

DCEtool: Efficient and Accessible Discrete Choice Experiments
What is dcetool and how to install it.
DCEtool is a R package to design, respond and analyze DCEs. DCEtool has been coded in R using the visual interface provided by the shiny package. DCEtool is available in the CRAN repository and can be easily installed typing the following code in R or RStudio.
('DCEtool') library(DCEtool) |
Before running the previous code, shiny must be installed and activated:
('shiny') library('shiny') |
Once shiny and DCEtool are installed and activated, the visual interface of DCEtool can be launched running the following code:
() |
How to use it
Design settings.
Next, you can decide whether to use the bayesian approach (learn more about it in Sándor & Wedel (2001) ). Then, save the inputs using the button ‘Save settings’. The prior coefficients must come from a previous DCE with the same characteristics (if there is no pilot experiment, use the default vector of zeros - learn more in the introduction section of this article ). Then, you can ‘Save options’ and move to the ‘Design matrix’ tab.
Design matrix
Experimentation.
Only when the final text is displayed, you can close the survey pop-up and move to the ‘Results’ tab by clicking on the ‘Analyze the results’ button. Moving to the ‘Results’ tab in the middle of the survey may cause data loss. Once in the ‘Results’ tab, it is highly recommended to save the results. Once the file is saved, it can be loaded at any time to redo the estimations.
Found a bug? Need help? Had an idea?
Help me improve DCEtool by going to https://github.com/danielpereztr/DCEtool and opening a new issue.
Further Reading
(R Code & Data) Optimal sequential strategy to improve the precision of the estimators in a discrete choice experiment: a simulation study
R code to replicate the simulations Constant simulations: Download Non-constant simulations: Download Results Excel file with the results: Download
El papel del análisis coste-beneficios en la toma de decisiones sanitarias: Metodología para el cálculo de la disposición a pagar como estimador del valor monetario de una mejora en la salud
choicedesign 0.1.6
pip install choicedesign Copy PIP instructions
Released: Mar 7, 2024
Experimental designs for discrete choice models.
Verified details
Maintainers.
Unverified details
- Author: José Ignacio Hernández
- Requires: Python >=3.8, <4.0
Classifiers
- Python :: 3
- Python :: 3.8
- Python :: 3.9
- Python :: 3.10
Project description
Choicedesign.
ChoiceDesign is a Python package tool to construct D-efficient designs for Discrete Choice Experiments. ChoiceDesign combines enough flexibility to construct from simple 2-alternative designs with few attributes, to more complex settings that may involve conditions between attributes. ChoiceDesign is a revamped version of EDT , a project I created some years ago for the same purpose. ChoiceDesign includes improvements over EDT such as class-based syntax, coding improvements, better documentation and making this package available to install via pip .
NEW: ChoiceDesign now integrates Biogeme , which allows you to customise the utility functions.
Installation
ChoiceDesign is available to install via the regular syntax of pip :
- python3 -m pip install choicedesign
The main features of ChoiceDesign are:
- D-efficient designs based on a random swapping algorithm
- Customisable utility functions (powered by Biogeme )
- Bayesian priors (experimental)
- Designs with conditions over different attribute levels
- Designs with blocks.
- Multiple stopping criteria (Fixed number of iterations, iterations without improvement or fixed time).
I provide some Jupyter notebooks that illustrate the use of ChoiceDesign in the examples/ folder of this repo.
How to contribute?
Any contributions to ChoiceDesign are welcome via this Git, or to my email joseignaciohernandezh at gmail dot com.
This software is provided for free and as it is, say with no warranty , and neither me nor my current institution is liable of any consequence of the use of it. In any case, integrity checks have been performed by comparing results with alternative software.
- Bierlaire, M. (2003). BIOGEME: A free package for the estimation of discrete choice models. In Swiss transport research conference .
- Kuhfeld, W. F. (2005). Experimental design, efficiency, coding, and choice designs. Marketing research methods in SAS: Experimental design, choice, conjoint, and graphical techniques , 47-97.
- Quan, W., Rose, J. M., Collins, A. T., & Bliemer, M. C. (2011). A comparison of algorithms for generating efficient choice experiments.
Project details
Release history release notifications | rss feed.
Mar 7, 2024
Dec 20, 2023
Oct 3, 2023
Nov 27, 2022
Nov 6, 2022
Download files
Download the file for your platform. If you're not sure which to choose, learn more about installing packages .
Source Distribution
Uploaded Mar 7, 2024 Source
Built Distribution
Uploaded Mar 7, 2024 Python 3
Hashes for choicedesign-0.1.6.tar.gz
Algorithm | Hash digest | |
---|---|---|
SHA256 | ||
MD5 | ||
BLAKE2b-256 |
Hashes for choicedesign-0.1.6-py3-none-any.whl
Algorithm | Hash digest | |
---|---|---|
SHA256 | ||
MD5 | ||
BLAKE2b-256 |
- português (Brasil)
Supported by
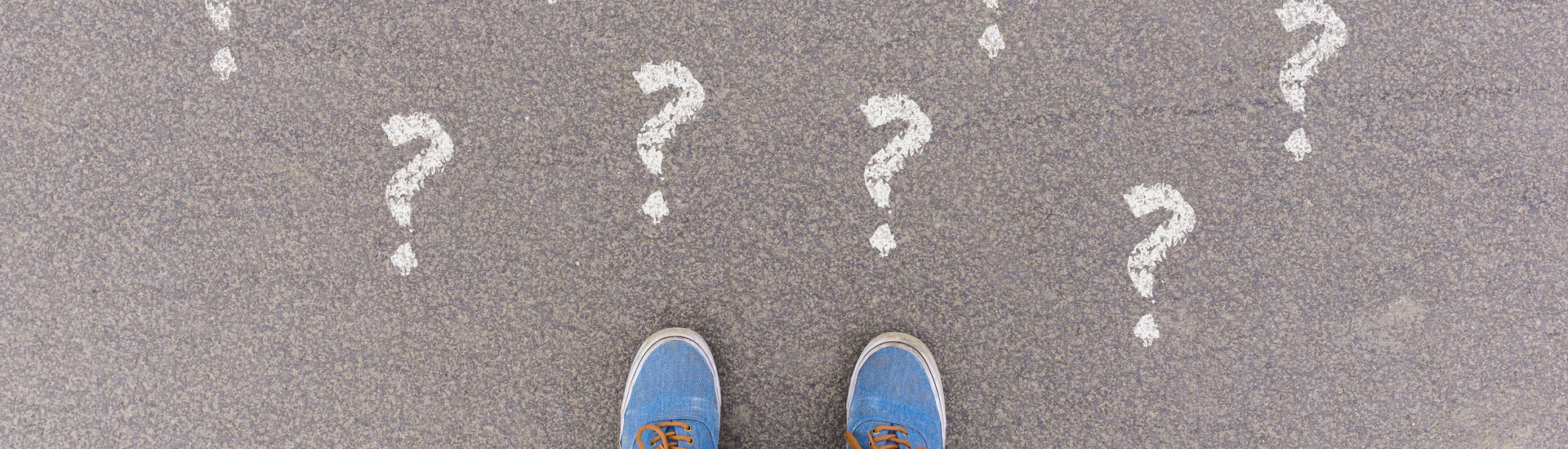
New software for the design of robust stated choice experiments
TU Delft researcher Sander van Cranenburgh, in collaboration with researchers from the University of Sydney, has developed an upgrade for leading software package Ngene. Ngene is used by researchers and professionals to design stated choice experiments. Such experiments are used to gain insight into how people weigh up various aspects when making a choice, for example travel time and travel costs. This software upgrade makes it possible to design statistically accurate choice experiments for so-called regret models, a choice behaviour model developed at Delft.
Choice experiments and behaviour models
‘Choice experiments are widely used in all manner of disciplines to help us to better understand and predict behaviour’, says Sander van Cranenburgh. ‘In a stated choice experiment the respondent is presented with various fictitious choice situations and asked to state which is the most attractive alternative.’ For example: ‘Would you rather take the intercity train to your destination and get there in 90 minutes, or take the high speed train which only takes an hour but costs an extra 10 euros?’. Researchers use special software to create their choice experiments.
Utility model
Researchers setting up choice experiments base these on a behaviour model that describes how people make choices. Until recently that model was always the utility model, a model that assumes that people choose the alternative that will give them the maximum utility (benefit or value). However, this utility model is not always realistic. An alternative behaviour model, developed by Professor Caspar Chorus at TU Delft, assumes that people make choices determined on their urge to minimise regret after the event as far as possible. This regret model regularly proves to be more reliable than the utility model, although this varies according to the context.
In his earlier research, Sander van Cranenburgh showed that choice experiments created for utility models often do not work well with regret models, and vice versa. That is why he advocates creating robust experiments that work well for more than one behaviour model, such as the regret model and the utility model. To make it easier for researchers to create robust choice experiments, Van Cranenburgh has developed an upgrade for the well-known Ngene software package. The latest version of Ngene, containing this upgrade, will be available this month. An article by Van Cranenburgh on this subject is published in the Journal of Choice Modelling .
More information
Sander van Cranenburg, [email protected] , +31(0)15-2786957 Inge Snijder, Science Communication Advisor TU Delft, [email protected] , +31 (0)15-2787538 Publication: New software tools for creating stated choice experimental designs efficient for regret minimisation and utility maximisation decision rules - Journal of Choice Modelling DOI: https://doi.org/10.1016/j.jocm.2019.04.002
Share this page:
Discrete Choice Experiments: A Guide to Model Specification, Estimation and Software
- Practical Application
- Published: 03 April 2017
- Volume 35 , pages 697–716, ( 2017 )
Cite this article
- Emily Lancsar 1 ,
- Denzil G. Fiebig 2 &
- Arne Risa Hole 3
9864 Accesses
191 Citations
10 Altmetric
Explore all metrics
We provide a user guide on the analysis of data (including best–worst and best–best data) generated from discrete-choice experiments (DCEs), comprising a theoretical review of the main choice models followed by practical advice on estimation and post-estimation. We also provide a review of standard software. In providing this guide, we endeavour to not only provide guidance on choice modelling but to do so in a way that provides a ‘way in’ for researchers to the practicalities of data analysis. We argue that choice of modelling approach depends on the research questions, study design and constraints in terms of quality/quantity of data and that decisions made in relation to analysis of choice data are often interdependent rather than sequential. Given the core theory and estimation of choice models is common across settings, we expect the theoretical and practical content of this paper to be useful to researchers not only within but also beyond health economics.
This is a preview of subscription content, log in via an institution to check access.
Access this article
Subscribe and save.
- Get 10 units per month
- Download Article/Chapter or eBook
- 1 Unit = 1 Article or 1 Chapter
- Cancel anytime
Price includes VAT (Russian Federation)
Instant access to the full article PDF.
Rent this article via DeepDyve
Institutional subscriptions
Similar content being viewed by others
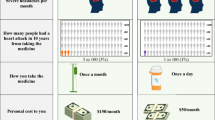
What Can Discrete-Choice Experiments Tell Us about Patient Preferences? An Introduction to Quantitative Analysis of Choice Data
How well do discrete choice experiments predict health choices a systematic review and meta-analysis of external validity, scale heterogeneity in healthcare discrete choice experiments: a primer.
This can include presenting a single profile and asking respondents to accept or reject it.
In our case study, the status quo is no treatment; however, more generally, status quo and no treatment need not coincide.
Our overview is not exhaustive, as other software packages capable of estimating some of the discrete-choice models in our review are available. However, the three packages we have reviewed are among the most commonly used for estimating these models.
This implies we will not cover software such as Gauss, Matlab and R, despite there being excellent routines written in these packages for estimating, for example, mixed logit models. A prominent example is Kenneth Train’s codes for mixed logit estimation ( http://eml.berkeley.edu/~train/software.html ), which served as inspiration for many of the routines later introduced in other statistical packages.
Two versions of Biogeme are available: BisonBiogeme and PythonBiogeme. We focus on BisonBiogeme, which is designed to estimate a range of commonly used discrete-choice models.
Nlogit also optionally allows the data to be organized in wide form, although the manual suggests that long form is typically more convenient.
Interested readers are referred to chapters 8–10 in Train [ 49 ] for more information about the issues covered in this section.
Both Nlogit and Stata will use a default set of starting values unless explicitly specified by the user, whereas Biogeme requires the user to specify the starting values.
The default number of draws is 100 in Nlogit, 50 in Stata and 150 in Biogeme.
In models with several random coefficients, alternative approaches such as shuffled or scrambled Halton draws [ 50 ] or Sobol draws [ 51 , 52 ] are sometimes used to minimize the correlation between the draws, which can be substantial for standard Halton draws in higher dimensions. See chapter 9 in Train [ 49 ] for a discussion.
Nlogit and Stata’s default starting values are the MNL parameters for the means of the random coefficients and 0 (Nlogit)/0.1 (Stata) for the standard deviations.
Differences can still arise, for example because the optimization algorithms differ in the three packages, subtle differences in terms of how the Halton draws are generated and different starting values (in this case Stata/Biogeme vs Nlogit).
Applying this procedure modifies the data from the standard set-up in Supplementary Appendix 1 to the exploded set-up in Supplementary Appendix 3.
Log-normal parameter distributions are supported by all of the packages. The negative of the log-normal can easily be implemented by multiplying the price attribute by −1 before entering the model. This is equivalent to specifying the negative of the price coefficient to be log-normally distributed. The sign of the coefficient can easily be reversed post-estimation.
One exception is when both the attribute coefficient and the negative of the price coefficient are log-normally distributed, in which case the distribution of mWTP is also log-normal.
Louviere JJ, Hensher DA, Swait JD. Stated choice methods: analysis and applications. Cambridge: Cambridge University Press; 2000.
Book Google Scholar
de Bekker-Grob EW, Ryan M, Gerard K. Discrete choice experiments in health economics: a review of the literature. Health Econ. 2012;21(2):145–72.
Article PubMed Google Scholar
Clark MD, Determann D, Petrou S, Moro D, de Bekker-Grob EW. Discrete choice experiments in health economics: a review of the literature. Pharmacoeconomics. 2014;32(9):883–902.
Viney R, Lancsar E, Louviere J. Discrete choice experiments to measure consumer preferences for health and healthcare. Expert Rev Pharmacoeconomics Outcomes Res. 2002;2(4):319–26.
Article Google Scholar
Lancsar E, Louviere J. Conducting discrete choice experiments to inform healthcare decision making. Pharmacoeconomics. 2008;26(8):661–77.
Bridges J, Hauber A, Marshall D, Lloyd A, Prosser L, Regier D, et al. A checklist for conjoint analysis applications in health: report of the ISPOR Conjoint Analysis Good Research Practices Taskforce. Value Health. 2011;14(4):403–13.
Coast J, Horrocks S. Developing attributes and levels for discrete choice experiments using qualitative methods. J Health Serv Res Policy. 2007;12(1):25–30.
Johnson FR, Lancsar E, Marshall D, Kilambi V, Mühlbacher A, Regier DA, et al. Constructing experimental designs for discrete-choice experiments: report of the ISPOR conjoint analysis experimental design good research practices task force. Value Health. 2013;16(1):3–13.
Lancsar E, Swait J. Reconceptualising the external validity of discrete choice experiments. Pharmacoeconomics. 2014;32(10):951–65.
Hauber B, Gonzalez J, Groothuis-Oudshoorn C, Prior T, Marshall D, Cunningham C, et al. Statistical methods for the analysis of discrete choie experiments: a report of the ISPOR conjinta analysis good research practice task force. Value Health. 2016;19:300–15.
Ghijben P, Lancsar E, Zavarsek S. Preferences for oral anticoagulants in atrial fibrillation: a best–best discrete choice experiment. Pharmacoeconomics. 2014;32(11):1115–27.
Dillman DA. Mail and internet surveys: the tailored design method. New York: Wiley; 2000.
Google Scholar
Tourangeau R, Rips LJ, Rasinski K. The psychology of survey response. Cambridge: Cambridge University Press; 2000.
Lancsar E, Louviere J, Donaldson C, Currie G, Burgess L. Best worst discrete choice experiments in health: methods and an application. Soc Sci Med. 2013;76:74–82.
Louviere JJ, Flynn TN, Marley A. Best–worst scaling: theory, methods and applications. Cambridge: Cambridge University Press; 2015.
Bartels R, Fiebig DG, van Soest A. Consumers and experts: an econometric analysis of the demand for water heaters. Empir Econ. 2006;31(2):369–91.
King MT, Hall J, Lancsar E, Fiebig D, Hossain I, Louviere J, et al. Patient preferences for managing asthma: results from a discrete choice experiment. Health Econ. 2007;16(7):703–17.
Jung K, Feldman R, Scanlon D. Where would you go for your next hospitalization? J Health Econ. 2011;30(4):832–41.
Article PubMed PubMed Central Google Scholar
Harris KM, Keane MP. A model of health plan choice: inferring preferences and perceptions from a combination of revealed preference and attitudinal data. J Econ. 1998;89(1):131–57.
Swait J, Erdem T. Brand effects on choice and choice set formation under uncertainty. Market Sci. 2007;26(5):679–97.
Swait J, et al. Context dependence and aggregation in disaggregate choice analysis. Market Lett. 2002;13:195–205.
McFadden D. Conditional logit analysis of qualitative choice behavior. Berkeley, CA: University of California; 1974.
McFadden D. Disaggregate behavioral travel demand’s RUM side. A 30 year retrospective. Travel Behav Res. 2000:17–63.
Maddala G. Limited dependent and qualitative variables in econometrics. Cambridge: Cambridge University Press; 1983.
Fiebig DG, Keane MP, Louviere J, Wasi N. The generalized multinomial logit model: accounting for scale and coefficient heterogeneity. Market Sci. 2010;29(3):393–421.
Hess S, Rose JM. Can scale and coefficient heterogeneity be separated in random coefficients models? Transportation. 2012;36(6):1225–39.
Revelt D, Train K. Mixed logit with repeated choices: households’ choices of appliance efficiency level. Rev Econ Stat. 1998;80(4):647–57.
Brownstone D, Train K. Forecasting new product penetration with flexible substitution patterns. J Econ. 1998;89(1):109–29.
Hall J, Fiebig DG, King MT, Hossain I, Louviere JJ. What influences participation in genetic carrier testing? Results from a discrete choice experiment. J Health Econ. 2006;25(3):520–37.
Hole AR. Modelling heterogeneity in patients’ preferences for the attributes of a general practitioner appointment. J Health Econ. 2008;27(4):1078–94.
Louviere JJ, Street D, Burgess L, Wasi N, Islam T, Marley AA. Modeling the choices of individual decision-makers by combining efficient choice experiment designs with extra preference information. J Choice Model. 2008;1(1):128–64.
Keane M, Wasi N. Comparing alternative models of heterogeneity in consumer choice behavior. J Appl Econ. 2013;28(6):1018–45.
Lancsar E, Louviere J, Flynn T. Several methods to investigate relative attribute impact in stated preference experiments. Soc Sci Med. 2007;64(8):1738–53.
Fiebig DG, Knox S, Viney R, Haas M, Street DJ. Preferences for new and existing contraceptive products. Health Econ. 2011;20(S1):35–52.
Lancsar E, Louviere J. Estimating individual level discrete choice models and welfare measures using best–worst choice experiments and sequential best–worst MNL. Sydney: University of Technology, Centre for the Study of Choice (Censoc); 2008. p. 08-004.
Scarpa R, Notaro S, Louviere J, Raffaelli R. Exploring scale effects of best/worst rank ordered choice data to estimate benefits of tourism in alpine grazing commons. Am J Agric Econ. 2011;93(3):813–28.
Punj GN, Staelin R. The choice process for graduate business schools. J Market Res. 1978;15(4):588–98.
Chapman RG, Staelin R. Exploiting rank ordered choice set data within the stochastic utility model. J Market Res. 1982;19(3):288–301.
Beggs S, Cardell S, Hausman J. Assessing the potential demand for electric cars. J Econ. 1981;17(1):1–19.
Gu Y, Hole AR, Knox S. Fitting the generalized multinomial logit model in Stata. Stata J. 2013;13(2):382–97.
Hole AR. Fitting mixed logit models by using maxium simulated likelihood. Stata J. 2007;7:388–401.
Pacifico D, Yoo HI. lclogit: a stata module for estimating latent class conditional logit models via the Expectation-Maximization algorithm. Stata J. 2013;13(3):625–39.
Baker MJ. Adaptive Markov chain Monte Carlo sampling and estimation in Mata. Stata J. 2014;14(3):623–61.
Suits DB. Dummy variables: mechanics v. interpretation. Rev Econ Stat. 1984;66:177–80.
Bech M, Gyrd-Hansen D. Effects coding in discrete choice experiments. Health Econ. 2005;14(10):1079–83.
Hole AR, Yoo I. The use of heuristic optimization algorithms to facilitate maximum simulated likelihood estimation of random parameter logit models. J R Stat Soc C. 2017;. doi: 10.1111/rssc.12209 .
Czajkowski M, Budziński W. An insight into the numerical simulation bias—a comparison of efficiency and performance of different types of quasi Monte Carlo simulation methods under a wide range of experimental conditions. In: Environmental Choice Modelling Conference; Copenhagen; 2015.
Bhat CR. Quasi-random maximum simulated likelihood estimation of the mixed multinomial logit model. Transp Res Part B: Methodol. 2001;35(7):677–93.
Train KE. Discrete choice methods with simulation. Cambridge: Cambridge University Press; 2009.
Hess S, Train KE, Polak JW. On the use of a Modified Latin Hypercube Sampling (MLHS) method in the estimation of a Mixed Logit Model for vehicle choice. Transp Res Part B: Methodol. 2006;40(2):147–63.
Garrido RA. Estimation performance of low discrepancy sequences in stated preferences. In: 10th International Conference on Travel Behaviour Research; Lucerne; 2003.
Munger D, L’Ecuyer P, Bastin F, Cirillo C, Tuffin B. Estimation of the mixed logit likelihood function by randomized quasi-Monte Carlo. Transp Res Part B: Methodol. 2012;46(2):305–20.
Hole AR. A comparison of approaches to estimating confidence intervals for willingness to pay measures. Health Econ. 2007;16(8):827–40.
Train K, Weeks M. Discrete choice models in preference space and willingness-to-pay space: Applications of simulation methods in environmental and resource economics. Berlin: Springer; 2005. p. 1–16.
Ben-Akiva M, McFadden D, Train K. Foundations of stated preference elicitation consumer behavior and choice-based conjoint analysis. In: 2015, Society for economic measurement annual conference, Paris, 24 July 2015.
Greene WH, Hensher DA. A latent class model for discrete choice analysis: contrasts with mixed logit. Transp Res Part B: Methodol. 2003;37(8):681–98.
Johar M, Fiebig DG, Haas M, Viney R. Using repeated choice experiments to evaluate the impact of policy changes on cervical screening. Appl Econ. 2013;45(14):1845–55.
Lancsar E, Wildman J, Donaldson C, Ryan M, Baker R. Deriving distributional weights for QALYs through discrete choice experiments. J Health Econ. 2011;30:466–78.
Lancsar E, Savage E. Deriving welfare measures from discrete choice experiments: inconsistency between current methods and random utility and welfare theory. Health Econ. 2004;13(9):901–7.
Elshiewy O, Zenetti G, Boztug Y. Differences between classical and Bayesian estimates for mixed logit models: a replication study. J Appl Econ. 2017;32(2):470–76.
Ryan M, Bate A. Testing the assumptions of rationality, continuity and symmetry when apply- ing discrete choice experiments in health care. Appl Econ Lett. 2001;8:59–63.
Ryan M, San MF. Revisiting the axiom of completeness in health care. Health Econ. 2003;12:295–307.
Lancsar E, Louviere J. Deleting, “irrational” responses from discrete choice experiments: a case of investigating or imposing preferences? Health Econ. 2006;15(8):797–811.
Fiebig DG, Viney R, Knox S, Haas M, Street DJ, Hole AR, et al. Consideration sets and their role in modelling doctor recommendations about contraceptives. Health Econ. 2017;26(1):54–73.
Hensher DA, Greene WH. Non-attendance and dual processing of common-metric attributes in choice analysis: a latent class specification. Empir Econ. 2010;39(2):413–26.
Lagarde M. Investigating attribute non-attendance and its consequences in choice experiments with latent class models. Health Econ. 2013;22(5):554–67.
Hole AR, Kolstad JR, Gyrd-Hansen D. Inferred vs. Stated attribute non-attendance in choice experiments: a study of doctors’ prescription behaviour. J Econ Behav Organ. 2013;96:21–31.
Flynn TN, Bilger M, Finkelstein EA. Are efficient designs used in discrete choice experiments too difficult for some respondents? A case study eliciting preferences for end-of-life care. Phamacoeconomics. 2016;34(3):273–84.
Mark TL, Swait J. Using stated preference and revealed preference modeling to evaluate prescribing decisions. Health Econ. 2004;13(6):563–73.
Ben-Akiva M, Bradley M, Morikawa TJ, Benjamin T, Novak H, Oppewal H, et al. Combining revealed and stated preferences data. Market Lett. 1994;5(4):335–49.
Lancsar E, Burge P. Choice modelling research in health economics. In: Hess S, Daly A, editors. Handbook of choice modelling. Cheltenham, UK: Edward Elgar Press; 2014. p. 675–87.
Hess S, Daly A. Handbook of choice modelling. Cheltenham: Edward Elgar Publishing; 2014.
Download references
Acknowledgements
The authors thank Peter Ghijben, Emily Lancsar and Silva Zavarsek for making available the data used in Ghijben et al. [ 11 ]. All authors jointly conceived the intent of the paper, drafted the manuscript and approved the final version.
Author information
Authors and affiliations.
Centre for Health Economics, Monash Business School, Monash University, 75 Innovation Walk, Clayton, VIC, 3800, Australia
Emily Lancsar
School of Economics, University of New South Wales, Sydney, NSW, Australia
Denzil G. Fiebig
Department of Economics, University of Sheffield, Sheffield, UK
Arne Risa Hole
You can also search for this author in PubMed Google Scholar
Corresponding author
Correspondence to Emily Lancsar .
Ethics declarations
No funding was received for the preparation of this paper.
Conflicts of interest
Emily Lancsar is funded by an ARC Fellowship (DE1411260). Emily Lancsar, Denzil Fiebig and Arne Risa Hole have no conflicts of interest.
Electronic supplementary material
Below is the link to the electronic supplementary material.
Supplementary material 1 (DTA 877 kb)
Supplementary material 2 (dta 135 kb), supplementary material 3 (dta 1469 kb), supplementary material 4 (docx 84 kb), rights and permissions.
Reprints and permissions
About this article
Lancsar, E., Fiebig, D.G. & Hole, A.R. Discrete Choice Experiments: A Guide to Model Specification, Estimation and Software. PharmacoEconomics 35 , 697–716 (2017). https://doi.org/10.1007/s40273-017-0506-4
Download citation
Published : 03 April 2017
Issue Date : July 2017
DOI : https://doi.org/10.1007/s40273-017-0506-4
Share this article
Anyone you share the following link with will be able to read this content:
Sorry, a shareable link is not currently available for this article.
Provided by the Springer Nature SharedIt content-sharing initiative
- Mixed Logit
- Supplementary Appendix
- Preference Heterogeneity
- Scale Heterogeneity
- Choice Occasion
- Find a journal
- Publish with us
- Track your research
Help | Advanced Search
Economics > Econometrics
Title: a step-by-step guide to design, implement, and analyze a discrete choice experiment.
Abstract: Discrete Choice Experiments (DCE) have been widely used in health economics, environmental valuation, and other disciplines. However, there is a lack of resources disclosing the whole procedure of carrying out a DCE. This document aims to assist anyone wishing to use the power of DCEs to understand people's behavior by providing a comprehensive guide to the procedure. This guide contains all the code needed to design, implement, and analyze a DCE using only free software.
Subjects: | Econometrics (econ.EM) |
Cite as: | [econ.EM] |
(or [econ.EM] for this version) | |
Focus to learn more arXiv-issued DOI via DataCite |
Submission history
Access paper:.
- Other Formats
References & Citations
- Google Scholar
- Semantic Scholar
BibTeX formatted citation

Bibliographic and Citation Tools
Code, data and media associated with this article, recommenders and search tools.
- Institution
arXivLabs: experimental projects with community collaborators
arXivLabs is a framework that allows collaborators to develop and share new arXiv features directly on our website.
Both individuals and organizations that work with arXivLabs have embraced and accepted our values of openness, community, excellence, and user data privacy. arXiv is committed to these values and only works with partners that adhere to them.
Have an idea for a project that will add value for arXiv's community? Learn more about arXivLabs .

- Erasmus University Rotterdam
What is the best Stated Choice Experiment Software?
Most recent answer.

Popular answers (1)

Top contributors to discussions in this field

- The Boeing Company

- Temple University

- The World Islamic Science and Education University (WISE)

- Dr.-Ing. Michael Lersow

Get help with your research
Join ResearchGate to ask questions, get input, and advance your work.
All Answers (4)

Similar questions and discussions
- Asked 23 April 2014

- Asked 28 April 2022

- Asked 29 October 2021

- Asked 2 August 2016

- Asked 25 November 2014

- Asked 14 March 2014

- Asked 13 March 2023

- Asked 28 February 2022

- Asked 25 September 2021

Related Publications

- Recruit researchers
- Join for free
- Login Email Tip: Most researchers use their institutional email address as their ResearchGate login Password Forgot password? Keep me logged in Log in or Continue with Google Welcome back! Please log in. Email · Hint Tip: Most researchers use their institutional email address as their ResearchGate login Password Forgot password? Keep me logged in Log in or Continue with Google No account? Sign up
Navigation Menu
Search code, repositories, users, issues, pull requests..., provide feedback.
We read every piece of feedback, and take your input very seriously.
Saved searches
Use saved searches to filter your results more quickly.
To see all available qualifiers, see our documentation .
- Notifications You must be signed in to change notification settings
Experimental designs for discrete choice models
ighdez/choicedesign
Folders and files.
Name | Name | |||
---|---|---|---|---|
13 Commits | ||||
Repository files navigation
Choicedesign.
ChoiceDesign is a Python package tool to construct D-efficient designs for Discrete Choice Experiments. ChoiceDesign combines enough flexibility to construct from simple 2-alternative designs with few attributes, to more complex settings that may involve conditions between attributes. ChoiceDesign is a revamped version of EDT , a project I created some years ago for the same purpose. ChoiceDesign includes improvements over EDT such as class-based syntax, coding improvements, better documentation and making this package available to install via pip .
NEW: ChoiceDesign now integrates Biogeme , which allows you to customise the utility functions.
Installation
ChoiceDesign is available to install via the regular syntax of pip :
- python3 -m pip install choicedesign
The main features of ChoiceDesign are:
- D-efficient designs based on a random swapping algorithm
- Customisable utility functions (powered by Biogeme )
- Bayesian priors (experimental)
- Designs with conditions over different attribute levels
- Designs with blocks.
- Multiple stopping criteria (Fixed number of iterations, iterations without improvement or fixed time).
I provide some Jupyter notebooks that illustrate the use of ChoiceDesign in the examples/ folder of this repo.
How to contribute?
Any contributions to ChoiceDesign are welcome via this Git, or to my email joseignaciohernandezh at gmail dot com.
This software is provided for free and as it is, say with no warranty , and neither me nor my current institution is liable of any consequence of the use of it. In any case, integrity checks have been performed by comparing results with alternative software.
- Bierlaire, M. (2003). BIOGEME: A free package for the estimation of discrete choice models. In Swiss transport research conference .
- Kuhfeld, W. F. (2005). Experimental design, efficiency, coding, and choice designs. Marketing research methods in SAS: Experimental design, choice, conjoint, and graphical techniques , 47-97.
- Quan, W., Rose, J. M., Collins, A. T., & Bliemer, M. C. (2011). A comparison of algorithms for generating efficient choice experiments.
Contributors 2
- Python 100.0%
Our systems are now restored following recent technical disruption, and we’re working hard to catch up on publishing. We apologise for the inconvenience caused. Find out more: https://www.cambridge.org/universitypress/about-us/news-and-blogs/cambridge-university-press-publishing-update-following-technical-disruption
We use cookies to distinguish you from other users and to provide you with a better experience on our websites. Close this message to accept cookies or find out how to manage your cookie settings .

Login Alert
- > Applied Choice Analysis
- > Experimental design and choice experiments
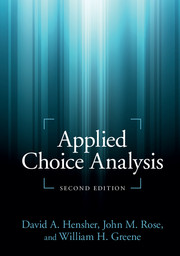
Book contents
- Frontmatter
- List of Figures
- List of Tables
- Part I Getting started
- 1 In the beginning
- 3 Choice and utility
- 4 Families of discrete choice models
- 5 Estimating discrete choice models
- 6 Experimental design and choice experiments
- 7 Statistical inference
- 8 Other matters that analysts often inquire about
- Part II Software and data
- Part III The suite of choice models
- Part IV Advanced topics
- Select glossary
6 - Experimental design and choice experiments
from Part I - Getting started
Published online by Cambridge University Press: 05 June 2015
As far as the laws of mathematics refer to reality, they are not certain; and as far as they are certain, they do not refer to reality.
This chapter was co-authored with Michiel Bliemer and Andrew Collins.
Introduction
This chapter might be regarded as a diversion from the main theme of discrete choice models and estimation; however, the popularity of stated choice (SC) data developed within a formal framework known as the “design of choice experiments” is sufficient reason to include one chapter on the topic, a topic growing in such interest that it justifies an entire book-length treatment. In considering the focus of this chapter (in contrast to the chapter in the first edition), we have decided to focus on three themes. The first is a broad synthesis of what is essentially experimental design in the context of data needs for choice analysis (essentially material edited from the first edition). The second is an overview in reasonable chronological order of the main developments in the literature on experimental design, drawing on the contribution of Rose and Bliemer (2014), providing an informative journey on the evolution of approaches that are used to varying degrees in the design and implementation of choice experiments. With the historical record in place, we then focus on a number of topics which we believe need to be given a more detailed treatment, which includes sample size issues, best–worst designs, and pivot designs. We draw on the key contributions in Rose and Bliemer (2012, 2013); Rose (2014); and Rose et al . (2008). We use Ngene (Choice Metrics 2012), a comprehensive tool that complements Nlogit5 and which has the capability to design the wide range of choice experiments discussed in this chapter, and to provide syntax for use in a few of the designs. We refer the reader to the Ngene manual for more details (www.choice-metrics.com/documentation.html).
Access options
Save book to kindle.
To save this book to your Kindle, first ensure [email protected] is added to your Approved Personal Document E-mail List under your Personal Document Settings on the Manage Your Content and Devices page of your Amazon account. Then enter the ‘name’ part of your Kindle email address below. Find out more about saving to your Kindle .
Note you can select to save to either the @free.kindle.com or @kindle.com variations. ‘@free.kindle.com’ emails are free but can only be saved to your device when it is connected to wi-fi. ‘@kindle.com’ emails can be delivered even when you are not connected to wi-fi, but note that service fees apply.
Find out more about the Kindle Personal Document Service .
- Experimental design and choice experiments
- David A. Hensher , University of Sydney , John M. Rose , University of Sydney , William H. Greene , New York University
- Book: Applied Choice Analysis
- Online publication: 05 June 2015
- Chapter DOI: https://doi.org/10.1017/CBO9781316136232.008
Save book to Dropbox
To save content items to your account, please confirm that you agree to abide by our usage policies. If this is the first time you use this feature, you will be asked to authorise Cambridge Core to connect with your account. Find out more about saving content to Dropbox .
Save book to Google Drive
To save content items to your account, please confirm that you agree to abide by our usage policies. If this is the first time you use this feature, you will be asked to authorise Cambridge Core to connect with your account. Find out more about saving content to Google Drive .
Choice-based Conjoint
Choice-Based Conjoint (CBC) Analysis
Introduction to conjoint analysis
Conjoint analysis is a category of research methods, among which choice-based conjoint is the most popular, that mimics the respondent’s real world tradeoffs when making decisions. It is used for pricing studies, product optimization, healthcare options and many other things. To get a more in-depth understanding of conjoint analysis, refer to our page on conjoint analysis.
What is choice-based conjoint (CBC)?
When you want to understand and predict how people make choices when facing challenging tradeoffs, Choice-Based Conjoint (CBC) is the most widely-used survey-based approach. CBC also is known as discrete choice modeling (DCM) or discrete choice experiments (DCE).
Related video: Creating a Choice-Based Conjoint Exercise with Discover
What is the difference between conjoint analysis and discrete choice?
Choosing a preferred product from a group of products is a simple and natural task that everyone can understand. The difference between choice-based conjoint and the earliest approaches to conjoint analysis is that the respondent expresses preferences by choosing from sets of concepts, rather than rating or ranking them.
How Does The Choice Model Work?
In this example of a choice model, the user is given the choice of four truck types with various attributes.
Example of a choice task
The combination of attribute (feature) levels we ask respondents to evaluate is critical to making choice-based conjoint analysis work properly. Fortunately, Sawtooth Software takes care of those details (though you may import your own designs if you wish).
Each level appears nearly an equal number of times and appears with levels from other attributes nearly an equal number of times. This makes for a fair and balanced (orthogonal) experiment where the utility value (the preference) of each attribute level can be measured independently and with high precision.
Build a preference model
We can assess the relative impact of each attribute level on choice just by counting "wins." But, it’s more precise to fit statistical models such as multinomial logit (MNL), latent class MNL, and hierarchical Bayesian (HB) estimation.
HB is the most popular approach and leads to a set of utility scores for the attribute list for each respondent. Latent class can find groups of respondents who are very similar to one another in their choice preferences, while being very different between the groups. Thus, latent class is an excellent approach to leverage choice-based conjoint data for needs-based segmentation and strategy.
Want help with your first discrete choice analysis conjoint study?
Want in-depth help with your first project that goes beyond our free technical support? Our Sawtooth Analytics consulting team has deep expertise in discrete choice modeling, decades of experience, and is ready to help.
Learn about our choice-based conjoint consulting team
Use Cases for Discrete Choice Analysis
Researchers often use discrete choice modeling to study the relationship between price and demand, especially when the price-demand relationship can differ from brand to brand. One of the strengths of choice-based conjoint is its ability to deal with interactions, such as between brand and price, or when different colors work better with different styles.
Choice-Based Conjoint (CBC) is used in marketing and economics applications across a variety of cases and industries, including:
- New product design, existing product redesign or line extension
- Pricing studies
- Market segmentation
- Healthcare choices, including cancer regimens
- Employee research (health plans, benefits)
- Transportation choice
- Legal disputes
- Green energy, electric vehicles, ride sharing
- Public health
- Environmental impact
- Finance, banking
- Technology and innovation
Technical Papers on Conjoint Analysis
Technical Paper
Try Choice-Based Conjoint in our products
Lighthouse studio.
For developing web-based, CAPI (mobile devices not connected to the web), or paper-and-pencil (3rd party platform) choice-based conjoint studies. This is installed, Windows-based software that seamlessly integrates with our web-based services for free survey hosting.
Our streamlined web-based platform. Simple CBC capabilities in an intuitive, easy to use tool. Low cost entry point and you can import your projects to Lighthouse Studio if you ever need more advanced capabilities.
Sawtooth Software
3210 N Canyon Rd Ste 202
Provo UT 84604-6508
United States of America
Support: [email protected]
Consulting: [email protected]
Sales: [email protected]
Products & Services
Support & Resources
Build Ngene™ Experiments in SurveyEngine
You can now create experiment designs directly in SurveyEngine using Ngene™ the preferred choice of professional researchers.
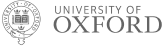
All Major Ngene™ Features
- Orthogonal designs
- Efficient designs based on local or Bayesian priors
- Full factorial & random fractional factorial designs
- Avoidance of dominant alternatives
- User-specified profile constraints
- Main and interaction effects, linear and nonlinear
.. with SurveyEngine usability
- Automatic Ngene™ script creation from your experiment
- Iterate and document versions of your working designs
- Output of D-error and A-error criteria
- One-click import into SurveyEngine
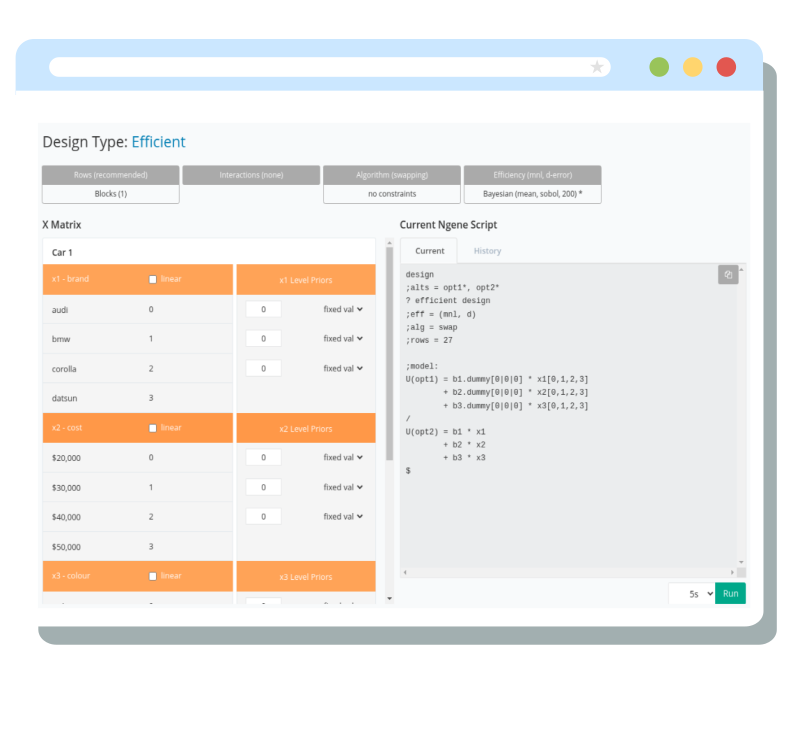
Frequently Asked Questions
Do i need to buy an ngene™ desktop license no you don’t. once you sign up to a surveyengine subscription you can start building ngene ™ designs from within your browser while you are creating your experiment..
Does Ngene ™ within SurveyEngine do all the things Ngene™ desktop does? Almost. All the Ngene design types, specification of priors, constraints and interactions are included. What it doesn’t do are designs that take hours or days to run as well as some advanced specifications such as candidate design import. You can always use the desktop version of Ngene™ at http://www.choice-metrics.com/ and import your designs directly into SurveyEngine.
I already have Ngene™, can I use it instead and just import my designs? Yes of course, this type of use is supported by all subscription plans. You can also import any design matrix you’ve generated offline, or create a survey from scratch by importing an Ngene design; all pages, scenarios and experiment structure will be automatically created.
What is Ngene and what is an experimental design? Experimental designs are ‘plans’ on how to run experiments within a survey: what levels of attributes to show and when. Ngene™ is widely used, cited and is regarded as the best tool to generate experimental designs for Academic and Commercial Stated Choice Experiments. For more information visit www.choice-metrics.com .
Do I even need to use Experiment Designs, what’s wrong with randomised treatments? You don’t, but we hope you have deep pockets and a taste for gambling. Without a directed, fit-for-purpose design one has no control over learning effects and dominance. An appropriate design controls for these and gives you statistical confidence that you can capture the necessary variation for valid models. For small or limited samples an experiment design is critical to success.
What does it cost? Current pricing for SurveyEngine monthly subscription with and without Ngene can be found here . For Ngene desktop licensing visit www.choice-metrics.com/download.html#licence .
I’m new to Experiment Designs, How do I get help? ChoiceMetrics runs an active forum and courses through the year. More information can be found here www.choice-metrics.com/support.html#forum
An excellent book my Michiel Bliemer and John Rose is available as pre-print and will be published in the Handbook of Choice Modelling this year: https://bit.ly/3OKjCVS
Read the publications by Deborah Street and Leonie Burgess, pioneers in the field. For example “The Construction of Optimal Stated Choice Experiments” ( onlinelibrary.wiley.com/doi/book/10.1002/9780470148563 )
Or just contact SurveyEngine at [email protected] , we may be able to help.
About Ngene™ and SurveyEngine
Ngene™ is developed and maintained by ChoiceMetrics in Sydney who are: John Rose, Michiel Bliemer, Andrew Collins and David Hensher. The Ngene™ syntax was borne out of the NLOGIT software by William Greene and David Hensher.
SurveyEngine has collaborated with ChoiceMetrics, running courses and projects together. In 2022 it was decided to embark on a technical integration with Ngene™ and bring the benefits of truly world-class experiment design software into the SurveyEngine Experiment and Data collection platform.
A first demonstration of the power of this integration was made in Reykjavik to the International Choice Modelling Conference (ICMC) early 2022 with the integration released commercially in December 2022.
For more information on Ngene™ visit the ChoiceMetrics website choice-metrics.com .
Talk to one of our Experts
We’re always here to help you with your research projects.
- Open access
- Published: 19 September 2024
Musculoskeletal patients’ preferences for care from physiotherapists or support workers: a discrete choice experiment
- Panos Sarigiovannis ORCID: orcid.org/0000-0001-7960-1500 1 , 2 ,
- Luis Enrique Loría-Rebolledo 3 ,
- Nadine E. Foster 1 , 4 ,
- Sue Jowett 5 &
- Benjamin Saunders 1
BMC Health Services Research volume 24 , Article number: 1095 ( 2024 ) Cite this article
166 Accesses
3 Altmetric
Metrics details
Delegation of clinical tasks from physiotherapists to physiotherapy support workers is common yet varies considerably in musculoskeletal outpatient physiotherapy services, leading to variation in patient care. This study aimed to explore patients’ preferences and estimate specific trade-offs patients are willing to make in treatment choices when treated in musculoskeletal outpatient physiotherapy services.
A discrete choice experiment was conducted using an efficient design with 16 choice scenarios, divided into two blocks. Adult patients with musculoskeletal conditions recruited from a physiotherapy service completed a cross-sectional, online questionnaire. Choice data analyses were conducted using a multinomial logit model. The marginal rate of substitution for waiting time to first follow-up physiotherapy appointment and distance from the physiotherapy clinic was calculated and a probability model was built to estimate the probability of choosing between two distinct physiotherapy service options under different scenarios.
382 patient questionnaires were completed; 302 participants were treated by physiotherapists and 80 by physiotherapists and support workers. There was a significant preference to be seen by a physiotherapist, have more follow-up treatments, to wait less time for the first follow-up appointment, to be seen one-to-one, to see the same clinician, to travel a shorter distance to get to the clinic and to go to clinics with ample parking. Participants treated by support workers did not have a significant preference to be seen by a physiotherapist and it was more likely that they would choose to be seen by a support worker for clinic scenarios where the characteristics of the physiotherapy service were as good or better.
Conclusions
Findings highlight that patients treated by support workers are likely to choose to be treated by support workers again if the other service characteristics are as good or better compared to a service where treatment is provided only by physiotherapists. Findings have implications for the design of physiotherapy services to enhance patient experience when patients are treated by support workers. The findings will contribute to the development of “best practice” recommendations to guide physiotherapists in delegating clinical work to physiotherapy support workers for patients with musculoskeletal conditions.
Peer Review reports
Musculoskeletal (MSK) conditions such as arthritis and low back pain are the leading cause of years lived with disability worldwide; they affect an estimated 20.2 million people across the UK, where they are the second leading cause of sickness absence from work [ 41 ]. Most MSK conditions can be managed in primary care or outpatient services in hospitals; evaluation and treatment by physiotherapists are frequently part of the treatment pathway [ 4 ]. Patients are assessed by physiotherapists and if they need follow-up treatments, these are provided by either a physiotherapist or a physiotherapy support worker.
The physiotherapy support worker role was developed to address some of the challenges affecting healthcare service delivery and the physiotherapy workforce worldwide. This includes an increasingly ageing population and an associated burden of healthcare; spiralling costs; increased patient expectations and a shortage of registered physiotherapists [ 3 , 11 ] (Lizarondo et al., 2010; Munn et al., 2013). Physiotherapy support workers, who may also be known as physiotherapy assistants, rehabilitation assistants, technical instructors or physiotherapy technicians, are non-registered staff who work alongside physiotherapists to provide delegated interventions and responsibilities [ 33 ]. They do not hold a qualification accredited by a professional association and are not formally regulated by a statutory body.
In the UK, physiotherapy support workers form approximately 15% of the total physiotherapy workforce [ 37 ] and a significant proportion of them work in MSK physiotherapy services within the National Health Service (NHS). They may undertake any activity that is in pursuit of physiotherapy goals provided that the activity is delegated to them by a registered healthcare professional with appropriate supervision in place and, where necessary or indicated, access to support and advice from a registered physiotherapist [ 38 ]. However, physiotherapy support workers’ roles are relatively undefined and as such, there is considerable variation in the duties and tasks they undertake [ 31 ]. National guidance from the Chartered Society of Physiotherapy (CSP Footnote 1 ) about delegation of tasks to support workers largely leave decision-making to the individual physiotherapist, their judgement of the task and their assessment of the competence of the support worker [ 39 ]. Consequently, in some physiotherapy services, physiotherapy support workers have a predominantly clinical role whereas in others they fulfil primarily an administrative role such as inputting data and booking appointments which leads to variation in clinical care [ 31 ].
A recent survey that explored current practice of UK MSK physiotherapists in relation to delegating clinical tasks to physiotherapy support workers, demonstrated that there is considerable variation in practice and delegation appears very patient-dependent [ 33 ]. This evidence suggests the need for a best practice framework to guide physiotherapists when delegating clinical tasks to support workers and standardise delegation. This need is being addressed through the Musculoskeletal Outpatient Physiotherapy Delegation (MOPeD) mixed methods study [ 34 ]. The first stage of the MOPeD study was a focused ethnographic study which explored how the culture in physiotherapy services may influence how tasks are delegated to physiotherapy support workers. It included detailed observations in two NHS musculoskeletal outpatient physiotherapy services and interviews with patients and clinicians. Stage 2a was a consensus study to reach agreement about what ‘best practice’ delegation recommendations should include.
A crucial step in the process of developing the ‘best practice’ recommendations is to understand musculoskeletal patients’ preferences about whether they are treated by physiotherapists or by physiotherapy support workers. The aim of the current study, which forms stage 2b of the MOPeD study, was to explore patients’ preferences about their care in MSK outpatient physiotherapy services and estimate specific trade-offs patients are willing to make in treatment choices when they are treated by physiotherapy support workers in MSK outpatient physiotherapy services.
- Discrete choice experiment
A discrete choice experiment (DCE) was carried out. The DCE was conducted to elicit patients’ preferences when treated by physiotherapists and physiotherapy support workers in NHS MSK physiotherapy services. DCEs are an attribute-based survey method for measuring benefits (utility). Within healthcare, DCEs have been applied to address a wide range of issues in the delivery of healthcare including measuring and valuing attributes of a healthcare service and identifying the factors that influence choices and decisions of patients, the public and healthcare professionals [ 28 , 35 ]. DCEs are based on the assumption that a service can be described by its characteristics or attributes, and the extent to which an individual values the service depends on the levels of these characteristics [ 36 ]. In a DCE, respondents are asked to choose between two or more alternatives, implicitly trading between the characteristics’ levels.
Ethical approval for the study was granted by the South West – Frenchay NHS Research Ethics Committee (REC) and the UK Health Research Authority (REC reference 21/SW/0158, IRAS project 297095).
Attributes and levels
Development of the attributes and levels was guided by the qualitative findings from the first stage of the MOPeD study, which included semi-structured interviews of 19 patients who were treated by physiotherapists and support workers for a MSK condition (Sarigiovannis et al., in preparation), and further input from the study’s patient and public involvement and engagement (PPIE) and clinical advisory groups [ 34 ]. From the thematic analysis of the interviews, we identified factors that influenced patient preference. In addition, we explicitly asked participants of the PPIE group to identify the most important physiotherapy service characteristics that would influence their choice if they had to choose between two different physiotherapy services. The qualitative findings and the feedback from the PPIE and clinical advisory group were reviewed and combined to inform the selection of attributes and levels for the DCE [ 7 ]. The findings were reviewed by the PPIE group again and finally, seven attributes were included in the DCE. Table 1 shows the attributes and levels used in the MOPeD DCE.
For each choice task, participants were asked to select one of the two physiotherapy services (for further details refer to DCE instrument section). An opt out option was considered but it was decided not to include one as the choice frame reflects the real choice a patient would face. Additionally, it has been shown that inclusion of an opt out option results in small differences between the forced and unforced choice model while at the same time it compromises statistical significance [ 5 , 40 ]. Given the attributes and levels, there were 1536 possible combinations of choice tasks (2 3 × 3 × 4 3 ). A D-efficient design, for a multinomial logit model (MNL) Footnote 2 was used to select 16 choice tasks using the NGENE software. The design included an alternative specific constant (ASC) for the second alternative, which captures the likelihood of choice with respect to the first alternative when all attributes are held equal. The design was blocked into two versions of eight choice tasks [ 15 , 21 ]. The final design was selected based on the lowest D-error, maximum level balance and utility balance, and minimal within-alternative correlation, minimal overlap [ 26 , 29 , 36 ].
DCE instrument
The DCE was administered as a cross-sectional questionnaire online survey with different sections which included questions about the participants’ health as well as sociodemographic data such as ethnicity, education and employment. The questionnaire was available online on the Qualtrics platform and participants completed it using tablet devices which were available at the participating clinics. However, a paper version of the questionnaire was produced to facilitate the ethical approval process (additional file 1). Participants were randomised to complete one of the two versions of the choice-set questions. An example of a choice-set question is shown in Fig. 1 together with the text that introduced the choice tasks.
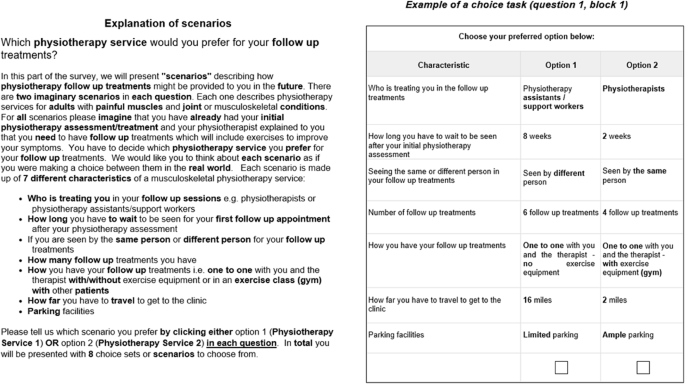
Explanation of scenarios and example of a choice task (question 1, block 1)
The order of the choice tasks was randomised in each version. The questionnaire was piloted three times prior to the final version, to ensure ease of comprehension and test completion times. Following the first pilot, the order and wording of questions changed and after the second pilot an additional section about participants’ current treatment was added to facilitate comprehension. No changes were made after the third and final pilot. Depending on their answers, each participant was required to answer 22 to 25 questions in total. For example, only participants who answered that they used the car park at their physiotherapy clinic were asked to answer if the car park was ample or limited.
Patient and public involvement and engagement
Meetings were held throughout the study with a group of seven patients to develop the DCE: three male and four female. All patients in the group had experience of treatment by a physiotherapist and/or physiotherapy support worker for a MSK condition. This support and feedback were essential in selecting the DCE attributes, designing and piloting the questionnaire, producing the participant.
information leaflets and interpreting the results. For example, based on the feedback from the PPIE group, it was decided to only offer the questionnaire in an online format which participants would complete using tablet devices in the participating physiotherapy clinics.
Clinical advisory group
The design and methods of the DCE were also informed by discussion with a group of clinicians consisting of four physiotherapists, three physiotherapy support workers, one physiotherapy operational lead and one clinical lead. Based on the feedback received from the study’s clinical advisory group, it was decided that patient recruitment would be completed by the lead author, who was employed by the participating Trust, and three research facilitators. The group supported the delivery of the study, interpretation of results and dissemination.
DCE sample size
The minimum sample size needed for DCEs depends on the specific hypotheses to be tested [ 10 ]. The sample size calculation was based on Orme’s formula which takes into account the number of choice tasks, the number of alternatives and the maximum number of levels for all attributes [ 24 ]. The absolute minimum number of participants required for the DCE would be 125 participants per block or 250 participants in total. However, as the need to conduct subgroup analysis was taken into consideration, it was decided to recruit between 180 and 200 per block or 360–400 participants in total [ 25 ].
Participant eligibility criteria and recruitment
Adult patients (18 years old or older) who were treated for a MSK condition in one of the eight participating physiotherapy clinics in Midlands Partnership University NHS Foundation Trust (MPFT) were eligible to participate. The clinics were part of a primary care musculoskeletal physiotherapy service which served urban and rural areas covering the geographical area of North and South Staffordshire. Patients were referred to the physiotherapy service predominantly by general practitioners (GPs), first contact practitioners (FCPs) or advanced physiotherapy practitioners (APPs).
Physiotherapists within participating site(s), invited patients to self-complete the questionnaire when patients were either attending for their follow-up physiotherapy appointment or were discharged from treatment during pre-arranged recruitment days during the 4-month-recruitment period. Patients were directed to the researcher (PS) or the research facilitator when they expressed their interest to participate or to find out more information about the study. Patients who agreed to participate were asked to provide informed, written consent prior to completing the DCE questionnaire. Patients were provided with a tablet to complete the survey in a quiet office where the researcher (PS) or a research facilitator were available to help them if they encountered any difficulties with the equipment.
Data analyses
Data analyses were conducted in STATA (Stata Corporation 2023, Stata Statistical Software: Release 18. College Station, Texas: Stata Corporation LLC). Data were analysed using an MNL model with robust standard errors. Additionally, subgroup analyses were conducted to identify the potential effect of observed patient characteristics [ 8 ]. For example, the sample was split into various subgroups including patients who were referred for physiotherapy because of a spinal condition or conditions other than spinal, patients with reduced or not reduced activities of daily living (ADL) due to their musculoskeletal condition(s), patients with or without at least a degree qualification, patients aged either under 65 years old or 65 years old and older, patients who were female or not and patients being treated by physiotherapists or by support workers. Marginal rates of substitution (MRS) (e.g., the ratio of two parameters) were calculated in terms of waiting times and travelling distance to allow direct comparison across subgroups which facilitated interpretation of data and account for observed heterogeneity [ 9 , 12 , 42 ]. Waiting times and travelling distance were selected since they have been reported to be important for patients [ 17 ] (Pitkänen and Linnosmaa, 2021). Finally, mean estimates were used to estimate the probability of choosing one of the two physiotherapy services based on scenarios of interest (e.g., between services provided by physiotherapist and support worker).
Study participants
382 patients were recruited, with 232 (60.73%) attending their physiotherapy appointments within North Staffordshire and 150 (39.27%) in South Staffordshire. Mean completion time of the online survey was 11.16 min. 251 participants (65.71%) were female and 190 patients (49.74%) were 65 years old or older. 371 participating patients (97.12%) described their ethnic group as “white”. 155 participants (40.57%) reported having no qualifications, whereas 142 (37.17%) held at least a degree qualification. 192 participants (50.26%) were retired and 117 (30.63%) preferred not to reveal their household income. 176 (46.07%) participants stated that they suffered from long-term conditions which affected their activities of daily living. Characteristics of the study participants are shown in Table 2 .
MNL model analysis
There was no evidence the position of the alternative scenario impacted the likelihood of choosing a service (e.g., no left to right bias). The results (Table 3 ) showed patients prefer being seen by a physiotherapist as opposed to a support worker; waiting less time to be seen for the first follow up appointment and receiving continuity of care. Furthermore, the results indicated that patients prefer to have more follow-up treatments; travel less distance to get to the physiotherapy clinic and have appointments in clinics with ample parking. Finally, being treated in an exercise class with other patients was preferred less than when being treated one-to-one without equipment. However, when the treatment was delivered one-to-one, they were indifferent to having access to equipment. Appendix shows the MNL model analysis for the relevant subgroups.
Marginal rates of substitution for waiting time and distance
Figure 2 shows the MRS in terms of waiting time for the two subgroups: patients currently treated by support workers and those treated by physiotherapists. Patients treated by physiotherapists were willing to wait an additional period of 8.76 weeks to be seen by a physiotherapist, 13.57 weeks to be treated by the same clinician for their follow-up treatments, 0.35 weeks for each additional follow-up appointment, 8.82 weeks to be treated one-to-one without equipment instead for being treated in a class with other patients, 0.34 weeks to be seen one-to-one with equipment instead of one-to-one without equipment, 1.16 weeks per mile being closer to the physiotherapy clinic and 3.53 weeks to go to a clinic with an ample parking. Patients who were treated by physiotherapy support workers were willing to wait an additional period of 0.23 weeks to be seen by a physiotherapist, 7.71 weeks to be treated by the same clinician for their follow-up treatments, 0.95 weeks for each additional follow-up appointment, 1.07 weeks to be treated one-to-one without equipment, 0.49 weeks to be seen one-to-one with equipment, 1.40 weeks per mile being closer to the physiotherapy clinic and 4.10 weeks to go to a clinic with an ample parking.
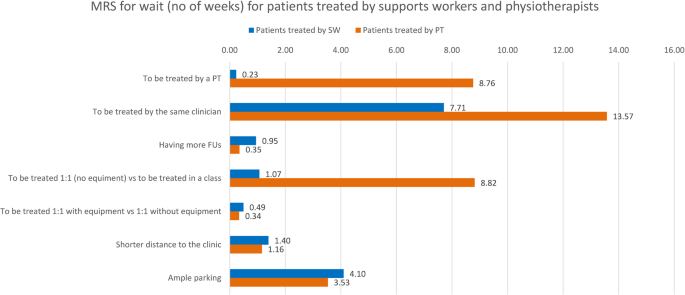
Willingness to wait values to get a marginal change per attribute for patients treated by support workers and physiotherapists
Figure 3 shows the MRS for travelling distance for the same subgroups. Patients treated by physiotherapists were willing to travel an additional distance of 7.57 miles to be seen by a physiotherapist, 0.86 miles for each week that they have to wait less to be seen for their first follow-up appointment, 11.72 miles to be treated by the same clinician for their follow-up treatments, 0.30 miles for each additional follow-up appointment, 7.61 weeks to be treated one-to-one without equipment instead for being treated in a class with other patients, 0.29 miles to be seen one-to-one with equipment instead of being treated one-to-one without equipment, and 3.53 miles to go to a clinic with an ample parking. Patients treated by physiotherapy support workers were willing to travel an additional distance of 0.17 miles to be seen by a physiotherapist, 0.72 miles for each week that they have to wait less to be seen for their first follow-up appointment, 5.52 miles to be treated by the same clinician, 0.68 miles for each additional follow-up appointment, 0.76 miles to be treated one-to-one without equipment instead for being treated in a class, 0.35 miles to be seen one-to-one with equipment, and 2.94 miles to go to a clinic with an ample parking.
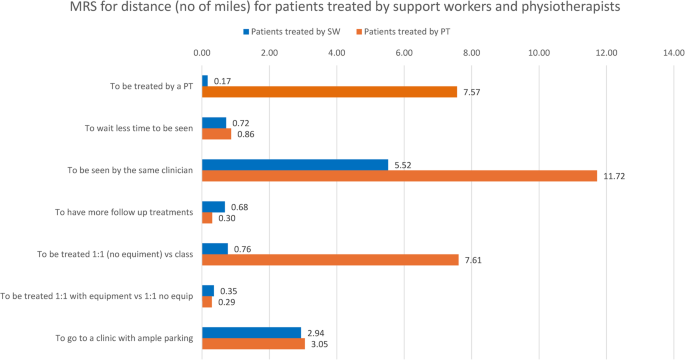
Willingness to travel values to get a marginal change per attribute for patients treated by support workers and physiotherapists
Probability of choosing services
Table 4 shows the probability of participants selecting one of the two physiotherapy service options listed in three different scenarios with two services each. Scenario 1 describes a choice between a service ( Service 1 ) where patients are treated by physiotherapists and one ( Service 2 ) where patients are treated by physiotherapy support workers in which other service characteristics are seemingly less favourable (e.g., farther away and more waiting time). Scenario 2 is similar to Scenario 1 with the only difference being the distance to the physiotherapy clinic has been reversed between the two services (i.e. 16 miles distance for Service 1 and 2 miles distance for Service 2 ). Scenario 3 also describes a Service 1 where the treatment is provided by physiotherapists and a Service 2 where treatment is provided by support workers, but with other service characteristics that seemingly favour the latter.
Results showed that in Scenario 1, on average most patients (96.72%) would choose the service with the physiotherapist ( Service 1 ). Furthermore, who the patient’s current clinician is does not seem to impact the likelihood of choice: 97.80% patients being treated by physiotherapists and 90.74% patients being treated by support workers would choose the service with the physiotherapist ( Service 1 ). Similarly, in Scenario 2, on average most patients (77.88%) would choose the service with the physiotherapist ( Service 1) . However, given the change in travel distances to each clinic, there was a bigger difference between the two subgroups: 83.81% of patients treated by physiotherapists would choose Service 1 compared to 50.10% of patients treated by support workers.
In Scenario 3, given the least favourable service characteristics of the service where the treatment is provided by physiotherapists ( Service 1 ), on average the choice proportions between the two services were more equally split with 46.66% of patients would choose a service with a support worker. At the same time, there was also evidence that current treating clinician had an effect on probabilities: 69.56% of patients treated by support workers were likely to choose the service which included a support worker versus 40.18% of those treated by physiotherapists. Conversely, 59.82% of participants who have been seen by a physiotherapist versus 30.44% of those seen by a support worker would choose a service where treatment is provided exclusively by physiotherapists even when the other characteristics are less favourable.
Main findings
This paper reports the findings of a study which used DCE methodology to elicit patients’ preferences when they are treated by physiotherapists and physiotherapy support workers in MSK outpatient physiotherapy services. Patients elicited expected preferences. Nevertheless, the results of this DCE showed that patients’ experience of being treated by a physiotherapist or support worker previously is a significant factor when making a choice about the future. Patients who had been seen by support workers were likely to choose to be seen by a support worker again provided the other service characteristics such as travelling distance, parking facilities or number of follow-up treatments, are similar or marginally better when compared to those of a physiotherapy service where follow up treatments are provided by physiotherapists.
Comparison with previous research literature
Charles et al. [ 6 ] conducted a DCE to explore older patients’ preferences for hip fracture rehabilitation services. The DCE attributes included whether the treating clinician was a qualified physiotherapist/occupational therapist or a support worker. The authors reported that there was a significant preference for the healthcare professional delivering the rehabilitation sessions to be a qualified physiotherapist or occupational therapist. Nevertheless, their results should be viewed with caution as the study sample used in their DCE was small (41 patients). Additionally, Charles et al. [ 6 ] described support workers in their DCE as “supervised unqualified assistants” which may have been confusing and misleading for participants. Physiotherapy support workers are non-registered clinicians but not unqualified as the vast majority of them have qualifications that enable them to work safely and effectively in their role [ 31 ].
Uncertainty and lack of clarity in relation to support workers’ role as well as lack of previous experience of being treated by a physiotherapy support worker, may have been among the factors which influenced patients, who were not treated by support workers in our study, to choose to be treated by a physiotherapist instead of a support worker. Historically, there has been a lack of regulation and registration for physiotherapy support workers, and as a result, many of these positions have evolved with variations in the title, and an inconsistent understanding of the role as well as the educational and supervision requirements [ 14 , 32 ].
Convenience is a well-known factor for treatment choice. It has been reported that patients tend to choose their preferred location taking into account parking availability, duration of trip and transport services’ availability and costs (Perry et al., 2015, Pitkänen and Linnosmaa 2021). Pitkänen and Linnosmaa (2021) reported that, in general, all patients prefer high-quality providers within short distances. This is supported by the findings of this study as patients preferred to travel the shortest possible travelling distance to get to the physiotherapy clinic. Nevertheless, patients in our study were prepared to travel longer distances to be seen by the same clinician. Continuity of care of care is known to have important benefits for patients, healthcare professionals and healthcare systems [ 13 , 16 , 22 ].
Mason [ 23 ] reported that between 70 and 90% of patients who accessed hospitals used cars to attend for outpatient appointments and that and the parking experience could be an additional source of financial pressure, worry and stress, which appeared to affect patient satisfaction principally when it was either very good or very bad. Participants in our sample clearly demonstrated their significant preference for physiotherapy clinics with ample parking.
Physiotherapy treatments for MSK conditions are usually delivered on a one-to-one basis or in group settings. There is evidence that physiotherapy treatments provided in groups are similarly effective to one to one care and cost-effective [ 1 , 30 ]. While group-based treatments may be appropriate for some patients, they will not suit all patients [ 19 ]. Participants in our study preferred to be seen one to one instead of in a group; however, patients treated by support workers did not have a strong preference to be seen one to one. It is reported that healthcare providers increasingly seek to improve quality of patient care by focusing on patients’ needs and preferences, with ‘patient-centredness’ recognised as a domain of quality in its own right [ 27 ]. To implement patient-centred care, it is essential that clinicians take into consideration patient preferences when they formulate their treatment plan [ 18 ].
Strengths and limitations
A robust process was followed in designing the DCE, involving incorporation of findings of the previous stages of the MOPeD study and input from different stakeholders, including patients. This process was reflected in the face validity of the analysis results. Additionally, a face-to-face data collection approach in this DCE resulted in high response rate and in receiving no incomplete questionnaires. Finally, we recruited 382 patients which allowed us to conduct various subgroup analyses. However, this study comes with limitations. First, the data analysis used a conditional or multinomial logit model (MNL) which has restrictive assumptions. For example, independence or irrelevant alternatives, independent and identically distributed errors and identical preferences across respondent e.g., no preference homogeneity [ 20 , 29 ]. An MNL was used, instead of a more flexible model that explores unobserved heterogeneity (but also contains requires other assumptions), to focus on more policy actionable observed heterogeneity as shown in the subgroup analysis and calculation of MRS and probabilities.
Second, linearity of the continuous variables was not tested because these were designed as continuous variables and not as categorical. The reason for this design specification was to minimise the degrees of freedom needed to estimate the model given the expected sample size [ 2 ]. Furthermore, the decision to treat them as continuous variables was supported by the guidance from the literature and the clinical advisory group of the study given the focus and ease of interpretation of the results if presented as marginal rates of substitution.
Third, participation of the study was limited to patients attending appointments in the eight participating physiotherapy MSK clinics within MPFT in North and South Staffordshire. Although the participants sample was not a representative sample of the UK population of MSK patients for ethnicity as most participants described their ethnic group as “white”, it was representative of the population of MSK patients that attend physiotherapy appointments in North and South Staffordshire. Partial postcode data were collected (the first part of the participants’ postcode) to ensure anonymity and comply with ethical approval but it was not possible to link the collected postcode data to full postcode data from other studies and such comparisons could not be made. We thus acknowledge that our findings may not be generalisable to all UK MSK patients including those who attend physiotherapy appointments for a MSK condition in the private sector. Nevertheless, the findings of this study highlight issues which are not pertinent exclusively to the NHS or the MSK clinical setting in relation to patients’ preferences when they are treated by support workers. Therefore, the findings may have wider applicability beyond the MSK setting or the NHS context and as such may be useful for informing research and practice in other settings both in the UK and internationally.
Conclusions and implications
The findings reported in this paper provide evidence about patients’ preferences as well as what service characteristics patients consider important when they are treated by physiotherapy support workers in MSK outpatient physiotherapy services. The findings demonstrated that patient experience of being treated by a physiotherapist or support worker is a significant factor when making a choice about the future. Unless patients have the experience of being treated by a physiotherapy support worker, they prefer to be treated by a physiotherapist regardless of any other less favourable characteristics of the physiotherapy service such as longer waits, distance and limited parking. Even when patients are treated by support workers, they seem to choose to be seen by a support worker again only when the other service characteristics are as good or favourable when compared to a service where treatment is provided only by physiotherapists.
The findings of this study indicate that there is a lack of understanding of the support workers’ role. They also suggest that it would be useful for patients to be given a clear explanation about the support worker role, their capabilities and why they are the most suitable and competent person for particular aspects of their treatment, in order to increase patients’ confidence and acceptability in being seen by a support worker. Finally, our findings highlight that continuity of care is important for patients. Within the study’s limitations, these findings can inform design of a physiotherapy service delivery to enhance patient experience when patients are treated by physiotherapists and support workers.
The findings of this study will be triangulated together with the results from the other stages of the MOPeD study to directly inform the development of “best practice” recommendations which incorporate the preference of patients, to guide physiotherapists to delegate clinical tasks to physiotherapy support workers in the MSK setting.
Availability of data and materials
The data that support the findings of this study are not openly available due to reasons of sensitivity and are available from the corresponding author upon reasonable request.
The Chartered Society of Physiotherapy (CSP) is the professional, educational and trade union body for the UK’s chartered physiotherapists, physiotherapy students and support workers.
The MNL model is described as the ‘workhorse of choice modelling’ and it has been recommended as a natural first model to estimate [ 20 ].
Abbreviations
Activities of Daily Living
Advanced Physiotherapy Practitioners
Alternative Specific Constant
Chartered Society of Physiotherapy
Discrete Choice Experiment
First Contact Practitioners
General Practitioners
Integrated Research Application System
Multinomial Logit
Musculoskeletal Outpatient Physiotherapy Delegation
Midlands Partnership University NHS Foundation Trust
Marginal Rates of Substitution
Musculoskeletal
National Health Service
Patient and Public Involvement and Engagement
Research Ethics Committee
United Kingdom
Allen KD, Sheets B, Bongiorni D, Choate A, Coffman CJ, Hoenig H, Huffman K, Mahanna EP, Oddone EZ, Van Houtven C, Wang V, Woolson S, Hastings SN. Implementation of a group physical therapy program for veterans with knee osteoarthritis. BMC Musculoskelet Disord. 2020;21(67). https://doi.org/10.1186/s12891-020-3079-x .
Assele SY, Meulders M, Vandebroek M. Sample size selection for discrete choice experiments using design features. J Choice Modelling. 2023. https://doi.org/10.1016/j.jocm.2023.100436 . 49,100436.
Article Google Scholar
Bosley S, Dale J. Healthcare assistants in general practice: practical and conceptual issues of skill-mix change. Br J Gen Pract. 2008;58:118–24. https://doi.org/10.3399/bjgp08X277032 .
Article PubMed PubMed Central Google Scholar
Budtz CR, Rikke Hansen RP, Thomsen JN, Christiansen DH. The prevalence of serious pathology in musculoskeletal physiotherapy patients – a nationwide register-based cohort study. Physiotherapy. 2021;112:96–102. https://doi.org/10.1016/j.physio.2021.03.004 .
Article PubMed Google Scholar
Campbell D, Erdem S. Including 2019 opt-out options in Discrete Choice experiments: issues to consider. Patient. 12(1):1–14. https://doi.org/10.1007/s40271-018-0324-6 .
Charles JM, Roberts JL, Ud Din N, Williams NH, Yeo ST, Edwards RT. Preferences of older patients regarding hip fracture rehabilitation service configuration: a feasibility discrete choice experiment. J Rehabil Med. 2018;50(7):636–42. https://doi.org/10.2340/16501977-2350 .
Coast J, Al-Janabi H, Sutton EJ, Horrocks SA, Vosper AJ, Swancutt DR, Flynn TN. Using qualitative methods for attribute development for discrete choice experiments: issues and recommendations. Health Econ. 2012;21:730–41. https://doi.org/10.1002/hec.1739 .
Cornelissen D, Boonen A, Bours S, Evers S, Dirksen C, Hiligsmann M. Understanding patients’ preferences for osteoporosis treatment: the impact of patients’ characteristics on subgroups and latent classes. Osteoporos Int. 2020;31(1):85–96. https://doi.org/10.1007/s00198-019-05154-9 .
Article CAS PubMed Google Scholar
de Bekker-Grob EW, Bliemer MC, Donkers B, Essink-Bot ML, Korfage IJ, Roobol MJ, Bangma CH, Steyerberg EW. Patients’ and urologists’ preferences for prostate cancer treatment: a discrete choice experiment. Br J Cancer. 2013;109(3):633–40. https://doi.org/10.1038/bjc.2013.370 .
de Bekker-Grob EW, Donkers B, Jonker MF, Stolk EA. Sample size requirements for Discrete-Choice experiments in Healthcare: a practical guide. Patient. 2015;8:373–84. https://doi.org/10.1007/s40271-015-0118-z .
Eliassen M, Henriksen N, Moe S. The practice of support personnel, supervised by physiotherapists, in Norwegian reablement services. Physiother Res Int. 2019;24:e1754. https://doi.org/10.1002/pri.1754 .
Fiebig DG, Haas M, Hossain I, Street DJ, Viney R. Decisions about pap tests: what influences women and providers? Soc Sci Med. 2009;68(10):766–1774. https://doi.org/10.1016/j.socscimed.2009.03.002 .
Gray SE, Tudtud B, Sheehan LR, Di Donato M. The association of physiotherapy continuity of care with duration of time loss among compensated Australian workers with low back pain. J Occup Rehabil. 2024. https://doi.org/10.1007/s10926-024-10209-8 .
Griffin R. Healthcare support workers. A practical guide for training and development. Abingdon: Routledge; 2023.
Google Scholar
Hensher DA, Rose JM, Greene WH. Applied Choice Analysis. 2nd ed. Cambridge: Cambridge University Press; 2015.
Book Google Scholar
Hull SA, Williams C, Schofield P, Boomla K, Ashworth M. Measuring continuity of care in general practice: a comparison of two methods using routinely collected data. Br J Gen Pract. 2022;72(724):e773–9. https://doi.org/10.3399/BJGP.2022.0043 .
Hush JM, Cameron K, Mackey M. Patient satisfaction with Musculoskeletal Physical Therapy Care: a systematic review. Phys Ther. 2011;91(1):25–36. https://doi.org/10.2522/ptj.20100061 .
Hutting N, Caneiro JP, Ong’wen OM, Miciak M, Roberts L. Patient-centered care in musculoskeletal practice: key elements to support clinicians to focus on the person. Musculoskelet Sci Pract. 2022;57:102434. https://doi.org/10.1016/j.msksp.2021.102434 .
Jack K, McLean SM, Moffett JK, Gardiner E. Barriers to treatment adherence in physiotherapy outpatient clinics: a systematic review. Man Ther. 2010;15(3):220–8. https://doi.org/10.1016/j.math.2009.12.004 .
Lancsar E, Fiebig DG, Hole AR. Discrete choice experiments: a guide to Model Specification, Estimation and Software. PharmacoEconomics. 2017;35(7):697–716. https://doi.org/10.1007/s40273-017-0506-4 .
Louviere JJ, Hensher DA, Swait JD. Stated choice methods: analysis and applications. Cambridge: Cambridge University Press; 2000.
Magel J, Kim J, Anne Thackeray A, Hawley A, Petersen S, Fritz JM. associations between Physical Therapy Continuity of Care and Health Care utilization and costs in patients with Low Back Pain: a retrospective cohort study. Phys Ther. 2018;98(12):990–9. https://doi.org/10.1093/ptj/pzy103 .
Mason A. Hospital car parking the impact of access costs. Working Paper. CHE Research Paper. University of York, Centre for Health Economics, York, UK. 2010. https://eprints.whiterose.ac.uk/139245/1/CHERP59_hospital_car_parking.pdf . Accessed 3/03/2024.
Orme B. Sample size issues for conjoint analysis studies. Sequim: Sawtooth Software Technical Paper; 1998.
Orme B. Getting Started with Conjoint Analysis: Strategies for Product Design and Pricing Research. 4th ed. Manhattan Beach: Research Publishers LLC.; 2020.
Reed Johnson F, Lancsar E, Marshall D, Kilambi V, Mühlbacher A, Regier DA, Bresnahan BW, Kanninen B, Bridges JF. Constructing experimental designs for discrete-choice experiments: report of the ISPOR Conjoint Analysis Experimental Design Good Research practices Task Force. Value Health. 2013;16(1):3–13. https://doi.org/10.1016/j.jval.2012.08.2223 .
Renedo A, Marston C. Developing patient-centred care: an ethnographic study of patient perceptions and influence on quality improvement. BMC Health Serv Res. 2015;15:122. https://doi.org/10.1186/s12913-015-0770-y .
Ryan M, Bate A, Eastmond C, Ludbrook A. Use of discrete choice experiments to elicit preferences. Quality in Health Care. 2001;10(Suppl I):i55–60 https://qualitysafety.bmj.com/content/10/suppl_1/i55 .
Ryan M, Watson V, Gerard K. Practical issues in conducting a discrete choice experiment. In: Ryan M, Gerard K, Amaya-Amaya M, editors. Using discrete choice experiments to value health and health care. The economics of non-market goods and resources series. Volume 11. Dordrecht: Springer; 2008.
Chapter Google Scholar
Ryans I, Galway R, Harte A, Verghis R, Agus A, Heron N, McKane R. The Effectiveness of Individual or Group Physiotherapy in the Management of Sub-Acromial Impingement: A Randomised Controlled Trial and Health Economic Analysis. Int J Environ Res Public Health. 2020;17(15):5565. https://doi.org/10.3390/ijerph17155565 .
Article CAS PubMed PubMed Central Google Scholar
Sarigiovannis P, Cropper S. An audit of the utilization of physiotherapy assistants in the musculoskeletal outpatients setting within a primary care physiotherapy service. Musculoskelet Care. 2018;16(3):405–8. https://doi.org/10.1002/msc.1238 .
Sarigiovannis P, Jowett S, Saunders B, Corp N, Bishop A. Delegation by Allied Health Professionals To Allied Health Assistants: a mixed methods systematic review. Physiotherapy. 2020;112:16–30. https://doi.org/10.1016/j.physio.2020.10.002 .
Sarigiovannis P, Foster NE, Jowett S, Saunders B. Delegation of workload from musculoskeletal physiotherapists to physiotherapy assistants/support workers: A UK online survey. Musculoskeletal Sci Pract. 2022;62(102631). https://doi.org/10.1016/j.msksp.2022.102631 .
Sarigiovannis P, Foster NE, Jowett S, Saunders B. Developing a best practice framework for musculoskeletal outpatient physiotherapy delegation: the MOPeD mixed-methods research study protocol. BMJ Open. 2023;13:e072989. https://doi.org/10.1136/bmjopen-2023-072989 .
Soekhai V, de Bekker-Grob EW, Ellis AR, Vass CM. Discrete choice experiments in Health Economics: past. Present Future Pharmacoeconomics. 2019;37(2):201–26. https://doi.org/10.1007/s40273-018-0734-2 .
Street DJ, Burgess L, Viney R, Louviere J. Designing discrete choice experiments for health care. In: Ryan M, Gerard K, Amaya-Amaya M, editors. Using discrete choice experiments to value health and health care. The economics of non-market goods and resources series. Volume 11. Dordrecht: Springer; 2008.
The CSP. UK physiotherapy workforce review. 2024. https://www.csp.org.uk/campaigns-influencing/shaping-healthcare/review-physiotherapy-workforce-uk . Accessed 09/04/2024.
The Chartered Society of Physiotherapy (CSP) Scope of practice for support workers. 2019. https://www.csp.org.uk/networks/associates-support-workers/scope-practice-support-workers . Accessed 6/11/2023.
The Chartered Society of Physiotherapy (CSP). Information Paper PD126 Supervision, Accountability, Delegation. 2020. https://www.csp.org.uk/system/files/publication_files/PD126%20Supervision_accountability_delegation_final%20%282020%29.pdf . Accessed on 06/11/2023.
Veldwijk J, Lambooij MS, de Bekker-Grob E, Smit HA, de Wit GA. The effect of including an opt-out option in discrete choice experiments. Value Health. 2013;16(3):PA46. https://doi.org/10.1016/j.jval.2013.03.260 .
Versus Arthritis. The State of Musculoskeletal Health. Arthritis and other Musculoskeletal conditions in number. 2023. https://www.versusarthritis.org/media/duybjusg/versus-arthritis-state-msk-musculoskeletal-health-2023pdf.pdf . Accessed on 09/04/2024.
Wright SJ, Vass CM, Sim G, Burton M, Fiebig DG, Payne K. Accounting for Scale Heterogeneity in Healthcare-Related Discrete Choice experiments when comparing stated preferences: a systematic review. Patient. 2018;11:475–88. https://doi.org/10.1007/s40271-018-0304-x .
Download references
Acknowledgements
The authors would like to thank the study participants, members of the study’s Patient and Public Involvement and Engagement (PPIE) group and members of the study’s Clinical Advisory group for their valuable input and advice.
Clinical trial number
Not applicable.
Panos Sarigiovannis was funded by National Institute for Health and Care Research award (Clinical Doctoral Research Fellowship NIHR301550) for this research project. The views expressed in this publication are those of the author(s) and not necessarily those of the NIHR, NHS or the UK Department of Health and Social Care.
NE Foster is funded through an Australian National Health and Medical Research Council Investigator grant (ID: 2018182).
Author information
Authors and affiliations.
Primary Care Centre Versus Arthritis, School of Medicine, Keele University, Staffordshire, ST5 5BG, UK
Panos Sarigiovannis, Nadine E. Foster & Benjamin Saunders
Midlands Partnership University NHS Foundation Trust, Newcastle under Lyme, Staffordshire, ST5 2BQ, UK
Panos Sarigiovannis
Health Economics Research Unit, University of Aberdeen, Aberdeen, AB25 2ZD, UK
Luis Enrique Loría-Rebolledo
STARS Education and Research Alliance, Surgical Treatment and Rehabilitation Service (STARS), The University of Queensland and Metro North Health, , Herston, Brisbane, Australia
Nadine E. Foster
Health Economics Unit, Institute of Applied Health Research, Public Health Building, University of Birmingham, Edgbaston, Birmingham, B15 2TT, UK
You can also search for this author in PubMed Google Scholar
Contributions
PS conceptualised and developed the idea for the study, designed the study, led the analysis of the data, wrote the first draft of the manuscript and critically edited the manuscript. LLR provided guidance in designing the study as well as conducting the data analysis and critically edited the manuscript. NF, SJ and BS conceptualised and developed the idea for the study, were involved in designing the study, analysing the data and critically edited the manuscript. All authors read and approved the final manuscript.
Author’s Twitter handles
Twitter:@sarigiovannis1
Corresponding author
Correspondence to Panos Sarigiovannis .
Ethics declarations
Ethics approval and consent to participate.
Ethical approval was granted by the South West – Frenchay NHS Research Ethics Committee and the UK Health Research Authority (REC reference 21/SW/0158, IRAS project 297095).
All participants were approached within the recruiting physiotherapy departments and provided informed written consent prior to completion of the DCE. All methods were carried out in accordance with the Declaration of Helsinki.
Competing interests
The authors have no competing interests to declare.
Additional information
Publisher’s note.
Springer Nature remains neutral with regard to jurisdictional claims in published maps and institutional affiliations.
Supplementary Information
Supplementary material 1, mnl model analysis - coefficient values for all participants and various subgroups a.
Variables and levels | ALL | Spinal = 1 | Spinal = 0 | ADL = 1 | ADL = 0 | Degree = 1 | Degree = 0 | SW = 1 | SW = 0 | 65+ = 1 | 65+ = 0 | Female = 1 | Female = 0 |
---|---|---|---|---|---|---|---|---|---|---|---|---|---|
Alternative Specific Constant (asc) | |||||||||||||
| 0.02 | 0.07 | -0.003 | 0.015 | 0.025 | 0.0383 | 0.01 | -0.027 | 0.031 | 0.002 | 0.047 | -0.013 | 0.086 |
Treating clinician | |||||||||||||
| -0.445 |
|
|
|
|
|
| -0.013 |
|
|
|
|
|
Waiting time for FU treatments | |||||||||||||
| -0.061 |
|
|
|
|
|
|
|
|
|
|
|
|
Continuity of treatment | |||||||||||||
| -0.787 |
|
|
|
|
|
|
|
|
|
|
|
|
Number of follow up (FU) treatments | |||||||||||||
| 0.030 | 0.027 |
|
|
|
|
|
|
|
|
|
| 0.021 |
Treatment mode | |||||||||||||
| -0.466 |
|
|
|
|
|
| -0.062 |
|
|
|
|
|
| 0.022 | -0.014 | 0.038 | -0.027 | 0.072 | 0.152 |
| 0.028 | 0.022 | 0.053 | 0 | 0 | 0.079 |
Distance to the clinic | |||||||||||||
| -0.075 |
|
|
|
|
|
|
|
|
|
|
|
|
Parking at the clinic | |||||||||||||
|
|
|
|
|
|
|
|
|
|
|
|
|
|
- Note: Due to scaling issues, the magnitude of the coefficient cannot be directly used to compare preference estimates. However, the significance provides insight as to how preferences change across subgroups
- a Coefficient values in bold indicate that the p value ≤ 0.05
Rights and permissions
Open Access This article is licensed under a Creative Commons Attribution 4.0 International License, which permits use, sharing, adaptation, distribution and reproduction in any medium or format, as long as you give appropriate credit to the original author(s) and the source, provide a link to the Creative Commons licence, and indicate if changes were made. The images or other third party material in this article are included in the article’s Creative Commons licence, unless indicated otherwise in a credit line to the material. If material is not included in the article’s Creative Commons licence and your intended use is not permitted by statutory regulation or exceeds the permitted use, you will need to obtain permission directly from the copyright holder. To view a copy of this licence, visit http://creativecommons.org/licenses/by/4.0/ .
Reprints and permissions
About this article
Cite this article.
Sarigiovannis, P., Loría-Rebolledo, L.E., Foster, N.E. et al. Musculoskeletal patients’ preferences for care from physiotherapists or support workers: a discrete choice experiment. BMC Health Serv Res 24 , 1095 (2024). https://doi.org/10.1186/s12913-024-11585-w
Download citation
Received : 03 May 2024
Accepted : 12 September 2024
Published : 19 September 2024
DOI : https://doi.org/10.1186/s12913-024-11585-w
Share this article
Anyone you share the following link with will be able to read this content:
Sorry, a shareable link is not currently available for this article.
Provided by the Springer Nature SharedIt content-sharing initiative
- Musculoskeletal physiotherapy
- Support workers
- Patient preferences
- Continuity of care
- Travel distance
BMC Health Services Research
ISSN: 1472-6963
- General enquiries: [email protected]

COMMENTS
Ngene is a powerful and flexible software tool to generate experimental designs for stated choice surveys. It is used by researchers, consultants, and students in more than 60 countries around the world. Stated choice surveys and experiments consist of a series of hypothetical choice tasks that are used to elicit behaviour and preferences from ...
DCEtool can use your design settings to reproduce a discrete choice experiment. This functionality can be used for practice, to test the performance of a given experimental design, or to conduct research with a small number of respondents. First, move to the 'Create a survey' tab and write an introductory and end text.
Ngene: the most comprehensive software for designing choice experiments. It is designed to be the single source of stated choice experimental designs. As such, it has an extensive range of features and outputs. ... but it will not show the levels of the experimental design. Download software. Download Ngene 1.4.
The design of choice experiments can be somewhat complex, consisting of several steps or stages. The typical steps involved in designing a choice experiment are: I. Determine whether an experiment is labelled or unlabelled depending on research questions; II. Determine the alternatives and attributes to include in the experiment;
RDG is confined to the design of unlabelled experiments with three alternatives. The second tool constitutes a newly developed extension of Ngene. Ngene is an established, highly versatile commercial software dedicated to the design of SC experiments (ChoiceMetrics, 2018). To facilitate creating decision rule robust efficient designs using the ...
arXiv:2009.11235v1 [econ.EM] 23 Sep 2020. Daniel Pérez-Troncoso A step-by-step guide to design, implement, and analyze a discrete choice experiment. T able 1: Example Choice Set. Attributes ...
ChoiceDesign is a Python package tool to construct D-efficient designs for Discrete Choice Experiments. ChoiceDesign combines enough flexibility to construct from simple 2-alternative designs with few attributes, to more complex settings that may involve conditions between attributes. ChoiceDesign is a revamped version of EDT, a project I ...
Features. Ngene is capable of generating design types for a wide range of discrete choice experiments and model types. Ngene assists you in getting the most out of a survey by maximising information and making choice tasks more realistic and familiar to respondents. Flexibility. Ease of use.
TU Delft researcher Sander van Cranenburgh, in collaboration with researchers from the University of Sydney, has developed an upgrade for leading software package Ngene. Ngene is used by researchers and professionals to design stated choice experiments. Such experiments are used to gain insight into how people weigh up various aspects when making a choice, for example travel time and travel costs.
We provide a user guide on the analysis of data (including best-worst and best-best data) generated from discrete-choice experiments (DCEs), comprising a theoretical review of the main choice models followed by practical advice on estimation and post-estimation. We also provide a review of standard software. In providing this guide, we endeavour to not only provide guidance on choice ...
EDT. EDT is a Python-based tool to construct D-efficient designs for Discrete Choice Experiments. EDT combines enough flexibility to construct from simple 2-alternative designs with few attributes, to more complex settings that may involve conditions between attributes. While EDT designs are based on the widely-used D-efficiency criterion (see ...
This tells the software that the attributes is generic and should use the same levels as in the first alternative. We'll leave this as is but come back to it. ... Experimental design is a critical aspect of choice experiments. An experimental design outlays the plan - what is shown in the experiment. This will be skimmed over here as it is ...
Abstract. Discrete choice experiment (DCE) is a well-established technique to elicit stated preferences. It is frequently used in social sciences, where revealed preferences are difficult or sometimes even impossible to collect. A DCE consists in a series of choice tasks, in which survey respondents are requested to select the alternative they ...
View a PDF of the paper titled A step-by-step guide to design, implement, and analyze a discrete choice experiment, by Daniel P\'erez-Troncoso. Discrete Choice Experiments (DCE) have been widely used in health economics, environmental valuation, and other disciplines. However, there is a lack of resources disclosing the whole procedure of ...
I would like to build a statistically optimal design for a Discrete Choice Experiment, using the idefix package in R, as described in Traets et al., 2020 (Generating Optimal Designs for Discrete ...
Choice modelling is frequently accompanied by a Choice Experiment when real-world data is weak or non-existent. This is a systematic and planned experiment, applied to respondents within a survey and used to generate preference data from hypotheticial scenarios. A short video on creating a choice experiment is here.
ChoiceDesign. ChoiceDesign is a Python package tool to construct D-efficient designs for Discrete Choice Experiments. ChoiceDesign combines enough flexibility to construct from simple 2-alternative designs with few attributes, to more complex settings that may involve conditions between attributes. ChoiceDesign is a revamped version of EDT, a ...
A discrete choice study uses experimental design to create sets of products, and subjects choose a product from each set. Like the conjoint model, the choice model has a linear utility function, but it is embedded in a nonlinear model (see page 71). The following table displays a choice design and the layout of a simple choice experiment ...
For example, in a paired choice design, an optimal choice sets design usually offers a large number of choice sets, more than a respondent can complete. However, the D. 1 2017 techniques offer designs consisting of 30 − 50 % fewer choice sets with near-optimal performance, i.e., designs have high D-efficiencies.
This chapter was co-authored with Michiel Bliemer and Andrew Collins. Introduction. This chapter might be regarded as a diversion from the main theme of discrete choice models and estimation; however, the popularity of stated choice (SC) data developed within a formal framework known as the "design of choice experiments" is sufficient ...
Conjoint analysis is a category of research methods, among which choice-based conjoint is the most popular, that mimics the respondent's real world tradeoffs when making decisions. It is used for pricing studies, product optimization, healthcare options and many other things. To get a more in-depth understanding of conjoint analysis, refer to ...
SurveyEngine has collaborated with ChoiceMetrics, running courses and projects together. In 2022 it was decided to embark on a technical integration with Ngene™ and bring the benefits of truly world-class experiment design software into the SurveyEngine Experiment and Data collection platform.
6.3 Constructing an experimental design that is appropriate for those attributes and levels, either from a design catalogue, or via a software program. ... An experimental design (below) in a Choice Experiment is a strict scheme for controlling and presenting hypothetical scenarios, or choice sets to respondents. For the same experiment ...
A discrete choice experiment was conducted using an efficient design with 16 choice scenarios, divided into two blocks. ... Footnote 2 was used to select 16 choice tasks using the NGENE software. The design included an alternative specific constant (ASC) for the second alternative, which captures the likelihood of choice with respect to the ...