabilities of conventional techniques. This leap in performance is attributed to the network's ability to implicitly understand the underlying structures of images and infer optimal restoration strategies.
Chunwei Tiana et al. (Tian and Fei 2020 ) provided an overview of deep network utilization in denoising images to eliminate Gaussian noise. They explored deep learning techniques for various noisy tasks, including additive white noisy images, blind denoising, and real noisy images. Through benchmark dataset analysis, they assessed the denoising outcomes, efficiency, and visual effects of distinct networks, followed by cross-comparisons of different image denoising methods against diverse types of noise. They concluded by addressing the challenges encountered by deep learning in image denoising.
Quan et al. ( 2020 ) introduced a self-supervised deep learning method named Self2Self for image denoising. Their study demonstrated that the denoising neural network trained with the Self2Self scheme outperformed non-learning-based denoisers and single-image-learning denoisers.
Yan et al. ( 2020 ) proposed a novel technique for removing speckle noise in digital holographic speckle pattern interferometry (DHSPI) wrapped phase. Their method employed improved denoising convolutional neural networks (DnCNNs) and evaluated noise reduction using Mean Squared Error (MSE) comparisons between noisy and denoised data.
Sori et al. ( 2021 ) presented lung cancer detection from denoised Computed Tomography images using a two-path convolutional neural network (CNN). They employed the denoised image by DR-Net as input for lung cancer detection, achieving superior results in accuracy, sensitivity, and specificity compared to recent approaches.
Pang et al. ( 2021 ) implemented an unsupervised deep learning method for denoising using unmatched noisy images, with a loss function analogous to supervised training. Their model, based on the Additive White Gaussian Noise model, attained competitive outcomes against unsupervised methods.
Hasti and Shin ( 2022 ) proposed a deep learning approach to denoise fuel spray images derived from Mie scattering and droplet center detection. A comprehensive comparison of diverse algorithms—standard CNN, modified ResNet, and modified U-Net—revealed the superior performance of the modified U-Net architecture in terms of Mean Squared Error (MSE) and Peak Signal-to-Noise Ratio (PSNR).
Niresi and Chi et al. ( 2022 ) employed an unsupervised HSI denoising algorithm under the DIP framework, which minimized the Half-Quadratic Lagrange Function (HLF) without regularizers, effectively removing mixed types of noises like Gaussian noise and sparse noise while preserving edges. Zhou et al. ( 2022 ) introduced a novel bearing fault diagnosis model called deep network-based sparse denoising (DNSD). They addressed the challenges faced by traditional sparse theory algorithms, demonstrating that DNSD overcomes issues related to generalization, parameter adjustment, and data-driven complexity. Tawfik et al. ( 2022 ) conducted a comprehensive evaluation of image denoising techniques, categorizing them as traditional (user-based) non-learnable denoising filters and DL-based methods. They introduced semi-supervised denoising models and employed qualitative and quantitative assessments to compare denoising performance. Meng and Zhang et al. ( 2022 ) proposed a gray image denoising method utilizing a constructed symmetric and dilated convolutional residual network. Their technique not only effectively removed noise in high-noise settings but also achieved higher SSIM, PSNR, FOM, and improved visual effects, offering valuable data for subsequent applications like target detection, recognition, and tracking.
In essence, image restoration encapsulates a continuous endeavor to salvage and improve the visual fidelity of images marred by degradation and noise. As technology advances, the integration of deep learning methodologies promises to propel this field forward, ushering in new standards of image quality and accuracy.
3.2 Image enhancement
Image enhancement refers to the process of manipulating an image to improve its visual quality and interpretability for human perception. This technique involves various adjustments that aim to reveal hidden details, enhance contrast, and sharpen edges, ultimately resulting in an image that is clearer and more suitable for analysis or presentation. The goal of image enhancement is to make the features within an image more prominent and recognizable, often by adjusting brightness, contrast, color balance, and other visual attributes.
Standard image enhancement methods encompass a range of techniques, including histogram matching to adjust the pixel intensity distribution, contrast-limited adaptive histogram equalization (CLAHE) to enhance local contrast, and filters like the Wiener filter and median filter to reduce noise. Linear contrast adjustment and unsharp mask filtering are also commonly employed to boost image clarity and sharpness.
In recent years, deep learning methods have emerged as a powerful approach for image enhancement. These techniques leverage large datasets and complex neural network architectures to learn patterns and features within images, enabling them to restore and enhance images with impressive results. Researchers have explored various deep learning models for image enhancement, each with its strengths and limitations. These insights are summarized in Table 2 .
The study encompasses an array of innovative techniques, including the integration of Retinex theory and deep image priors in the Novel RetinexDIP method, robustness-enhancing Fuzzy operation to mitigate overfitting, and the fusion of established techniques like Unsharp Masking, High-Frequency Emphasis Filtering, and CLAHE with EfficientNet-B4, ResNet-50, and ResNet-18 architectures to bolster generalization and robustness. Among these, FCNN Mean Filter exhibits computational efficiency, while CV-CNN leverages the capabilities of complex-valued convolutional networks. Additionally, the versatile pix2pixHD framework and the swift convergence of LE-net (Light Enhancement Net) contribute to the discourse. Deep Convolutional Neural Networks demonstrate robust enhancements, yet require meticulous hyperparameter tuning. Finally, MSSNet-WS (Multi-Scale-Stage Network) efficiently converges and addresses overfitting. This analysis systematically highlights their merits, encompassing improved convergence rates, overfitting mitigation, robustness, and computational efficiency.
Gao et al. ( 2022 ) proposed an inventive approach for enhancing low-light images by leveraging Retinex decomposition after initial denoising. In their method, the Retinex decomposition technique was applied to restore brightness and contrast, resulting in images that are clearer and more visually interpretable. Notably, their method underwent rigorous comparison with several other techniques, including LIME, NPE, SRIE, KinD, Zero-DCE, and RetinexDIP, showcasing its superior ability to enhance image quality while preserving image resolution and minimizing memory usage (Tables 1 , 2 , 3 , 4 and 5 ).
Liu et al. ( 2019 ) explored the application of deep learning in iris recognition, utilizing Fuzzy-CNN (F-CNN) and F-Capsule models. What sets their approach apart is the integration of Gaussian and triangular fuzzy filters, a novel enhancement step that contributes to improving the clarity of iris images. The significance lies in the method’s practicality, as it smoothly integrates with existing networks, offering a seamless upgrade to the recognition process.
Munadi et al. ( 2020 ) combined deep learning techniques with image enhancement methodologies to tackle tuberculosis (TB) image classification. Their innovative approach involved utilizing Unsharp Masking (UM) and High-Frequency Emphasis Filtering (HEF) in conjunction with EfficientNet-B4, ResNet-50, and ResNet-18 models. By evaluating the performance of three image enhancement algorithms, their work demonstrated remarkable accuracy and Area Under Curve (AUC) scores, revealing the potential of their method for accurate TB image diagnosis.
Lu et al. ( 2021 ) introduced a novel application of deep learning, particularly the use of a fully connected neural network (FCNN), to address impulse noise in degraded images with varying noise densities. What's noteworthy about their approach is the development of an FCNN mean filter that outperformed traditional mean/median filters, especially when handling low-noise density environments. Their study thus highlights the promising capabilities of deep learning in noise reduction scenarios. Quan et al. ( 2020 ) presented a non-blind image deblurring technique employing complex-valued CNN (CV-CNN). The uniqueness of their approach lies in incorporating Gabor-domain denoising as a prior step in the deconvolution model. By evaluating their model using quantitative metrics like Peak Signal-to-Noise Ratio (PSNR) and Structural Similarity Index (SSIM), their work showcased effective deblurring outcomes, reaffirming the potential of complex-valued CNNs in image restoration.
Jin et al. ( 2021 ) harnessed the power of deep learning, specifically the pix2pixHD model, to enhance multidetector computed tomography (MDCT) images. Their focus was on accurately measuring vertebral bone structure. By utilizing MDCT images, their approach demonstrated the potential of deep learning techniques in precisely enhancing complex medical images, which can play a pivotal role in accurate clinical assessments.
Li et al. ( 2021a ) introduced a CNN-based LE-net tailored for image recovery in low-light conditions, catering to applications like driver assistance systems and connected autonomous vehicles (CAV). Their work highlighted the significance of their model in outperforming traditional approaches and even other deep learning models. The research underscores the importance of tailored solutions for specific real-world scenarios.
Mehranian et al. ( 2022 ) ventured into the realm of Time-of-Flight (ToF) enhancement in positron emission tomography (PET) images using deep convolutional neural networks. Their innovative use of the block-sequential-regularized-expectation–maximization (BSREM) algorithm for PET data reconstruction in combination with DL-ToF(M) demonstrated superior diagnostic performance, measured through metrics like SSIM and Fréchet Inception Distance (FID).
Kim et al. ( 2022 ) introduced the Multi-Scale-Stage Network (MSSNet), a pioneering deep learning-based approach for single image deblurring. What sets their work apart is their meticulous analysis of previous deep learning-based coarse-to-fine approaches, leading to the creation of a network that achieves state-of-the-art performance in terms of image quality, network size, and computation time.
In the core, image enhancement plays a crucial role in improving the visual quality of images, whether for human perception or subsequent analytical tasks. The combination of traditional methods and cutting-edge deep learning techniques continues to advance our ability to reveal and amplify important information within images. Each of these studies contributes to the expanding landscape of image enhancement and restoration, showcasing the immense potential of deep learning techniques in various domains, from medical imaging to low-light scenarios, while addressing specific challenges and advancing the state-of-the-art in their respective fields.
However, the study recognizes inherent limitations, including constrained adaptability, potential loss of intricate details, and challenges posed by complex scenes or real-world images. Through a meticulous exploration of these advantages and disadvantages, the study endeavors to offer a nuanced perspective on the diverse applicability of these methodologies across various image enhancement scenarios.
4 Image segmentation
Image segmentation is a pivotal process that involves breaking down an image into distinct segments based on certain discernible characteristics such as intensity, color, texture, or spatial proximity. This technique is classified into two primary categories: Semantic segmentation and Instance segmentation. Semantic segmentation assigns each pixel to a specific class within the input image, enabling the identification of distinct object regions. On the other hand, instance segmentation takes a step further by not only categorizing pixels into classes but also differentiating individual instances of those classes within the image.
Traditional segmentation methodologies entail the partitioning of data, such as images, into well-defined segments governed by predetermined criteria. This approach predates the era of deep learning and relies on techniques rooted in expert-designed features or domain-specific knowledge. Common techniques encompass thresholding, which categorizes pixels into object and background regions using specific intensity thresholds, region-based segmentation that clusters pixels with similar attributes into coherent regions, and edge detection to identify significant intensity transitions that might signify potential boundaries.Nonetheless, traditional segmentation techniques grapple with inherent complexities when it comes to handling intricate shapes, dynamic backgrounds, and noise within the data. Moreover, the manual craftsmanship of features for various scenarios can be laborious and might not extend well to different contexts. In contrast, deep learning has ushered in a paradigm shift in segmentation by introducing automated feature learning. Deep neural networks have the remarkable ability to extract intricate features directly from raw data, negating the necessity for manual feature engineering. This empowers them to capture nuanced spatial relationships and adapt to variations, effectively addressing the limitations inherent in traditional methods. This transformation, especially pronounced in image segmentation tasks, has opened doors to unprecedented possibilities in the field of computer vision and image analysis. Table 3 encapsulates the strengths and limitations of various explored deep learning models.
Ahmed et al. ( 2020 ) conducted a comprehensive exploration of deep learning-based semantic segmentation models for the challenging task of top-view multiple person segmentation. They assessed the performance of key models, including Fully Convolutional Neural Network (FCN), U-Net, and DeepLabV3. This investigation is particularly important as accurate segmentation of multiple individuals in top-view images holds significance in various applications like surveillance, crowd monitoring, and human–computer interaction. The researchers found that DeepLabV3 and U-Net outperformed FCN in terms of accuracy. These models achieved impressive accuracy and mean Intersection over Union (mIoU) scores, indicating the precision of segmentation, with DeepLabV3 and U-Net leading the way. The results underscore the value of utilizing advanced deep learning models for complex segmentation tasks involving multiple subjects.
Wang et al. ( 2020 ) proposed an adaptive segmentation algorithm employing the UNet structure, which is adept at segmenting both shallow and deep features. Their study addressed the challenge of segmenting complex boundaries within images, a crucial task in numerous medical imaging and computer vision applications. They validated their model's effectiveness on natural scene images and liver cancer CT images, highlighting its advantages over existing segmentation methods. This research contributes to the field by showcasing the potential of adaptive segmentation algorithms, emphasizing their superiority in handling intricate boundaries in diverse image datasets.
Ahammad et al. ( 2020 ) introduced a novel deep learning framework based on Convolutional Neural Networks (CNNs) for diagnosing Spinal Cord Injury (SCI) features through segmentation. This study's significance lies in its application to medical imaging, specifically spinal cord disease prediction. Their model’s high computational efficiency and remarkable accuracy underscore its potential clinical utility. The CNN-based framework leveraged sensor SCI image data, demonstrating the capacity of deep learning to contribute to accurate diagnosis and prediction in medical scenarios, enhancing patient care.
Lorenzoni et al. ( 2020 ) employed Deep Learning techniques based on Convolutional Neural Networks (CNNs) to automate the segmentation of microCT images of distinct cement-based composites. This research is essential in materials science and civil engineering, where automated segmentation can aid in understanding material properties. Their study emphasizes the adaptability of Deep Learning models, showcasing the transferability of network parameters optimized on high-strength materials to other related contexts. This work demonstrates the potential of CNN-based methodologies for advancing materials characterization and analysis.
Mahajan et al. ( 2021 ) introduced a clustering-based profound iterating Deep Learning model (CPIDM) for hyperspectral image segmentation. This research addresses the challenge of segmenting hyperspectral images, which are prevalent in fields like remote sensing and environmental monitoring. The proposed approach's superiority over state-of-the-art methods indicates its potential for enhancing the accuracy of hyperspectral image analysis. The study contributes to the field by providing an innovative methodology to tackle the unique challenges posed by hyperspectral data.
Jalali et al. ( 2021 ) designed a novel deep learning-based approach for segmenting lung regions from CT images using Bi-directional ConvLSTM U-Net with densely connected convolutions (BCDU-Net). This research is critical for medical image analysis, specifically lung-related diagnoses. Their model's impressive accuracy on a large dataset indicates its potential for aiding radiologists in identifying lung regions accurately. The application of advanced deep learning architectures to medical imaging tasks underscores the transformative potential of such technologies in healthcare.
Bouteldja et al. ( 2020 ) developed a CNN-based approach for accurate multiclass segmentation of stained kidney images from various species and renal disease models. This research’s significance lies in its potential contribution to histopathological analysis and disease diagnosis. The model's high performance across diverse species and disease models highlights its robustness and utility for aiding pathologists in accurate image-based diagnosis.
Liu et al. ( 2021 ) proposed a novel convolutional neural network architecture incorporating cross-connected layers and multi-scale feature aggregation for image segmentation. The research addresses the need for advanced segmentation techniques that can capture intricate features and relationships within images. Their model's impressive performance metrics underscore its potential for enhancing segmentation accuracy, which is pivotal in diverse fields, including medical imaging, robotics, and autonomous systems.
Saood and Hatem et al. ( 2021 ) introduced deep learning networks, SegNet and U-Net, for segmenting COVID-19-infected areas in CT scan images. This research's timeliness is evident, as it contributes to the fight against the global pandemic. Their comparison of network performance provides insights into the effectiveness of different deep learning architectures for accurately identifying infected regions in lung images. This work showcases the agility of deep learning in addressing real-world challenges.
Nurmain et al. ( 2020 ), a novel approach employing Mask-RCNN is introduced for accurate fetal septal defect detection. Addressing limitations in previous methods, the model demonstrates multiclass heart chamber detection with high accuracy: right atrium (97.59%), left atrium (99.67%), left ventricle (86.17%), right ventricle (98.83%), and aorta (99.97%). Competitive results are shown for defect detection in atria and ventricles, with MRCNN achieving around 99.48% mAP compared to 82% for FRCNN. The study concludes that the proposed MRCNN model holds promise for aiding cardiologists in early fetal congenital heart disease screening.
Park et al. ( 2021a ) propose a method for intelligently segmenting food in images using deep neural networks. They address labor-intensive data collection by utilizing synthetic data through 3D graphics software Blender, training Mask R-CNN for instance segmentation. The model achieves 52.2% on real-world food instances with only synthetic data, and + 6.4%p performance improvement after fine-tuning compared to training from scratch. Their approach shows promise for healthcare robot systems like meal assistance robots.
Pérez-Borrero et al. ( 2020 ) underscores the significance of fruit instance segmentation, specifically within autonomous fruit-picking systems. It highlights the adoption of deep learning techniques, particularly Mask R-CNN, as a benchmark. The review justifies the proposed methodology's alterations to address limitations, emphasizing its efficiency gains. Additionally, the introduction of the Instance Intersection Over Union (I2oU) metric and the StrawDI_Db1 dataset creation are positioned as contributions with real-world implementation potential.
These studies collectively highlight the transformative impact of deep learning in various segmentation tasks, ranging from medical imaging to materials science and computer vision. By leveraging advanced neural network architectures and training methodologies, researchers are pushing the boundaries of what is achievable in image segmentation, ultimately contributing to advancements in diverse fields and applications.
5 Feature extraction
Feature extraction is a fundamental process in image processing and computer vision that involves transforming raw pixel data into a more compact and informative representation, often referred to as features. These features capture important characteristics of the image, making it easier for algorithms to understand and analyze images for various tasks like object recognition, image classification, and segmentation. Traditional methods of feature extraction were prevalent before the rise of deep learning and involved techniques that analyzed pixel-level information.Some traditional methods are explained here. Principle Components Analysis (PCA) is a statistical technique that reduces the dimensionality of the data while retaining as much of the original variance as possible. It identifies the orthogonal axes (principal components) along which the data varies the most. Independent Component Analysis (ICA) aims to find a linear transformation of the data into statistically independent components. It is often used for separating mixed sources in images, such as separating different image sources from a single mixed image. Locally Linear Embedding (LLE) is a nonlinear dimensionality reduction technique that aims to preserve the local structure of data points. It finds a low-dimensional representation of the data while maintaining the neighborhood relationships.
These traditional methods of feature extraction have been widely used and have provided valuable insights and representations for various image analysis tasks. However, they often rely on handcrafted features designed by experts or domain knowledge, which can be labor-intensive and may not generalize well across different types of images or tasks.
Conventional methods of feature extraction encompass the conversion of raw data into a more concise and insightful representation by pinpointing specific attributes or characteristics. These selected features are chosen to encapsulate vital insights and patterns inherent in the data. This procedure often involves a manual approach guided by domain expertise or specific insights. For example, within image processing, methods like Histogram of Oriented Gradients (HOG) might extract insights about gradient distributions, while in text analysis, features such as word frequencies could be selected.
Despite the effectiveness of traditional feature extraction for particular tasks and its ability to provide data insights, it comes with inherent limitations. Conventional techniques frequently necessitate expert intervention to craft features, which can be a time-intensive process and might overlook intricate relationships or patterns within the data. Moreover, traditional methods might encounter challenges when dealing with data of high dimensionality or scenarios where features are not easily definable.
In contrast, the ascent of deep learning approaches has revolutionized feature extraction by automating the process. Deep neural networks autonomously learn to extract meaningful features directly from raw data, eliminating the need for manual feature engineering. This facilitates the capture of intricate relationships, patterns, and multifaceted interactions that traditional methods might overlook. Consequently, deep learning has showcased exceptional achievements across various domains, particularly in tasks involving intricate data, such as image and speech recognition. Table 4 succinctly outlines the metrics, strengths and limitations of diverse deep learning models explored for feature enhancement.
Magsi et al. ( 2020 ) embarked on a significant endeavor in the realm of disease identification within date palm trees by harnessing the power of deep learning techniques. Their study centered around texture and color extraction methods from images of various date palm diseases. Through the application of Convolutional Neural Networks (CNNs), they effectively created a system that could discern diseases based on specific visual patterns. The achieved accuracy of 89.4% signifies the model's proficiency in accurately diagnosing diseases within this context. This approach not only showcases the potential of deep learning in addressing agricultural challenges but also emphasizes the importance of automated disease detection for crop management and security.
Sharma et al. ( 2020 ) delved into the domain of medical imaging with a focus on chest X-ray images. They introduced a comprehensive investigation involving different deep Convolutional Neural Network (CNN) architectures to facilitate the extraction of features from these images. Notably, the study evaluated the impact of dataset size on CNN performance, highlighting the scalability of their approach. By incorporating augmentation and dropout techniques, the model achieved a high accuracy of 0.9068, suggesting its ability to accurately classify and diagnose chest X-ray images. This work underscores the potential of deep learning in aiding medical professionals in diagnosing diseases and conditions through image analysis.
Zhang et al. ( 2020 ) offered a novel solution to the challenge of distinguishing between genuine and counterfeit facial images generated using deep learning methods. Their approach relied on a Counterfeit Feature Extraction Method that employed a Convolutional Neural Network (CNN) model. This model demonstrated remarkable accuracy, achieving a rate of 97.6%. Beyond the impressive accuracy, the study also addressed a crucial aspect of computational efficiency, highlighting the potential for reducing the computational demands associated with counterfeit image detection. This research is particularly relevant in today's digital landscape where ensuring the authenticity of images has become increasingly vital.
Simon and V et al. ( 2020 ) explored the fusion of deep learning and feature extraction in the context of image classification and texture analysis. Their study involved Convolutional Neural Networks (CNNs) including popular architectures like AlexNet, VGG19, Inception, InceptionResNetV3, ResNet, and DenseNet201. These architectures were employed to extract meaningful features from images, which were then fed into a Support Vector Machine (SVM) for texture classification. The results were promising, with the model achieving good to superior accuracy levels ranging from 85 to 95% across different pretrained models and datasets. This approach showcases the ability of deep learning to contribute to image analysis tasks, particularly when combined with traditional machine learning techniques.
Sungheetha and Sharma et al. ( 2021 ) addressed the critical challenge of detecting diabetic conditions through the identification of specific signs within blood vessels of the eye. Their approach relied on a deep feature Convolutional Neural Network (CNN) designed to spot these indicators. With an impressive accuracy of 97%, the model demonstrated its efficacy in accurately identifying diabetic conditions. This work not only showcases the potential of deep learning in medical diagnostics but also highlights its ability to capture intricate visual patterns that are indicative of specific health conditions.
Devulapalli et al. ( 2021 ) proposed a hybrid feature extraction method that combined Gabor transform-based texture features with automated high-level features using the Googlenet architecture. By utilizing pre-trained models such as Alexnet, VGG 16, and Googlenet, the study achieved exceptional accuracy levels. Interestingly, the hybrid feature extraction method outperformed the existing pre-trained models, underscoring the potential of combining different feature extraction techniques to achieve superior performance in image analysis tasks. Shankar et al. ( 2022 ) embarked on the critical task of COVID-19 diagnosis using chest X-ray images. Their approach involved a multi-step process that encompassed preprocessing through Weiner filtering, fusion-based feature extraction using GLCM, GLRM, and LBP, and finally, classification through an Artificial Neural Network (ANN). By carefully selecting optimal feature subsets, the model exhibited the potential for robust classification between infected and healthy patients. This study showcases the versatility of deep learning in medical diagnostics, particularly in addressing urgent global health challenges.
Ahmad et al. ( 2022 ) made significant strides in breast cancer detection by introducing a hybrid deep learning model, AlexNet-GRU, capable of autonomously extracting features from the PatchCamelyon benchmark dataset. The model demonstrated its prowess in accurately identifying metastatic cancer in breast tissue. With superior performance compared to state-of-the-art methods, this research emphasizes the potential of deep learning in medical imaging, specifically for cancer detection and classification. Sharif et al. ( 2019 ) ventured into the complex field of detecting gastrointestinal tract (GIT) infections using wireless capsule endoscopy (WCE) images. Their innovative approach combined deep convolutional (CNN) and geometric features to address the intricate challenges posed by lesion attributes. The fusion of contrast-enhanced color features and geometric characteristics led to exceptional classification accuracy and precision, showcasing the synergy between deep learning and traditional geometric features. This approach is particularly promising in enhancing medical diagnostics through the integration of multiple information sources.
Aarthi and Rishma ( 2023 ) responded to the pressing challenges of waste management by introducing a real-time automated waste detection and segregation system using deep learning. Leveraging the Mask R-CNN architecture, their model demonstrated the capability to identify and classify waste objects in real time. Additionally, the study explored the extraction of geometric features for more effective object manipulation by robotic arms. This innovative approach not only addresses environmental concerns related to waste but also showcases the potential of deep learning in practical applications beyond traditional image analysis, with the aim of enhancing efficiency and reducing pollution risks.
These studies showcase the efficacy of methods like CNNs, hybrid approaches, and novel architectures in achieving high accuracies and improved performance metrics in applications such as disease identification, image analysis, counterfeit detection, and more. While these methods automate the extraction of meaningful features, they also encounter challenges like computational complexity, dataset quality, and real-world variability, which should be carefully considered in their practical implementation.
6 Image classification
Image classification is a fundamental task in computer vision that involves categorizing images into predefined classes or labels. The goal is to enable machines to recognize and differentiate objects, scenes, or patterns within images.
Traditional classification is a fundamental data analysis technique that involves categorizing data points into specific classes or categories based on predetermined rules and established features. Before the advent of deep learning, several conventional methods were widely used for this purpose, including Decision Trees, Support Vector Machines (SVM), Naive Bayes, and k-Nearest Neighbors (k-NN). In the realm of traditional classification, experts would carefully design and select features that encapsulate relevant information from the data. These features are typically chosen based on domain knowledge and insights, aiming to capture distinguishing characteristics that help discriminate between different classes. While effective in various scenarios, traditional classification methods often require manual feature engineering, which can be time-consuming and may not fully capture intricate patterns and relationships present in complex datasets. These selected features act as inputs for classification algorithms, which utilize predefined criteria to assign data points to specific classes. Table 5 provides a compact overview of strengths and limitations in the realm of image classification by examining various deep learning models.
In the realm of medical image analysis, Sarah Ali et al. (Ismael et al. 2020 ) introduced an advanced approach that harnesses the power of Residual Networks (ResNets) for brain tumor classification. Their study involved a comprehensive evaluation on a benchmark dataset comprising 3064 MRI images of three distinct brain tumor types. Impressively, their model achieved a remarkable accuracy of 99%, surpassing previous works in the same domain. Shifting focus to the domain of remote sensing, Xiaowei et al. ( 2020 ) embarked on a deep learning journey for remote sensing image classification. Their methodology combined Recurrent Neural Networks (RNN) with Random Forest, aiming to optimize cross-validation on the UC Merced dataset. Through rigorous experimentation and comparison with various deep learning techniques, their approach achieved a commendable accuracy of 87%.
Texture analysis and classification hold significant implications, as highlighted by Aggarwal and Kuma ( 2020 ). Their study introduced a novel deep learning-based model, centered around Convolution Neural Networks (CNN), specifically composed of two sub-models. The outcomes were noteworthy, with model-1 achieving an accuracy of 92.42%, while model-2 further improved the accuracy to an impressive 96.36%.
Abdar et al. ( 2021 ) unveiled a pioneering hybrid dynamic Bayesian Deep Learning (BDL) model that leveraged the Three-Way Decision (TWD) theory for skin cancer diagnosis. By incorporating different uncertainty quantification (UQ) methods and deep neural networks within distinct classification phases, they attained substantial accuracy and F1-score percentages on two skin cancer datasets.
The landscape of medical diagnostics saw another stride forward with Ibrahim et al. ( 2021 ), who explored a deep learning approach based on a pretrained AlexNet model for classifying COVID-19, pneumonia, and healthy CXR scans. Their model exhibited notable performance in both three-way and four-way classifications, achieving high accuracy, sensitivity, and specificity percentages.
In the realm of image classification under resource constraints, Ma et al. ( 2022 ) introduced a novel deep CNN classification method with knowledge transfer. This method showcased superior performance compared to traditional histogram-based techniques, achieving an impressive classification accuracy of 93.4%.
Diving into agricultural applications, Gill et al. ( 2022 ) devised a hybrid CNN-RNN approach for fruit classification. Their model demonstrated remarkable efficiency and accuracy in classifying fruits, showcasing its potential for aiding in quality assessment and sorting.
Abu-Jamie et al. et al. ( 2022 ) turned their attention to fruit classification as well, utilizing a deep learning-based approach. By employing CNN Model VGG16, they managed to achieve a remarkable 100% accuracy, underscoring the potential of such methodologies in real-world applications.
Medical imaging remained a prominent field of exploration, as Sharma et al. ( 2022 ) explored breast cancer diagnosis through Convolutional Neural Networks (CNN) with transfer learning. Their study showcased a promising accuracy of 98.4%, reinforcing the potential of deep learning in augmenting medical diagnostics.
Beyond the realm of medical imagery, Yang et al. ( 2022 ) applied diverse CNN models to an urban wetland identification framework, with DenseNet121 emerging as the top-performing model. The achieved high Kappa and OA values underscore the significance of deep learning in land cover classification.
Hussain et al. ( 2020 ) delved into Alzheimer's disease detection using a 12-layer CNN model. Their approach showcased a remarkable accuracy of 97.75%, surpassing existing CNN models on the OASIS dataset. Their study also provided a head-to-head comparison with pre-trained CNNs, solidifying the efficacy of their proposed approach in enhancing Alzheimer's disease detection.
In the textile industry, Gao et al. ( 2019 ) addressed fabric defect detection using deep learning. Their novel approach, involving a convolutional neural network with multi-convolution and max-pooling layers, showcased promising results with an overall detection accuracy of 96.52%, offering potential implications for real-world practical applications.
Expanding the horizon to neurological disorders, Vikas et al. study ( 2021 ) pioneered ADHD classification from resting-state functional MRI (rs-fMRI) data. Employing a hybrid 2D CNN–LSTM model, the study achieved remarkable improvements in accuracy, specificity, sensitivity, F1-score, and AUC when compared to existing methods. The integration of deep learning with rs-fMRI holds the promise of a robust model for effective ADHD diagnosis and differentiation from healthy controls.
Skouta et al. ( 2021 ) work focused on retinal image classification. By harnessing the capabilities of convolutional neural networks (CNNs), their approach achieved an impressive classification accuracy of 95.5% for distinguishing between normal and proliferative diabetic retinas. The inclusion of an expanded dataset contributed to capturing intricate features and ensuring accurate classification outcomes. These studies collectively illuminate the transformative influence of deep learning techniques across diverse classification tasks, spanning medical diagnoses, texture analysis, image categorization, and neurological disorder identification.
While traditional methods have their merits, they heavily rely on domain expertise for feature selection and algorithm tuning. However, these traditional classification approaches encounter limitations. They might struggle with complex and high-dimensional data, where identifying important features becomes intricate. Additionally, they demand substantial manual effort in feature engineering, making them less adaptable to evolving data distributions or novel data types. The emergence of deep learning has revolutionized classification by automating the process of feature extraction. Deep neural networks directly learn hierarchical representations from raw data, eliminating the need for manually crafted features. This enables them to capture intricate patterns and relationships that traditional methods might miss. Notably, Convolutional Neural Networks (CNNs) have excelled in image classification tasks, while Recurrent Neural Networks (RNNs) demonstrate proficiency in handling sequential data. These deep learning models often surpass traditional methods in tackling complex tasks across various domains.
7 Discussion
Among the deep learning model for image denoising, Self2Self NN for cost reduction with data augmentation dependency, Denoising CNNs enhancing accuracy but facing resource challenges, and DFT-Net managing image label imbalance while risking detail loss. Robustness and hyperparameter tuning characterize MPR-CNN, while R2R noise reduction balances results and computational demands. CNN architectures prevent overfitting in denoising, and HLF-DIP achieves high values despite complexity. (Noise 2Noise) models exhibit efficiency and generalization trade-offs, and ConvNet enhances receptive fields while grappling with interpretability. This collection offers insights into the evolving landscape of image processing techniques.
This compilation of studies showcases a variety of image enhancement techniques. Ming Liu et al. employ Fuzzy-CNN and F-Capsule for iris recognition, ensuring robustness and avoiding overfitting. Khairul Munadi combines various methods with EfficientNet and ResNets for tuberculosis image enhancement, enhancing generalization while facing time and memory challenges. Ching Ta Lu employs FCNN mean filters for noise reduction, addressing noise while considering potential detail loss. Yuhui Quan implements CV-CNN for image deblurring, providing an efficient model with overfitting prevention. Dan Jin employs pix2pixHD for high-quality MDCT image enhancement, achieving quality improvement with possible overfitting concerns. Guofa Li introduces LE-net for low-light image recovery, emphasizing generalization and robustness with real-world limitations. Xianjie Gao introduces RetinexDIP for image enhancement, offering faster convergence and reduced runtime, despite challenges in complex scenes. Kiyeon Kim unveils MSSNet-WS for single image deblurring, prioritizing computational efficiency in real-world scenarios.
This compilation of research papers presents a comprehensive exploration of deep learning methodologies applied to two prominent types of image segmentation: semantic segmentation and instance segmentation. In the realm of semantic segmentation, studies utilize architectures like FCN, U-Net, and DeepLabV3 for tasks such as efficient detection of multiple persons and robust object recognition in varying lighting and background conditions. These approaches achieve notable performance metrics, with IoU and mIoU ranging from 80 to 86%. Meanwhile, in the context of instance segmentation, methods like Mask-RCNN and AFD-UNet are employed to precisely delineate individual object instances within an image, contributing to efficient real-time waste collection, accurate medical image interpretation, and more. The papers highlight the benefits of these techniques, including enhanced boundary delineation, reduced manual intervention, and substantial time savings, while acknowledging challenges such as computational complexity, model customization, and hardware limitations. This compilation provides a comprehensive understanding of the strengths and challenges of deep learning-based semantic and instance segmentation techniques across diverse application domains.
This review explores deep learning methodologies tailored to different types of image feature extraction across varied application domains. Texture/color-based approaches encompass studies like Aurangzeb Magsi et al.’s disease classification achieving 89.4% ACC, and Weiguo Zhang’s counterfeit detection at 97% accuracy. Pattern-based analysis includes Akey Sungheetha’s 97% class score for retinal images, K. Shankar et al.'s 95.1%-95.7% accuracy using FM-ANN, GLCM, GLRM, and LBP for chest X-rays, and Shahab Ahmad's 99.5% accuracy with AlexNet-GRU for PCam images. Geometric feature extraction is demonstrated by Sharif, Muhammad with 99.4% accuracy in capsule endoscopy images and Aarthi.R et al. achieving 97% accuracy in real-time waste image analysis using MRCNN. This comprehensive review showcases deep learning's adaptability in extracting diverse image features for various applications.
This compilation of research endeavors showcases diverse deep learning models applied to distinct types of image classification tasks. For multiclass classification, studies like Sarah Ali et al.'s employment of Residual Networks attains 99% accuracy in MRI image classification, while Akarsh Aggarwal et al.'s CNN approach achieves 92.42% accuracy in Kylberg Texture datasets. Abdullahi Umar Ibrahim's utilization of an AlexNet model records a 94% accuracy rate for lung conditions. In multiclass scenarios, Harmandeep Singh Gill's hybrid CNN-RNN attains impressive results in fruit classification, and Tanseem N et al. achieve 100% accuracy with VGG16 on fruit datasets. For binary classification, Emtiaz Hussain et al.'s CNN achieves 97.75% accuracy in OASIS MRI data, while Can Gao et al. achieve 96.52% accuracy in defect detection for fabric images. Vikas Khullar et al.'s CNN-LSTM hybrid records 95.32% accuracy for ADHD diagnosis, and Ayoub Skouta's CNN demonstrates 95.5% accuracy in diabetic retinopathy detection. These studies collectively illustrate the efficacy and adaptability of deep learning techniques across various types of classification tasks while acknowledging challenges such as dataset biases, computational intensity, and interpretability.
8 Conclusions
This comprehensive review paper embarks on an extensive exploration across the diverse domains of image denoising, enhancement, segmentation, feature extraction, and classification. By meticulously analyzing and comparing these methodologies, it offers a panoramic view of the contemporary landscape of image processing. In addition to highlighting the unique strengths of each technique, the review shines a spotlight on the challenges that come hand in hand with their implementation.
In the realm of image denoising, the efficacy of methods like Self2Self NN, DnCNNs, and DFT-Net is evident in noise reduction, although challenges such as detail loss and hyperparameter optimization persist. Transitioning to image enhancement, strategies like Novel RetinexDIP, Unsharp Masking, and LE-net excel in enhancing visual quality but face complexities in handling intricate scenes and maintaining image authenticity.
Segmentation techniques span the gamut from foundational models to advanced ones, providing precise object isolation. Yet, challenges arise in scenarios with overlapping objects and the need for robustness. Feature extraction methodologies encompass a range from CNNs to LSTM-augmented CNNs, unveiling crucial image characteristics while requiring careful consideration of factors like efficiency and adaptability.
Within classification, Residual Networks to CNN-LSTM architectures showcase potential for accurate categorization. However, data dependency, computational complexity, and model interpretability remain as challenges. The review's contributions extend to the broader image processing field, providing a nuanced understanding of each methodology's traits and limitations. By offering such insights, it empowers researchers to make informed decisions regarding technique selection for specific applications. As the field evolves, addressing challenges like computation demands and interpretability will be pivotal to fully realize the potential of these methodologies.
The scope of papers discussed in this review offers a panorama of DL methodologies that traverse diverse application domains. These domains encompass medical and satellite imagery, botanical studies featuring flower and fruit images, as well as real-time scenarios. The tailored DL approaches for each domain underscore the adaptability and efficacy of these methods across multifaceted real-world contexts.
Aarthi R, Rishma G (2023) A Vision based approach to localize waste objects and geometric features exaction for robotic manipulation. Int Conf Mach Learn Data Eng Procedia Comput Sci 218:1342–1352. https://doi.org/10.1016/j.procs.2023.01.113
Article Google Scholar
Abdar M, Samami M, Mahmoodabad SD, Doan T, Mazoure B, Hashemifesharaki R, Liu L, Khosravi A, Acharya UR, Makarenkov V, Nahavandi S (2021) Uncertainty quantification in skin cancer classification using three-way decision-based Bayesian deep learning. Comput Biol Med 135:104418. https://doi.org/10.1016/j.compbiomed.2021.104418
Aggarwal A, Kuma M (2020) Image surface texture analysis and classification using deep learning. Multimed Tools Appl 80(1):1289–1309. https://doi.org/10.1007/s11042-020-09520-2
Ahammad SH, Rajesh V, Rahman MZU, Lay-Ekuakille A (2020) A hybrid CNN-based segmentation and boosting classifier for real time sensor spinal cord injury data. IEEE Sens J 20(17):10092–10101. https://doi.org/10.1109/jsen.2020.2992879
Ahmad S, Ullah T, Ahmad I, Al-Sharabi A, Ullah K, Khan RA, Rasheed S, Ullah I, Uddin MN, Ali MS (2022) A novel hybrid deep learning model for metastatic cancer detection". Comput Intell Neurosci 2022:14. https://doi.org/10.1155/2022/8141530
Ahmed I, Ahmad M, Khan FA, Asif M (2020) Comparison of deep-learning-based segmentation models: using top view person images”. IEEE Access 8:136361–136373. https://doi.org/10.1109/access.2020.3011406
Aish MA, Abu-Naser SS, Abu-Jamie TN (2022) Classification of pepper using deep learning. Int J Acad Eng Res (IJAER) 6(1):24–31.
Google Scholar
Ashraf H, Waris A, Ghafoor MF et al (2022) Melanoma segmentation using deep learning with test-time augmentations and conditional random fields. Sci Rep 12:3948. https://doi.org/10.1038/s41598-022-07885-y
Bouteldja N, Klinkhammer BM, Bülow RD et al (2020) Deep learning based segmentation and quantification in experimental kidney histopathology. J Am Soc Nephrol. https://doi.org/10.1681/ASN.2020050597
Cheng G, Xie X, Han J, Guo L, Xia G-S (2020) Remote sensing image scene classification meets deep learning: challenges, methods, benchmarks, and opportunities. IEEE J Select Topics Appl Earth Observ Remote Sens 13:3735–3756. https://doi.org/10.1109/JSTARS.2020.3005403
Devulapalli S, Potti A, Rajakumar Krishnan M, Khan S (2021) Experimental evaluation of unsupervised image retrieval application using hybrid feature extraction by integrating deep learning and handcrafted techniques. Mater Today: Proceed 81:983–988. https://doi.org/10.1016/j.matpr.2021.04.326
Dey S, Bhattacharya R, Malakar S, Schwenker F, Sarkar R (2022) CovidConvLSTM: a fuzzy ensemble model for COVID-19 detection from chest X-rays. Exp Syst Appl 206:117812. https://doi.org/10.1016/j.eswa.2022.117812
Gao C, Zhou J, Wong WK, Gao T (2019) Woven Fabric Defect Detection Based on Convolutional Neural Network for Binary Classification. In: Wong W (ed) Artificial Intelligence on Fashion and Textiles AITA 2018 Advances in Intelligent Systems and Computing. Springer, Cham. https://doi.org/10.1007/978-3-319-99695-0_37
Chapter Google Scholar
Gao X, Zhang M, Luo J (2022) Low-light image enhancement via retinex-style decomposition of denoised deep image prior. Sensors 22:5593. https://doi.org/10.3390/s22155593
Gill HS, Murugesan G, Mehbodniya A, Sajja GS, Gupta G, Bhatt A (2023) Fruit Type Classification using Deep Learning and Feature Fusion. Comput Electronic Agric 211:107990 https://doi.org/10.1016/j.compag.2023.107990
Gite S, Mishra A, Kotecha K (2022) Enhanced lung image segmentation using deep learning. Neural Comput and Appl. https://doi.org/10.1007/s00521-021-06719-8
Hasti VR, Shin D (2022) Denoising and fuel spray droplet detection from light-scattered images using deep learning. Energy and AI 7:100130. https://doi.org/10.1016/j.egyai.2021.100130
Hedayati R, Khedmati M, Taghipour-Gorjikolaie M (2021) Deep feature extraction method based on ensemble of convolutional auto encoders: Application to Alzheimer’s disease diagnosis. Biomed Signal Process Control 66:102397. https://doi.org/10.1016/j.bspc.2020.102397
Hussain E, Hasan M, Hassan SZ, Azmi TH, Rahman MA, Parvez MZ (2020) [IEEE 2020 15th IEEE Conference on Industrial Electronics and Applications (ICIEA) - Kristiansand, Norway (2020.11.9–2020.11.13)] 2020 15th IEEE Conference on Industrial Electronics and Applications (ICIEA) - Deep Learning Based Binary Classification for Alzheimerâ™s Disease Detection using Brain MRI Images. pp. 1115–1120. https://doi.org/10.1109/iciea48937.2020.9248213
Ibrahim AU, Ozsoz M, Serte S, Al-Turjman F, Yakoi PS (2021) Pneumonia Classifcation Using Deep Learning from Chest X ray Images During COVID 19. Cognitive Computation. Springer, Berlin. https://doi.org/10.1007/s12559-020-09787-5
Ismael SAA, Mohammed A, Hefny H (2020) An enhanced deep learning approach for brain cancer MRI images classification using residual networks. Artif Intell Med 102:101779. https://doi.org/10.1016/j.artmed.2019.101779
Jalali Y, Fateh M, Rezvani M, Abolghasemi V, Anisi MH (2021) ResBCDU-Net: a deep learning framework for lung CT image segmentation. Sensors. https://doi.org/10.3390/s21010268
Jiang X, Zhu Y, Zheng B et al (2021) Images denoising for COVID-19 chest X-ray based on multi-resolution parallel residual CNN. July 2021 Machine Vision and Applications 32(4). https://doi.org/10.1007/s00138-021-01224-3
Jin D, Zheng H, Zhao Q, Wang C, Zhang M, Yuan H (2021) Generation of vertebra micro-CT-like image from MDCT: a deep-learning-based image enhancement approach. Tomography 7:767–782. https://doi.org/10.3390/tomography7040064
Kasongo SM, Sun Y (2020) A deep learning method with wrapper based feature extraction for wireless intrusion detection system. Comput Secur 92:101752. https://doi.org/10.1016/j.cose.2020.101752
Khullar V, Salgotra K, Singh HP, Sharma DP (2021) Deep learning-based binary classification of ADHD using resting state MR images. Augment Hum Res. https://doi.org/10.1007/s41133-020-00042-y
Kim K, Lee S, Cho S (2023) MSSNet: Multi-Scale-Stage Network for Single Image Deblurring. In: Karlinsky, L., Michaeli, T., Nishino, K. (eds) Computer Vision – ECCV 2022 Workshops. ECCV 2022. Lecture Notes in Computer Science, vol 13802. Springer, Cham. https://doi.org/10.1007/978-3-031-25063-7_32
Kim B, Ye JC (2019) Mumford-Shah Loss functional for image segmentation with deep learning. IEEE Trans Image Process. https://doi.org/10.1109/TIP.2019.2941265
Kong Y, Ma X, Wen C (2022) A new method of deep convolutional neural network image classification based on knowledge transfer in small label sample environment. Sensors 22:898. https://doi.org/10.3390/s22030898
Li G, Yang Y, Xingda Q, Cao D, Li K (2021a) A deep learning based image enhancement approach for autonomous driving at night. Knowl-Based Syst 213:106617. https://doi.org/10.1016/j.knosys.2020.106617
Li W, Raj ANJ, Tjahjadi T, Zhuang Z (2021b) Digital hair removal by deep learning for skin lesion segmentation”. Pattern Recog 117:107994. https://doi.org/10.1016/j.patcog.2021.107994
Liu M, Zhou Z, Shang P, Xu D (2019) Fuzzified image enhancement for deep learning in iris recognition”. IEEE Trans Fuzzy Syst 2019:2912576. https://doi.org/10.1109/TFUZZ.2019.2912576
Liu D, Wen B, Jiao J, Liu X, Wang Z, Huang TS (2020) Connecting image denoising and high-level vision tasks via deep learning. IEEE Trans Image Process 29:3695–3706. https://doi.org/10.1109/TIP.2020.2964518
Liu L, Tsui YY, Mandal M (2021) Skin lesion segmentation using deep learning with auxiliary task. J Imag 7:67. https://doi.org/10.3390/jimaging7040067
Lorenzoni R, Curosu I, Paciornik S, Mechtcherine V, Oppermann M, Silva F (2020) Semantic segmentation of the micro-structure of strain-hardening cement-based composites (SHCC) by applying deep learning on micro-computed tomography scans. Cement Concrete Compos 108:103551. https://doi.org/10.1016/j.cemconcomp.2020.103551
Lu CT, Wang LL, Shen JH et al (2021) Image enhancement using deep-learning fully connected neural network mean filter. J Supercomput 77:3144–3164. https://doi.org/10.1007/s11227-020-03389-6
Ma S, Li L, Zhang C (2022) Adaptive Image denoising method based on diffusion equation and deep learning”. Internet of Robotic Things-Enabled Edge Intelligence Cognition for Humanoid Robots Volume 2022 | Article ID 7115551. https://doi.org/10.1155/2022/7115551
Magsi A, Mahar JA, Razzaq MA, Gill SH (2020) Date Palm Disease Identification Using Features Extraction and Deep Learning Approach. 2020 IEEE 23rd International Multitopic Conference (INMIC). https://doi.org/10.1109/INMIC50486.2020.9318158
Mahajan K, Garg U, Shabaz M (2021) CPIDM: a clustering-based profound iterating deep learning model for HSI segmentation Hindawi. Wireless Commun Mobile Comput 2021:12. https://doi.org/10.1155/2021/7279260
Mahmoudi O, Wahab A, Chong KT (2020) iMethyl-deep: N6 methyladenosine identification of yeast genome with automatic feature extraction technique by using deep learning algorithm. Genes 2020, 11(5), 529; https://doi.org/10.3390/genes11050529
Mehranian A, Wollenweber SD, Walker MD et al (2022) Deep learning–based time-of-flight (ToF) image enhancement of non-ToF PET scans. Eur J Nucl Med Mol Imag 49:3740–3749. https://doi.org/10.1007/s00259-022-05824-7
Meng Y, Zhang J (2022) A novel gray image denoising method using convolutional neural network”. IEEE Access 10:49657–49676 https://doi.org/10.1007/s00259-022-05824-7
Munadi K, Muchtar K, Maulina N (2020) And Biswajeet Pradhan”, image enhancement for tuberculosis detection using deep learning. IEEE Access 8:217897. https://doi.org/10.1109/ACCESS.2020.3041867
Niresi FK, Chi C-Y (2022) Unsupervised hyperspectral denoising based on deep image prior and least favorable distribution”. IEEE Journal of Selected Topics in Applied Earth Observations and Remote Sensing vol. 15, pp. 5967-5983, 2022. https://doi.org/10.1109/JSTARS.2022.3187722
Nurmaini S, Rachmatullah MN, Sapitri AI, Darmawahyuni A, Jovandy A, Firdaus F, Tutuko B, Passarella R (2020) Accurate detection of septal defects with fetal ultrasonography images using deep learning-based multiclass instance segmentation. IEEE Access 8:196160–196174. https://doi.org/10.1109/ACCESS.2020.3034367
Pang T, Zheng H, Quan Y, Ji H (2021) Recorrupted-to-Recorrupted: Unsupervised Deep Learning for Image Denoising” IEEE/CVF Conference on Computer Vision and Pattern Recognition (CVPR). https://doi.org/10.1109/CVPR46437.2021.00208
Park KH, Batbaatar E, Piao Y, Theera-Umpon N, Ryu KH (2021b) Deep learning feature extraction approach for hematopoietic cancer subtype classification. Int J Environ Res Public Health 18:2197. https://doi.org/10.3390/ijerph18042197
Park D, Lee J, Lee J, Lee K (2021) Deep Learning based Food Instance Segmentation using Synthetic Data, IEEE, 18th International Conference on Ubiquitous Robots (UR). https://doi.org/10.1109/UR52253.2021.9494704
Peng Z, Peng S, Lidan Fu, Binchun Lu, Tanga J, Wang Ke, Wenyuan Li, (2020) A novel deep learning ensemble model with data denoising for short-term wind speed forecasting”. Energy Convers Manag 207:112524. https://doi.org/10.1016/j.enconman.2020.112524
Pérez-Borrero I, Marín-Santos D, Gegúndez-Arias ME, Cortés-Ancos E (2020) A fast and accurate deep learning method for strawberry instance segmentation. Comput Electron Agric 178:105736. https://doi.org/10.1016/j.compag.2020.105736
Picon A, San-Emeterio MG, Bereciartua-Perez A, Klukas C, Eggers T, Navarra-Mestre R (2022) Deep learning-based segmentation of multiple species of weeds and corn crop using synthetic and real image datasets. Comput Electron Agric 194:10671. https://doi.org/10.1016/j.compag.2022.106719
Quan Y, Lin P, Yong X, Nan Y, Ji H (2021) Nonblind image deblurring via deep learning in complex field. IEEE Trans Neural Netw Learn Syst 33(10):5387–5400. https://doi.org/10.1109/TNNLS.2021.3070596
Quan, Y., Chen, M., Pang, T. and Ji, H., 2020 “Self2Self With Dropout: Learning Self-Supervised Denoising From Single Image”, IEEE 2020 IEEE/CVF Conference on Computer Vision and Pattern Recognition (CVPR) - Seattle, WA, 2020, pp. 1887–1895. https://doi.org/10.1109/CVPR42600.2020.00196
Robiul Islam Md, Nahiduzzaman Md (2022) Complex features extraction with deep learning model for the detection of COVID19 from CT scan images using ensemble based machine learning approach. Exp Syst Appl 195:116554. https://doi.org/10.1016/j.eswa.2022.116554
Saood A, Hatem I (2021) COVID-19 lung CT image segmentation using deep learning methods: U-Net versus SegNet”. BMC Med Imaging 21:19. https://doi.org/10.1186/s12880-020-00529-5
Sarki R, Ahmed K, Wang H et al (2020) Automated detection of mild and multi-class diabetic eye diseases using deep learning. Health Inf Sci Syst 8:32. https://doi.org/10.1007/s13755-020-00125-5
Shankar K, Perumal E, Tiwari P et al (2022) Deep learning and evolutionary intelligence with fusion-based feature extraction for detection of COVID-19 from chest X-ray images. Multimedia Syst 28:1175–1187. https://doi.org/10.1007/s00530-021-00800-x
Sharif M, Attique Khan M, Rashid M, Yasmin M, Afza F, Tanik UJ (2019) Deep CNN and geometric features-based gastrointestinal tract diseases detection and classification from wireless capsule endoscopy images. J Exp Theor Artif Intell 33:1–23. https://doi.org/10.1080/0952813X.2019.1572657
Sharma A, Mishra PK (2022) Image enhancement techniques on deep learning approaches for automated diagnosis of COVID-19 features using CXR images. Multimed Tools Appl 81:42649–42690. https://doi.org/10.1007/s11042-022-13486-8
Sharma T, Nair R, Gomathi S (2022) Breast cancer image classification using transfer learning and convolutional neural network. Int J Modern Res 2(1):8–16
Sharma, Harsh, Jain, Jai Sethia, Bansal, Priti, Gupta, Sumit (2020). [IEEE 2020 10th International Conference on Cloud Computing, Data Science and Engineering (Confluence) - Noida, India (2020.1.29–2020.1.31)] 2020 10th International Conference on Cloud Computing, Data Science and Engineering (Confluence) - Feature Extraction and Classification of Chest X-Ray Images Using CNN to Detect Pneumonia. pp. 227–231. https://doi.org/10.1109/Confluence47617.2020.9057809
Simon P, Uma V (2020) Deep learning based feature extraction for texture classification. Procedia Comput Sci 171:1680–1687. https://doi.org/10.1016/j.procs.2020.04.180
Skouta A, Elmoufidi A, Jai-Andaloussi S, Ochetto O (2021) Automated Binary Classification of Diabetic Retinopathy by Convolutional Neural Networks. In: Saeed F, Al-Hadhrami T, Mohammed F, Mohammed E (eds) Advances on Smart and Soft Computing, Advances in Intelligent Systems and Computing. Springer, Singapore. https://doi.org/10.1007/978-981-15-6048-4_16
Sori WJ, Feng J, Godana AW et al (2021) DFD-Net: lung cancer detection from denoised CT scan image using deep learning. Front Comput Sci 15:152701. https://doi.org/10.1007/s11704-020-9050-z
Sungheetha A, Rajesh Sharma R (2021) Design an early detection and classification for diabetic retinopathy by deep feature extraction based convolution neural network. J Trends Comput Sci Smart Technol (TCSST) 3(2):81–94. https://doi.org/10.36548/jtcsst.2021.2.002
Tang H, Zhu H, Fei L, Wang T, Cao Y, Xie C (2023) Low-Illumination image enhancement based on deep learning techniques: a brief review. Photonics 10(2):198. https://doi.org/10.3390/photonics10020198
Tanseem N. Abu-Jamie, Samy S. Abu-Naser, Mohammed A. Alkahlout, Mohammed A. Aish,“Six Fruits Classification Using Deep Learning”, International Journal of Academic Information Systems Research (IJAISR) ISSN: 2643–9026. 6(1):1–8
Tawfik MS, Adishesha AS, Hsi Y, Purswani P, Johns RT, Shokouhi P, Huang X, Karpyn ZT (2022) Comparative study of traditional and deep-learning denoising approaches for image-based petrophysical characterization of porous media. Front Water 3:800369 https://doi.org/10.3389/frwa.2021.800369
Tian C, Xu Y, Fei L, Yan K (2019) Deep Learning for Image Denoising: A Survey. In: Pan JS, Lin JW, Sui B, Tseng SP (eds) Genetic and Evolutionary Computing. ICGEC 2018. Advances in Intelligent Systems and Computing. Springer, Singapore. https://doi.org/10.48550/arXiv.1810.05052
Tian C, Fei L, Zheng W, Xu Y, Zuof W, Lin CW (2020) Deep Learning on Image Denoising: An Overview. Neural Networks 131:251-275 https://doi.org/10.1016/j.neunet.2020.07.025
Wang D, Su J, Yu H (2020) Feature Extraction and analysis of natural language processing for deep learning english language. IEEE Access 8:46335–46345. https://doi.org/10.1109/ACCESS.2020.2974101
Wang EK, Chen CM, Hassan MM, Almogren A (2020) A deep learning based medical image segmentation technique in Internet-of-Medical-Things domain. Future Gen Comput Syst 108:135–144. https://doi.org/10.1016/j.future.2020.02.054
Xiaowei Xu, Chen Y, Junfeng Zhang Y, Chen PA, Manickam A (2020) A novel approach for scene classification from remote sensing images using deep learning methods. Eur J Remote Sens 54:383–395. https://doi.org/10.1080/22797254.2020.1790995
Yan K, Chang L, Andrianakis M, Tornari V, Yu Y (2020) Deep learning-based wrapped phase denoising method for application in digital holographic speckle pattern interferometry. Appl Sci 10:4044. https://doi.org/10.3390/app10114044
Yang R, Luo F, Ren F, Huang W, Li Q, Du K, Yuan D (2022) Identifying urban wetlands through remote sensing scene classification using deep learning: a case study of Shenzhen. China ISPRS Int J Geo-Inf 11:131. https://doi.org/10.3390/ijgi11020131
Yoshimura N, Kuzuno H, Shiraishi Y, Morii M (2022) DOC-IDS: a deep learning-based method for feature extraction and anomaly detection in network traffic. Sensors 22:4405. https://doi.org/10.3390/s22124405
Zhang W, Zhao C, Li Y (2020) A novel counterfeit feature extraction technique for exposing face-swap images based on deep learning and error level analysis. Entropy 22(2):249. https://doi.org/10.3390/e22020249
Article MathSciNet Google Scholar
Zhou Y, Zhang C, Han X, Lin Y (2021) Monitoring combustion instabilities of stratified swirl flames by feature extractions of time-averaged flame images using deep learning method. Aerospace Sci Technol 109:106443. https://doi.org/10.1016/j.ast.2020.106443
Zhou X, Zhou H, Wen G, Huang X, Le Z, Zhang Z, Chen X (2022) A hybrid denoising model using deep learning and sparse representation with application in bearing weak fault diagnosis. Measurement 189:110633. https://doi.org/10.1016/j.measurement.2021.110633
Download references
Author information
Authors and affiliations.
Department of Computer Science, Bishop Heber College (Affiliated to Bharathidasan University), Tiruchirappalli, Tamil Nadu, India
R. Archana & P. S. Eliahim Jeevaraj
You can also search for this author in PubMed Google Scholar
Contributions
All authors reviewed the manuscript.
Corresponding author
Correspondence to P. S. Eliahim Jeevaraj .
Ethics declarations
Conflict of interest.
The authors declare no competing interests.
Additional information
Publisher's note.
Springer Nature remains neutral with regard to jurisdictional claims in published maps and institutional affiliations.
Rights and permissions
Springer Nature or its licensor (e.g. a society or other partner) holds exclusive rights to this article under a publishing agreement with the author(s) or other rightsholder(s); author self-archiving of the accepted manuscript version of this article is solely governed by the terms of such publishing agreement and applicable law.
Reprints and permissions
About this article
Archana, R., Jeevaraj, P.S.E. Deep learning models for digital image processing: a review. Artif Intell Rev 57 , 11 (2024). https://doi.org/10.1007/s10462-023-10631-z
Download citation
Accepted : 17 December 2023
Published : 07 January 2024
DOI : https://doi.org/10.1007/s10462-023-10631-z
Share this article
Anyone you share the following link with will be able to read this content:
Sorry, a shareable link is not currently available for this article.
Provided by the Springer Nature SharedIt content-sharing initiative
- Image processing
- Deep learning models
- Convolutional neural networks (CNN)
- Find a journal
- Publish with us
- Track your research
