Reference Library
Collections
- See what's new
- All Resources
- Student Resources
- Assessment Resources
- Teaching Resources
- CPD Courses
- Livestreams
Study notes, videos, interactive activities and more!
Psychology news, insights and enrichment
Currated collections of free resources
Browse resources by topic
- All Psychology Resources
Resource Selections
Currated lists of resources
Study Notes

Working Memory Model
Last updated 7 Nov 2023
- Share on Facebook
- Share on Twitter
- Share by Email
Baddeley and Hitch (1974) developed the Working Memory Model (WMM), which focuses specifically on the workings of short-term memory (STM).
Atkinson and Shiffrin’s Multi-Store Model of memory (MSM) was criticised for over-simplifying STM (as well as LTM) as a single storage system, so the WMM alternative proposed that STM is composed of three, limited capacity stores:
- Central Executive – this manages attention, and controls information from the two ‘slave stores’ [below]
- An articulatory rehearsal process (‘inner voice’) of language, including any language presented visually to convert to a phonological state, for storage in the:
- Phonological store (‘inner ear’), which holds auditory speech information and the order in which it was heard (or any visually-presented language converted by the articulatory process)
- Visuo-Spatial Sketchpad – this temporarily retains visual and spatial information
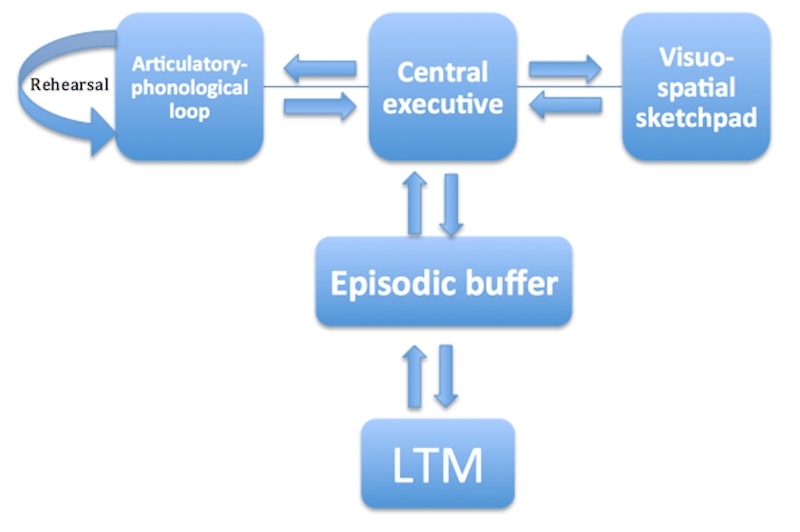
A later addition was the episodic buffer which facilitates communication between the central executive and long term memory.
The three-store STM stemmed from research using a ‘dual-task technique’ (or ‘interference tasks’), whereby performance is measured as participants perform two tasks simultaneously. The following observations provided evidence to suggest different, limited-capacity STM stores process different types of memory:
- If one store is utilised for both tasks, then task performance is poorer than when they are completed separately, due to the store’s limited capacity e.g. repeating “the the the” aloud and reading some text silently would use the articulatory-phonological loop for both tasks, slowing performance.
- If the tasks require different stores, performance would be unaffected when performing them simultaneously e.g. repeating “the the the” aloud whilst performing a reasoning task (requiring attention, i.e. the central executive), or whilst following a mobile stimulus with your eyes (using the visuo-spatial sketchpad).
Evaluation of the Working Memory Model
- The WMM provides an explanation for parallel processing (i.e. where processes involved in a cognitive task occur at once), unlike Atkinson and Shiffrin’s MSM.
- A Shallice and Warrington (1974) case study reported that brain-damaged patient KF could recall verbal but not visual information immediately after its presentation, which supports the WMM’s claim that separate short-term stores manage short-term phonological and visual memories.
- The model was developed based on evidence from laboratory experiments, so confounding variables could be carefully controlled to produce reliable results (that can be replicated).
- Despite providing more detail of STM than the multi-store model, the WMM has been criticized for being too simplistic and vague, e.g. it is unclear what the central executive is, or its exact role in attention.
- Results from laboratory experiments researching the WMM will often have low ecological validity (i.e. may not relate to real life), as tasks such as repeating ‘the the the’ are arguably not representative of our everyday activities.
- Working memory model
- Baddeley and Hitch (1974)
You might also like
duration of short-term memory, types of long term memory.
Quizzes & Activities
Multi-Store Model of Memory
Memory - key term "conundrum" activity, memory: mcq revision test 1 for aqa a level psychology.
Topic Videos
Example Answers for Memory: A Level Psychology, Paper 1, June 2018 (AQA)
Exam Support
Memory - "Connection Wall" activity
Related products.
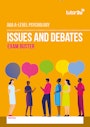
Issues & Debates Exam Buster Revision Guide for AQA A-Level Psychology
03-4130-30182-03
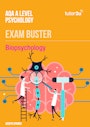
Biopsychology Exam Buster Revision Guide for AQA A-Level Psychology
03-4130-30181-03
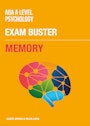
Memory Exam Buster Revision Guide for AQA A-Level Psychology
03-4130-30098-03
- View full selection ›
Our subjects
- › Criminology
- › Economics
- › Geography
- › Health & Social Care
- › Psychology
- › Sociology
- › Teaching & learning resources
- › Student revision workshops
- › Online student courses
- › CPD for teachers
- › Livestreams
- › Teaching jobs
Boston House, 214 High Street, Boston Spa, West Yorkshire, LS23 6AD Tel: 01937 848885
- › Contact us
- › Terms of use
- › Privacy & cookies
© 2002-2024 Tutor2u Limited. Company Reg no: 04489574. VAT reg no 816865400.
- Abnormal Psychology
- Assessment (IB)
- Biological Psychology
- Cognitive Psychology
- Criminology
- Developmental Psychology
- Extended Essay
- General Interest
- Health Psychology
- Human Relationships
- IB Psychology
- IB Psychology HL Extensions
- Internal Assessment (IB)
- Love and Marriage
- Post-Traumatic Stress Disorder
- Prejudice and Discrimination
- Qualitative Research Methods
- Research Methodology
- Revision and Exam Preparation
- Social and Cultural Psychology
- Studies and Theories
- Teaching Ideas
Limitations of the multi-store model of memory (Atkinson and Shiffrin, 1968)
Travis Dixon October 4, 2021 Cognitive Psychology

- Click to share on Facebook (Opens in new window)
- Click to share on Twitter (Opens in new window)
- Click to share on LinkedIn (Opens in new window)
- Click to share on Pinterest (Opens in new window)
- Click to email a link to a friend (Opens in new window)
Atkinson and Shiffrin’s MSM is over 50 years old yet it’s still in every introduction to Psychology textbook and still influences modern psychologists. But it’s not without its critics. This post will examine some of their critiques.
Because the MSM was so popular, it received a lot of criticism. But “…criticism could itself be viewed as a success, given the goal of science should be progress, and everyone should want to see old ideas be refined or replaced.” (Malmberg et al. 2019)
It’s difficult to find flaws with the MSM because if it had obvious flaws it wouldn’t have stood the test of time and still be mandatory material in most introduction to Psychology courses. Even experts struggle to find faults. Reviews of the MSM’s claims “…reveals them to be state-of-the-art today, uncovering, testing, and verifying fundamental processes of rehearsal, storage, and retrieval.” (Malmberg et al. 2019). That being said, we’ll review four common critiques of the MSM:

Atkinson and Shiffrin’s multi-store model of memory. (Wikicommons).
- Methodological limitations of the studies,
- Contradictory evidence,
- Oversimplification,
- Originality.
- The multi-store model of memory (Atkinson and Shiffrin, 1968)
- 5 things you didn’t know about the MSM
- Let’s make a D.E.A.L: evaluating theories in three simple steps
Methodological Limitations
The easiest (but least effective) way of critiquing a theory is by looking for methodological flaws in the supporting evidence. Laboratory experiments on memory like Peterson and Peterson’s and Glanzer and Cunitz’s use abstract, meaningless information to test subjects’ memory (e.g. trigrams or random word lists.) A&S themselves call these tasks “often meaningless.” It’s done to avoid prior knowledge affecting recall – how can you test someone’s ability to create new memories if the information you’re asking them to remember is already in their long-term memory? Thus, the information participants are asked to remember has to be meaningless in order to accurately test short-term memory.
But this raises the question of generalizability. To what extent can we use these findings to explain memory as it happens in real life? We don’t spend hours remembering meaningless information that has no personal relevance (although in exam season you might argue otherwise!) So can we really use findings from studies about trigrams to explain memory in other contexts? For instance, do you always have to rehearse information for it to be stored in your long-term memory?
A strong evaluation of generalizability would include real-life examples of memory of meaningful information that doesn’t need heaps of rehearsal.
Contradictory Evidence
Levels of processing.
Among numerous other critiques in their 1972 paper, Craik and Lockhart challenged the idea that rehearsal is the primary factor that influences the transfer of memory from the STS to the LTS. With their levels of processing model and supporting studies they showed that the depth with which information is processed can affect memory. They suggested three types of processing:
- Structural (based on physical shape; most shallow type of processing)
- Phonological (based on sound)
- Semantic (based on meaning; the deepest type of processing).
Information that is processed more deeply (e.g. semantically) will be remembered better than information that is processed superficially.
The results of their studies showed that how we process information in our STS affects the transfer to the LTS. This highlights one of the limitations of the MSM – it’s focus on maintenance rehearsal (saying things over and over) over other types of rehearsal and different ways of encoding information.
You can d ownload and read Craik and Lockhart’s original 1972 paper to find many more of their critiques of the multi-store model of memory.
The Primacy and Recency Effects
These have been used as evidence to support the MSM ( Read more ). However, one study showed a recency effect in students’ recall of US presidents (Roediger & Crowder, 1976) and another found a recency effect for rugby players recalling teams they’d played that season (Baddeley and Hitch, 1977). Why would a recency effect occur for information that was already in long-term memory? This information would have already been transferred to the long-term store, so theoretically a recency effect should not occur because the subject is not drawing this information from their short-term store. There must be another explanation, which challenges the idea that the recency effect supports the existence of a short-term store.
The peculiar case of KF
You probably know about the famous case study of HM – a man who lost the ability to make new memories. HM’s study supports the claim that short-term and long-term memory are different stores because HM could hold information in his STS but he could not make new memories (i.e. he could not transfer the information from his STS to his LTS). If memory was one single store then if he lost long-term storage abilities he would lose short-term storage as well.
So while you might know about HM, you might not know about KF. Like HM, KF also suffered brain damage but his was the result of a motorcycle accident when he was 17 years old. After the accident, his short-term memory was reduced drastically. He could only hold about two units of information in his working memory at any time (most people can hold around four). Remember that according to the MSM, information flows from the STS to the LTS. Because KF has almost zero capacity in his STS he should have an impaired ability to make new long-term memories. But this is not the case. Studies on KF found that his long-term memory abilities were normal. It seems from the studies that the information was bypassing the STS and going straight to KF’s LTS. This challenges the MSM’s claim that information flows from the STS to the LTS.
Oversimplification
Another critique of the MSM is that it oversimplified memory processes. For instance, the original theory focused primarily on maintenance rehearsal as the main way that memories transfer from STS to LTS. Studies have shown that other types of rehearsal, such as elaborative rehearsal , are more effective. This raises another issue with the MSM – it treats all memories as the same. But does all information pass through the stores similarly? Does all information need the same amount of rehearsal to transfer?
Maintenance rehearsal is a type of rote rehearsal which involves just reciting items over and over, whereas elaborative rehearsal is when we rehearse information by making connections between the new information and what we already know.
These studies also focus on information processed verbally (i.e. listening to words and letters). Some studies focus on visual information as well, but what about other sensory information? Does the process of memory formation happen the same with tastes and smells? How could this be tested? Do we need to “rehearse” this information? A&S admit in their original paper that the studies focus primarily on AVL information (auditory-verbal-linguistic). Perhaps then the model might not apply to all types of sensory information.
Originality
Could we critique the theory based on its originality? It’s a stretch, but it’s not as if the distinction between short-term and long-term memories was a unique idea. William James, known as the father of American psychology, proposed this distinction as early as 1890. Also, they were not even the first to present these ideas. Murdoch (1967) presented his modal model which attempted “…to synthesize some recent theoretical conceptions; the components include sensory, short-term and long-term stores with three different forgetting mechanisms (decay, displacement and interference, respectively).” That being said, even his modal model drew on ideas from Atikinson and Shiffrin’s 1965 paper. So really it’s not a critique of the model perhaps as much as a question regarding the singular praise A&S seem to have received for the ideas of a multi-store model of memory.
Atkinson and Shiffrin’s original 1968 paper they did not “present a finished theory” but they “set forth a general framework within which specific models can be formulated.” (p91)
As a teacher and writer I don’t like to “give the answers” when it comes to critical thinking. But with theories like the MSM it’s quite tricky. It’s an extremely valid theory with scores of supporting studies. This makes it almost impossible for a novice psychologists just starting out in the subject to independently come up with unique evaluations. In this post I’ve tried to give some guiding points but still leaving enough room for students to add their own critical thinking.
Travis Dixon is an IB Psychology teacher, author, workshop leader, examiner and IA moderator.
Support for the Working Memory Model
Shallice and warrington (1974) - study of kf.
The working memory model is supported by evidence from brain damaged patients such as KF.

Research aim & method
- Aim: To investigate a patient KF who had suffered brain damage in a motorcycle accident.
- Method: A case study using numerous psychometric tests, experiments and observations.

- KF’s short term memory problems were much greater for auditory information than visual, suggesting his brain damage was restricted to the phonological loop.

- The case of KF supports both the MSM and the WMM as his LTM was unaffected by his injury, suggesting LTM and STM are different stores.
- His case supports the WMM as his visuospatial sketchpad seems unaffected by his injury, suggesting that resides in a different area of the brain to the phonological loop which was damaged.

- In depth and detailed.
- Cannot generalise from a case study.
Limitations of the Working Memory Model
Here are some limitations of the working memory model.

Central executive
- What does the central executive actually do?
- The model suggests that it allocates attention, but it is not fully explained why it is needed.

Lacks ecological validity
- Dual task experiments are very artificial – lacks ecological validity.

Lack of generalisability
- Cannot generalise from case studies on brain damaged patients.

Ambiguity about the LTM
- It is only a model of working memory and leaves many unanswered questions about the structure of LTM.
1 Social Influence
1.1 Social Influence
1.1.1 Conformity
1.1.2 Asch (1951)
1.1.3 Sherif (1935)
1.1.4 Conformity to Social Roles
1.1.5 BBC Prison Study
1.1.6 End of Topic Test - Conformity
1.1.7 Obedience
1.1.8 Analysing Milgram's Experiment
1.1.9 Agentic State & Legitimate Authority
1.1.10 Variables of Obedience
1.1.11 Resistance to Social Influence
1.1.12 Minority Influence & Social Change
1.1.13 Minority Influence & Social Impact Theory
1.1.14 End of Topic Test - Social Influences
1.1.15 Exam-Style Question - Conformity
1.1.16 Top Grade AO2/AO3 - Social Influence
2.1.1 Multi-Store Model of Memory
2.1.2 Short-Term vs Long-Term Memory
2.1.3 Long-Term Memory
2.1.4 Support for the Multi-Store Model of Memory
2.1.5 Duration Studies
2.1.6 Capacity Studies
2.1.7 Coding Studies
2.1.8 The Working Memory Model
2.1.9 The Working Memory Model 2
2.1.10 Support for the Working Memory Model
2.1.11 Explanations for Forgetting
2.1.12 Studies on Interference
2.1.13 Cue-Dependent Forgetting
2.1.14 Eye Witness Testimony - Loftus & Palmer
2.1.15 Eye Witness Testimony Loftus
2.1.16 Eyewitness Testimony - Post-Event Discussion
2.1.17 Eyewitness Testimony - Age & Misleading Questions
2.1.18 Cognitive Interview
2.1.19 Cognitive Interview - Geiselman & Fisher
2.1.20 End of Topic Test - Memory
2.1.21 Exam-Style Question - Memory
2.1.22 A-A* (AO3/4) - Memory
3 Attachment
3.1 Attachment
3.1.1 Caregiver-Infant Interaction
3.1.2 Condon & Sander (1974)
3.1.3 Schaffer & Emerson (1964)
3.1.4 Multiple Attachments
3.1.5 Studies on the Role of the Father
3.1.6 Animal Studies of Attachment
3.1.7 Explanations of Attachment
3.1.8 Attachment Types - Strange Situation
3.1.9 Cultural Differences in Attachment
3.1.10 Disruption of Attachment
3.1.11 Disruption of Attachment - Privation
3.1.12 Overcoming the Effects of Disruption
3.1.13 The Effects of Institutionalisation
3.1.14 Early Attachment
3.1.15 Critical Period of Attachment
3.1.16 End of Topic Test - Attachment
3.1.17 Exam-Style Question - Attachment
3.1.18 Top Grade AO2/AO3 - Attachment
4 Psychopathology
4.1 Psychopathology
4.1.1 Definitions of Abnormality
4.1.2 Definitions of Abnormality 2
4.1.3 Phobias, Depression & OCD
4.1.4 Phobias: Behavioural Approach
4.1.5 Evaluation of Behavioural Explanations of Phobias
4.1.6 Depression: Cognitive Approach
4.1.7 OCD: Biological Approach
4.1.8 Evidence for the Biological Approach
4.1.9 End of Topic Test - Psychopathy
4.1.10 Exam-Style Question - Phobias
4.1.11 Top Grade AO2/AO3 - Psychopathology
5 Approaches in Psychology
5.1 Approaches in Psychology
5.1.1 Psychology as a Science
5.1.2 Origins of Psychology
5.1.3 Reductionism & Problems with Introspection
5.1.4 The Behaviourist Approach - Classical Conditioning
5.1.5 Pavlov's Experiment
5.1.6 Little Albert Study
5.1.7 The Behaviourist Approach - Operant Conditioning
5.1.8 Social Learning Theory
5.1.9 The Cognitive Approach 1
5.1.10 The Cognitive Approach 2
5.1.11 The Biological Approach
5.1.12 Gottesman (1991) - Twin Studies
5.1.13 Brain Scanning
5.1.14 Structure of Personality & Little Hans
5.1.15 The Psychodynamic Approach (A2 only)
5.1.16 Humanistic Psychology (A2 only)
5.1.17 Aronoff (1957) (A2 Only)
5.1.18 Rogers' Client-Centred Therapy (A2 only)
5.1.19 End of Topic Test - Approaches in Psychology
5.1.20 Exam-Style Question - Approaches in Psychology
5.2 Comparison of Approaches (A2 only)
5.2.1 Psychodynamic Approach
5.2.2 Cognitive Approach
5.2.3 Biological Approach
5.2.4 Behavioural Approach
5.2.5 End of Topic Test - Comparison of Approaches
6 Biopsychology
6.1 Biopsychology
6.1.1 Nervous System Divisions
6.1.2 Neuron Structure & Function
6.1.3 Neurotransmitters
6.1.4 Endocrine System Function
6.1.5 Fight or Flight Response
6.1.6 The Brain (A2 only)
6.1.7 Localisation of Brain Function (A2 only)
6.1.8 Studying the Brain (A2 only)
6.1.9 CIMT (A2 Only) & Postmortem Examinations
6.1.10 Biological Rhythms (A2 only)
6.1.11 Studies on Biological Rhythms (A2 Only)
6.1.12 End of Topic Test - Biopsychology
6.1.13 Top Grade AO2/AO3 - Biopsychology
7 Research Methods
7.1 Research Methods
7.1.1 Experimental Method
7.1.2 Observational Techniques
7.1.3 Covert, Overt & Controlled Observation
7.1.4 Self-Report Techniques
7.1.5 Correlations
7.1.6 Exam-Style Question - Research Methods
7.1.7 End of Topic Test - Research Methods
7.2 Scientific Processes
7.2.1 Aims, Hypotheses & Sampling
7.2.2 Pilot Studies & Design
7.2.3 Questionnaires
7.2.4 Variables & Control
7.2.5 Demand Characteristics & Investigator Effects
7.2.6 Ethics
7.2.7 Limitations of Ethical Guidelines
7.2.8 Consent & Protection from Harm Studies
7.2.9 Peer Review & The Economy
7.2.10 Validity (A2 only)
7.2.11 Reliability (A2 only)
7.2.12 Features of Science (A2 only)
7.2.13 Paradigms & Falsifiability (A2 only)
7.2.14 Scientific Report (A2 only)
7.2.15 Scientific Report 2 (A2 only)
7.2.16 End of Topic Test - Scientific Processes
7.3 Data Handling & Analysis
7.3.1 Types of Data
7.3.2 Descriptive Statistics
7.3.3 Correlation
7.3.4 Evaluation of Descriptive Statistics
7.3.5 Presentation & Display of Data
7.3.6 Levels of Measurement (A2 only)
7.3.7 Content Analysis (A2 only)
7.3.8 Case Studies (A2 only)
7.3.9 Thematic Analysis (A2 only)
7.3.10 End of Topic Test - Data Handling & Analysis
7.4 Inferential Testing
7.4.1 Introduction to Inferential Testing
7.4.2 Sign Test
7.4.3 Piaget Conservation Experiment
7.4.4 Non-Parametric Tests
8 Issues & Debates in Psychology (A2 only)
8.1 Issues & Debates in Psychology (A2 only)
8.1.1 Culture Bias
8.1.2 Sub-Culture Bias
8.1.3 Gender Bias
8.1.4 Ethnocentrism
8.1.5 Cross Cultural Research
8.1.6 Free Will & Determinism
8.1.7 Comparison of Free Will & Determinism
8.1.8 Reductionism & Holism
8.1.9 Reductionist & Holistic Approaches
8.1.10 Nature-Nurture Debate
8.1.11 Interactionist Approach
8.1.12 Nature-Nurture Methods
8.1.13 Nature-Nurture Approaches
8.1.14 Idiographic & Nomothetic Approaches
8.1.15 Socially Sensitive Research
8.1.16 End of Topic Test - Issues and Debates
9 Option 1: Relationships (A2 only)
9.1 Relationships: Sexual Relationships (A2 only)
9.1.1 Sexual Selection & Human Reproductive Behaviour
9.1.2 Intersexual & Intrasexual Selection
9.1.3 Evaluation of Sexual Selection Behaviour
9.1.4 Factors Affecting Attraction: Self-Disclosure
9.1.5 Evaluation of Self-Disclosure Theory
9.1.6 Self Disclosure in Computer Communication
9.1.7 Factors Affecting Attraction: Physical Attributes
9.1.8 Matching Hypothesis Studies
9.1.9 Factors Affecting Physical Attraction
9.1.10 Factors Affecting Attraction: Filter Theory 1
9.1.11 Factors Affecting Attraction: Filter Theory 2
9.1.12 Evaluation of Filter Theory
9.1.13 End of Topic Test - Sexual Relationships
9.2 Relationships: Romantic Relationships (A2 only)
9.2.1 Social Exchange Theory
9.2.2 Evaluation of Social Exchange Theory
9.2.3 Equity Theory
9.2.4 Evaluation of Equity Theory
9.2.5 Rusbult’s Investment Model
9.2.6 Evaluation of Rusbult's Investment Model
9.2.7 Relationship Breakdown
9.2.8 Studies on Relationship Breakdown
9.2.9 Evaluation of Relationship Breakdown
9.2.10 End of Topic Test - Romantic relationships
9.3 Relationships: Virtual & Parasocial (A2 only)
9.3.1 Virtual Relationships in Social Media
9.3.2 Evaluation of Reduced Cues & Hyperpersonal
9.3.3 Parasocial Relationships
9.3.4 Attachment Theory & Parasocial Relationships
9.3.5 Evaluation of Parasocial Relationship Theories
9.3.6 End of Topic Test - Virtual & Parasocial Realtions
10 Option 1: Gender (A2 only)
10.1 Gender (A2 only)
10.1.1 Sex, Gender & Androgyny
10.1.2 Gender Identity Disorder
10.1.3 Biological & Social Explanations of GID
10.1.4 Biological Influences on Gender
10.1.5 Effects of Hormones on Gender
10.1.6 End of Topic Test - Gender 1
10.1.7 Kohlberg’s Theory of Gender Constancy
10.1.8 Evaluation of Kohlberg's Theory
10.1.9 Gender Schema Theory
10.1.10 Psychodynamic Approach to Gender Development 1
10.1.11 Psychodynamic Approach to Gender Development 2
10.1.12 Social Approach to Gender Development
10.1.13 Criticisms of Social Theory
10.1.14 End of Topic Test - Gender 2
10.1.15 Media Influence on Gender Development
10.1.16 Cross Cultural Research
10.1.17 Childcare & Gender Roles
10.1.18 End of Topic Test - Gender 3
11 Option 1: Cognition & Development (A2 only)
11.1 Cognition & Development (A2 only)
11.1.1 Piaget’s Theory of Cognitive Development 1
11.1.2 Piaget's Theory of Cognitive Development 2
11.1.3 Schema Accommodation Assimilation & Equilibration
11.1.4 Piaget & Inhelder’s Three Mountains Task (1956)
11.1.5 Conservation & Class Inclusion
11.1.6 Evaluation of Piaget
11.1.7 End of Topic Test - Cognition & Development 1
11.1.8 Vygotsky
11.1.9 Evaluation of Vygotsky
11.1.10 Baillargeon
11.1.11 Baillargeon's studies
11.1.12 Evaluation of Baillargeon
11.1.13 End of Topic Test - Cognition & Development 2
11.1.14 Sense of Self & Theory of Mind
11.1.15 Baron-Cohen Studies
11.1.16 Selman’s Five Levels of Perspective Taking
11.1.17 Biological Basis of Social Cognition
11.1.18 Evaluation of Biological Basis of Social Cognition
11.1.19 Important Issues in Social Neuroscience
11.1.20 End of Topic Test - Cognition & Development 3
11.1.21 Top Grade AO2/AO3 - Cognition & Development
12 Option 2: Schizophrenia (A2 only)
12.1 Schizophrenia: Diagnosis (A2 only)
12.1.1 Classification & Diagnosis
12.1.2 Reliability & Validity of Diagnosis
12.1.3 Gender & Cultural Bias
12.1.4 Pinto (2017) & Copeland (1971)
12.1.5 End of Topic Test - Scizophrenia Diagnosis
12.2 Schizophrenia: Treatment (A2 only)
12.2.1 Family-Based Psychological Explanations
12.2.2 Evaluation of Family-Based Explanations
12.2.3 Cognitive Explanations
12.2.4 Drug Therapies
12.2.5 Evaluation of Drug Therapies
12.2.6 Biological Explanations for Schizophrenia
12.2.7 Dopamine Hypothesis
12.2.8 End of Topic Test - Schizoprenia Treatment 1
12.2.9 Psychological Therapies 1
12.2.10 Psychological Therapies 2
12.2.11 Evaluation of Psychological Therapies
12.2.12 Interactionist Approach - Diathesis-Stress Model
12.2.13 Interactionist Approach - Triggers & Treatment
12.2.14 Evaluation of the Interactionist Approach
12.2.15 End of Topic Test - Scizophrenia Treatments 2
13 Option 2: Eating Behaviour (A2 only)
13.1 Eating Behaviour (A2 only)
13.1.1 Explanations for Food Preferences
13.1.2 Birch et al (1987) & Lowe et al (2004)
13.1.3 Control of Eating Behaviours
13.1.4 Control of Eating Behaviour: Leptin
13.1.5 Biological Explanations for Anorexia Nervosa
13.1.6 Psychological Explanations: Family Systems Theory
13.1.7 Psychological Explanations: Social Learning Theory
13.1.8 Psychological Explanations: Cognitive Theory
13.1.9 Biological Explanations for Obesity
13.1.10 Biological Explanations: Studies
13.1.11 Psychological Explanations for Obesity
13.1.12 Psychological Explanations: Studies
13.1.13 End of Topic Test - Eating Behaviour
14 Option 2: Stress (A2 only)
14.1 Stress (A2 only)
14.1.1 Physiology of Stress
14.1.2 Role of Stress in Illness
14.1.3 Role of Stress in Illness: Studies
14.1.4 Social Readjustment Rating Scales
14.1.5 Hassles & Uplifts Scales
14.1.6 Stress, Workload & Control
14.1.7 Stress Level Studies
14.1.8 End of Topic Test - Stress 1
14.1.9 Physiological Measures of Stress
14.1.10 Individual Differences
14.1.11 Stress & Gender
14.1.12 Drug Therapy & Biofeedback for Stress
14.1.13 Stress Inoculation Therapy
14.1.14 Social Support & Stress
14.1.15 End of Topic Test - Stress 2
15 Option 3: Aggression (A2 only)
15.1 Aggression: Physiological (A2 only)
15.1.1 Neural Mechanisms
15.1.2 Serotonin
15.1.3 Hormonal Mechanisms
15.1.4 Genetic Factors
15.1.5 Genetic Factors 2
15.1.6 End of Topic Test - Aggression: Physiological 1
15.1.7 Ethological Explanation
15.1.8 Innate Releasing Mechanisms & Fixed Action Pattern
15.1.9 Evolutionary Explanations
15.1.10 Buss et al (1992) - Sex Differences in Jealousy
15.1.11 Evaluation of Evolutionary Explanations
15.1.12 End of Topic Test - Aggression: Physiological 2
15.2 Aggression: Social Psychological (A2 only)
15.2.1 Social Psychological Explanation
15.2.2 Buss (1963) - Frustration/Aggression
15.2.3 Social Psychological Explanation 2
15.2.4 Social Learning Theory (SLT) 1
15.2.5 Social Learning Theory (SLT) 2
15.2.6 Limitations of Social Learning Theory (SLT)
15.2.7 Deindividuation
15.2.8 Deindividuation 2
15.2.9 Deindividuation - Diener et al (1976)
15.2.10 End of Topic Test - Aggression: Social Psychology
15.2.11 Institutional Aggression: Prisons
15.2.12 Evaluation of Dispositional & Situational
15.2.13 Influence of Computer Games
15.2.14 Influence of Television
15.2.15 Evaluation of Studies on Media
15.2.16 Desensitisation & Disinhibition
15.2.17 Cognitive Priming
15.2.18 End of Topic Test - Aggression: Social Psychology
16 Option 3: Forensic Psychology (A2 only)
16.1 Forensic Psychology (A2 only)
16.1.1 Defining Crime
16.1.2 Measuring Crime
16.1.3 Offender Profiling
16.1.4 Evaluation of Offender Profiling
16.1.5 John Duffy Case Study
16.1.6 Biological Explanations 1
16.1.7 Biological Explanations 2
16.1.8 Evaluation of the Biological Explanation
16.1.9 Cognitive Explanations
16.1.10 Moral Reasoning
16.1.11 Psychodynamic Explanation 1
16.1.12 Psychodynamic Explanation 2
16.1.13 End of Topic Test - Forensic Psychology 1
16.1.14 Differential Association Theory
16.1.15 Custodial Sentencing
16.1.16 Effects of Prison
16.1.17 Evaluation of the Effects of Prison
16.1.18 Recidivism
16.1.19 Behavioural Treatments & Therapies
16.1.20 Effectiveness of Behavioural Treatments
16.1.21 Restorative Justice
16.1.22 End of Topic Test - Forensic Psychology 2
17 Option 3: Addiction (A2 only)
17.1 Addiction (A2 only)
17.1.1 Definition
17.1.2 Brain Neurochemistry Explanation
17.1.3 Learning Theory Explanation
17.1.4 Evaluation of a Learning Theory Explanation
17.1.5 Cognitive Bias
17.1.6 Griffiths on Cognitive Bias
17.1.7 Evaluation of Cognitive Theory (A2 only)
17.1.8 End of Topic Test - Addiction 1
17.1.9 Gambling Addiction & Learning Theory
17.1.10 Social Influences on Addiction 1
17.1.11 Social Influences on Addiction 2
17.1.12 Personal Influences on Addiction
17.1.13 Genetic Explanations of Addiction
17.1.14 End of Topic Test - Addiction 2
17.2 Treating Addiction (A2 only)
17.2.1 Drug Therapy
17.2.2 Behavioural Interventions
17.2.3 Cognitive Behavioural Therapy
17.2.4 Theory of Reasoned Action
17.2.5 Theory of Planned Behaviour
17.2.6 Six Stage Model of Behaviour Change
17.2.7 End of Topic Test - Treating Addiction
Jump to other topics
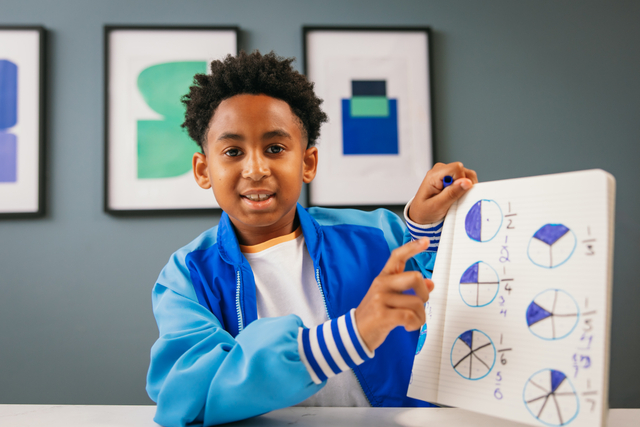
Unlock your full potential with GoStudent tutoring
Affordable 1:1 tutoring from the comfort of your home
Tutors are matched to your specific learning needs
30+ school subjects covered
The Working Memory Model 2
Explanations for Forgetting
The Working Memory Model, Baddeley And Hitch (1974)
March 5, 2021 - paper 1 introductory topics in psychology | memory.
Description, AO1 The Working Memory Model

Baddeley & Hitch’s (1974) Working Memory Model (WMM) arose out of criticisms aimed at the Multi-Store Model (MSM), particularly the idea that STM was a unitary store to test this Baddeley and Hitch devised the dual-task technique.
Research: D’Esposito et al found using MRI scans the prefrontal cortex was activated when verbal and spatial tasks were preformed simultaneously but not when performed separately, suggesting the brain area indicates the working of the CE.
Evaluation, A03 Little is known about the CE, therefore it is very difficult to know exactly what its role is in memory.
(4) Episodic buffer:
(2) Point: Support for the working memory model comes from the case study of KF. Evidence: For example, KF suffered a motorcycle accident and was left with considerable damage to his memory. His short-term forgetting of auditory information was greater than for visual information, suggesting that his memory damage was restricted to the phonological loop. Evaluation: This is a strength because it demonstrates that it’s possible to damage just part of the short-term memory, which can be accounted for by the WMM, as if all short-term memories were stored in the same place KF’s entire STM would be damaged.
Weaknesses:
To learn about the alternative model of memory, The Multi-Store Model of memory, click here.

psychologyrocks
Working memory model, baddeley and hitch (1974, 2000).
- an active processor with a number of different components which work together to allow verbal reasoning, text comprehension, mental arithmetic and other complex tasks
- a mental workspace
where we can bring items to mind from LTM or information that has been attended to from the outside world , manipulate it and work with it temporarily.
Relevant study for SAQ: Robbins et al. (1996)
Robbinsetal1996
This would also make a great IA if adapted slightly to make it simpler, i.e. smaller board? less pieces? Simplified scoring system and just the VS suppression task plus control.
Case studies to evaluate WMM
KF supports the idea of the Visuo-Spatial sketchpad separate from Verbal STM (against MSM and for WMM)
LH who had good spatial but poor visual memory for objects and faces (Farah: farah1988case study of LH; damaged visual cache) and MV who had poor spatial (damaged inner scribe) but good visual memory (Carlesimo) supporting separations to the visuo-spatial sketchpad into visual cache and inner scribe.
Describe working memory model with reference to one research. (9) SAQ WMM and Robbins
Describe one model of memory with reference to one research study. (9)
Evaluate one of model of memory. (22)
Contrast two models of memory with reference to research evidence. (22)
Share this:
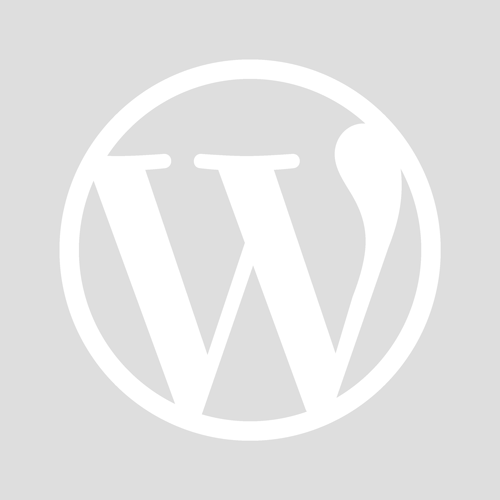
- Already have a WordPress.com account? Log in now.
- Subscribe Subscribed
- Copy shortlink
- Report this content
- View post in Reader
- Manage subscriptions
- Collapse this bar
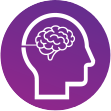
Personalised lessons and regular feedback to ensure you ace your exams! Book a free consultation today
Gain hands-on experience of how physics is used in different fields. Experience life as a uni student and boost your university application with our summer programme!
- Revision notes >
- A-Level Psychology Revision Notes >
The working memory model -A-Level Psychology
The working memory model:.
-explains how short term memory is organised and functions
Table of Contents
-is concerned with part of the brain that is active when we are temporarily storing and manipulating information
Central executive:
-monitors incoming data
-coordinates the activities of the 3 slave subsystems in memory.
-directs attention to a specific slave system.
-has a very limited processing capacity so is unable to store data.
The phonological loop :
-deals with auditory information
– preserves the order in which information arrives.
-It has two subdivisions: the phonological store which stores words you hear and the articulatory process which allows maintenance rehearsal (inner voice).
The Visuo-spatial sketchpad:
-stores visual and spatial information
-has a limited capacity.
– has two subdivisions: the visual cache which stores visual data and the inner scribe which records the arrangements of objects in the visual field
The episodic buffer:
-a temporary store for information that integrates visual,spatial and verbal information processed by the other stores into a single memory and maintains a sense of time sequencing.It also links working memory to LTM and wider cognitive processes such as perception.
-also has a limited capacity
Evaluation:
Clinical evidence- Shallice and Warrington (1970) carried out a case study on patient KF who had brain damage.The patient had poor STM ability and struggled to process auditory material presented verbally but could process visual information presented visually.This suggests that his phonological loop had been damaged leaving the other areas of memory intact thus supporting the existence of a separate visual and acoustic store which are present in the WMM called the phonological loop and the visuo-spatail sketchpad
Constricted to only STM-There is no explanation of LTM so therefore it is not a complete accurate model of memory,so has limited application into the everyday processes of human memory .This is a limitation because it considers how the WMM is unable to convey the full process of memory.
The Working Memory Model is a cognitive theory that explains how our short-term memory works. It was proposed by Baddeley and Hitch in 1974 and has been refined over the years.
The Working Memory Model has three main components: the phonological loop, the visuospatial sketchpad, and the central executive.
The phonological loop is responsible for the storage and manipulation of auditory information, such as sounds and words.
The visuospatial sketchpad is responsible for the storage and manipulation of visual and spatial information, such as images and locations.
The central executive is responsible for coordinating the activities of the phonological loop and the visuospatial sketchpad, as well as for controlling attention and making decisions.
The episodic buffer is a component that was added to the Working Memory Model later on. It is responsible for integrating information from the phonological loop, visuospatial sketchpad, and long-term memory to create a complete representation of an event or experience.
The Working Memory Model proposes that short-term memory is made up of several different components that work together, whereas the Multi-Store Model proposes that short-term memory and long-term memory are separate stores.
The Working Memory Model can be applied in many areas of everyday life, such as learning, problem-solving, decision-making, and communication. Understanding the limitations and functions of working memory can help students develop effective study strategies and improve their performance in exams.
Some limitations of the Working Memory Model include the lack of clarity around the exact role of the central executive and the episodic buffer, as well as the difficulty in measuring and manipulating working memory in experiments.
Research on the Working Memory Model has evolved over time to include new technologies and methodologies, such as brain imaging and computational modeling. This has led to a better understanding of the neural mechanisms and cognitive processes underlying working memory.
Still got a question? Leave a comment
Leave a comment, cancel reply.
Save my name, email, and website in this browser for the next time I comment.
Get an A* in A-Level Psychology
- A-Level Psychology Past Papers
Boost your A-Level Psychology Performance
Get a 9 in A-Level Psychology with our Trusted 1-1 Tutors. Enquire now.
Gain hands-on experience of how physics is used in different fields. Boost your university application with our summer programme!
Let's get acquainted ? What is your name?
Nice to meet you, {{name}} what is your preferred e-mail address, nice to meet you, {{name}} what is your preferred phone number, what is your preferred phone number, just to check, what are you interested in, when should we call you.
It would be great to have a 15m chat to discuss a personalised plan and answer any questions
What time works best for you? (UK Time)
Pick a time-slot that works best for you ?
How many hours of 1-1 tutoring are you looking for?
My whatsapp number is..., for our safeguarding policy, please confirm....
Please provide the mobile number of a guardian/parent
Which online course are you interested in?
What is your query, you can apply for a bursary by clicking this link, sure, what is your query, thank you for your response. we will aim to get back to you within 12-24 hours., lock in a 2 hour 1-1 tutoring lesson now.
If you're ready and keen to get started click the button below to book your first 2 hour 1-1 tutoring lesson with us. Connect with a tutor from a university of your choice in minutes. (Use FAST5 to get 5% Off!)
Up Learn – A Level Psychology (AQA) – Memory
What is the working memory model .
There are two main features of the working memory model… Firstly, the working memory model sees short-term memory as an active store that holds information while it’s being ‘worked on’ and also enables us to manipulate the information. Secondly, the working memory model says that there are multiple components to working memory.
A*/A guaranteed or your money back
Really? Yes, really. Find out more about our A*/A Guarantee below.
Want to see the whole course?
No payment info required!
More videos on The Working Memory Model
Introduction (free trial)
Limitations of the Multi-store Model: Patient KF Case Study
Limitations of the Multi-store Model: Short-term Memory Stores (free trial)
Limitations of the Multi-store Model: the Role of Rehearsal (free trial)
Progress Quiz: Limitations of the Multi-store Model (free trial)
The Working Memory Model
Phonological Loop (free trial)
Sub-components of the Phonological Loop (free trial)
Rehearsal and the Word-length Effect (free trial)
Visuo-spatial Sketchpad (free trial)
Sub-components of the Visuo-spatial Sketchpad (free trial)
Episodic Buffer (free trial)
What is Memory?
Types of memory, types of long-term memory, memory accuracy: how good is our memory, exam questions: memory.
Last time, we saw three limitations of the multi-store model…
Last time, we saw three limitations of the multi-store model, which were:
First, the model isn’t supported by findings from case studies. The model predicts that damage to the short-term memory store will lead to problems with long-term memory… but case studies show that people can have damage to the short-term memory store without damage to long term memory.
Second, the model is oversimplified; it says that there is only one type of short-term memory… but again, patients show that this isn’t true!
And finally, the model puts too much emphasis on the role of rehearsal in the transfer of information to long-term memory… when there are other ways that information can be transferred to long-term memory.
Patients like KF challenged the multi-store model of memory…
…So, to explain what happened in cases like KF’s accident, psychologists needed a new and improved memory model!
In 1974, researchers called Alan Baddeley and Graham Hitch rose to the challenge and came up with the working memory model, which is still accepted to this day as the best model of how memory works!
So, what does the working memory model say?
Rather than totally replacing the multi-store model, you can think of the working memory model as an extension of the multi-store model, that explains the short-term memory store in more detail…
There are two main new features of Baddeley and Hitch’s improved short-term memory store…
First, in Atkinson and Shiffrin’s multi-store memory model, they saw short-term memory as being a passive store that just holds information temporarily until it is transferred into long-term memory.
But Baddeley and Hitch didn’t agree with this.
For instance, if you’re having a conversation with someone and you need to hold in mind what someone is saying to you…
You’re not just passively holding that information in mind.
You have to actively interpret what’s being said and use it to select an appropriate reply…
So Baddeley and Hitch said that short term memory isn’t just a passive store; it’s an active store that enables us to manipulate pieces of information.
And so, to emphasise the active nature of short-term memory, Baddeley and Hitch called their short-term memory store the working memory store.
So the first main feature of the working memory model sees short-term memory as an active store.
The second main feature of the working memory model is that it is a multi-store model.
We’ve seen that Atkinson and Shiffrin’s multi-store model said…
We’ve seen that Atkinson and Shiffrin’s multi-store model said that there was only one short-term memory store.
But evidence from patients like KF suggested that this is not the case, as KF struggled to keep verbal information like word lists in short-term memory, but he could manage to retain visual information in short-term memory…
So, the second main feature of Baddeley and Hitch’s model was that there are multiple different components to working memory, that all store different types of memory, and that could all be separately impaired.
These multiple short-term memory stores explain why patients like KF can have impaired short-term memory without having long-term memory damage:
If only one of the working memory stores is damaged, information can still be held in one of the other working memory stores… meaning that it can then still be transferred into long-term memory!
Baddeley and Hitch suggested that there were four different components to working memory…
We’ll look at the different components of the working memory model next.
But first, to sum up…
So, to sum it up, there are two main features of the working memory model…
Firstly, the working memory model sees short-term memory as an active store that holds information while it’s being ‘worked on’ and also enables us to manipulate the information.
Secondly, the working memory model says that there are multiple components to working memory.
Multi-Store Memory Model: Atkinson and Shiffrin
Saul McLeod, PhD
Editor-in-Chief for Simply Psychology
BSc (Hons) Psychology, MRes, PhD, University of Manchester
Saul McLeod, PhD., is a qualified psychology teacher with over 18 years of experience in further and higher education. He has been published in peer-reviewed journals, including the Journal of Clinical Psychology.
Learn about our Editorial Process
Olivia Guy-Evans, MSc
Associate Editor for Simply Psychology
BSc (Hons) Psychology, MSc Psychology of Education
Olivia Guy-Evans is a writer and associate editor for Simply Psychology. She has previously worked in healthcare and educational sectors.
On This Page:
What is the Multi-Store Model?
- The multi-store model is an explanation of memory proposed by Atkinson and Shiffrin which assumes there are three unitary (separate) memory stores, and that information is transferred between these stores in a linear sequence.
- The three main stores are the sensory memory, short-term memory (STM) and long-term memory (LTM).
- Each of the memory stores differs in the way information is processed (encoding), how much information can be stored (capacity), and for how long (duration).
- Information passes from store to store in a linear way, and has been described as an information processing model (like a computer) with an input, process and output.
- Information is detected by the sense organs and enters the sensory memory , which stores a fleeting impression of sensory stimuli. If attended to this information enters the STM and if the information is given meaning (elaborative rehearsal) it is passed on to the LTM
The multi-store model of memory (also known as the modal model) was proposed by Richard Atkinson and Richard Shiffrin (1968) and is a structural model. They proposed that memory consisted of three stores: a sensory register, short-term memory (STM) and long-term memory (LTM).

The Memory Stores
Each store is a unitary structure and has its own characteristics in terms of encoding, capacity and duration.
Encoding is the way information is changed so that it can be stored in the memory. There are three main ways in which information can be encoded (changed):
1. visual (picture),
2. acoustic (sound),
3. semantic (meaning).
Capacity concerns how much information can be stored.
Duration refers to the period of time information can last in the memory stores.
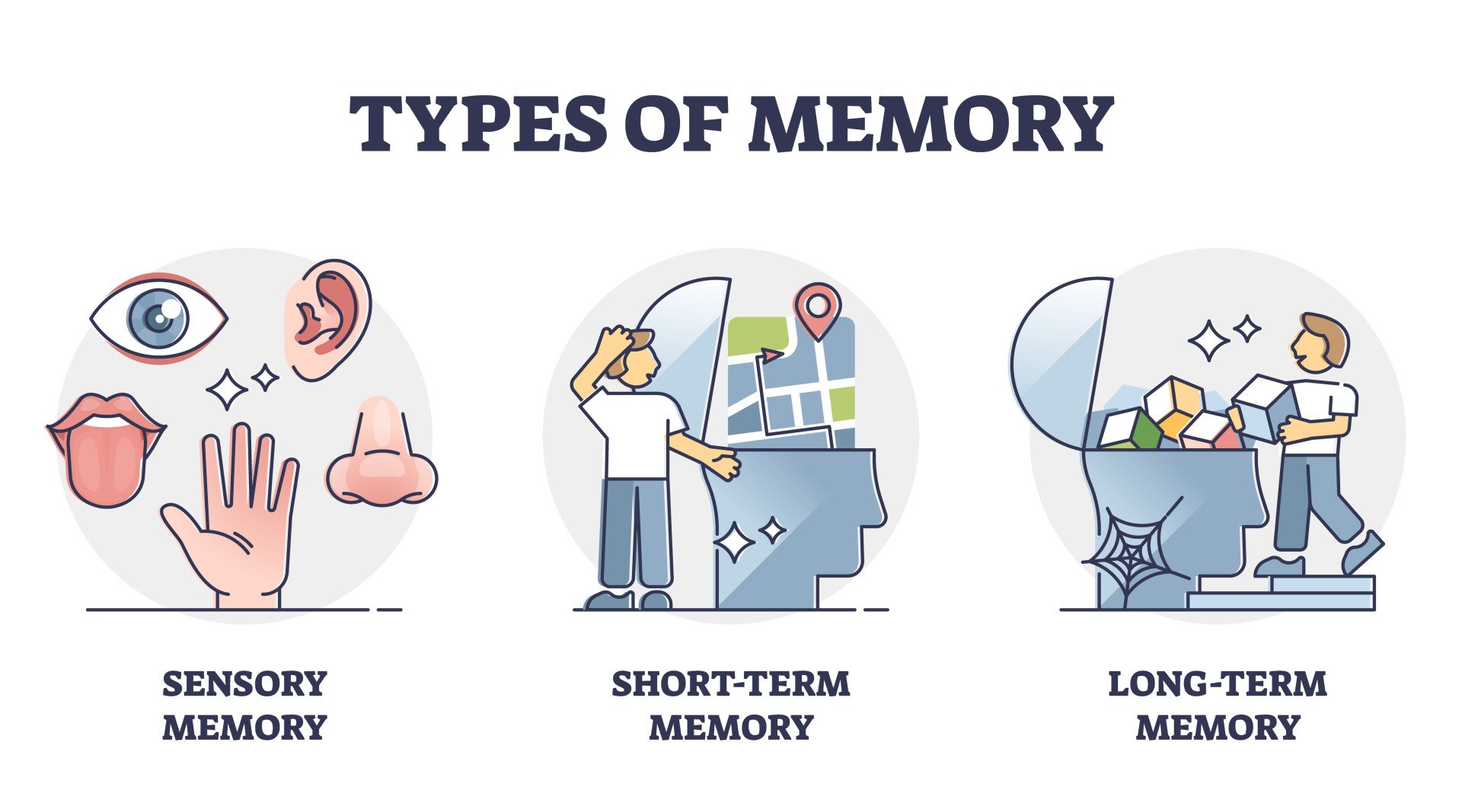
Sensory Memory
• Duration: ¼ to ½ second
• Capacity: all sensory experience (v. larger capacity)
• Encoding: sense specific (e.g. different stores for each sense)
The sensory stores are constantly receiving information but most of this receives no attention and remains in the sensory register for a very brief period.
In the sensory memory store , information arrives from the 5 senses such as sight (visual information), sounds and touch. The sensory memory store has a large capacity but a very brief duration, it can encode information from any of the senses and most of the information is lost through decay.
Attention is the first step in remembering something, if a person’s attention is focused on one of the sensory stores then the data is transferred to STM.
Short Term Memory
• Duration: 0-18 seconds
• Capacity: 7 +/- 2 items
• Encoding: mainly auditory
The short-term memory store has a duration of up to 30 seconds, has a capacity of 7+/-2 chunks and mainly encodes information acoustically. Information is lost through displacement or decay.
Maintenance rehearsal is the process of verbally or mentally repeating information, which allows the duration of short-term memory to be extended beyond 30 seconds. An example of maintenance rehearsal would be remembering a phone number only long enough to make the phone call.
This type of rehearsal usually involves repeating information without thinking about its meaning or connecting it to other information.
Continual rehearsal “regenerates” or “renews” the information in the memory trace, thus making it a stronger memory when transferred to the Long Term store.
If maintenance rehearsal (repetition) does not occur, then information is forgotten, and lost from short term memory through the processes of displacement or decay.
Long Term Memory
• Duration: Unlimited
• Capacity: Unlimited
• Encoding: Mainly Semantic (but can be visual and auditory)
Long-term memory store has unlimited capacity and duration and encodes information semantically. Information can be recalled from LTM back into the STM when it is needed.
If the information is given meaning (elaborative rehearsal) it is passed on to the LTM.
Elaborative rehearsal involves the process of linking new information in a meaningful way with information already stored in long-term memory. For example,
you could learn the lines in a play by relating the dialogue and behavior of your character to similar personal experiences you remember.
Elaborative rehearsal is more effective than maintenance rehearsal for remembering new information as it helps to ensure that information is encoded well. It is a deeper level of information-processing.
Key Studies
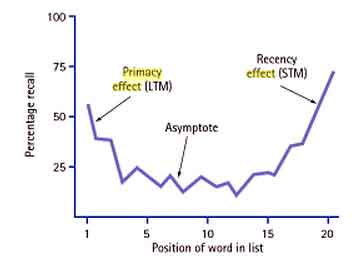
Glanzer and Cunitz showed that when participants are presented with a list of words, they tend to remember the first few and last few words and are more likely to forget those in the middle of the list, i.e. the serial position effect.
This supports the existence of separate LTM and STM stores because they observed a primacy and recency effect.
Words early on in the list were put into long term memory (primacy effect) because the person has time to rehearse the word, and words from the end went into short term memory (recency effect).
Other compelling evidence to support this distinction between STM and LTM is the case of KF (Shallice & Warrington, 1977) who had been in a motorcycle crash where he had sustained brain damage.
His LTM seemed to be unaffected but he was only able to recall the last bit of information he had heard in his STM.
Critical Evaluation
One strength of the multistore model is that is gives us a good understanding of the structure and process of the STM. This is good because this allows researchers to expand on this model.
This means researchers can do experiments to improve on this model and make it more valid and they can prove what the stores actually do. Therefore, the model is influential as it has generated a lot of research into memory.
Many memory studies provide evidence to support the distinction between STM and LTM (in terms of encoding, duration and capacity). The model can account for primacy & recency effects .
The case of HM also supports the MSM as he was unable to encode new long-term memories after surgery during which his hippocampus was removed but his STM was unaffected.
He has remembered little of personal (death of mother and father) or public events (Watergate, Vietnam War) that have occurred over the last 45 years. However his short-term memory remains intact.This supports the view that the LTM and the STM are two separate stores.
The model is oversimplified, in particular when it suggests that both short-term and long-term memory each operate in a single, uniform fashion. We now know is this not the case.
It has now become apparent that both short-term and long-term memory are more complicated that previously thought. For example, the Working Model of Memory proposed by Baddeley and Hitch (1974) showed that short term memory is more than just one simple unitary store and comprises different components (e.g. central executive, Visuospatial etc.).
In the case of long-term memory, it is unlikely that different kinds of knowledge, such as remembering how to play a computer game, the rules of subtraction and remembering what we did yesterday are all stored within a single, long-term memory store.
Indeed different types of long-term memory have been identified, namely episodic (memories of events), procedural (knowledge of how to do things) and semantic (general knowledge).
Rehearsal is considered a too simple explanation to account for the transfer of information from STM to LTM. For instance, the model ignores factors such as motivation, effect and strategy (e.g. mnemonics) which underpin learning.
Also, rehearsal is not essential to transfer information into LTM. For example, why are we able to recall information which we did not rehearse (e.g. swimming) yet unable to recall information which we have rehearsed (e.g. reading your notes while revising).
Therefore, the role of rehearsal as a means of transferring from STM to LTM is much less important than Atkinson and Shiffrin (1968) claimed in their model.
The models main emphasis was on structure and tends to neglect the process elements of memory (e.g. it only focuses on attention and maintenance rehearsal). For example, elaboration rehearsal leads to recall of information than just maintenance rehearsal.
Elaboration rehearsal involves a more meaningful analysis (e.g. images, thinking, associations etc.) of information and leads to better recall. For example, giving words a meaning or linking them with previous knowledge. These limitations are dealt with by the levels of processing model (Craik, & Lockhart, 1972).
Note: although rehearsal was initially described by Atkinson and Shiffrin as maintenance rehearsal (repetition of information), Shiffrin later suggested that rehearsal could be elaborative (Raaijmakers, & Shiffrin, 2003).
The multi store model has been criticized for being a passive/one way/linear model.
Atkinson, R. C., & Shiffrin, R. M. (1968). Chapter: Human memory: A proposed system and its control processes. In Spence, K. W., & Spence, J. T. The psychology of learning and motivation (Volume 2). New York: Academic Press. pp. 89–195.
Baddeley, A .D., & Hitch, G. (1974). Working memory. In G.H. Bower (Ed.), The psychology of learning and motivation: Advances in research and theory (Vol. 8, pp. 47–89). New York: Academic Press.
Craik, F. I. M., & Lockhart, R. S. (1972). Levels of processing: A framework for memory research. Journal of Verbal Learning and Verbal behavior, 11, 671-684.
Raaijmakers, J.G.W. & Shiffrin, R.M. (2003). Models versus descriptions: Real differences and langiage differences . behavioral and Brain Sciences , 26, 753.
Shallice, T., & Warrington, E. K. (1977). Auditory-verbal short-term memory impairment and conduction aphasia. Brain and Language, 4(4) , 479-491.

Skip to content
Get Revising
Join get revising, already a member.

Evaluating the working memory model
- Created by: maddieecarr
- Created on: 13-06-21 14:11
Overall the WMM is more complete than the MSM, but has its own strengths and limitations. |
No comments have yet been made
Similar Psychology resources:
Memory mind map 0.0 / 5
Memory Mind Map 5.0 / 5 based on 1 rating
Evaluation of the Working Memory Model - Remembering and Forgetting 0.0 / 5
Memory worksheet 0.0 / 5
Memory - Things to know 0.0 / 5
The Multi-store Model and The Working Memory Model 5.0 / 5 based on 3 ratings
Multi store model evaluation 0.0 / 5
MEMORY - Evaluation (A03) of the Multi-Store Model (MSM) 4.0 / 5 based on 1 rating
Working memory model evaluation 0.0 / 5
Working Memory Mode 0.0 / 5
Thank you for visiting nature.com. You are using a browser version with limited support for CSS. To obtain the best experience, we recommend you use a more up to date browser (or turn off compatibility mode in Internet Explorer). In the meantime, to ensure continued support, we are displaying the site without styles and JavaScript.
- View all journals
- Explore content
- About the journal
- Publish with us
- Sign up for alerts
- Open access
- Published: 19 September 2024
Maintenance and transformation of representational formats during working memory prioritization
- Daniel Pacheco-Estefan ORCID: orcid.org/0000-0003-2253-5172 1 na1 ,
- Marie-Christin Fellner 1 na1 ,
- Lukas Kunz ORCID: orcid.org/0000-0002-0665-7703 2 ,
- Hui Zhang 1 ,
- Peter Reinacher ORCID: orcid.org/0000-0003-1691-546X 3 , 4 ,
- Charlotte Roy 5 ,
- Armin Brandt 5 ,
- Andreas Schulze-Bonhage ORCID: orcid.org/0000-0003-2382-0506 5 ,
- Linglin Yang 6 ,
- Shuang Wang 7 ,
- Jing Liu 8 ,
- Gui Xue ORCID: orcid.org/0000-0001-7891-8151 9 &
- Nikolai Axmacher ORCID: orcid.org/0000-0002-0475-6492 1 , 9
Nature Communications volume 15 , Article number: 8234 ( 2024 ) Cite this article
981 Accesses
28 Altmetric
Metrics details
- Cognitive control
- Human behaviour
- Working memory
Visual working memory depends on both material-specific brain areas in the ventral visual stream (VVS) that support the maintenance of stimulus representations and on regions in the prefrontal cortex (PFC) that control these representations. How executive control prioritizes working memory contents and whether this affects their representational formats remains an open question, however. Here, we analyzed intracranial EEG (iEEG) recordings in epilepsy patients with electrodes in VVS and PFC who performed a multi-item working memory task involving a retro-cue. We employed Representational Similarity Analysis (RSA) with various Deep Neural Network (DNN) architectures to investigate the representational format of prioritized VWM content. While recurrent DNN representations matched PFC representations in the beta band (15–29 Hz) following the retro-cue, they corresponded to VVS representations in a lower frequency range (3–14 Hz) towards the end of the maintenance period. Our findings highlight the distinct coding schemes and representational formats of prioritized content in VVS and PFC.
Similar content being viewed by others
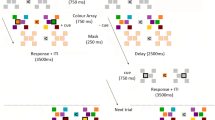
Recruitment of a long-term memory supporting neural network during repeated maintenance of a multi-item abstract visual image in working memory
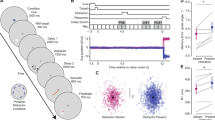
Working memory representations in visual cortex mediate distraction effects
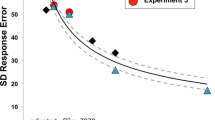
Electrophysiological correlates of the flexible allocation of visual working memory resources
Introduction.
Visual working memory (VWM) refers to the ability to store visual information for a short period of time and to flexibly manipulate this information according to task demands. One essential aspect of VWM memory is prioritization, i.e., the ability to selectively allocate attention to particular features or items depending on behavioral or cognitive requirements. Influential theories have proposed that WM prioritization entails the transformation of maintained representations from a purely mnemonic to a task-optimized state 1 , 2 . On a neurophysiological level, these accounts predict that working memory prioritization involves a task-dependent transformation of representational patterns in executive control areas which can be disentangled from a mnemonic coding scheme that maintains perceptual stimulus features in sensory brain regions 1 , 3 , 4 , 5 . Here we set out to test this prediction. We analyzed the representational format of VWM stimuli using electrophysiological recordings in human epilepsy patients implanted with electrodes in ventral visual stream (VVS) and/or prefrontal cortex (PFC).
There is now abundant evidence on the neural correlates of VWM control processes in humans and animals 6 , 7 , 8 , 9 , 10 . Early studies focused on prioritization of to-be-encoded items, using paradigms in which participants were asked to selectively attend to particular items before these items were shown (e.g., refs. 6 , 11 , 12 , 13 , 14 ). More recent investigations often employed retrospective cueing paradigms, in which prioritization is applied to information after its encoding into VWM 10 , 15 , 16 , 17 , 18 . These studies revealed that prefrontal and parietal regions which underlie the allocation of attention during perception are also engaged in the prioritization of items in VWM 2 , 7 , 8 , 9 , 19 , 20 , 21 , 22 . Notably, a recent meta-analysis observed selective responses to retro-cues but not to cues that allocate attention prior to item encoding in VWM in various prefrontal areas 1 , 23 . This literature suggests that prioritization affects VWM representations in the PFC, yet this prediction has not been tested experimentally in humans.
The critical role of the PFC in WM prioritization is commonly believed to depend on dynamic recurrent computations. A classical model of WM suggests that persistent activity depends on reverberatory excitation within a local recurrent neural network 24 , 25 . Computational studies have shown that recurrence is crucial for the selection and integration of task-relevant features in the PFC 26 , the integration of working memory and planning 27 , the flexibility of WM and the avoidance of interference in the presence of competing representations 28 , and—most importantly for our study—WM prioritization 29 , 30 . Recurrent computations might be particularly relevant for selective attention to specific features or items in WM because they enable the stabilization of reverberating activity in attractor states that modulate the excitability of assemblies which represent prioritized contents 24 , 25 , 31 . In addition to their theorized role in PFC prioritization, recurrent computations have been proposed to be critical for information processing in the VVS during visual perception 32 , 33 , and for offline ‘generation’ of stimuli during visual imagery 34 . However, a specific role of recurrency in the VVS for VWM maintenance has not been previously investigated.
In addition (and possibly related) to the relevance of recurrent computations, theories have emphasized the important role of brain oscillations for VWM, in particular for the prioritization process. Oscillatory activity in the gamma frequency range (50–120 Hz) is thought to convey bottom-up information during VWM encoding, while oscillations at beta frequency (20–35 Hz) are supposed to provide top-down control over VWM contents 4 , 35 , 36 , 37 , 38 . The significance of these oscillatory patterns has been validated experimentally in a series of studies in macaques 4 , 20 , 35 . Furthermore, a recent study in humans confirmed the crucial role of gamma-band activity (30–75 Hz) for conveying bottom-up information from lower-level visual areas to regions processing higher-level information 36 . In addition to their role in top-down control over WM content, several studies have now associated beta oscillations with the reactivation of stimulus-specific activity during the VWM prioritization process. Content-specific beta activity has been shown to carry information about internalized task rules 39 , stimulus categories 40 , 41 , 42 , scalar magnitudes 43 , 44 and perceptual decisions 45 ; for review, see ref. 46 . These studies highlight the role of beta oscillations in encoding task-relevant stimulus properties.
In humans, intracranial EEG (iEEG) recordings in epilepsy patients have been used to investigate the neurophysiological patterns underlying content-specific memory representations. This research has employed multivariate analysis techniques, such as pattern classification and representational similarity analysis (RSA), to identify representations of specific stimuli 47 , 48 . Studies have demonstrated that frequency-specific representations in the gamma, beta and theta (3–8 Hz) frequency bands contain item- and category-specific information, playing a crucial role in episodic memory retrieval 49 , 50 . In addition to identifying the relevant oscillatory frequencies that carry representational content during visual perception and episodic memory, recent iEEG studies have investigated the ‘formats’ of VWM representations. This research employed deep neural networks (DNNs) to investigate how different aspects of natural images are represented in the brain during mnemonic processing. These studies assume that mnemonic representations require specialized circuits for processing distinct aspects (or formats) of natural images, from low-level sensory features to higher-level contents and conceptual/semantic information 51 , 52 , 53 , 54 , 55 , 56 . Indeed, several studies assessed the different representational formats during VWM encoding and maintenance and demonstrated substantial transformations of VWM representations into a format that aligns with late layers of a convolutional DNN 57 , 58 . While these results and methodological advancements have provided valuable insights into the format of VWM representations, no study so far has investigated the representational transformations that accompany VWM prioritization in humans. Thus, whether the prioritization of VWM representations involves a change in the representational format of the stored content and distinct coding schemes of attended (i.e., task-relevant) items is currently unknown.
Here, we leveraged the heuristic potential of DNNs as models of visual representation, the flexibility of RSA, and the high spatiotemporal resolution of iEEG to investigate this topic. We analyzed electrophysiological activity from VVS and PFC while patients performed a multi-item VWM paradigm involving a retro-cue. Participants encoded a sequence of three images and were then prompted by a retro-cue to maintain either one of these items or all items (Fig. 1A ; Methods). The objects belonged to six categories, each containing ten exemplars (60 images in total). With the exception of the behavioral data, we only analyzed activity during the single item condition in this study, given our focus on the prioritization process. This experimental design allowed us to evaluate how information about specific contents is represented in the brain during initial encoding and how it is transformed due to the retro-cue, both in terms of representational formats and regarding the frequencies of brain oscillations in VVS (438 electrodes) and PFC (146 electrodes; Fig. 1B ). We hypothesized that frequency-specific representations would reflect bottom-up storage and top-down information transfer, respectively, with a particular role of gamma and beta oscillations 4 , 35 , 36 , 37 , 38 . Specifically, we predicted that oscillatory PFC activity in the beta frequency range may reflect representational transformations due to top-down control following the retro-cue 4 . In addition, we expected recurrent convolutional architectures to better explain representations than feedforward DNNs during VWM maintenance 25 , 28 , 32 , 33 , 59 , 60 .
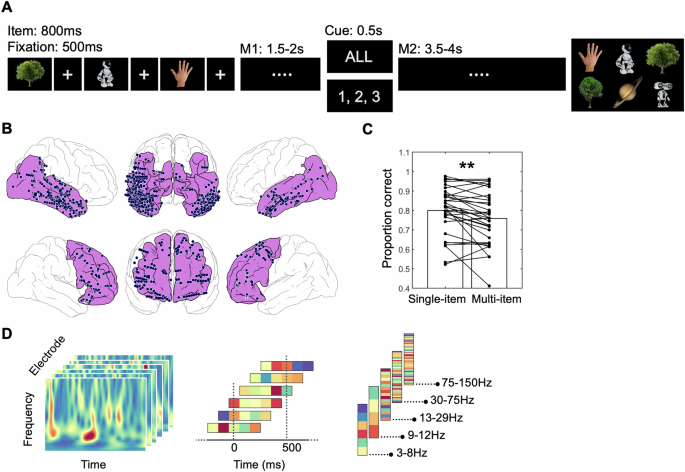
A Participants encoded a sequence of 3 images of natural objects and were asked to remember this content during two subsequent maintenance periods that were separated by a retro-cue (M1 – retro-cue – M2). The cue prompted participants to either selectively maintain items at particular list positions (single-item trials, “1, 2, 3” in the figure) or to maintain all items in their order of presentation (multi-item trials, “All” in the figure). During the probe, six items were presented, which included all encoded items and three exemplars from previously presented or novel categories (Methods). The figure displays representative images very similar to those shown during the experiment, in compliance with a CC BY license ( https://creativecommons.org/licenses/by/4.0/ ). B Electrode implantation included 438 electrodes in the ventral visual stream (VVS, N = 28 participants; top) and 146 electrodes in the prefrontal cortex (PFC, N = 16 participants; bottom). Cortical areas included in each region are highlighted in pink. C Behavioral performance was significantly higher for single as compared to multi-item trials in our patient group (paired t -test, two-sided, n = 32). D Left: Representational patterns in the RSA analyses included neural activity across electrodes, time points (5 time points in each 500 ms window), and frequencies. Spearman correlations were computed in windows of 500 ms, incrementing in 100 ms steps (middle). Analyses were performed in individual frequencies in the 3-150 Hz range in the model-based RSA analyses, and within different frequency bands (theta, alpha, beta, low-gamma, high-gamma) in the contrast-based analyses. Source data are provided as a Source Data file. Image sources ( A ): Tree 1: https://www.istockphoto.com/en/portfolio/YutthasartYanakornsiri ; Tree 2: https://www.istockphoto.com/en/portfolio/Coldimages ; Robot 1 and 2: https://www.istockphoto.com/en/portfolio/Ociacia ; Hand: https://www.istockphoto.com/en/portfolio/Hanis ; Planet: https://www.istockphoto.com/en/portfolio/GeorgeManga . ** p < 0.01.
Behavioral results
Successful prioritization in the single-item condition should result in better performance than in the multi-item trials. Indeed, we found that participants performed significantly better in single-item trials (proportion correct trials: 0.8 ± 0.12) than in the multi-item condition (0.75 ± 0.13; t(31) = 3.21, p = 0.0031; Fig. 1C ). This suggests that participants followed instructions and benefited from prioritizing task-relevant representations in the single-item trials.
Maintenance and transformation of category-specific representations
We investigated the electrophysiological patterns supporting the representation of category-specific information in VVS and PFC. As a first approach, we assessed the presence of categorical representations, employing RSA and a simple model of category information (Fig. 2A ). We constructed an item-by-item representational similarity matrix (RSM) reflecting the hypothesis that items of the same category would elicit more similar patterns of brain activity compared to items of different categories (Fig. 2A ). We correlated this model RSM with temporally resolved neural RSMs (windows of 500 ms, overlapping by 400 ms). Representational patterns included power values across electrodes (16.85 ± 8.92 electrodes in VVS, 9.73 ± 11.1 in PFC; Mean ± STD) and time points (5 time points of 100 ms in each time window; Fig. 1D , Methods) and were analyzed separately in each of 52 different frequencies between 3 and 150 Hz. To determine the similarity between feature vectors, we used Spearman’s Rho 50 , 57 .
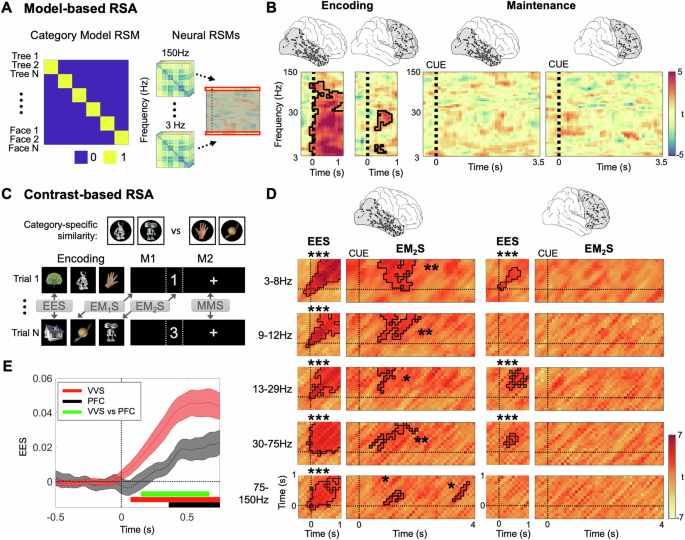
A Model-based RSA. Representational similarity matrix (RSM) reflecting the hypothesis that category information structures the representational geometry of stimuli (left) were correlated with a time-series of neural RSMs (right) at each individual frequency. B Fit of the category model in the VVS and the PFC during the encoding (left) and maintenance (right) period. Zero indicates the onset of image presentation during encoding, and the onset of the presentation of the cue during maintenance. C Contrast-based RSA. Top: Category-specific similarity was computed by contrasting correlations between (different) items of the same category vs items of different categories. Bottom: Similarity was calculated between the encoding periods of different trials (encoding-encoding similarity, EES), between the encoding and the maintenance periods of different trials (encoding-maintenance similarity, EMS), and between maintenance periods of different trials (maintenance-maintenance similarity, MMS). Note that two types of EMS analysis were conducted for the first and second maintenance periods (EM 1 S, see Supplementary Fig 2 , and EM 2 S). D EES and EM 2 S analyses: Category contrasts for five frequency bands during encoding and maintenance in VVS (left) and PFC (right). In the EES plots, zero indicates the onset of image presentation on both time axes. In the EM 2 S plots, zero indicates image onset during encoding and retro-cue onset during maintenance, respectively. Significant differences between same and different categories were assessed using two-sided paired t -tests at each time bin. Significant time periods surviving correction for multiple comparisons using cluster-based permutation statistics are outlined in black. E Category specificity analysis at higher temporal resolution showing different latencies of effects in VVS (red) and PFC (black). Each line shows the time course of within minus between category correlations in each region (Mean ± S.E.). Horizontal bars indicate time-periods when EES values are significantly different from zero in each region, and significantly different between the two regions (green). Please note that t-maps in B and D have the same color scale, indicated in the color bar at the right of each panel. Source data are provided as a Source Data file. Images in C are published in compliance with a CC BY license ( https://creativecommons.org/licenses/by/4.0/ ). Sources: Robot 1 and 2: https://www.istockphoto.com/en/portfolio/Ociacia ; Hand: https://www.istockphoto.com/en/portfolio/Hanis ; Planet: https://www.istockphoto.com/en/portfolio/GeorgeManga . Tree 1: https://www.istockphoto.com/en/portfolio/YutthasartYanakornsiri ; House: https://www.istockphoto.com/en/portfolio/SittidhetJoollasawok . *** p < 0.001; ** p < 0.01; * p < 0.05.
Our simple category model revealed a marked presence of categorical information during encoding in the VVS. This was observed in a significant frequency cluster ranging from 3–120 Hz that started immediately after stimulus presentation and lasted for the whole encoding period (0.8 s; p = 0.001). In the PFC, we observed two clusters of significant fit in the beta (17–28 Hz; 200–800 ms; p = 0.001) and the theta frequency range (3–7 Hz; 200–600 ms; p = 0.044; Fig. 2B , left). During maintenance, we did not observe any significant fits between model and neural RSMs in either VVS or PFC (VVS: all p > 0.51; PFC: all p > 0.105; Fig. 2B , right).
The absence of fit of the category model during maintenance might be attributed to a weakening of the representations during the maintenance period—e.g., due to a decrease in signal to noise ratio—or to a rapid transformation of activity patterns during encoding 58 . To evaluate whether transformed activity patterns from encoding reoccur during the maintenance period, we performed a category-specific pattern similarity analysis (Methods). This analysis involved contrasting correlations of items belonging to the same category with correlations of items from different categories (Fig. 2C , top), both during encoding (encoding-encoding similarity; EES) and between encoding and maintenance (encoding-maintenance similarity, EMS; Fig. 2C , bottom). Notably, while the category model can track the presence of categorical representations at the level of the representational geometry of our stimuli set, the EES and EMS analyses test for re-occurrence of category-related neural activity patterns from different encoding periods. This analysis was conducted in five conventional frequency bands (theta, 3–8 Hz; alpha, 9–12 Hz; beta, 13–29 Hz; low-gamma, 30–75 Hz; high-gamma, 75–150 Hz), with electrodes, time points (including both matching and non-matching time points; see Methods), and frequencies in each band as features.
We first analyzed the timing and temporal stability of representations during encoding, using EES. Consistent with the results observed in the category model analysis, the EES analysis revealed prominent category-specific information during the encoding phase in both VVS and PFC. In the VVS, this was observed in all frequency bands (all p corr < 0.005; Fig. 2D , first column). In the PFC, category-specific information was found in the theta, beta and low-gamma frequency bands (all p corr < 0.01; Fig. 2D , third column). To examine the relative timing of the effects in greater detail, we increased the temporal resolution by shortening the sliding windows to steps of 10 ms and including all frequencies in the 3–150 Hz range as RSA features. This analysis demonstrated that categorical information reached the PFC 360 ms after stimulus presentation, i.e., 290 ms later than the VVS (Fig. 2E ). Indeed, a direct comparison of latencies showed significantly higher EES in VVS than PFC starting 150 ms after stimulus onset ( p corr = 0.007). Furthermore, in the majority of frequency bands category-specific representations were most pronounced at matching time points across trials (diagonal values in the EES analyses; Fig. 2D ) and did not generalize to other time periods, in line with theories on dynamic coding 5 , 57 .
Next, we analyzed encoding-maintenance similarity during the second maintenance period (EM 2 S) to investigate whether category-specific representations established during encoding reoccurred after the presentation of the retro-cue. In the EM 2 S analysis, we observed significant reoccurrence of category-specific information in all frequency bands in the VVS (all p corr < 0.025). These effects were transient and most pronounced within the first 2 seconds after the retro-cue (Fig. 2D , second column). In contrast, we did not observe reoccurrence of category-specific information in the PFC in any frequency band (all p corr > 0.19; Fig. 2D , fourth column), suggesting a transformation of representational formats in this region.
In several additional control analyses, we comprehensively characterized the representational formats in VVS and PFC (Supplementary Fig 1 ), evaluated the presence of representations in the Maintenance 1 period (EM 1 S analysis; Supplementary Fig 2 and Supplementary Note 1 ), investigated the representation of individual exemplars during encoding and maintenance (Supplementary Fig 3 and Supplementary Note 2 ), and evaluated differences in performance and neural representations for items encoded in different positions during encoding (EES, EM 2 S and MMS analyses; Supplementary Fig 4 and Supplementary Note 3 ).
Together, these results show the formation of category-specific representations in both VVS and (later) in PFC during encoding, but reoccurrence of encoding patterns during maintenance in the VVS only.
Representational formats of category-specific representations
Our findings presented thus far indicate maintenance of category-specific representations from encoding in the VVS that was not observed in the PFC. The absence of an effect in the PFC may be attributed to a transformation of VWM representations driven by the prioritization process. Indeed, recent behavioral 61 , neuroimaging 53 and iEEG studies 57 , 58 established a crucial role of transformed representational formats, particularly abstract representational formats devoid of specific sensory information, in VWM maintenance. Based on these insights, we hypothesized that the PFC might represent stimuli in a representational format devoid of low-level sensory information that maps to deep DNN layers during the prioritization period.
To evaluate this hypothesis, we employed different deep neural network (DNN) architectures. First, we used the feedforward DNN ‘AlexNet’ 62 that has been extensively employed to characterize neural representations of natural images during perceptual and mnemonic processes 36 , 57 , 58 , 63 , 64 , 65 , 66 , 67 , 68 , 69 , 70 . Additionally, we applied two recurrent DNNs, the BL-NET and the corNET-RT. The BL-NET consists of seven convolutional layers which include lateral recurrent connections and has previously been applied to predict human behavior, specifically reaction times, in a perceptual task 71 . The corNET-RT has a relatively shallow architecture compared to similarly performing networks for image classification and has been designed to model information processing dynamics in the primate VVS 72 . Similar to the BL-NET, corNET-RT exhibits recurrent dynamics that propagate within (but not between) layers. All 3 DNNs represent stimuli in various representational formats, ranging from low-level visual features in superficial layers to higher-level properties in deep layers. While AlexNet processes stimulus features in a single feedforward pass, the lateral recurrent connections of BL-NET and corNET-RT generate temporally evolving time-series of stimulus representations in each layer, thus capturing core properties of recurrent dynamics during WM processing in the PFC. The number of recurrent passes is fixed to 8 time-points in BL-NET, while the corNET-RT model exhibits layer-specific recurrent passes that range from 2 to 5 time points (see Methods). Following previous studies, and in order to ensure that the networks achieved stable representations of our images in each layer, we focused on the RSMs at the last time-point of each layer 73 .
We first characterized stimulus representations in different layers of AlexNet. We constructed RSMs from DNN representations by computing the similarities between all unit activations in each layer for all pairs of images (see refs. 57 , 58 ; Fig. 3A , top). For visualization, we projected the data into two-dimensional space using Multidimensional Scaling (Fig. 3A , bottom). To evaluate representational changes throughout the DNN, we correlated the RSMs between different layers. RSMs were most similar among the convolutional layers 2–5 and among the fully connected layers 6-7, while the input layer 1 and the output layer 8 exhibited the most distinct representational patterns (Fig. 3B ). We computed the Category Cluster Index (CCI; see refs. 74 , 75 ), defined as the difference in average distances of stimulus pairs from the same category vs. stimulus pairs from different categories (Fig. 3C ). CCI takes a value of 1 if clusters are exclusively built by stimuli from the same category and approaches 0 if the representational geometry shows no categorical organization. Using permutation statistics (i.e., label shuffling), we observed that CCI values were significantly higher than chance in all layers of the network (all p corr = 0.008, Bonferroni corrected for the 8 layers). Notably, we observed a four-fold increase in CCI scores from the first (CCI = 0.11) to the last layer (CCI = 0.46) of the AlexNet (Fig. 3C ). This effect was explained by both an increase of within-category correlations (average slope of linear fit across layers = 0.046; p = 0; Supplementary Fig 5 , top left), and a decrease of between-category correlations across layers (average slope across layers = −0.008; p = 0; Supplementary Fig 5 , top right).
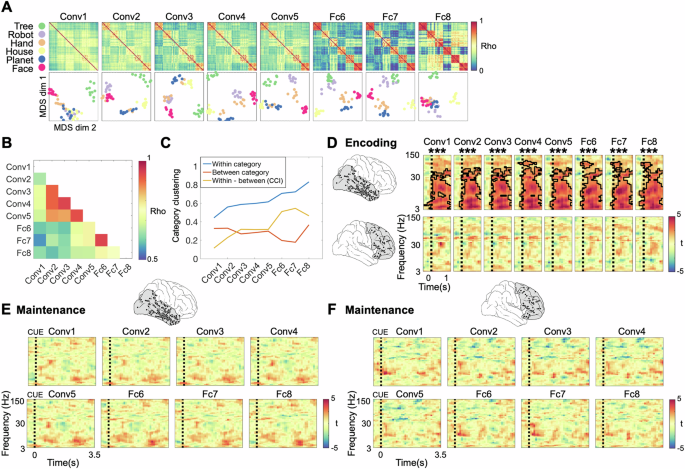
A Top: Representations in the feedforward network AlexNet. Representational Similarity Matrices (RSMs) reflecting pairwise correlations of unit activations in each layer of the network. Bottom: 2D Multidimensional Scaling (MDS) projections of RSMs at each layer, color-coded according to categories. B Representational consistency plot showing pairwise correlations (Spearman’s rho) of RSMs at each network layer. C Within-category, between-category and within-category vs. between-category correlations (i.e., Category Cluster Index, CCI) as a function of network layer. D Top: Correlations between RSMs from the DNN and neural data, for each AlexNet layer and each encoding time-frequency window in the VVS. Each time-frequency plot shows the correlation values of representations in one particular layer to neural representations. Clusters outlined in black indicate time-frequency periods where correlation values are significantly higher than zero at the group level (two-sided t -tests, Bonferroni corrected for 8 layers). Bottom: Same analysis for PFC data. Time zero in all panels indicates the onset of stimulus presentation E No matching of VVS RSMs with AlexNet RSMs during the maintenance period. Time zero indicates the onset of the cue. F Same analysis as in E for the PFC data. Color scale of all t-maps in F and G is indicated at the right of each panel. Source data are provided as a Source Data file. *** p < 0.001.
We next set out to evaluate the similarity between stimulus representations in AlexNet and neural representations in VVS and PFC. In order to characterize the frequency profile of reactivations, we performed a frequency-resolved analysis of fits between neural and DNN representations: We constructed RSM time-series for every frequency independently and grouped them into a time-frequency map of model fits (Methods). In the VVS, we found that representational geometries during encoding were captured by network representations in all layers in the 3–75 Hz range (all p corr < 0.008); Fig. 3D , top row). In layers 4 and 6–8, this effect extended into the high-gamma frequency range. Similar to the results observed in the category model analysis (Fig. 2B ), we did not observe any matching between neural and AlexNet representations during the maintenance period (all p corr > 0.056; Fig. 3E ). In the PFC, we did not observe any significant fit during either encoding (all p > 0.064; Fig. 3D , bottom row) or maintenance (all p > 0.168; Fig. 3F ).
Taken together, these results show that representations in the AlexNet are aligned with encoding representations in VVS but not PFC. Importantly, during the maintenance period neither VVS or PFC representations showed a significant fit with representations in the AlexNet network, suggesting that the format of prioritized VWM representations cannot be explained by feedforward DNNs.
We thus employed the recurrent neural networks BL-NET and corNET-RT to characterize representational formats in VVS and PFC. We first assessed the temporal evolution of network representations in the different layers of BL-NET and correlated the layer-wise RSMs between successive time points (Methods). In all layers, representations changed most prominently between intervals 1–2 and least between intervals 7–8 (Fig. 4A ). In layers 2 to 7, representations remained largely constant following time step 3, while the first layer showed more substantial dynamics until the last time interval (Fig. 4A ). Directly comparing the representations between the initial (1st) and the final (8th) time points separately for each layer revealed larger changes in the first two layers and substantially smaller changes in layers 3–7 (Fig. 4 B, C ). Similar to the AlexNet, CCI values were significantly higher than chance in all layers (all p = 0.007, Bonferroni corrected for 7 layers), and we observed a fourfold increase of CCI values from the first (CCI = 0.07) to the last layer (CCI = 0.40) of the network (Fig. 4D ). Contrary to the AlexNet, the increases in CCI in the BL-NET network were only due to a decrease in between-category correlations (average slope of linear fit across layers = −0.06; p = 0; Supplementary Fig 5 , middle right), while the within-category correlations did not change across layers (average slope of linear fit across layers = −0.00062; p = 0.72; Supplementary Fig 5 , middle left). Furthermore, BL-NET between-category correlations decreased significantly more across layers than AlexNet between-category correlations (Alexnet vs. BL-NET slope difference = 0.1061; p = 0).
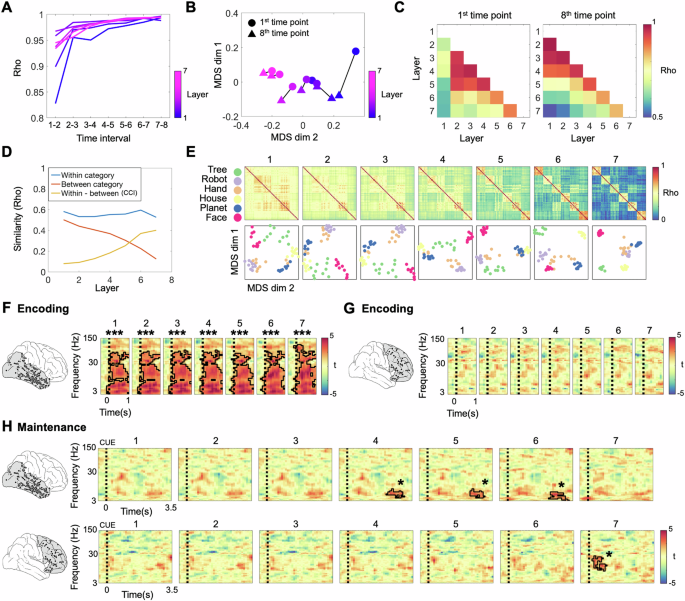
A Representational consistency at each time interval of the BL-NET network was computed by correlating representations formed at successive time points. Each curve represents one layer of the network, color-coded from early (blue) to deep layers (pink). B Two-dimensional projections of the first and last time point of each layer in the BL-NET network showing greater representational distances in the first layer than in all other layers. C Pairwise correlations of RSMs corresponding to the first (left) and last (right) time points in each layer of BL-NET. D Within-category, between-category and within-category vs. between-category correlations (i.e., Category Cluster Index, CCI) for each layer of BL-NET (last time point). E RSMs and corresponding MDS projections for the last time point of all BL-NET layers. In the MDS plots, items are color-coded according to category. F Correlations between BL-NET RSMs (last time point in each layer) and neural RSMs during encoding in VVS. Outlined clusters indicate time-frequency periods where correlation values are significantly higher than zero at the group level (two-sided t -tests, Bonferroni corrected for 7 layers). G Same analysis as in F for the PFC. H Same analysis as in F for the maintenance period in the VVS (top) and PFC (bottom). In the VVS, BL-NET representations in layers 4, 5, and 6 matched representations in the theta/alpha frequency range (3-14 Hz) prior to the probe. In the PFC, BL-NET representations in the last layer matched representations in the beta band (16–29 Hz) following presentation of the retro-cue. Color scale of all t-maps in F , G and H is indicated at the right of each panel. Source data are provided as a Source Data file. *** p < 0.001; * p < 0.05.
Together, these results show that the BL-NET network represents low-level features more dynamically than high-level visual features and that it clusters categorical information more strongly in deep than superficial layers. Contrary to the AlexNet network, this clustering is exclusively due to a reduction of between-category correlations rather than an increase in within-category correlations across network layers.
We next compared neural and BL-NET representations, focusing on the RSMs at the last time-point of each layer (Fig. 4E ). During encoding, results were similar to those in the AlexNet analysis: Network representations of all layers matched VVS representations for a wide range of frequencies between 3 and 75 Hz ( p corr = 0.007; Fig. 4F ), and these extended into the high-gamma range (i.e., until 110 Hz) in layer 7. No significant correlations were observed in the PFC (all p corr > 0.263; Fig. 4G ). During maintenance, no significant fits were observed in the VVS following the retro-cue, again consistent with the AlexNet analysis. Interestingly, however, we observed a significant matching of VVS representations in the theta/alpha frequency range (3–14 Hz) with BL-NET representations in layers 4 ( p corr = 0.035), 5 ( p corr = 0.035) and 6 ( p corr = 0.014). These effects occurred in a late maintenance time period from 2.1 s to 3.2 s, close to the presentation of the probe (Fig. 4H , top row). Critically, in the PFC, we observed a significant fit between neural and network RSMs following presentation of the retro-cue, i.e. time-locked to the prioritization process. This effect started 200 ms after the onset of the retro-cue and lasted for 800 ms; It was specifically observed for the last layer of the BL-NET (final layer: p corr = 0.021; all other layers: p corr > 0.43), and related to neural representations in the beta frequency range (15–29 Hz; Fig. 4H , bottom row).
The specific alignment of the representational geometry of PFC activity with the last layer of BL-NET during the prioritization period suggests that the format of representations has been transformed in this region—from a purely categorical format during encoding into a format that incorporates distinctions among stimuli between categories during maintenance. To corroborate this transformation and characterize the representational formats observed in the PFC more comprehensively, we performed several additional analyses. First, we tested whether the average pairwise neural correlations differed between encoding and maintenance. Higher correlations of items during maintenance may point towards clustering of representations, while lower correlations would reflect the opposite, i.e. representations in a more widely spread representational space. Second, we analyzed the variance of correlations during encoding and prioritization. Higher variances would reflect less uniform (i.e., more distinctly organized and thus lower dimensional) distributions of items, while lower variances would correspond to an opposite pattern. We performed both analyses separately for items of the same category (within-category correlations) and items of different categories (between-category correlations). We found a trend for higher average between-category correlations during maintenance as compared to encoding (t(14) = −2.12, p = 0.053), and no significant differences in the average same-category correlations (t(14) = −0.185, p = 0.856). Moreover, the variance of between-item correlations decreased significantly from encoding to maintenance, both for items from different categories (t(14) = 5.87, p = 4.05e−05) and from the same category (t(14) = 5.37, p = 9.89e−05). We next compared the dimensionality of RSMs during encoding and maintenance. We projected the data in various dimensions using Multidimensional Scaling (MDS), and computed the stress of the MDS projections. Stress indicates the goodness of fit of a particular projection, and thus lower stress values during encoding or maintenance would indicate lower-dimensional representations during that time period. We observed that stress values were systematically lower during encoding as compared to prioritization in a cluster of significant dimensions (from 4 to 33 dimensions; p = 0.0148; Supplementary Fig 1A ). Taken together, these results indicate a change from a purely categorical representation during encoding to a representation that matches the fine-grained architecture of the BL-NET during prioritization: PFC representations occur in a smaller representational space, occupy less clustered regions in this space, and rely on a higher-dimensional neural code. Thus, our results point to a transformation of the representational format of PFC activity from encoding to maintenance.
We next investigated the fit of the BL-NET and the AlexNet networks during the prioritization period separately for within-category and between-category correlations. We observed a significant fit of the between-category correlations of RSMs from the BL-NET and neural data in the PFC (t(14) = 3.69, p = 6.76e−05; Supplementary Fig 1B ), while this was not true for the AlexNet (t(14) = 1.61, p = 0.13). None of our models could explain the structure of within-category correlations (BL-NET: t(14) = 0.42, p = 0.67; AlexNet: t(14) = −0.18, p = 0.86; Supplementary Fig 1B ). The results of the same analysis performed during encoding confirmed that neither BL-NET nor AlexNet are good models of activity in the PFC during this time period (BL-NET within-category correlations: t(14) = −0.37, p = 0.71; BL-NET between-category correlations: t(14) = −1.84, p = 0.086; AlexNet within-category correlations: t(14) = 0.17, p = 0.86; AlexNet between-category correlations: t(14) = −1.31, p = 0.21). These results demonstrate that the fine-grained structure in PFC that is captured by the BL-NET model is due to the geometry of between-category correlations—i.e., that the BL-NET corresponds to the relative representational distances of individual exemplars to exemplars of other categories.
In additional control analyses, we investigated the functional relevance of representations in VVS and PFC during the maintenance period (Supplementary Fig 6 ), compared the fits of the BL-NET, AlexNet and the category model in VVS and PFC (Supplementary Note 4 ; Supplementary Fig 7 and Supplementary Fig 8 ), dissociated the representational formats of the category model and the BL-NET through simulations and analyses conducted in individual participants (Supplementary Note 5 ; Supplementary Fig 8 and Supplementary Fig 9 ) and evaluated the fits of the BL-NET in the PFC with a variant of this network trained with a recently released dataset of images (Ecoset 76 ; Supplementary Fig 10 ).
In our final analysis, we employed the corNET-RT model to account for VVS and PFC representations. Consistent with the BL-NET analyses, we first evaluated the representational consistency across successive time points in each layer of the network. The final layer (IT) showed the lowest correlation across consecutive time points compared to all other recurrent passes in the network (Rho = 0.78; note the first recurrent pass in IT is the fourth overall pass in the network, Methods). This demonstrates that contrary to the BL-NET, corNET-RT represents stimuli more dynamically in its deepest layer. In addition, we observed that representations in layers V2 and V4 clustered together in representational space, while representations in V1 and IT were segregated (Fig. 5 B, C ). Categorical clustering of representations was found in all layers, as evidenced by significant CCI scores in each layer and at each time point (all p corr > 0.004; Fig. 5D ). Similar to BL-NET and contrary to AlexNet, we observed a prominent increase in CCI scores across layers, which was mostly due to a decrease in between-category correlations (average slope across layers = −0.14; p = 0; Fig. 5D and Supplementary Fig 5 ). However, within-category correlations were also reduced across network layers (average slope across layers = −0.02; p = 1.63e−11; Fig. 5D and Supplementary Fig 5 ).
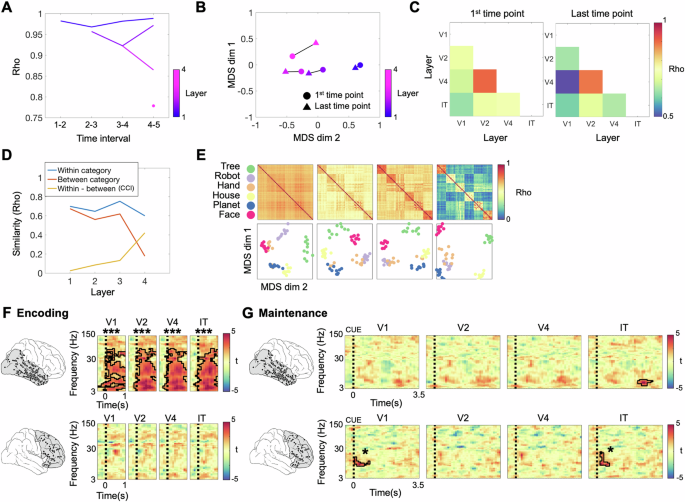
A Representational consistency at each time interval of the corNET-RT network. Note that in this architecture, different layers have different numbers of recurrent passes, and deep layers do not receive input until activity has propagated from early layers. Each curve represents one layer, color-coded from early (blue) to deep (pink). B MDS projections of first (circle) and last (triangle) time point in each layer show relatively higher temporal dynamics in layer IT (output) compared to the other layers. MDS results have been scaled for visualization. C Pairwise correlations of RSMs corresponding to the first (left) and last (right) time points in each layer of corNET-RT. D Within-category, between-category and within-category vs. between-category correlations (i.e., Category Cluster Index, CCI) at each layer of the network (final time point). E RSMs (top) and corresponding MDS projections (bottom) for each layer of the corNET-RT network (final time-point). Items are color-coded by category. F Correlations between corNET-RT RSMs (last time point in each layer) and neural RSMs during encoding in VVS (top) in the 3–150 Hz frequency range. Colors indicate resulting t-maps in the comparison of group-level correlation values against zero (two-sided t -tests). Significant regions after multiple comparisons correction are outlined in black (Bonferroni corrected for 4 layers). G Same analysis as in F for the maintenance period. Top: A match between network and neural representations was observed in the VVS in a late period, close to the presentation of the probe, in the IT layer. Bottom: In the PFC, correlations were significant with representations in the beta frequency range (15–29 Hz) following the onset of the retro-cue with both V1 and IT layer. Color scale of all t-maps in F and G is indicated at the right of each panel. Source data are provided as a Source Data file. *** p < 0.001; * p < 0.05.
We compared corNET-RT RSMs to neural representations, focusing on the last time point in each layer, again consistent with the BL-NET analysis (Fig. 5E ). During encoding, we found a significant match of VVS representations across a wide range of frequencies with corNET-RT representations in all layers (3–105 Hz; all p corr < 0.004; Fig. 5F , top row). No significant correlations were found in the PFC (all p corr > 0.053, Fig. 5F , bottom row). During the maintenance phase, corNET-RT representations in IT matched those in the VVS towards the end of the maintenance period, specifically in the theta-alpha frequency range, consistent with the results observed in the BL-NET analysis (6–11 Hz; p corr = 0.044; Fig. 5G , top row). Critically, we again observed a significant match of corNET-RT representations in IT with PFC representations time-locked to the presentation of the retro-cue and in the beta band (15–29 Hz; p corr = 0.016; Fig. 5G , bottom row). This effect lasted for 500 ms, similar to the results observed in the BL-NET analysis. In addition, we observed a significant correlation with representations in V1 ( p corr = 0.036).
We performed control analyses using parameter-matched versions of our recurrent architectures to evaluate the effect of recurrency, while isolating other possible confounding variables (Supplementary Note 6 ). Results suggested that recurrent computations are indeed crucial for tracking cognitive representations in PFC, because the fit observed with the recurrent networks could not be found in any of the feedforward models we tested. They also show that recurrency may play a relatively less prominent role in the VVS (Supplementary Note 6 ).
Taken together, these results show that PFC representations following the retro-cue matched those in two recurrent neural network architectures (the BL-NET and the corNET-RT) but not those of a purely feedforward network (the Alexnet), and that these effects were specific to the beta-frequency range and most prominent for late layers of the networks. VVS representations did not show correspondence with representations in recurrent networks following the retro-cue, but prior to the probe.
Our study aimed to unravel representational formats and neural coding schemes in sensory and executive control regions during WM prioritization. Specifically, we analyzed the impact of WM prioritization on stimulus-specific activity patterns in VVS and PFC and assessed their representational formats using feedforward and recurrent DNN models of natural image processing. The VVS exhibited pronounced category-specific representations during encoding which were reinstated during the maintenance period, reflecting a shared (or ‘mnemonic’) coding scheme across both experimental phases. The PFC exhibited robust category-specific representations during WM encoding as well, but did not show reinstatement of encoding patterns during the maintenance period. Subsequent in-depth analyses showed that this lack of reinstatement in PFC was not due to memory decay or reduced signal to noise ratio, but due to a transformation of representations between different task-dependent formats, in line with a dynamic ‘prioritization’ coding scheme: Representations in PFC corresponded to a simple categorical model during encoding, but matched only the deepest layer of a recurrent DNN following retro-cue, suggesting a prioritized format in which high-level visual features of images are preponderant. This shift was also reflected at the level of the neurophysiological substrates of WM representations, since PFC representations during encoding were observed in theta, beta and gamma frequency bands but exclusively in beta frequency oscillations during the retro-cue. Taken together, these results demonstrate that WM prioritization relies on a distinct recruitment of specific task-depend representational formats in the PFC.
Recent investigations showed a transformation of visual representations from perceptual to abstract formats during VWM encoding 57 , 58 . While representations in these studies were based on patterns across the entire brain, we here focused on representations in two brain regions that are critical for VWM storage and control, respectively: VVS and PFC. We note that our initial RSA analysis of category representations (Fig. 2B ) could not explain representations during the maintenance period in either of these regions. The EMS analysis (Fig. 2D ), however, revealed a distinct set of results in VVS and PFC: While encoding activity patterns reoccurred during the maintenance period in the VVS, this was not the case in the PFC. In the VVS, representations towards the end of the maintenance period matched representations in intermediate and deep layers of two recurrent DNN architectures (BL-NET and corNET-RT), suggesting a transformation during encoding from a purely ‘categorical’ format into a format that incorporates high-level visual and semantic relationships among stimuli. Thus, despite a relative stability of neural activity patterns (as revealed by the EMS analysis), their representational geometry changes and eventually results in less categorical representations during the maintenance period.
By contrast, in the PFC, encoding activity patterns did not reoccur during the maintenance period, suggesting a more pronounced transformation in this region; however, neural representations following the retro-cue matched representations in deep layers of two recurrent DNN architectures. Notably, in the VVS, all representational signatures that were observed during the second maintenance period (corresponding to the deeper layers of the two recurrent networks) were already apparent during encoding, and this likely explains the significant encoding-maintenance similarity (EM 2 S) in this region. Thus, maintenance in VVS corresponds to a partial and selective re-appearance of encoding formats, corresponding to a ‘mnemonic’ coding scheme. By contrast, in the PFC, the representational signatures that were observed during maintenance did not already occur during encoding, and thus the PFC does not show such a mnemonic coding scheme but exhibits a more profound transformation. We refer to the format of PFC representations after the retro-cue as ‘prioritized’.
Our results provide a comprehensive description of the representational transformation observed in the PFC during the prioritization period, from a purely categorical to a less categorical and higher-dimensional format that specifically maps with the BL-NET and corNET-RT but not with other DNN models. In detailed analyses of the geometry of PFC representations during encoding and maintenance, we found that PFC representations occur in a smaller representational space during the prioritization period, occupy less clustered regions, and rely on a higher-dimensional neural code. Notably, these differences were mostly observed for the between-category correlations (Supplementary Fig 1 ), whose structure cannot be explained by the category model. Considered together with the lack of EM 2 S in PFC, these results point to a transformation of the representational format of PFC activity from encoding to maintenance, which is particularly due to a transformation of the geometry of the between-category correlations.
An important difference between VVS and PFC relates to the time period at which representations matched those from a recurrent DNN: directly following the retro-cue in PFC, but prior to the probe in the VVS and thus in preparation for the response. This suggests that maintenance in the two different regions likely serves different functional roles.
What could be the functional role of the transformation of category-specific representations in the PFC? Our data is consistent with a capacity-limited view of WM which would benefit from compressed stimulus representations in this region, while still maintaining high-level visual properties of images. This notion has been recently supported by behavioral 61 and neuroimaging 53 studies. In particular, ref. 61 . demonstrated that semantic aspects of images are selectively prioritized during WM maintenance in a multi-item WM task, while no selective storage of abstract features of images is present in single-item tests. This fits to our findings in the VVS that contained both perceptually detailed and abstract representations during maintenance of single items, while we additionally found high-level visual representations in the PFC. In the fMRI study of ref. 53 , representational abstraction was observed in parietal and visual cortices, but not in prefrontal regions. The differences between our results and those of ref. 53 . might relate to the particular stimuli employed (natural images with semantic content versus low-level visual features), and to our use of a paradigm involving prioritization, which preferentially engages the PFC 1 , 23 .
Prioritized information in PFC was specifically detected in the 15–29 Hz frequency range, i.e., within the beta band (13–29 Hz). Previous studies showed a prominent role of prefrontal beta oscillations for top-down control of information in WM 4 , 35 , and oscillatory activity in the beta range has also been associated with transient task-dependent activation of stimulus-specific information during WM maintenance 46 . Our results in PFC are well consistent with these interpretations: The representations we observed are content-specific and locked to the presentation of the retro-cue, which is when the prioritization process takes place. This aligns with previous studies that have reported stimulus-specific activity in the beta range during WM maintenance (e.g., ref. 45 ; see ref. 46 . for review). In contrast to standard delay tasks where beta modulations occur late in the WM delay period 43 , 45 , our study demonstrates brief and cue-locked effects, consistent with previous retro-cue paradigms 77 . Our findings are also consistent with the widely accepted role of the PFC in the top-down control of information stored in other brain regions, in line with previous studies on both episodic and working memory 78 . Indeed, activity in the PFC has been linked to task-dependent executive control over specific contents in several studies (for a review, see ref. 79 ). This could be achieved by modulating the activation state of distributed perceptual and mnemonic representations 78 , for instance through PFC connectivity with the VVS 80 . The transient beta-frequency reactivation we observed in the PFC is suggestive of a top-down signal prompted by presentation of the cue that might affect information processing in downstream regions 81 . Further studies are required to investigate this possibility. Nevertheless, our results confirm previously untested views of PFC functioning by demonstrating its engagement in the transformation of VWM representations during VWM prioritization.
DNNs are increasingly used in cognitive neuroscience to characterize the representational formats and temporal dynamics of perceptual and mnemonic representations in the brain. While different feedforward and recurrent architectures have been applied in the domain of vision, resulting in a wide variety of models employed to fit neural data (e.g., refs. 32 , 33 , 59 , 65 , 67 , 82 ), this approach has only started to be employed in memory research. Pioneering investigations have applied the feedforward neural network AlexNet to study representational formats during visual working memory in humans 57 , 58 . Notably, these studies did not investigate the representational formats during WM maintenance but focused solely on the encoding period. While theoretical and experimental considerations have strongly argued for the use of recurrent architectures in the domain of visual perception 33 , 60 , 83 , they have so far not been applied to memory research. The use of recurrent architectures in the context of working memory is particularly important given the relevance of recurrent computations for PFC processing 26 , 84 and WM functions in general 27 , 28 , 30 , 85 . In our study, we tested a feedforward and two recurrent models in their ability to predict representational distances in human iEEG data. During encoding, both types of models captured the representational geometry of stimuli across all layers and a wide range of frequencies in the VVS, while no fits were observed in the PFC (Figs. 3 , 4 and 5 ). During maintenance, however, the two architectural families strongly differed in their fit to the neural data: the AlexNet was unable to capture representations in either region, while BL-NET and corNET-RT matched representations in both VVS and PFC (Figs. 4 H and 5G ). Control analyses using parameter-matched versions of the BL-NET without recurrency indicated that recurrent computations are indeed crucial for tracking cognitive representations in PFC, while they appear to play a relatively less critical role in the VVS (Supplementary Note 6 ). Together, these results demonstrate that only recurrent architectures can explain the representational geometry of stimuli during VWM prioritization in PFC, while a feedforward architecture and a simple model of category information do not provide good fits.
What are the differences in the representational geometries of AlexNet, BL-NET and corNET-RT that can explain the different fits to the neural data we observed? We thoroughly characterized within-category, between-category and within- vs. between-category correlations (i.e., CCI) in all three architectures to investigate their differences in stimuli representation. We found that all networks represented increasingly category-specific information across layers, as assessed by prominent increases in CCI, yet this was achieved through different representational changes. While the AlexNet showed both an increase in within-category correlations and a decrease in between-category correlations, the recurrent models only showed decreases in between-category correlations (Supplementary Fig 5 ), suggesting that recurrence particularly supports distinct representations of different categories. Again, further studies are needed to unravel the possible neurophysiological basis and cognitive function of these representational transformations.
Computational models of WM have proposed that prioritization requires a transformation of the neural space of activity in which the items are represented, involving, for example, a rotation or “flip” of the format of prioritized content in neural activity space 29 , 30 . These models have recently received empirical support from studies in monkeys (e.g., ref. 86 ), suggesting an efficient neural code that organizes and structures neural representations during the prioritization process. Consistently, a recent iEEG study in humans demonstrated a role of PFC in resolving cognitive interference between competing sensory features by transforming their representational population geometry into distinct neural subspaces to accommodate flexible task-switching 87 . Our work contributes to this literature by establishing that the PFC not only supports a transformation of the representational geometry of stimuli but also a differential representation of particular visual formats in the context of VWM prioritization. In particular, we argue that the degree of matching to RSMs derived from DNNs is of heuristic value because these models have previously been shown to match representations during sensory processing and have been widely applied to analyze representational transformations during various cognitive tasks (see ref. 83 ).
It has been recently proposed that the selection of network training sets critically influence the matching of DNN and neural representations, and that this influence may be more important than specific architectural constraints 88 . For this reason, in our study we employed two different datasets of images (ImageNet and Ecoset), which provided consistent results (Supplementary Fig 10 ). Other limitations remain, however: First, the BL-NET and corNET-RT networks were not trained to memorize stimuli but to solve the task of image classification, which may be argued to limit their value as models of WM representation. However, we note that the use of networks trained in a lower dimensional task objective, i.e., image classification, to model cognitive representations embedded in a higher-level cognitive process, i.e., VWM prioritization, has received some theoretical support. Indeed, representational accounts of memory have argued that it is not the cognitive process (e.g., memory versus perception) that defines representations, but rather the content that any given cognitive process requires. Indeed, regions representing particular content in the brain (e.g., low-level visual features in early visual regions) are involved in the representation of these features irrespective of the cognitive process in which they are engaged 89 , 90 , 91 . Since the VVS plays a role both during object recognition and VWM for these objects, it is relevant to investigate the representational format of items during both processes, and DNNs are arguably strong tools to capture these formats 58 , 92 . Beyond these theoretical considerations, we underscore the widespread practice in our field of using networks pretrained in particular tasks to characterize representations formed in different tasks. Previous studies have employed the AlexNet network to investigate the representational formats of representations during both VWM and long-term memory 57 , 58 , 92 . A similar trend is observed in natural language processing, where language models trained in the task of next word prediction have been applied to model language-related brain responses more broadly 93 , 94 , 95 , 96 , 97 .
A second limitation of the models we employed relates to their architecture: BL-NET and corNET-RT do not include top-down connections but only lateral connectivity, and thus cannot account for PFC-VVS interactions. In future work, novel architectures should be employed that mimic brain connectivity more accurately at least at a high-level of description (i.e., containing top-down as well as within-layer connections). Finally, while we decided to focus on the prioritization process and the single-item trials in this study, we aim to further investigate the representation of multiple items in the future. A promising avenue for this purpose is the use of sequential recurrent convolutional networks that receive multiple consecutive images as input and can be employed to track multi-item representations (e.g., ref. 98 ).
We note that the different models we employed (e.g., BL-NET, AlexNet, category model) do not only represent different hypotheses about how the brain represents visual information, but they also differ in the aspects of the representational geometry they can model. For instance, the category model only codes binary information about category membership, while the DNNs’ deep layers in addition reflect more subtle differences among stimuli which encode high-level visual properties of images. The category model is by definition agnostic to any structure in the within-category and between-category correlations (which are all modelled identically, with ones and zeros), while the DNN models propose a very specific geometry for these two types of relationships. Thus, fitting the two models to neural data provides complementary information regarding the geometry of representations. Notably, while the category model and BL-NET are not mutually exclusive (orthogonal), we have shown a dissociation in their levels of fit during encoding and prioritization: The category model explains well representations during encoding but not maintenance, while the reverse is true for the recurrent DNNs.
Many important previous studies on representational transformations during VWM prioritization have been conducted with non-human primates (e.g., refs. 6 , 86 ). Our study is the first report on prioritized representations using human intracranial EEG, which provides a level of analysis ideally suited to bridge network level (EEG/MEG) studies on VWM 99 to invasive recordings in monkey studies. In addition, while previous studies have employed analyses on representational subspaces based on single unit data (e.g., ref. 86 ) or computer simulations 29 , 30 , we employ DNNs and RSA. While both methods have their complementary value and importance, a critical difference is the mapping of DNN onto different processing stages during perception, which adds heuristic value to our findings 83 .
In summary, we present evidence of successive representational transformations during VWM encoding and after item prioritization in the VVS and the PFC that critically depend on recurrent computations and abstract representational formats. This result shows that percepts originally formed during encoding are differentially abstracted and reshaped in VVS and PFC to enable flexible task-dependent manipulations during working memory prioritization.
Participants
Thirty-two patients (17 females, 30 ± 10.04 years) with medically intractable epilepsy participated in the study. Data were collected at the Freiburg Epilepsy Center, Freiburg, Germany; the Epilepsy center, Second Affiliated Hospital, School of Medicine, Zhejiang University, Hangzhou, China; and the Center of Epileptology, Xuanwu Hospital, Capital Medical University, Beijing, China. The study was conducted according to the latest version of the Declaration of Helsinki and approved by the ethical committee at the Albert-Ludwigs-Universität Freiburg. All patients provided written informed consent. The number of patients included in the study was determined based on previous literature and is substantially higher than previous iEEG studies on VWM.
Experimental design
Participants performed a multi-item working memory paradigm involving a retro-cue. They encoded a sequence of 3 images of natural objects from different categories and were asked to remember this content during a subsequent maintenance period. This period consisted of two phases that were separated by a retro-cue. The retro-cue prompted participants to selectively maintain items from particular encoding positions (single-item trials, 50%), or to maintain all items in their order of presentation (multi-item trials, 50%) for a subsequent memory test. Note that with the exception of the behavioral data, we only focused on the single-item trials in this study. In the test, six items were presented, which included all 3 presented items from encoding, and three new exemplars. Of these three new exemplars, one was always from a different category. The other two were either both from categories presented during encoding (50% of trials), or only one of them (50% of the trials, Fig. 1A ). In the single-item trials, one of the lure items in the test was from the same category as the cued item in 40% of the trials. This was done to disable inferences from the probe items to the categories of the presented items. Objects pertained to six categories (trees, robots, hands, houses, planets and faces) with ten exemplars each (60 images in total). In order to perform the task correctly, participants needed to remember not only categorical information about the items but also the specific perceptual information identifying each individual exemplar.
Performance in the task was quantified separately for each encoding position. We calculated the proportion of correct responses for positions 1, 2 and 3 independently and averaged these values to obtain an overall metric of performance in the single and multi-item trials (Fig. 1C ). The task was divided into blocks and sessions. Each block consisted of 60 trials. Each session consisted of at least one block, but most participants performed between 1 and 3 blocks in each session (2.59 ± 1.04) and between 1 and 2 sessions (1.19 ± 0.39) in total. The order and frequency of image presentations was pseudorandomized to balance repetitions of images across blocks and sessions. The experiment was programmed in Presentation (Neurobehavioral systems, California, USA), and was deployed on Samsung 12” tablet computers running Microsoft Windows. Patients performed the experiment while sitting in their hospital beds and responded to the memory test utilizing the touch-screen of the tablet.
Two versions of the experiment were implemented for the different patient populations in Germany and China. The two versions had identical stimuli in all categories except for the category “Faces”. The German version of the experiment included faces of former German chancellor Angela Merkel and the Chinese version included faces of the actor Jackie Chan. This was made to ensure that the face represented was equally well known to the different patient populations.
Intracranial EEG recordings
IEEG data were recorded using amplifiers from Compumedics (Compumedics, Abbotsford, Victoria, Australia), and Brain Products GmbH with sampling rates of 2000 Hz and 2500 Hz, respectively. Patients were surgically implanted with intracranial depth electrodes for seizure monitoring and potential subsequent surgical resection. The exact electrode numbers and implantation locations varied across patients and were determined by clinical needs. Online recording data was referenced to a common scalp reference contact which was simultaneously recorded with the depth electrodes. Data was downsampled to 1000 Hz and bipolarized by subtracting the activity of one contact point with that from the nearest contact of the same electrode, resulting in a total of N-1 virtual channels for an electrode with N channels after bipolarization.
Channel localization
Electrodes employed were standard depth electrodes (Ad-Tech Medical Instrument Corporation, Winsconsin, USA). Electrodes contained variable number of contacts and inter-contact distances. In the data collected at Zhejiang University, Hangzhou, and Medical University, Beijing, each depth electrode was 0.8 mm in diameter and had either 8, 12 or 16 contacts (channels) that were 1.5 cm apart, with a contact length of 2 mm. Channel locations were identified by coregistering the post-implantation computed tomography (CT) images to the pre-implantation Magnetic Resonance Images (MRIs) acquired for each patient, which were afterwards normalized to Montreal Neurological Institute (MNI) space using Statistical Parametric Mapping (SPM; https://www.fil.ion.ucl.ac.uk/spm/ ). We then determined the location of all electrode channels in MNI space using PyLocator ( http://pylocator.thorstenkranz.de/ ), 3DSlicer ( https://www.slicer.org ) and FreeSurfer ( http://surfer.nmr.mgh.harvard.edu ). In a group of patients (data collected in Beijing), we determined MNI coordinates using the pipeline described in ref. 100 , and identified the closest cortical or subcortical label for each channel in each patient. In all patients, we removed channels located in white matter, resulting in 588 clean channels across all patients (18.4 ± 11.9 channels per patient).
ROI selection
We selected two main regions of interest given their well-known involvement in VWM: the ventral visual stream (VVS) and the prefrontal cortex (PFC). The VVS has been widely studied in the context of object recognition during visual perception 101 . Previous work employing iEEG and Deep Neural Networks often applied RSA metrics to activity from distributed electrodes across the whole brain (e.g. 57 ,), and we specifically aimed to extend these studies by investigating region-specific representations in the context of VWM (see also ref. 80 ).
The role of the PFC in working memory has been linked to executive control processes that enable the task-dependent manipulation and transformation of information 1 , 4 , 102 . However, relatively little is known about the representational formats of VWM representations in this region during prioritization. Moreover, no previous study investigated region-specific representational similarity during VWM.
Electrodes located at the following freesurfer locations were labeled as VVS electrodes: ‘inferior temporal’, ‘middle temporal’, ‘superior temporal’, ‘bankssts’, ‘fusiform’, ‘cuneus’, ‘entorhinal’. Electrodes with the following labels were categorized as PFC electrodes: ‘medial orbitofrontal’, ‘pars triangularis’, ‘superior frontal’, ‘lateral orbitofrontal’, ‘pars opercularis’, ‘rostral anterior cingulate’, ‘rostral middle frontal’, ‘superior frontal’. Electrodes from both left and right hemispheres were included in our ROIs. This resulted in a total number of 147 electrodes (16 subjects) in PFC and 441 in VVS (28 Subjects). The different number of subjects and channels in our two ROIs implies different levels of statistical power. Since an important objective of our study was to characterize how representations in PFC and VVS differ during VWM maintenance and prioritization, we confirmed that our main findings replicate when matching statistical power in VVS and PFC through several control analyses (Supplementary Fig 11 and Supplementary Note 7 ).
In additional analyses, we specifically analyzed activity in the lateral prefrontal cortex (LPFC), a brain region that has been associated with attentional prioritization 6 , the representation of rules 103 and categories 104 in non-human primates. We excluded all PFC electrodes with MNI x-coordinates smaller than −35 or larger than +35 and z-coordinate < −15. The new selection resulted in a group of 9 subjects with a total number of 38 electrodes in the LPFC, which were located in the following Freesurfer regions: ‘rostral middlefrontal’, ‘pars triangularis’, ‘caudal middlefrontal’, ‘pars orbitalis’ and ‘pars opercularis’ (Supplementary Fig 12 ).
Preprocessing
We visually inspected raw traces from all channels in each subject independently and removed noisy segments without any knowledge about the experimental events/conditions. All channels located within the epileptic seizure onset zone or severely contaminated by epileptiform activity were removed from further analyses. We divided the data into 9-second epochs (from −2 to 7 s) around the presentation of each stimulus at encoding or the onset of the retro-cue during the maintenance period. After epoching the data, we completely removed epochs containing artifacts that were identified and marked in the non-epoched (continuous) data. We visually plotted spectrograms to verify the presence of artifacts in the frequency domain in the resulting epochs. The number of epochs corresponding to item or cue presentation that were removed varied depending on the quality of the signal in each subject (15.10 ± 13.14 in each session, corresponding to around 6.9% of all epochs in each session).
Preprocessing was performed on the entire raw data using EEGLAB 105 , and included high-pass filtering at a frequency of 0.1 Hz and low-pass filtering at a frequency of 200 Hz. We also applied a band-stop (notch) filter with frequencies of 49–51 Hz, 99–101 Hz, and 149–151 Hz.
Time-frequency analysis
Using the FieldTrip toolbox 106 , we decomposed the signal using complex Morlet wavelets with a variable number of cycles, i.e., linearly increasing in 29 steps between 3 cycles (at 3 Hz) and 6 cycles (at 29 Hz) for the low-frequency range, and in 25 steps from 6 cycles (at 30 Hz) to 12 cycles (at 150 Hz) for the high-frequency range. These time-frequency decomposition parameters were taken following previous research that used iEEG oscillatory power as features for RSA 49 , 57 , 107 . The resulting time-series of frequency-specific power values were then z-scored by taking as a reference the mean activity across all trials within an individual session 108 . This type of normalization was applied to remove any common feature of the signal unrelated to the encoding of stimulus-specific information. We z-scored across trials in individual sessions in our final analyses, but similar results were obtained when we z-scored the data by considering the activity of all trials irrespective of the session. We employed the resulting time-frequency data to build representational feature vectors in our pattern similarity analyses (see below).
Pattern similarity analysis: representational patterns
We employed different representational features in our analyses involving model RSMs (i.e., the category model RSA analysis and the DNN-based RSA analyses), and our analyses involving particular contrasts (i.e., encoding-encoding similarity analysis [EES] and the encoding-maintenance similarity analysis [EMS]; see below). In both types of analyses, representational feature vectors were defined by specifying a 500 ms time window in which we included the time courses of frequency-specific power values in time-steps of 100 ms (5 time points) across all contacts in the respective ROI (VVS or PFC). In the RSM based analyses, we performed this analysis separately for each individual frequency in the 3–150 Hz range, while in the EES and EMS analyses, we analyzed activity patterns across individual frequencies within five different bands (theta, 3–8 Hz; alpha, 9–12 Hz; beta, 13–29 Hz; low-gamma, 30–75 Hz, high-gamma, 75–150 Hz). In the RSM-based analyses, a frequency-specific representational pattern was thus composed of activity of N electrodes x 5 time-points in each 500 ms window. In the EES and EMS analyses, this representational feature vector consisted of N electrodes x M frequencies x 5 time-points. Note that the number of channels included in the representational feature vectors varied depending on the number of electrodes available for a particular subject/ROI, and the number of frequencies included in each band in the EES and EMS analyses varied as well (theta: 6 frequencies; alpha: 4 frequencies; beta: 17 frequencies; low-gamma: 9 frequencies, high-gamma: 16 frequencies; see section Time-frequency decomposition above). These two- or three-dimensional arrays were concatenated into 1D vectors for similarity comparisons. Only subjects with at least 2 electrodes in a particular ROI were included in all RSA analyses, leading to 15 subjects in the PFC and 26 subjects in the VVS.
Model-based RSA
We employed temporally resolved Representational Similarity Analysis (RSA) to evaluate the dynamics of categorical information in our data following previous work 33 , 101 . A main assumption of this research is that stimuli from the same categories will have greater neural similarity than stimuli from different categories. To evaluate this hypothesis, we constructed a representational similarity matrix (RSM) in which a value of 1 was assigned to pairs of items of the same category and a value of zero to items of different categories (‘category model’, Fig. 2A ). We also built an ‘item model’ to track the presence of item-specific information, in which correlations of items of the same category were coded with a 1 and correlations of different items were coded with a zero (Supplementary Fig 3A ). Finally, we used RSMs extracted from layers of DNNs as models of representation (see section Stimulus representations in DNNs below).
The different model RSM were correlated with time series of neural RSMs in each of our ROIs. Pairwise correlations among stimuli were computed in windows of 500 ms, overlapping by 400 ms, using the representational patterns described in the section above, resulting in an RSA time-frequency map in each of our ROIs. In order to obtain a robust estimate of the multivariate patterns representing individual items in the category model analysis, we averaged the time-frequency activity across repetitions of items throughout the experiment in each channel independently before building the neural RSMs (note that this was not done in the item model analysis where repeated presentations of exemplars were required). RSM time-series were vectorized by removing the diagonal values and taking only half of the matrix given its symmetry at each time-frequency point. We correlated vectorized model RSMs and neural RSMs at each time-frequency point using Spearman’s rho, and evaluated whether the resulting Fisher z-transformed rho-values were different from zero at the group level to determine statistical significance (two-sided tests). Multiple comparisons correction was performed using cluster-based permutation statistics (see below), and—in the DNN analyses—, we Bonferroni corrected the final results to account for the number of layers tested in each network.
Contrast-based RSA
In order to test the reoccurrence and stability of activity patterns in our two regions of interest during encoding and between encoding and maintenance, we performed two contrast-based pattern similarity analyses, as a complementary analysis to the model-based RSA approach (Fig. 2 ). In particular, we investigated the presence of category-specific information in our data by contrasting correlations between different items of the same category with correlations between different items from different categories. This was done separately for items presented in different trials during encoding (encoding-encoding similarity, EES) and between encoding and maintenance (encoding-maintenance similarity, EMS). Only items belonging to different trials were included in this analysis to avoid any spurious correlations driven by the autocorrelation of the signal. Similar to the model-based RSA approach, we averaged across item repetitions before conducting the similarity comparisons.
We computed similarities for same-category and different-category item pairs and averaged across all combinations of items in the same condition in each subject independently (rho values were Fisher z-transformed before averaging). The same-category and different-category correlations were then statistically compared at the group level using t -tests. In the different-category condition, we excluded item pairs containing stimuli presented in overlapping trials after averaging, again to avoid any possible bias related to the autocorrelation of the signal. As an example, if a Robot exemplar was presented in trials 2, 4 and 8, and a planet was presented in trials 7, 9 and 8, the average correlation corresponding to these items would contain activity of an overlapping trial (8 in the example). The correlation corresponding to these two items would therefore not be included. Note that this was not necessary for the same category correlations.
We quantified the similarity of neural representations by comparing epochs of brain activity separately in VVS and PFC. Note that contrary to the model-based RSA approach (see above) this analysis was not performed at each individual frequency but frequencies were grouped into five frequency bands. This effectively increased the information content (variance) of our representational patterns, making them more suitable to investigate their reoccurrence during encoding and maintenance. Moreover, combining individual frequencies into bands allowed us to reduce the dimensionality of the results when comparing all pairwise combinations of time points in the temporal generalization analysis. We computed the correlation of these representational patterns across all available time-points using a sliding time window approach proceeding in time steps of 100 ms (i.e., with an 80% overlap). This resulted in a temporal generalization matrix with two temporal dimensions on the vertical and horizontal axes (Fig. 2D ). Note that values in these matrices reflect both lagged (off-diagonal) and non-lagged (on-diagonal) correlations and were thus informative about the stability of neural representations over time 5 .
Pattern similarity maps were computed for each pair of items in a correspondent condition at each time-window and rho values in these maps were Fisher z-transformed for statistical analysis. The temporal generalization maps were averaged across conditions for each subject independently, and the resulting average maps were contrasted via paired t -tests across conditions at the group level.
Please note that in all pattern similarity plots (and also in the DNN-RSA plots, see below), correlations corresponding to each 500 ms window were assigned to the time point at the center of the respective window (e.g., a time bin corresponding to activity from 0 to 500 ms was assigned to 250 ms).
Please note that while the contrast-based and the model-based RSA analyses have been employed as complementary approaches to investigate neural representations 109 , they differ in two important aspects. The first distinction relates to the level at which the two methods assess similarities in the representations. While the model-based analysis captures differences in the representational geometry of stimuli (it correlates RSMs of neural data with RSMs of models, a second level analysis), the contrast-based analysis directly correlates neural patterns and is thus sensitive to reoccurrence and transformation of specific neural features. For example, the same representational distances (and thus RSMs) may depend on one particular brain region (i.e., set of electrodes) during encoding and a different brain region during maintenance, leading to significant RSM-based similarities in the absence of encoding-maintenance similarity (EMS). The model-based analyses, on the other hand, correlates representational distances during either encoding or maintenance with distances in particular RSM models, and does not directly compare levels of model fits between encoding and maintenance. Thus, in a strict sense, this approach does not directly test the reoccurrence of the representational geometry, but whether a particular geometry is present during a specific time period. To test for the reoccurrence of a particular representational geometry in the model-based analyses, we directly contrasted the different levels of fit during particular time periods using paired t -tests. A second difference relates to the specific neural features that were included in each analysis. The RSM-based analysis was conducted separately for each individual frequency in the 3–150 Hz range, which allowed for a fine-grained assessment of the contribution of individual frequencies. By contrast, feature vectors in the EMS analyses included power values across several individual frequencies within particular frequency bands, and thus contained higher variance. This was done in order to reduce dimensionality of the representational patterns and facilitate the process of multiple comparisons correction (see below). To corroborate that our results were not affected by differences in the frequency features that we selected in each analysis, we conducted the (RSM-based) category model analysis in the same frequency bands as the EMS analyses (theta, alpha, beta, low-gamma, high-gamma). Our results revealed a significant fit of the category model during encoding in all frequency bands in the VVS, and a more restricted fit in the PFC in the beta band ( p corr = 0.015, Bonferroni corrected for 5 bands; Supplementary Fig 13A ). During maintenance, we did not observe any significant fit in VVS or PFC in any band (VVS: all p corr = 1; PFC: all p corr = 1; Supplementary Fig 13B ).
RSA at high temporal resolution
We increased the temporal resolution of our sliding time window approach to compare the onset of category-specific information in VVS and PFC (Fig. 2E ). In this analysis, power values were computed with the same method and parameters as in the main contrast-based analysis, but at an increased temporal resolution (10 ms). Feature vectors were constructed in 500 ms time windows and the 50 time-points included in each window were averaged separately for electrodes and frequencies, resulting in a two-dimensional representational pattern. We included all individual frequencies in the 3–150 Hz range (a total of 52). These two-dimensional frequency x electrode vectors were concatenated into one-dimensional arrays for similarity analyses. We employed a sliding time-window approach with incremental steps of 10 ms resulting in an overlap of 490 ms between two consecutive windows, focusing only on matching time points (non-lagged correlations). We performed this analysis separately for the VVS and the PFC and assessed the statistical significance of the resulting time-series in each region. At each time point, we compared the group-level Fisher z-transformed rho values against zero. We also directly compared the values between PFC and VVS at the group level. Given that not all subjects had implanted electrodes in both of our two ROIs, we performed unpaired t -tests at each time-point. We corrected for multiple comparisons by applying cluster-based permutation statistics in the temporal dimension in all the pattern similarity analyses (see section Multiple Comparisons Correction below).
Feedforward and recurrent DNN models
We compared VWM representations in the iEEG data with those formed in two types of convolutional deep neural network (DNN) architectures: feedforward and recurrent DNNs. We used AlexNet 62 , a widely applied network in computational cognitive neuroscience to model visual perception and WM, as our feedforward model 36 , 57 . We also employed two recurrent convolutional DNNs: BL-NET, which has been recently applied to model human reaction times in a perceptual recognition task 71 , and corNET-RT, a network recently developed to model information processing in the primate ventral visual stream 72 . AlexNet is a deep convolutional feedforward neural network composed of five convolutional layers and 3 fully connected layers that simulates the hierarchical structure of neurons along the ventral visual stream. AlexNet was trained in the task of object identification, i.e., the assignment of object labels to visual stimuli, using the ImageNet dataset 110 . When learning to identify images, AlexNet develops layered representations of stimuli that hierarchically encode increasingly abstract visual properties: Early layers reflect low-level features of images such as edges or textures while deeper layers are sensitive to more complex visual information, such as the presence of objects or object parts. Several studies demonstrated the validity of AlexNet as a model of neural representations during biological vision, showing that it can capture relevant features of information processing in the VVS of humans during perceptual and mnemonic processing 36 , 58 , 64 . We computed RSMs at every convolutional and fully connected layers of the network, following previous work 57 , 58 .
The BL-NET is a deep recurrent convolutional neural network consisting of 7 convolutional layers with feedforward and lateral recurrent connections, followed by 7 batch normalization and RELU layers. Every unit in the BL-NET network receives lateral input from other units within feature maps. BL-NET has demonstrated high accuracy in the task of object recognition 71 after being trained with two large-scale image datasets (i.e., ImageNet and Ecoset 71 , 76 ;). We tested the network trained with these two different datasets in our analyses. Given that the output of each layer, which combines activity of lateral and feedforward connections, is computed at every single time-step in the RELU layers of the model, we selected these specific layers to compute the RSMs in our main analyses 71 . We obtained similar results when we compared the activations extracted from the convolutional layers (after batch normalization).
The corNET-RT network is another prominent example of recurrent architectures that have been employed to model neural activity in the VVS of primates. It comprises four layers designed to capture information processing in the main four VVS regions: V1, V2, V4, and IT. Like the BL-NET, corNET-RT exclusively incorporates lateral and not across area connectivity. Each layer of the network consists of an input and output convolutional layer, group normalization and RELU non-linearities. Unlike the BL-NET, the number of recurrent steps in each layer is not fixed but varies from 5 (in layer V1) to 2 (in layer IT). RSMs were computed specifically for the convolutional layers (we selected the output convolutional modules in each layer), although similar results were observed when RSMs were computed from the outputs of the non-linear layers.
BL-NET and corNET-RT are two of the most prominent task-performing convolutional DNN models for image classification that have introduced recurrence as a main architectural feature. These networks have shown improvements in performance as compared to parameter-matched feedforward networks in the complex task of object recognition 71 , 72 . Theoretical accounts and experimental findings have proposed that recurrent DNNs can better explain neural activity in the VVS and behavioral data than feedforward networks 33 , 59 , 69 , 72 , 111 . While previous studies characterized neural representations in humans using recurrent models in the domain of visual perception 33 , no study so far has used these types of architectures to model VWM, and no study has applied them to iEEG data.
Note that the BL-NET and the corNET-RT networks have different unrolling schemes across time, which affects how activity propagates through the networks. In BL-NET, feedforward and recurrent processing happen in parallel: a feedforward pass takes no time, while each recurrent step takes 1 time point. Thus, each layer receives a time-varying feedforward input. In corNET-RT, on the other hand, the onset of responses at deep layers is delayed when recurrence is engaged in earlier layers. These two approaches have been referred to as unrolling in ‘biological’ time (corNET-RT) vs ‘engineering’ time (BL-NET, see refs. 71 , 72 ).
Stimulus representations in DNNs
In order to analyze how the different DNN architectures represented the stimuli in our study, we presented the networks with our images and computed unit activations at each layer. We calculated Spearman’s correlations between the DNN features for every pair of pictures, resulting in a 60 × 60 representational similarity matrix (RSM) in each layer 47 . The AlexNet unit activations were computed using the Matlab Deep Learning Toolbox. Images were scaled to fit the 227 x 227 input layer of the network. The unit activations in the BL-NET network were extracted using the pipeline described in https://github.com/cjspoerer/rcnn-sat . Images were scaled to 128 × 128 pixels, and normalized to values between −1 and 1 to fit the input layer of the network as it was originally trained. The number of recurrent passes in the BL-NET architecture was set to 8 time-steps in each layer. We extracted the unit activations at each of these time points and computed RSMs, resulting in a total of 7 (layers) × 8 (time-points) = 56 RSMs. corNET-RT activations were extracted using TorchLens 112 , and we corroborated the results using the ‘TorchVision’ toolbox 73 . Images were z-scored to the mean and standard deviation of the ImageNet database and scaled to 224 × 224 pixels to match the training parameters of the network.
To visualize the representations of stimuli in our networks, we employed Multidimensional Scaling (MDS). MDS is a dimensionality reduction technique which exploits the geometric properties of RSMs, projecting the high-dimensional network activation patterns into lower-dimensional spaces. To apply the MDS algorithm to our RSMs, we subtracted the correspondent values in the matrix from 1 to obtain a distance metric and projected the data into two dimensions (Figs. 3 A, 4 E and 5E ).
Importantly, all three architectures we employed were trained with the ImageNet dataset, in which none of the categories included in our study (‘house’, ‘robot’, ‘hand’, ‘face’, ‘planet’, and ‘tree’) are present as object labels. For this reason, we did not focus our analysis on network classification performance but characterized categorical representations that were formed across layers, computing within-category, between-category correlations and their difference (CCI scores, see below). Moreover, we performed an additional control analysis involving a variant of BL-NET trained with the Ecoset dataset 76 , which contains part of our stimuli labels (i.e., labels ‘house’, ‘robot’ and ‘tree’) to corroborate our main results (Supplementary Fig 10 ).
The segregated representation of images according to their classes in deep layers of convolutional DNNs is a well-documented phenomenon 113 , 114 . This segregation however can be achieved by (1) grouping together items belonging to the same category, (2) separating items belonging to different categories, or (3) a combination of these two processes. To distinguish among these possibilities, we separately computed within-category and between-category correlations in all DNN layers. Results are presented in Figs. 3 C, 4 D and 5D , and in Supplementary Fig 5 . In addition to computing the representational geometry of stimuli across all layers of the networks, we quantified the similarity between RSMs across layers using Spearman’s Rho (a “second-level” similarity metric; see ref. 74 ). We applied MDS to visualize the similarity structure of the initial and last time points in each layer of BL-NET and Cornet-RT (Figs. 4 B and 5B ).
To quantify the amount of category information in the different layers of the networks, we computed a Category Cluster Index (CCI), defined as the difference of average within-category and between-category correlations in the DNN representations of the stimuli. Both within and across category correlation averages were computed after removing the diagonal of the RSM matrices (which only contains values of 1 by definition) and duplicated values due to the symmetry of the RSMs. CCI approaches 1 if representations in all categories are perfectly clustered and 0 if no categorical structure is present in the data 74 . We computed CCI at each layer of the AlexNet (Fig. 3C ), and for each time point in each layer of the BL-Net and the corNET-RT networks (Figs. 4 D and 5D ). To assess whether the observed CCI values were significant, we implemented a permutation procedure. We built a distribution of CCI values expected by chance by shuffling the trial labels of the network RSMs 1000 times and recomputing CCI values. We considered significant CCI values that exceeded the 95 th percentile of these null distributions.
In order to better characterize categorical representations in our networks and directly compare them, we performed a linear fit of within-category and between-category correlations across layers (Supplementary Fig 5 ). We specifically focused on the last time point in each layer in our recurrent architectures. We computed the correlation of the activations corresponding to every pair of items in each layer and performed a linear least-squares fit with the resulting values (270 within-category correlations and 1500 between-category correlations were computed in each layer). To evaluate whether correlations increased or decreased linearly across layers, we compared the distribution of slopes taken from the linear fit against zero (representing the null hypothesis of an average flat line) in each individual network. In addition, we compared these distributions across networks using paired t -tests.
Please note that given that two versions of the experiment were created for German and Chinese participants (with Angela Merkel and Jackie Chan as face stimuli, respectively), we passed through the networks two different datasets of images. For visualization of network RSMs and corresponding MDS plots (Figs. 3 A, 4 E and 5E ), we employed the German version of the stimuli. In the analyses focusing on the network representations, we generated independent statistics for the two stimuli sets and then averaged them. This applies to the plots showing the representational consistency of networks across layers and time-points, within- and between-category correlations and CCI scores (Figs. 3 B, C, 4 A–D, and 5A–D ).
Representational similarity analyses based on DNNs: modeling neural representations with deep neural networks
We compared the representations formed in the DNN architectures with the iEEG representations using RSA. Neural RSMs were constructed following the procedure described in the section Model-based RSA above.
Similar to the category model analysis, we performed a time-frequency resolved analysis of fits of neural and DNN-based RSMs. In this analysis, neural RSM time series (same time windows as described above) were computed with feature vectors comprising information of each individual frequency independently (e.g., for 3 Hz, 4 Hz, … 150 Hz). The resulting RSM time-series were correlated with network RSMs at each individual layer. Individual frequencies were extracted using the same parameters as in the category model and the contrast-based analyses (see section Time-frequency analysis ). The resulting time-series of correlation values were stacked into time-frequency maps of model fits. Significance was determined by contrasting the observed Fischer-Z transformed rho values against zero at the group level. Results were corrected for multiple comparisons using cluster-based permutation statistics and we additionally applied Bonferroni corrections across layers (see below).
We separately analyzed the fit of particular DNN models to the within-category and the between-category correlations in the neural data (Supplementary Fig 1 , Supplementary Fig 3 ). In these analyses, we excluded the within-category or the between-category correlations from both the neural data and the models before vectorizing the RSMs and computing the correlations.
Trial-based DNN analyses: correct vs incorrect
Since the number of incorrect trials was substantially lower than the number of correct trials in our data, directly comparing DNN correlations of the RSMs of incorrect vs. correct trials would be unbalanced. We thus computed a single-trial metric of model fits by correlating each row in the model RSMs and the neural RSMs independently 92 . Because of the unbalanced trial numbers, we then analyzed the match of these representations at all time-frequency points and for all DNN layers, instead of selecting the time periods where we had observed the original effects (which were mainly driven by the correct trials, given their larger number). This resulted in one time-frequency map of model fits for each trial. We averaged these trial-specific fits separately for correct and incorrect trials and in the two ROIs and evaluated whether there was a difference between correct and incorrect trials at all time-frequency bins. We only included subjects with at least 5 trials in each condition, leading to a total of 18 participants for VVS and 13 in PFC (paired t -tests were applied to the average time-frequency maps across conditions). Given the relevance of categorical information for PFC and VVS representations, trials in which the correct category of the cued item was reported were considered as correct, and trials in which subjects failed to retrieve the correct category were considered incorrect. This led to a total number of 13.22 ± 9.12 incorrect trials and 65.23 ± 30.64 correct trials in the VVS analysis (Mean ± STD), and of 11.77 ± 4.94 incorrect trials and 60.26 ± 26.55 correct trials in the PFC analysis (Mean ± STD).
Stress analysis
We performed Multidimensional Scaling (MDS) on the RSMs during encoding and maintenance at various levels of dimensionality and computed the stress value of the MDS projections. Stress (a.k.a. Stress-1) is a metric of the goodness of fit of a particular MDS projection that reflects how well a lower-dimensional embedding—in a specific dimension—reflects the structure of the high-dimensional data. Stress values are low if the data can be relatively well embedded in lower dimensions, and high if the embedding is less accurate. We performed the MDS analysis for all dimensions in the 1–60 range (corresponding to the size of the average RSM). For every subject, we converted the RSMs into distance matrices (1-correlation), performed MDS and computed stress in each time period. Given that the stress metric is sensitive to the number of dimensions of the distance matrix, we randomly removed items in RSMs to match the number of the condition with less items (some subjects had less than 60 trials during the maintenance period because some trials were removed during artifact rejection). This was done 100 times to corroborate that the results were not affected by the specific random selection of trials. We subsequently compared the group level stress values during encoding and maintenance for every dimension independently, and assessed regions of contiguous dimensions with significant differences between the two time periods. We applied cluster-based permutations statistics to control for multiple comparisons correction (see below).
Multiple comparisons corrections
We performed cluster-based permutation statistics to correct for multiple comparisons in the pattern similarity analyses (Fig. 2 ), in the RSA-DNN analyses (Figs. 3 – 5 ), and in the analysis of different levels of stress during encoding and maintenance (Supplementary Fig 1 ).
In the pattern similarity analyses, we applied cluster-based permutation statistics both for the temporal generalization analysis (Fig. 2 D, F ), and for the temporally resolved analysis (Fig. 2E ). For both analyses, we contrasted same and different category correlations at different time-points using t -tests, as in the main analysis, after shuffling the trial labels 1000 times. We considered significant a time-point if the difference between these surrogate conditions was significant at p < 0.05 (two-tailed tests were employed). At every permutation, we computed clusters of significant values defined as contiguous regions in time where significant correlations were observed and took the largest cluster at each permutation. Please note that in the temporal generalization analysis, time was defined in two dimensions and clusters were formed by grouping significant values across both of these dimensions, while in the temporally resolved analysis (Fig. 2E ), correlations were computed at matching time-points and clusters were formed along one temporal dimension. In both analyses, the permutation procedure resulted in a distribution of surrogate t -values under the assumption of the null hypothesis. We only considered significant those contiguous time pairs in the empirical (non-shuffled) data whose summed t -values exceeded the summed t -value of 95% of the distribution of surrogate clusters (corresponding to a corrected P < 0.05; see ref. 115 ).
We also performed cluster-based permutation statistics in the analysis at high temporal resolution in which we directly compared similarity values between VVS and PFC. In this analysis, we computed clusters of significant EES differences between the two regions for every time-point by applying unpaired t -tests. We repeated this analysis 1000 times after shuffling the region labels and kept the summed value of the largest cluster at every permutation. We only considered significant those clusters in the empirical data above the 95 th percentile of the shuffled distribution.
In the RSA-DNN analyses (and also in the category model RSA analysis, Fig. 2 ), we applied cluster-based permutation statistics. To determine the significance of the correlations between neural and model RSMs, we recalculated the model RSMs at each layer of the network after randomly shuffling the labels of the images. The surrogate model similarity matrices were then correlated with the neural similarity matrix 1000 times at all time-frequency pairs. As in the original analysis, we computed the correlations after removing the diagonal of the RSMs and only took half of the matrices given their symmetry. We identified clusters of contiguous windows in the time-frequency domain where the group-level correlations between neural and network RSMs were significantly different from zero at p < 0.05 (two-sided test) and selected the maximum cluster size of summed t -values for every permutation. This resulted in a distribution of surrogate t -values. The statistical significance was then determined by comparing the correlation values for the empirical data with the distribution of correlation values for the surrogate data (clusters whose summed t -values exceeded the 95% of the null distribution were considered significant).
In addition to cluster-based permutations, we also corrected our results for multiple comparisons using the Bonferroni method in the contrast-based RSA analyses (Fig. 2 ) and in the model-based RSA analyses (Figs. 2 , 3 , 4 , and 5 ). In the contrast-based analyses, given that we tested five different frequency bands, we only considered p -values significant that were below an alpha of 0.05/5. In the model-based analyses, we adjusted the significance threshold according to the correspondent number of layers in each network that was tested (AlexNet= 8; BL-NET = 7, corNET-RT = 4). The same correction by number of layers was applied in the CCI analysis (Figs. 3 C, 4 D and 5D ).
To correct for multiple comparisons in the correct versus incorrect trial level DNN fit analysis, we shuffled the condition labels (correct versus incorrect) 1000 times. At each permutation, we calculated the summed t-values of the significant differences between conditions with shuffled labels, resulting in a distribution of summed t -values under the null hypothesis. We then ranked the observed t -value with respect to this distribution to assess statistical significance.
In the analysis of different levels of stress during encoding and maintenance, we randomly shuffled the condition labels (encoding or maintenance) in each subject independently 1000 times and recomputed the condition differences. At each permutation, we summed the t -values of the largest cluster of significant dimensions, resulting in a distribution of t -values expected by chance. We ranked the observed t -values with respect to this null distribution to assess statistical significance.
Reporting summary
Further information on research design is available in the Nature Portfolio Reporting Summary linked to this article.
Data availability
Anonymized intracranial EEG data supporting the findings of this study have been deposited in the Open Science Framework ( https://osf.io/mw8cf/ ). Source data are provided with this paper.
Code availability
Custom-written Matlab and Python code supporting the findings of this study are available at https://github.com/dpachec/WM .
Myers, N. E., Stokes, M. G. & Nobre, A. C. Prioritizing information during working memory: beyond sustained internal attention. Trends Cogn. Sci. 21 , 449–461 (2017).
Article PubMed PubMed Central Google Scholar
Chatham, C. H., Frank, M. J. & Badre, D. Corticostriatal output gating during selection from working memory. Neuron 81 , 930–942 (2014).
Article CAS PubMed PubMed Central Google Scholar
Liebe, S., Hoerzer, G. M., Logothetis, N. K. & Rainer, G. Theta coupling between V4 and prefrontal cortex predicts visual short-term memory performance. Nat. Neurosci. 15 , 456–462 (2012).
Article CAS PubMed Google Scholar
Miller, E. K., Lundqvist, M. & Bastos, A. M. Working Memory 2.0. Neuron 100 , 463–475 (2018).
Stokes, M. G. et al. Dynamic coding for cognitive control in prefrontal cortex. Neuron 78 , 364–375 (2013).
Everling, S., Tinsley, C. J., Gaffan, D. & Duncan, J. Filtering of neural signals by focused attention in the monkey prefrontal cortex. Nat. Neurosci. 5 , 671–676 (2002).
Lepsien, J., Thornton, I. & Nobre, A. C. Modulation of working-memory maintenance by directed attention. Neuropsychologia 49 , 1569–1577 (2011).
Article PubMed Google Scholar
Lepsien, J. & Nobre, A. C. Cognitive control of attention in the human brain: Insights from orienting attention to mental representations. Brain Res. 1105 , 20–31 (2006).
Nee, D. E. & Jonides, J. Neural correlates of access to short-term memory. Proc. Natl Acad. Sci. 105 , 14228–14233 (2008).
Article ADS CAS PubMed PubMed Central Google Scholar
Nee, D. E. & Jonides, J. Common and distinct neural correlates of perceptual and memorial selection. Neuroimage 45 , 963–975 (2009).
Griffin, I. C. & Nobre, A. C. Orienting attention to locations in internal representations. J. Cogn. Neurosci. 15 , 1176–1194 (2003).
Posner, M. I. Orienting of attention. Q. J. Exp. Psychol. 32 , 3–25 (1980).
Schmidt, B. K., Vogel, E. K., Woodman, G. F. & Luck, S. J. Voluntary and automatic attentional control of visual working memory. Percept. Psychophys. 64 , 754–763 (2002).
Vogel, E. K. & Machizawa, M. G. Neural activity predicts individual differences in visual working memory capacity. Nature 428 , 748–751 (2004).
Article ADS CAS PubMed Google Scholar
Nelissen, N., Stokes, M., Nobre, A. C. & Rushworth, M. F. S. Frontal and parietal cortical interactions with distributed visual representations during selective attention and action selection. J. Neurosci. 33 , 16443–16458 (2013).
Higo, T., Mars, R. B., Boorman, E. D., Buch, E. R. & Rushworth, M. F. S. Distributed and causal influence of frontal operculum in task control. Proc. Natl Acad. Sci. 108 , 4230–4235 (2011).
Ester, E. F., Nouri, A. & Rodriguez, L. Retrospective cues mitigate information loss in human cortex during working memory storage. J. Neurosci. 38 , 8538–8548 (2018).
Sprague, T. C., Ester, E. F. & Serences, J. T. Restoring latent visual working memory representations in human cortex. Neuron 91 , 694–707 (2016).
Buschman, T. J. & Kastner, S. From behavior to neural dynamics: an integrated theory of attention. Neuron 88 , 127–144 (2015).
Buschman, T. J. & Miller, E. K. Top-down versus bottom-up control of attention in the prefrontal and posterior parietal cortices. science 315 , 1860–1862 (2007).
D’Esposito, M. From cognitive to neural models of working memory. Philos. Trans. R. Soc. B: Biol. Sci. 362 , 761–772 (2007).
Article Google Scholar
Nobre, A. C. et al. Orienting attention to locations in perceptual versus mental representations. J. Cogn. Neurosci. 16 , 363–373 (2004).
Wallis, G., Stokes, M., Cousijn, H., Woolrich, M. & Nobre, A. C. Frontoparietal and cingulo-opercular networks play dissociable roles in control of working memory. J. Cogn. Neurosci. 27 , 2019–2034 (2015).
Barak, O. & Tsodyks, M. Working models of working memory. Curr. Opin. Neurobiol. 25 , 20–24 (2014).
Wang, X. J. Synaptic reverberation underlying mnemonic persistent activity. Trends Neurosci. 24 , 455–463 (2001).
Mante, V., Sussillo, D., Shenoy, K. V. & Newsome, W. T. Context-dependent computation by recurrent dynamics in prefrontal cortex. Nature 503 , 78–84 (2013).
Ehrlich, D. B. & Murray, J. D. Geometry of neural computation unifies working memory and planning. Proc. Natl Acad. Sci. 119 , e2115610119 (2022).
Bouchacourt, F. & Buschman, T. J. A flexible model of working memory. Neuron 103 , 147–160 (2019).
Piwek, E. P., Stokes, M. G. & Summerfield, C. A recurrent neural network model of prefrontal brain activity during a working memory task. PLOS Comput. Biol. 19 , e1011555 (2023).
Wan, Q., Menendez, J. A. & Postle, B. R. Priority-based transformations of stimulus representation in visual working memory. PLoS Comput. Biol. 18 , e1009062 (2022).
Compte, A., Brunel, N., Goldman-Rakic, P. S. & Wang, X.-J. Synaptic Mechanisms and Network Dynamics Underlying Spatial Working Memory in a Cortical Network Model. Cereb. Cortex 10 , 910–923 (2000).
Kar, K. & DiCarlo, J. J. Fast recurrent processing via ventrolateral prefrontal cortex is needed by the primate ventral stream for robust core visual object recognition. Neuron 109 , 164–176 (2021).
Kietzmann, T. C. et al. Recurrence is required to capture the representational dynamics of the human visual system. Proc. Natl Acad. Sci. 116 , 21854–21863 (2019).
Breedlove, J. L., St-Yves, G., Olman, C. A. & Naselaris, T. Generative Feedback Explains Distinct Brain Activity Codes for Seen and Mental Images. Curr. Biol. 30 , 2211–2224.e6 (2020).
Lundqvist, M., Herman, P., Warden, M. R., Brincat, S. L. & Miller, E. K. Gamma and beta bursts during working memory readout suggest roles in its volitional control. Nat. Commun. 9 , 1–12 (2018).
Article CAS Google Scholar
Kuzovkin, I. et al. Activations of deep convolutional neural networks are aligned with gamma band activity of human visual cortex. Commun. Biol. 1 , 1–12 (2018).
Engel, A. K. & Fries, P. Beta-band oscillations—signalling the status quo? Curr. Opin. Neurobiol. 20 , 156–165 (2010).
Vezoli, J. et al. Brain rhythms define distinct interaction networks with differential dependence on anatomy. Neuron 109 , 3862–3878 (2021).
Buschman, T. J., Denovellis, E. L., Diogo, C., Bullock, D. & Miller, E. K. Synchronous oscillatory neural ensembles for rules in the prefrontal cortex. Neuron 76 , 838–846 (2012).
Antzoulatos, E. G. & Miller, E. K. Increases in functional connectivity between prefrontal cortex and striatum during category learning. Neuron 83 , 216–225 (2014).
Antzoulatos, E. G. & Miller, E. K. Synchronous beta rhythms of frontoparietal networks support only behaviorally relevant representations. elife 5 , e17822 (2016).
Stanley, D. A., Roy, J. E., Aoi, M. C., Kopell, N. J. & Miller, E. K. Low-beta oscillations turn up the gain during category judgments. Cereb. Cortex 28 , 116–130 (2018).
Spitzer, B., Wacker, Evelin & Blankenburg, Felix Oscillatory Correlates of Vibrotactile Frequency Processing in Human Working Memory. J. Neurosci. 30 , 4496 (2010).
Spitzer, B., Fleck, S. & Blankenburg, F. Parametric alpha-and beta-band signatures of supramodal numerosity information in human working memory. J. Neurosci. 34 , 4293–4302 (2014).
Wimmer, K., Ramon, Marc, Pasternak, Tatiana & Compte, Albert Transitions between Multiband Oscillatory Patterns Characterize Memory-Guided Perceptual Decisions in Prefrontal Circuits. J. Neurosci. 36 , 489 (2016).
Spitzer, B. & Haegens, S. Beyond the Status Quo: A Role for Beta Oscillations in Endogenous Content (Re)Activation. eNeuro 4 , ENEURO.0170-17.2017 (2017).
Kriegeskorte, N., Mur, M. & Bandettini, P. A. Representational similarity analysis-connecting the branches of systems neuroscience. Front. Syst. Neurosci. 2 , 249 (2008).
Kriegeskorte, N. & Diedrichsen, J. Peeling the Onion of Brain Representations. Annu Rev. Neurosci. 42 , 407–432 (2019).
Pacheco Estefan, D. et al. Coordinated representational reinstatement in the human hippocampus and lateral temporal cortex during episodic memory retrieval. Nat. Commun. 10 , 1–13 (2019).
Pacheco Estefan, D. et al. Volitional learning promotes theta phase coding in the human hippocampus. Proc. Natl Acad. Sci. 118 , e2021238118 (2021).
Axmacher, N. Representational formats in medial temporal lobe and neocortex also determine subjective memory features. Behav. Brain Sci. 42 , e283 (2020).
Heinen, R., Bierbrauer, A., Wolf, O. T. & Axmacher, N. Representational formats of human memory traces. Brain Struct Funct https://doi.org/10.1007/s00429-023-02636-9 (2023).
Kwak, Y. & Curtis, C. E. Unveiling the abstract format of mnemonic representations. Neuron 110 , 1822–1828 (2022).
Tang, W., Shin, J. D. & Jadhav, S. P. Geometric transformation of cognitive maps for generalization across hippocampal-prefrontal circuits. Cell Rep. 42 , 112246 (2023).
Wu, X. & Fuentemilla, L. Distinct encoding and post-encoding representational formats contribute to episodic sequence memory formation. Cereb Cortex bhad138 https://doi.org/10.1093/cercor/bhad138 (2023).
Xue, G. From remembering to reconstruction: The transformative neural representation of episodic memory. Prog. Neurobiol. 219 , 102351 (2022).
Liu, J. et al. Stable maintenance of multiple representational formats in human visual short-term memory. Proc. Natl Acad. Sci. 117 , 32329–32339 (2020).
Liu, J. et al. Transformative neural representations support long-term episodic memory. Sci. Adv. 7 , eabg9715 (2021).
Article ADS PubMed PubMed Central Google Scholar
Kar, K., Kubilius, J., Schmidt, K., Issa, E. B. & DiCarlo, J. J. Evidence that recurrent circuits are critical to the ventral stream’s execution of core object recognition behavior. Nat. Neurosci. 22 , 974–983 (2019).
van Bergen, R. S. & Kriegeskorte, N. Going in circles is the way forward: the role of recurrence in visual inference. Curr. Opin. Neurobiol. 65 , 176–193 (2020).
Kerren, C., Linde-Domingo, J. & Spitzer, B. Prioritization of semantic over visuo-perceptual aspects in multi-item working memory. bioRxiv (2022).
Krizhevsky, A., Sutskever, I. & Hinton, G. E. Imagenet classification with deep convolutional neural networks. Commun. ACM 60 , 84–90 (2012).
Baek, S., Song, M., Jang, J., Kim, G. & Paik, S.-B. Face detection in untrained deep neural networks. Nat. Commun. 12 , 7328 (2021).
Bao, P., She, L., McGill, M. & Tsao, D. Y. A map of object space in primate inferotemporal cortex. Nature 583 , 103–108 (2020).
Cadieu, C. F. et al. Deep neural networks rival the representation of primate IT cortex for core visual object recognition. PLoS Comput. Biol. 10 , e1003963 (2014).
Cichy, R. M., Khosla, A., Pantazis, D., Torralba, A. & Oliva, A. Comparison of deep neural networks to spatio-temporal cortical dynamics of human visual object recognition reveals hierarchical correspondence. Sci. Rep. 6 , 27755 (2016).
Khaligh-Razavi, S.-M. & Kriegeskorte, N. Deep supervised, but not unsupervised, models may explain IT cortical representation. PLoS Comput.Biol. 10 , e1003915 (2014).
Lindsay, G. W. Convolutional neural networks as a model of the visual system: Past, present, and future. J. Cogn. Neurosci. 33 , 2017–2031 (2021).
Tang, H. et al. Recurrent computations for visual pattern completion. Proc. Natl Acad. Sci. 115 , 8835–8840 (2018).
Vinken, K. & Op de Beeck, H. Using deep neural networks to evaluate object vision tasks in rats. PLOS Comput. Biol. 17 , e1008714 (2021).
Spoerer, C. J., Kietzmann, T. C., Mehrer, J., Charest, I. & Kriegeskorte, N. Recurrent neural networks can explain flexible trading of speed and accuracy in biological vision. PLoS Comput. Biol. 16 , e1008215 (2020).
Kubilius, J. et al. Cornet: Modeling the neural mechanisms of core object recognition. BioRxiv 408385 (2018).
Muttenthaler, L. & Hebart, M. N. THINGSvision: A Python Toolbox for Streamlining the Extraction of Activations From Deep Neural Networks. Front. Neuroinform. 15 , 679838 (2021).
Mehrer, J., Spoerer, C. J., Kriegeskorte, N. & Kietzmann, T. C. Individual differences among deep neural network models. Nat. Commun. 11 , 1–12 (2020).
McKee, J. L., Riesenhuber, M., Miller, E. K. & Freedman, D. J. Task dependence of visual and category representations in prefrontal and inferior temporal cortices. J. Neurosci. 34 , 16065–16075 (2014).
Mehrer, J., Spoerer, C. J., Jones, E. C., Kriegeskorte, N. & Kietzmann, T. C. An ecologically motivated image dataset for deep learning yields better models of human vision. Proc. Natl Acad. Sci. 118 , e2011417118 (2021).
Spitzer, B. & Blankenburg, F. Stimulus-dependent EEG activity reflects internal updating of tactile working memory in humans. Proc. Natl Acad. Sci. 108 , 8444–8449 (2011).
Eichenbaum, H. Memory Organization and Control. Annu Rev. Psychol. 68 , 19–45 (2017).
Rissman, J. & Wagner, A. D. Distributed representations in memory: Insights from functional brain imaging. Annu. Rev. Psychol. 63 , 101–128 (2012).
Ten Oever, S., Sack, A. T., Oehrn, C. R. & Axmacher, N. An engram of intentionally forgotten information. Nat. Commun. 12 , 6443 (2021).
Lundqvist, M., Miller, E. K., Nordmark, J., Liljefors, J. & Herman, P. Beta: bursts of cognition. Trends Cognit. Sci. https://doi.org/10.1016/j.tics.2024.03.010 (2024).
Yamins, D. L. et al. Performance-optimized hierarchical models predict neural responses in higher visual cortex. Proc. Natl Acad. Sci. 111 , 8619–8624 (2014).
Yamins, D. L. & DiCarlo, J. J. Using goal-driven deep learning models to understand sensory cortex. Nat. Neurosci. 19 , 356–365 (2016).
Miller, E. K. & Cohen, J. D. An integrative theory of prefrontal cortex function. Annu Rev. Neurosci. 24 , 167–202 (2001).
Gelastopoulos, A., Whittington, M. A. & Kopell, N. J. Parietal low beta rhythm provides a dynamical substrate for a working memory buffer. Proc. Natl Acad. Sci. 116 , 16613–16620 (2019).
Panichello, M. F. & Buschman, T. J. Shared mechanisms underlie the control of working memory and attention. Nature 592 , 601–605 (2021).
Weber, J. et al. Subspace partitioning in the human prefrontal cortex resolves cognitive interference. Proc. Natl Acad. Sci. 120 , e2220523120 (2023).
Conwell, C., Jacob S. Prince, Kendrick N. Kay, George A. Alvarez, & Talia Konkle. What can 1.8 billion regressions tell us about the pressures shaping high-level visual representation in brains and machines? bioRxiv https://doi.org/10.1101/2022.03.28.485868 (2023).
Barense, M. D. & Lee, A. C. H. Perception and memory in the medial temporal lobe: Deep learning offers a new lens on an old debate. Neuron 109 , 2643–2645 (2021).
Cowell, R. A., Barense, M. D. & Sadil, P. S. A Roadmap for Understanding Memory: Decomposing Cognitive Processes into Operations and Representations. eNeuro 6 , ENEURO.0122-19.2019 (2019).
Murray, E. A., Bussey, T. J. & Saksida, L. M. Visual perception and memory: a new view of medial temporal lobe function in primates and rodents. Annu. Rev. Neurosci. 30 , 99–122 (2007).
Davis, S. W. et al. Visual and Semantic Representations Predict Subsequent Memory in Perceptual and Conceptual Memory Tests. Cereb. Cortex 31 , 974–992 (2021).
Caucheteux, C. & King, J.-R. Brains and algorithms partially converge in natural language processing. Commun. Biol. 5 , 134 (2022).
Goldstein, A. et al. Shared computational principles for language processing in humans and deep language models. Nat. Neurosci. 25 , 369–380 (2022).
Goldstein, A. et al. Alignment of brain embeddings and artificial contextual embeddings in natural language points to common geometric patterns. Nat. Commun. 15 , 2768 (2024).
Schrimpf, M. et al. The neural architecture of language: Integrative modeling converges on predictive processing. Proc. Natl Acad. Sci. 118 , e2105646118 (2021).
Tuckute, G. et al. Driving and suppressing the human language network using large language models. Nat. Hum. Behav. 8 , 544–561 (2024).
Sörensen, L. K. A., Bohté, S. M., de Jong, D., Slagter, H. A. & Scholte, H. S. Mechanisms of human dynamic object recognition revealed by sequential deep neural networks. PLOS Comput. Biol. 19 , e1011169 (2023).
Brookes, M. J. et al. Changes in brain network activity during working memory tasks: a magnetoencephalography study. Neuroimage 55 , 1804–1815 (2011).
Stolk, A. et al. Integrated analysis of anatomical and electrophysiological human intracranial data. Nat. Protoc. 13 , 1699–1723 (2018).
Cichy, R. M., Pantazis, D. & Oliva, A. Resolving human object recognition in space and time. Nat. Neurosci. 17 , 455–462 (2014).
D’Esposito, M. et al. The neural basis of the central executive system of working memory. Nature 378 , 279–281 (1995).
Article ADS PubMed Google Scholar
Wallis, J. D., Anderson, K. C. & Miller, E. K. Single neurons in prefrontal cortex encode abstract rules. Nature 411 , 953–956 (2001).
Cromer, J. A., Roy, J. E. & Miller, E. K. Representation of Multiple, Independent Categories in the Primate Prefrontal Cortex. Neuron 66 , 796–807 (2010).
Delorme, A. & Makeig, S. EEGLAB: an open source toolbox for analysis of single-trial EEG dynamics including independent component analysis. J. Neurosci. Methods 134 , 9–21 (2004).
Oostenveld, R., Fries, P., Maris, E. & Schoffelen, J.-M. FieldTrip: Open source software for advanced analysis of MEG, EEG, and invasive electrophysiological data. Comput. Intell. Neurosci. 2011 , 156869 (2011).
Staresina, B. P. et al. Hippocampal pattern completion is linked to gamma power increases and alpha power decreases during recollection. eLife 5 , e17397 (2016).
Fellner, M. C., Waldhauser, G. T. & Axmacher, N. Tracking Selective Rehearsal and Active Inhibition of Memory Traces in Directed Forgetting. Curr. Biol. 30 , 2638–2644.e4 (2020).
Reagh, Z. M. & Ranganath, C. Flexible reuse of cortico-hippocampal representations during encoding and recall of naturalistic events. Nat. Commun. 14 , 1279 (2023).
Deng, J. et al. Imagenet: A large-scale hierarchical image database. in 248–255 (Ieee, 2009).
Nayebi, A. et al. Task-driven convolutional recurrent models of the visual system. Adv. Neural Inform. Process. Syst. 31 , 5290–5301 (2018).
Taylor, J. & Kriegeskorte, N. Extracting and visualizing hidden activations and computational graphs of PyTorch models with TorchLens. Sci. Rep. 13 , 14375 (2023).
Cichy, R. M. & Kaiser, D. Deep neural networks as scientific models. Trends Cogn. Sci. 23 , 305–317 (2019).
Kriegeskorte, N. Deep Neural Networks: A New Framework for Modeling Biological Vision and Brain Information Processing. Annu Rev. Vis. Sci. 1 , 417–446 (2015).
Maris, E. & Oostenveld, R. Nonparametric statistical testing of EEG- and MEG-data. J. Neurosci. Methods 164 , 177–190 (2007).
Download references
Acknowledgements
We would like to acknowledge DFG funding via the ORA project “WMREPS Hidden brain states underlying efficient representations in working memory” (project number 396894956). N.A. also acknowledges DFG funding via the SFB 1280, project number 316803389. This project was a collaboration with Mark Stokes (Oxford) and Elkan Akyürek (Groningen) and is dedicated to the memory of Prof. Stokes.
Open Access funding enabled and organized by Projekt DEAL.
Author information
These authors contributed equally: Daniel Pacheco-Estefan, Marie-Christin Fellner.
Authors and Affiliations
Department of Neuropsychology, Institute of Cognitive Neuroscience, Faculty of Psychology, Ruhr University Bochum, 44801, Bochum, Germany
Daniel Pacheco-Estefan, Marie-Christin Fellner, Hui Zhang & Nikolai Axmacher
Department of Epileptology, University Hospital Bonn, Bonn, Germany
Department of Stereotactic and Functional Neurosurgery, Medical Center – Faculty of Medicine, University of Freiburg, Freiburg, Germany
Peter Reinacher
Fraunhofer Institute for Laser Technology, Aachen, Germany
Epilepsy Center, Medical Center – Faculty of Medicine, University of Freiburg, Freiburg, Germany
Charlotte Roy, Armin Brandt & Andreas Schulze-Bonhage
Department of Psychiatry, Second Affiliated Hospital, School of medicine, Zhejiang University, Hangzhou, China
Linglin Yang
Department of Neurology, Epilepsy center, Second Affiliated Hospital, School of medicine, Zhejiang University, Hangzhou, China
Shuang Wang
Department of Applied Social Sciences, The Hong Kong Polytechnic University, Hong Kong, Hong Kong SAR
State Key Laboratory of Cognitive Neuroscience and Learning and IDG/McGovern Institute for Brain Research, Beijing Normal University, Beijing, 100875, PR China
Gui Xue & Nikolai Axmacher
You can also search for this author in PubMed Google Scholar
Contributions
Conceptualization, M.-C.F., D.P.-E. and N.A.; methodology, D.P.-E., H.Z., L.K., G.X. and N.A.; data collection, M.-C.F., L.K., C.R., A.B., L.Y., S.W. and J.L.; data analysis: D.P.-E. and N.A.; writing—original draft, D.P.-E.; writing—review and editing, D.P.-E. and N.A.; funding acquisition, N.A.; resources, L.K., H.Z., P.R., A.B., A.S.-B., L.Y., S.W. and G.X.; supervision N.A.
Corresponding author
Correspondence to Daniel Pacheco-Estefan .
Ethics declarations
Competing interests.
The authors declare no competing interest.
Peer review
Peer review information.
Nature Communications thanks the anonymous reviewer(s) for their contribution to the peer review of this work. A peer review file is available.
Additional information
Publisher’s note Springer Nature remains neutral with regard to jurisdictional claims in published maps and institutional affiliations.
Supplementary information
Supplementary information, peer review file, reporting summary, source data, source data, rights and permissions.
Open Access This article is licensed under a Creative Commons Attribution 4.0 International License, which permits use, sharing, adaptation, distribution and reproduction in any medium or format, as long as you give appropriate credit to the original author(s) and the source, provide a link to the Creative Commons licence, and indicate if changes were made. The images or other third party material in this article are included in the article’s Creative Commons licence, unless indicated otherwise in a credit line to the material. If material is not included in the article’s Creative Commons licence and your intended use is not permitted by statutory regulation or exceeds the permitted use, you will need to obtain permission directly from the copyright holder. To view a copy of this licence, visit http://creativecommons.org/licenses/by/4.0/ .
Reprints and permissions
About this article
Cite this article.
Pacheco-Estefan, D., Fellner, MC., Kunz, L. et al. Maintenance and transformation of representational formats during working memory prioritization. Nat Commun 15 , 8234 (2024). https://doi.org/10.1038/s41467-024-52541-w
Download citation
Received : 28 September 2023
Accepted : 11 September 2024
Published : 19 September 2024
DOI : https://doi.org/10.1038/s41467-024-52541-w
Share this article
Anyone you share the following link with will be able to read this content:
Sorry, a shareable link is not currently available for this article.
Provided by the Springer Nature SharedIt content-sharing initiative
By submitting a comment you agree to abide by our Terms and Community Guidelines . If you find something abusive or that does not comply with our terms or guidelines please flag it as inappropriate.
Quick links
- Explore articles by subject
- Guide to authors
- Editorial policies
Sign up for the Nature Briefing newsletter — what matters in science, free to your inbox daily.


COMMENTS
The working memory model proposes that short-term memory is a multi-component system that includes the central executive, visuospatial sketchpad, phonological loop, and episodic buffer. The phonological loop is responsible for the manipulation of speech-based information, and the visuospatial sketchpad is responsible for the manipulation of visual images.
Learn about Baddeley and Hitch's Working Memory model, which includes the Phonological Loop, the Visuo Spatial Sketchpad and the Central Executive. Find out how Working Memory is applied, evaluated and improved with research examples and a patient case study.
Learn about the WMM, which proposes that STM is composed of three limited capacity stores: central executive, articulatory-phonological loop and visuo-spatial sketchpad. Find out how the WMM explains parallel processing, and how it was supported by the case study of brain-damaged patient KF.
The peculiar case of KF. You probably know about the famous case study of HM - a man who lost the ability to make new memories. HM's study supports the claim that short-term and long-term memory are different stores because HM could hold information in his STS but he could not make new memories (i.e. he could not transfer the information ...
The working memory model is supported by evidence from brain damaged patients such as KF. ... A case study using numerous psychometric tests, experiments and observations. ... Results . KF's short term memory problems were much greater for auditory information than visual, suggesting his brain damage was restricted to the phonological loop ...
Learn about the multi-store model, types of long-term memory, working memory, forgetting, and eyewitness testimony for A-level psychology. Find exam tips, model answers, and research studies to help you revise and prepare for your exams.
Learn how KF, a brain-damaged patient, supports the working memory model proposed by Baddeley and Hitch. The model suggests that working memory consists of three components: phonological loop, visuospatial sketchpad and central executive.
KF was a patient with severe verbal short-term memory impairment but intact long-term memory. This case study by Warrington and Shallice (1969) investigated the possibility of separate memory stores and the nature of short-term memory processes.
Learn about the dual-task technique used by Baddeley and Hitch (1974) to test their Working Memory Model (WMM), which consists of four components: Central Executive, Phonological Loop, Visuo-Spatial Sketchpad and Episodic Buffer. Find out the strengths and weaknesses of the WMM and how it differs from the Multi-Store Model.
Learn about the working memory model of memory proposed by Baddeley and Hitch (1979), which consists of three components: central executive, visuo-spatial sketchpad and phonological loop. Find out how this model is supported by research and what criticisms it faces.
Revision notes on 2.2.1 Working Memory Model for the AQA A Level Psychology syllabus, written by the Psychology experts at Save My Exams. ... Religious Studies. Revision Notes; Past Papers A (8062) Past Papers B (8063) Religious Studies Short Course. Past Papers; Edexcel. Religious Studies A.
Case studies to evaluate WMM. KF supports the idea of the Visuo-Spatial sketchpad separate from Verbal STM (against MSM and for WMM) LH who had good spatial but poor visual memory for objects and faces ... Describe working memory model with reference to one research. (9) ...
The VSS deals with visual and spatial information sent from LTM and sensory memory. The KF case study provides evidence for the WMM by demonstrating that the VSS and phonological loop are separate components. ... (maintaining the memory over long periods of time), and retrieval (recalling the memory), of information. The working memory model ...
The multi-store model predicts that if people have damage to their short-term memory, then they will also have damage to their long-term memory.But patients like patient KF have damage to their short-term memory without damage to their long-term memory.So, the first limitation of the multi-store model is that it isn't supported by findings from case studies.
Learn how the working memory model explains short-term memory as a system of three components: the central executive, the phonological loop, and the visuospatial sketchpad. Find out how this model differs from the multi-store model and how it can be applied in everyday life.
Study with Quizlet and memorise flashcards containing terms like Working memory model (WMM), Central executive, Phonological Loop and others. ... KF case study (Shallice and Warrington) 5 terms. ALEXANDRIUSHUBBARUS. Preview. Shallice & Warrington K.F. case study. 5 terms. nurettingunduz.
One strength of the working memory model is the support of evidence for the working memory model.The KF case study supports the working memory model whereby KF suffered brain damage from a motorcycle accident. As a result, his memory for verbal information was greatly impaired but his visual memory was relatively unaffected. This suggests and supports that verbal and visual memory's is ...
Luckily, the working memory model is also supported by other types of research, which we'll see in more detail next. But first, to recap… Case studies of patients like patient KF support the working memory model, because these studies suggest there are multiple short-term memory stores.
More videos on The Working Memory Model. Introduction . Limitations of the Multi-store Model: Patient KF Case Study. Limitations of the Multi-store Model: Short-term Memory Stores . Limitations of the Multi-store Model: the Role of Rehearsal . Progress Quiz: Limitations of the Multi-store Model. The Working Memory Model
Study with Quizlet and memorise flashcards containing terms like How is this model credible?, What did KF struggle to process? What was unaffected?, What does the KF case study show? and others.
The multi-store model of memory (also known as the modal model) was proposed by Richard Atkinson and Richard Shiffrin (1968) and is a structural model. ... Other compelling evidence to support this distinction between STM and LTM is the case of KF (Shallice & Warrington, 1977) who had been in a motorcycle crash where he had sustained brain ...
Evaluating the working memory model. 0.0 / 5? Created by: maddieecarr; Created on: 13-06-21 14:11; Evaluating the working memory model. Advantages. Clinical evidence - KF case study: he could not process auditory information but could process visual information. This supports the WMM as it shows there are separate stores for processing these ...
Computational studies have shown that recurrence is crucial for the selection and integration of task-relevant features in the PFC 26, the integration of working memory and planning 27, the ...