- For educators
- English (US)
- English (India)
- English (UK)
- Greek Alphabet

This problem has been solved!
You'll get a detailed solution from a subject matter expert that helps you learn core concepts.
Question: 9.1 image Labeling
Not the question you’re looking for?
Post any question and get expert help quickly.
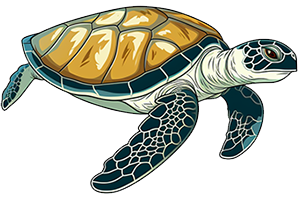
The Biology Corner
Biology Teaching Resources

Label the Eye
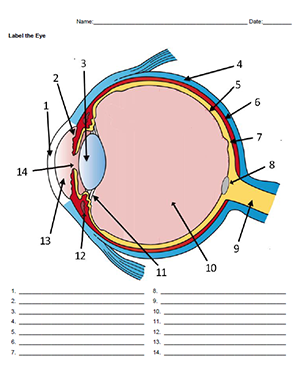
This worksheet shows an image of the eye with structures numbered. Students practice labeling the eye or teachers can print this to use as an assessment.
There are two versions on the google doc and pdf file, one where the word bank is included and another with no word bank for differentiation. You could also print both and students could practice with the word bank first and then try without.
The image was modified from an eye diagram at Wikimedia Commons . I added the numbers and additional errors to identify structures that weren’t on the original diagram.
You can also download this version of the same activity where students drag and drop labels to an image. The images are on google slides, which makes it easy to assign on Google Classroom.
There are a few terms that can be vague, for example, the aqueous humor could also be labeled as the aqueous chamber. Zonule of Zinn can also be called suspensory ligaments.
You could also have the students list the general functions of each of the structures shown as part of their assignment.
Shannan Muskopf
Human Anatomy & Physiology: A&P Chapter 11 Fundamentals of the Nervous System and Nervous Tissue Flashcards
Drag the appropriate labels to their respective targets.
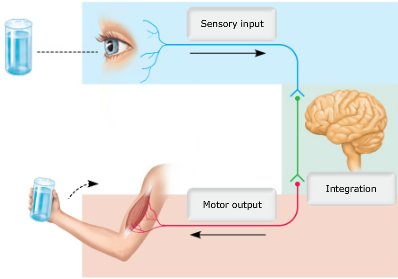
What structural classification describes the neuron associated with the neuroglia shown by E and F?
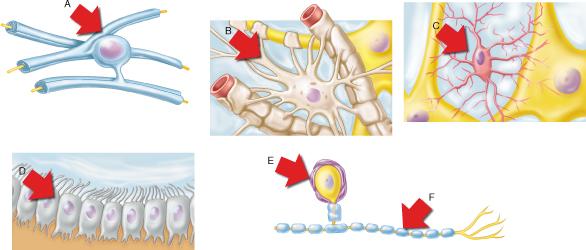
Destruction of which of the neuroglial cell types leads to the disease multiple sclerosis (MS)?
Which lettered region in the figure is referred to as the soma ?
Where do most action potentials originate?
Initial segment
What opens first in response to a threshold stimulus?
Voltage-gated Na + channels
What characterizes depolarization, the first phase of the action potential?
The membrane potential changes from a negative value to a positive value.
What characterizes repolarization, the second phase of the action potential?
Once the membrane depolarizes to a peak value of +30 mV, it repolarizes to its negative resting value of -70 mV.
What event triggers the generation of an action potential?
The membrane potential must depolarize from the resting voltage of -70 mV to a threshold value of -55 mV.
What is the first change to occur in response to a threshold stimulus?
Voltage-gated Na + channels change shape, and their activation gates open.
What type of conduction takes place in unmyelinated axons?
Continuous conduction
An action potential is self-regenerating because __________.
depolarizing currents established by the influx of Na + flow down the axon and trigger an action potential at the next segment
Why does regeneration of the action potential occur in one direction, rather than in two directions?
The inactivation gates of voltage-gated Na + channels close in the node, or segment, that has just fired an action potential.
What is the function of the myelin sheath?
The myelin sheath increases the speed of action potential conduction from the initial segment to the axon terminals.
What changes occur to voltage-gated Na + and K + channels at the peak of depolarization?
Inactivation gates of voltage-gated Na + channels close, while activation gates of voltage-gated K + channels open.
In which type of axon will velocity of action potential conduction be the fastest?
Myelinated axons with the largest diameter
Ions are unequally distributed across the plasma membrane of all cells. This ion distribution creates an electrical potential difference across the membrane. What is the name given to this potential difference?
Resting membrane potential (RMP)
Sodium and potassium ions can diffuse across the plasma membranes of all cells because of the presence of what type of channel?
Leak channels
On average, the resting membrane potential is -70 mV. What does the sign and magnitude of this value tell you?
The inside surface of the plasma membrane is much more negatively charged than the outside surface.
The plasma membrane is much more permeable to K + than to Na + . Why?
There are many more K + leak channels than Na + leak channels in the plasma membrane.
The resting membrane potential depends on two factors that influence the magnitude and direction of Na + and K + diffusion across the plasma membrane. Identify these two factors.
The presence of concentration gradients and leak channels
What prevents the Na + and K + gradients from dissipating?
Na + -K + ATPase
Which of the following describes the nervous system integrative function?
analyzes sensory information, stores information, makes decisions
Which of the following types of glial cells monitors the health of neurons, and can transform into a special type of macrophage to protect endangered neurons?
Which of the following are bundles of neurofilaments that are important in maintaining the shape and integrity of neurons?
neurofibrils
Which of the following is true of axons?
A neuron can have only one axon, but the axon may have occasional branches along its length.
Which of the following is NOT a functional classification of neurons?
Which of the following is NOT true of association neurons?
Most association neurons are confined within the peripheral nervous system (PNS).
Neurons are also called nerve cells.
Unmyelinated fibers conduct impulses faster than myelinated fibers.
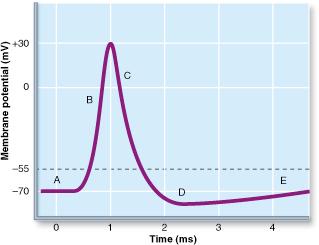
At which point of the illustrated action potential are the most gated Na + channels open?
In myelinated axons the voltage-regulated sodium channels are concentrated at the nodes of Ranvier.
Collections of nerve cell bodies in the peripheral nervous system are called ________.
What does the central nervous system use to determine the strength of a stimulus?
frequency of action potentials
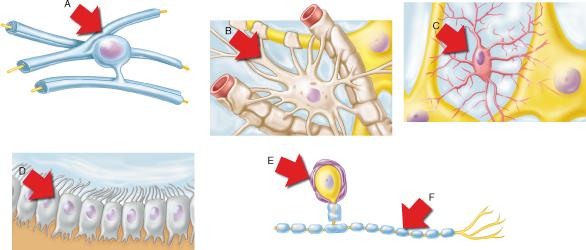
Which of the neuroglial cell types shown form myelin sheaths within the CNS?
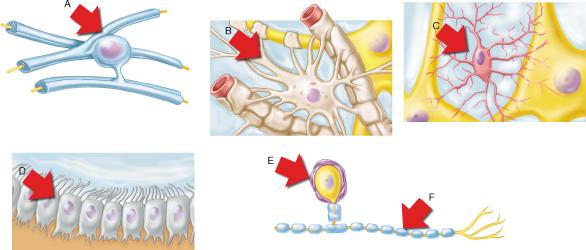
Which of the neuroglial cell types shown is the most abundant in the CNS?
Saltatory conduction is made possible by ________.
the myelin sheath
Large-diameter nerve fibers conduct impulses much faster than small-diameter fibers.
The part of a neuron that conducts impulses away from its cell body is called a(n) ________.
Schwann cells are functionally similar to ________.
oligodendrocytes
Bipolar neurons are commonly ________.
found in the retina of the eye
If bacteria invaded the CNS tissue, microglia would migrate to the area to engulf and destroy them.
Myelination of the nerve fibers in the central nervous system is the job of the oligodendrocyte.
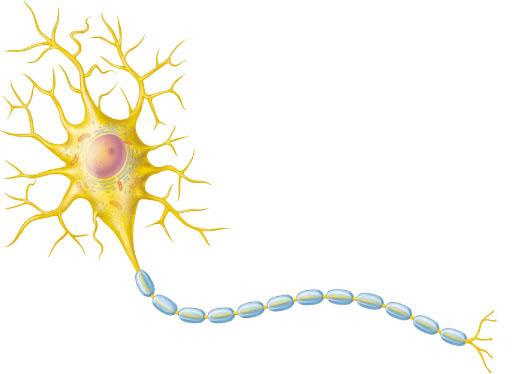
What structural classification describes this neuron?
What part of the nervous system performs information processing and integration?
central nervous system
Which of the following types of neurons carry impulses away from the central nervous system (CNS)?
Efferent nerve fibers may be described as motor nerve fibers.
The period after an initial stimulus when a neuron is not sensitive to another stimulus is the ________.
absolute refractory period
Which of the following is NOT a type of circuit?
pre-discharge circuits
Which part of the neuron is responsible for generating a nerve impulse?
What are ciliated CNS neuroglia that play an active role in moving the cerebrospinal fluid called?
ependymal cells
Which of the following is NOT one of the basic functions of the nervous system?
regulation of neurogenesis
The oligodendrocytes can myelinate several axons.
Immediately after an action potential has peaked, which cellular gates open?
What type of stimulus is required for an action potential to be generated?
a threshold level depolarization
Which of the following is not a function of the autonomic nervous system?
innervation of skeletal muscle
A neuron that has as its primary function the job of connecting other neurons is called a(n) ________.
association neuron
Which of the following is a factor that determines the rate of impulse propagation, or conduction velocity, along an axon?
degree of myelination of the axon
Neurons in the CNS are organized into functional groups.
Which of the following peripheral nervous system (PNS) neuroglia form the myelin sheaths around larger nerve fibers in the PNS?
Schwann cells
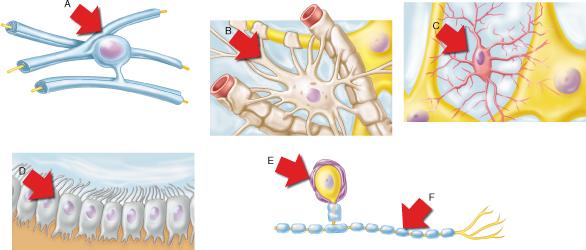
Neuroglia that control the chemical environment around neurons by buffering ions such as potassium and recapturing and recycling neurotransmitters are ________.
The action potential is caused by permeability changes in the plasma membrane.
Which of the choices below describes the ANS?
motor fibers that conduct nerve impulses from the CNS to smooth muscle, cardiac muscle, and glands
Which of the following is not characteristic of neurons?
They are mitotic.
Which ion channel opens in response to a change in membrane potential and participates in the generation and conduction of action potentials?
voltage-gated channel
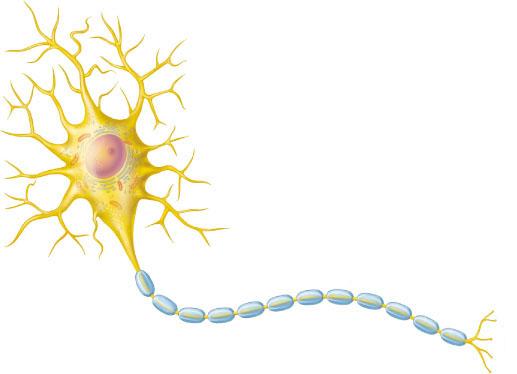
Which of the following circuit types is involved in the control of rhythmic activities such as the sleep-wake cycle, breathing, and certain motor activities (such as arm swinging when walking)?
reverberating circuits
The __________ is due to the difference in K + and Na + concentrations on either side of the plasma membrane, and the difference in permeability of the membrane to these ions.
resting membrane potential
During depolarization, the inside of the neuron's membrane becomes less negative.
The all-or-none phenomenon as applied to nerve conduction states that the whole nerve cell must be stimulated for conduction to take place.
Image Labeling by Assignment
- Published: 12 January 2017
- Volume 58 , pages 211–238, ( 2017 )
Cite this article
- Freddie Åström 1 ,
- Stefania Petra 2 ,
- Bernhard Schmitzer 3 &
- Christoph Schnörr ORCID: orcid.org/0000-0002-8999-2338 4
1028 Accesses
45 Citations
1 Altmetric
Explore all metrics
We introduce a novel geometric approach to the image labeling problem. Abstracting from specific labeling applications, a general objective function is defined on a manifold of stochastic matrices, whose elements assign prior data that are given in any metric space, to observed image measurements. The corresponding Riemannian gradient flow entails a set of replicator equations, one for each data point, that are spatially coupled by geometric averaging on the manifold. Starting from uniform assignments at the barycenter as natural initialization, the flow terminates at some global maximum, each of which corresponds to an image labeling that uniquely assigns the prior data. Our geometric variational approach constitutes a smooth non-convex inner approximation of the general image labeling problem, implemented with sparse interior-point numerics in terms of parallel multiplicative updates that converge efficiently.
This is a preview of subscription content, log in via an institution to check access.
Access this article
Subscribe and save.
- Get 10 units per month
- Download Article/Chapter or eBook
- 1 Unit = 1 Article or 1 Chapter
- Cancel anytime
Price includes VAT (Russian Federation)
Instant access to the full article PDF.
Rent this article via DeepDyve
Institutional subscriptions
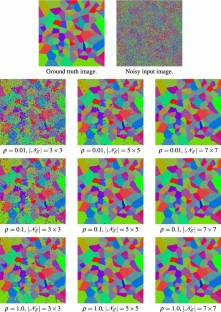
Similar content being viewed by others
Geometric Image Labeling with Global Convex Labeling Constraints
A Variational Perspective on the Assignment Flow
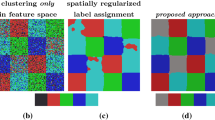
Unsupervised Assignment Flow: Label Learning on Feature Manifolds by Spatially Regularized Geometric Assignment
For locations i close to the boundary of the image domain where patch supports \({\mathscr {N}}_{p}(i)\) shrink, the definition of the vector \(w^{p}\) has to be adapted accordingly.
Amari, S.I., Nagaoka, H.: Methods of Information Geometry. American Mathematical Society, Oxford University Press, Oxford (2000)
MATH Google Scholar
Aujol, J.F., Gilboa, G., Chan, T., Osher, S.: Structure-texture image decomposition-modeling, algorithms, and parameter selection. Int. J. Comput. Vis. 67 (1), 111–136 (2006)
Article MATH Google Scholar
Ball, K.: An elementary introduction to modern convex geometry. In: Levy, S. (ed.) Flavors of Geometry, MSRI Publ., vol. 31, pp. 1–58. Cambridge University Press (1997)
Bayer, D., Lagarias, J.: The nonlinear geometry of linear programming. I. Affine and projective scaling trajectories. Trans. Am. Math. Soc. 314 (2), 499–526 (1989)
MathSciNet MATH Google Scholar
Bayer, D., Lagarias, J.: The nonlinear geometry of linear programming. II. Legendre transform coordinates and central trajectories. Trans. Am. Math. Soc. 314 (2), 527–581 (1989)
Bishop, C.: Pattern Recognition and Machine Learning. Springer, Berlin (2006)
Bomze, I., Budinich, M., Pelillo, M., Rossi, C.: Annealed replication: a new heuristic for the maximum clique problem. Discr. Appl. Math. 121 , 27–49 (2002)
Article MathSciNet MATH Google Scholar
Bomze, I.M.: Regularity versus degeneracy in dynamics, games, and optimization: a unified approach to different aspects. SIAM Rev. 44 (3), 394–414 (2002)
Buades, A., Coll, B., Morel, J.: A review of image denoising algorithms, with a new one. SIAM Multiscale Model. Simul. 4 (2), 490–530 (2005)
Buades, A., Coll, B., Morel, J.M.: Neighborhood filters and PDEs. Numer. Math. 105 , 1–34 (2006)
Cabrales, A., Sobel, J.: On the limit points of discrete selection dynamics. J. Econ. Theory 57 , 407–419 (1992)
Čencov, N.: Statistical Decision Rules and Optimal Inference. American Mathematical Society, Providence (1982)
Google Scholar
Chambolle, A., Cremers, D., Pock, T.: A convex approach to minimal partitions. SIAM J. Imaging Sci. 5 (4), 1113–1158 (2012)
Chan, T., Esedoglu, S., Nikolova, M.: Algorithms for Finding Global Minimizers of Image Segmentation and Denoising Models. SIAM J. Appl. Math. 66 (5), 1632–1648 (2006)
Hérault, L., Horaud, R.: Figure-ground discrimination: a combinatorial optimization approach. IEEE Trans. Pattern Anal. Mach. Intell. 15 (9), 899–914 (1993)
Article Google Scholar
Heskes, T.: Convexity arguments for efficient minimization of the Bethe and Kikuchi free energies. J. Artif. Intell. Res. 26 , 153–190 (2006)
Hofbauer, J., Siegmund, K.: Evolutionary game dynamics. Bull. Am. Math. Soc. 40 (4), 479–519 (2003)
Hofman, T., Buhmann, J.: Pairwise data clustering by deterministic annealing. IEEE Trans. Pattern Anal. Mach. Intell. 19 (1), 1–14 (1997)
Horst, R., Tuy, H.: Global Optimization: Deterministic Approaches, 3rd edn. Springer, Berlin (1996)
Book MATH Google Scholar
Hummel, R., Zucker, S.: On the foundations of the relaxation labeling processes. IEEE Trans. Pattern Anal. Mach. Intell. 5 (3), 267–287 (1983)
Jost, J.: Riemannian Geometry and Geometric Analysis, 4th edn. Springer, Berlin (2005)
Kappes, J., Andres, B., Hamprecht, F., Schnörr, C., Nowozin, S., Batra, D., Kim, S., Kausler, B., Kröger, T., Lellmann, J., Komodakis, N., Savchynskyy, B., Rother, C.: A comparative study of modern inference techniques for structured discrete energy minimization problems. Int. J. Comput. Vis. 115 (2), 155–184 (2015)
Article MathSciNet Google Scholar
Kappes, J., Savchynskyy, B., Schnörr, C.: A bundle approach to efficient MAP-inference by Lagrangian relaxation. In: Proc. CVPR (2012)
Kappes, J., Schnörr, C.: MAP-inference for highly-connected graphs with DC-programming. In: Pattern Recognition—30th DAGM Symposium, LNCS, vol. 5096, pp. 1–10. Springer (2008)
Karcher, H.: Riemannian center of mass and mollifier smoothing. Commun. Pure Appl. Math. 30 , 509–541 (1977)
Karcher, H.: Riemannian center of mass and so called karcher mean. arxiv:1407.2087 (2014)
Kass, R.: The geometry of asymptotic inference. Stat. Sci. 4 (3), 188–234 (1989)
Kolmogorov, V.: Convergent tree-reweighted message passing for energy minimization. IEEE Trans. Pattern Anal. Mach. Intell. 28 (10), 1568–1583 (2006)
Kolmogorov, V., Zabih, R.: What energy functions can be minimized via graph cuts? IEEE Trans. Pattern Anal. Mach. Intell. 26 (2), 147–159 (2004)
Ledoux, M.: The Concentration of Measure Phenomenon. American Mathematical Society, Providence (2001)
Lellmann, J., Lenzen, F., Schnörr, C.: Optimality bounds for a variational relaxation of the image partitioning problem. J. Math. Imaging Vis. 47 (3), 239–257 (2013)
Lellmann, J., Schnörr, C.: Continuous multiclass labeling approaches and algorithms. SIAM J. Imaging Sci. 4 (4), 1049–1096 (2011)
Losert, V., Alin, E.: Dynamics of games and genes: discrete versus continuous time. J. Math. Biol. 17 (2), 241–251 (1983)
Luce, R.: Individual Choice Behavior: A Theoretical Analysis. Wiley, New York (1959)
Milanfar, P.: A tour of modern image filtering. IEEE Signal Process. Mag. 30 (1), 106–128 (2013)
Milanfar, P.: Symmetrizing smoothing filters. SIAM J. Imaging Sci. 6 (1), 263–284 (2013)
Montúfar, G., Rauh, J., Ay, N.: On the fisher metric of conditional probability polytopes. Entropy 16 (6), 3207–3233 (2014)
Nesterov, Y., Todd, M.: On the riemannian geometry defined by self-concordant barriers and interior-point methods. Found. Comput. Math. 2 , 333–361 (2002)
Orland, H.: Mean-field theory for optimization problems. J. Phys. Lett. 46 (17), 763–770 (1985)
Pavan, M., Pelillo, M.: Dominant sets and pairwise clustering. IEEE Trans. Pattern Anal. Mach. Intell. 29 (1), 167–172 (2007)
Pelillo, M.: The dynamics of nonlinear relaxation labeling processes. J. Math. Imaging Vis. 7 , 309–323 (1997)
Pelillo, M.: Replicator equations, maximal cliques, and graph isomorphism. Neural Comput. 11 (8), 1933–1955 (1999)
Rosenfeld, A., Hummel, R., Zucker, S.: Scene labeling by relaxation operations. IEEE Trans. Syst. Man Cybern. 6 , 420–433 (1976)
Singer, A., Shkolnisky, Y., Nadler, B.: Diffusion interpretation of non-local neighborhood filters for signal denoising. SIAM J. Imaging Sci. 2 (1), 118–139 (2009)
Sutton, R., Barto, A.: Reinforcement Learning, 2nd edn. MIT Press, Cambridge (1999)
Swoboda, P., Shekhovtsov, A., Kappes, J., Schnörr, C., Savchynskyy, B.: Partial optimality by pruning for MAP-inference with general graphical models. IEEE Trans. Patt. Anal. Mach. Intell. 38 (7), 1370–1382 (2016)
Wainwright, M., Jordan, M.: Graphical models, exponential families, and variational inference. Found. Trends Mach. Learn. 1 (1–2), 1–305 (2008)
Weickert, J.: Anisotropic Diffusion in Image Processing. B.G Teubner, Leipzig (1998)
Werner, T.: A linear programming approach to max-sum problem: a review. IEEE Trans. Pattern Anal. Mach. Intell. 29 (7), 1165–1179 (2007)
Yedidia, J., Freeman, W., Weiss, Y.: Constructing free-energy approximations and generalized belief propagation algorithms. IEEE Trans. Inf. Theory 51 (7), 2282–2312 (2005)
Download references
Acknowledgements
Support by the German Research Foundation (DFG) was gratefully acknowledged, Grant GRK 1653.

Author information
Authors and affiliations.
Heidelberg Collaboratory for Image Processing, Heidelberg University, Heidelberg, Germany
Freddie Åström
Mathematical Imaging Group, Heidelberg University, Heidelberg, Germany
Stefania Petra
CEREMADE, University Paris-Dauphine, Paris, France
Bernhard Schmitzer
Image and Pattern Analysis Group, Heidelberg University, Heidelberg, Germany
Christoph Schnörr
You can also search for this author in PubMed Google Scholar
Corresponding author
Correspondence to Christoph Schnörr .
Appendix 1: Basic Notation
For \(n \in {\mathbb {N}}\) , we set \([n] = \{1,2,\ldots ,n\}\) . \({\mathbbm {1}}= (1,1,\ldots ,1)^{\top }\) denotes the vector with all components equal to 1, whose dimension can either be inferred from the context or is indicated by a subscript, e.g., \({\mathbbm {1}}_{n}\) . Vectors \(v^{1}, v^{2},\ldots \) are indexed by lowercase letters and superscripts, whereas subscripts \(v_{i},\, i \in [n]\) , index vector components. \(e^{1},\ldots ,e^{n}\) denotes the canonical orthonormal basis of \(\mathbb {R}^{n}\) .
We assume data to be indexed by a graph \({\mathscr {G}}=({\mathscr {V}},{\mathscr {E}})\) with nodes \(i \in {\mathscr {V}}=[m]\) and associated locations \(x^{i} \in \mathbb {R}^{d}\) , and with edges \({\mathscr {E}}\) . A regular grid graph and \(d=2\) is the canonical example. But \({\mathscr {G}}\) may also be irregular due to some preprocessing like forming super-pixels, for instance, or correspond to 3D images or videos ( \(d=3\) ). For simplicity, we call i location although this actually is \(x^{i}\) .
If \(A \in \mathbb {R}^{m \times n}\) , then the row and column vectors are denoted by \(A_{i} \in \mathbb {R}^{n},\, i \in [m]\) and \(A^{j} \in \mathbb {R}^{m},\, j \in [n]\) , respectively, and the entries by \(A_{ij}\) . This notation of row vectors \(A_{i}\) is the only exception from our rule of indexing vectors stated above.
The componentwise application of functions \(f :\mathbb {R}\rightarrow \mathbb {R}\) to a vector is simply denoted by f ( v ), e.g.,
Likewise, binary relations between vectors apply componentwise, e.g., \(u \ge v \;\Leftrightarrow \; u_{i} \ge v_{i},\; i \in [n]\) , and binary componentwise operations are simply written in terms of the vectors. For example,
where the latter operation is only applied to strictly positive vectors \(q > 0\) . The support \({{\mathrm{supp}}}(p) = \{p_{i} \ne 0 :i \in {{\mathrm{supp}}}(p)\} \subset [n]\) of a vector \(p \in \mathbb {R}^{n}\) is the index set of all non-nonvanishing components of p .
\(\langle x, y \rangle \) denotes the standard Euclidean inner product and \(\Vert x\Vert = \langle x, x \rangle ^{1/2}\) the corresponding norm. Other \(\ell _{p}\) -norms, \(1 \le p \ne 2 \le \infty \) , are indicated by a corresponding subscript, \( \Vert x\Vert _{p} = \big (\sum _{i \in [d]} |x_{i}|^{p}\big )^{1/p}, \) except for the case \(\Vert x\Vert = \Vert x\Vert _{2}\) . For matrices \(A, B \in \mathbb {R}^{m \times n}\) , the canonical inner product is \( \langle A, B \rangle = \hbox {tr}(A^{\top } B) \) with the corresponding Frobenius norm \(\Vert A\Vert = \langle A, A \rangle ^{1/2}\) . \({{\mathrm{Diag}}}(v) \in \mathbb {R}^{n \times n},\, v \in \mathbb {R}^{n}\) , is the diagonal matrix with the vector v on its diagonal.
Other basic sets and their notation are
the positive orthant
the set of strictly positive vectors
the ball of radius r centered at p
the unit sphere
the probability simplex
and its relative interior
closure (not regarded as manifold)
the sphere with radius 2
the assignment manifold
and its closure (not regarded as manifold)
For a discrete distribution \(p \in \varDelta _{n-1}\) and a finite set \(S=\{s^{1},\ldots ,s^{n}\}\) vectors, we denote by
the mean of S with respect to p .
Let \({\mathscr {M}}\) be a any differentiable manifold. Then \(T_{p}{\mathscr {M}}\) denotes the tangent space at base point \(p \in {\mathscr {M}}\) and \(T{\mathscr {M}}\) the total space of the tangent bundle of \({\mathscr {M}}\) . If \(F :{\mathscr {M}} \rightarrow {\mathscr {N}}\) is a smooth mapping between differentiable manifold \({\mathscr {M}}\) and \({\mathscr {N}}\) , then the differential of F at \(p \in {\mathscr {M}}\) is denoted by
If \(F :\mathbb {R}^{m} \rightarrow \mathbb {R}^{n}\) , then \(DF(p) \in \mathbb {R}^{n \times m}\) is the Jacobian matrix at p , and the application DF ( p )[ v ] to a vector \(v \in \mathbb {R}^{m}\) means matrix-vector multiplication. We then also write DF ( p ) v . If \(F = F(p,q)\) , then \(D_{p}F(p,q)\) and \(D_{q}F(p,q)\) are the Jacobians of the functions \(F(\cdot ,q)\) and \(F(p,\cdot )\) , respectively.
The gradient of a differentiable function \(f :\mathbb {R}^{n} \rightarrow \mathbb {R}\) is denoted by \(\nabla f(x) = \big (\partial _{1} f(x),\ldots ,\partial _{n} f(x)\big )^{\top }\) , whereas the Riemannian gradient of a function \(f :{\mathscr {M}} \rightarrow \mathbb {R}\) defined on Riemannian manifold \({\mathscr {M}}\) is denoted by \(\nabla _{{\mathscr {M}}} f\) . Eq. ( 2.5 ) recalls the formal definition.
The exponential mapping [ 21 , Def. 1.4.3]
maps the tangent vector v to the point \(\gamma _{v}(1) \in {\mathscr {M}}\) , uniquely defined by the geodesic curve \(\gamma _{v}(t)\) emanating at p in direction v . \(\gamma _{v}(t)\) is the shortest path on \({\mathscr {M}}\) between the points \(p, q \in {\mathscr {M}}\) that \(\gamma _{v}\) connects. This minimal length equals the Riemannian distance \(d_{{\mathscr {M}}}(p,q)\) induced by the Riemannian metric , denoted by
i.e., the inner product on the tangent spaces \(T_{p}{\mathscr {M}},\,p \in {\mathscr {M}}\) , that smoothly varies with p . Existence and uniqueness of geodesics will not be an issue for the manifolds \({\mathscr {M}}\) considered in this paper.
The exponential mapping \({{\mathrm{Exp}}}_{p}\) should not be confused with
the exponential function \(e^{v}\) used, e.g., in ( 6.1 );
the mapping \(\exp _{p} :T_{p}{\mathscr {S}} \rightarrow {\mathscr {S}}\) defined by Eq. ( 3.8a ).
The abbreviations “l.h.s.” and “r.h.s.” mean left-hand side and right-hand side of some equation, respectively. We abbreviate with respect to by “wrt.”
Appendix 2: Proofs and Further Details
1.1 proofs of section 2.
(of Lemma 1 ) Let \(p \in {\mathscr {S}}\) and \(v \in T_{p}{\mathscr {S}}\) . We have
and \(\big \langle \psi (p), D\psi (p)[v] \big \rangle = \langle 2 \sqrt{p}, \frac{v}{\sqrt{p}} \rangle = 2 \langle {\mathbbm {1}}, v \rangle = 0\) , that is, \(D\psi (p)[v] \in T_{\psi (p)}{\mathscr {N}}\) . Furthermore,
i.e., the Riemannian metric is preserved and hence also the length L ( s ) of curves \(s(t) \in {\mathscr {N}},\, t \in [a,b]\) : Put \(\gamma (t) = \psi ^{-1}\big (s(t)\big ) = \frac{1}{4} s^{2}(t) \in {\mathscr {S}},\, t \in [a,b]\) . Then \(\dot{\gamma }(t)=\frac{1}{2} s(t) \dot{s}(t) = \frac{1}{2} \psi \big (\gamma (t)\big ) \dot{s}(t) = \sqrt{\gamma (t)} \dot{s}(t)\) and
\(\square \)
(of Prop. 1 ) Setting \(g:{\mathscr {N}} \rightarrow \mathbb {R}\) , \(q \mapsto g(s) := f\big (\psi ^{-1}(s)\big )\) with \(s = \psi (p) = 2 \sqrt{p}\) from ( 2.3 ), we have
because the 2-sphere \({\mathscr {N}}=2{\mathbb {S}}^{n-1}\) is an embedded submanifold, and hence the Riemannian gradient equals the orthogonal projection of the Euclidean gradient onto the tangent space. Pulling back the vector field \(\nabla _{{\mathscr {N}}} g\) by \(\psi \) using
we get with ( 7.1 ), ( 7.4 ) and \(\Vert s\Vert =2\) and hence \(s/\Vert s\Vert = \frac{1}{2} \psi (p)=\sqrt{p}\)
which equals ( 2.6 ). We finally check that \(\nabla f_{{\mathscr {S}}}(p)\) satisfies ( 2.5 ) (with \({\mathscr {S}}\) in place of \({\mathscr {M}}\) ). Using ( 2.1 ), we have
(of Prop. 2 ) The geodesic on the 2-sphere emanating at \(s(0) \in {\mathscr {N}}\) in direction \(w=\dot{s}(0) \in T_{s(0)}{\mathscr {N}}\) is given by
Setting \(s(0)=\psi (p)\) and \(w = D\psi (p)[v]=v/\sqrt{p}\) , the geodesic emanating at \(p=\gamma _{v}(0)\) in direction v is given by \(\psi ^{-1}\big (s(t)\big )\) due to Lemma 1 , which results in ( 2.7a ) after elementary computations. \(\square \)
1.2 Proofs of Section 3 and Further Details
(of Prop. 3 ) We have \(p = \exp _{p}(0)\) and
which confirms ( 3.10 ), is equal to ( 3.9 ) at \(t=0\) and hence yields the first expression of ( 3.11 ). The second expression of ( 3.11 ) follows from a Taylor expansion of ( 2.7a )
(of Lemma 4 ) By construction, \(S(W) \in {\mathscr {W}}\) , that is, \(S_{i}(W) \in {\mathscr {S}},\; i \in [m]\) . Consequently,
The upper bound corresponds to matrices \(\overline{W}^{*} \in \overline{{\mathscr {W}}}\) and \(S(\overline{W}^{*})\) where for each \(i \in [m]\) , both \(\overline{W}^{*}_{i}\) and \(S_{i}(\overline{W}^{*})\) equal the same unit vector \(e^{k_{i}}\) for some \(k_{i} \in [m]\) . \(\square \)
(Explicit form of ( 3.27 )) The matrices \(T^{ij}(W) = \frac{\partial }{\partial W_{ij}} S(W)\) are implicitly given through the optimality condition ( 2.9 ) that each vector \(S_{k}(W),\, k \in [m]\) , defined by ( 3.13 ) has to satisfy
while temporarily dropping below W as argument to simplify the notation, and using the indicator function \(\delta _{{\mathrm {P}}} = 1\) if the predicate \({\mathrm {P}}={\mathrm {true}}\) and \(\delta _{{\mathrm {P}}} = 0\) otherwise, we differentiate the optimality condition on the r.h.s. of (7.12),
Since the vectors \(\phi (S_{k},L_{r})\) given by ( 7.13 ) are the negative Riemannian gradients of the (locally) strictly convex objectives ( 2.8 ) defining the means \(S_{k}\) [ 21 , Thm. 4.6.1], the regularity of the matrices \(H^{k}(W)\) follows. Thus, using ( 7.14f ) and defining the matrices
results in ( 3.27 ). The explicit form of this expression results from computing and inserting into ( 7.14f ) the corresponding Jacobians \(D_{p}\phi (p,q)\) and \(D_{q}\phi (p,q)\) of
The term ( 7.16b ) results from mapping back the corresponding vector from the 2-sphere \({\mathscr {N}}\) ,
where \(\psi \) is the sphere map ( 2.3 ) and \(d_{{\mathscr {N}}}\) is the geodesic distance on \({\mathscr {N}}\) . The term ( 7.16c ) results from directly evaluating ( 3.12 ). \(\square \)
(of Lemma 5 ) We first compute \(\exp _{p}^{-1}\) . Suppose
Thus, in view of ( 3.9 ), we approximate
Applying this to the point set \({\mathscr {P}}\) , i.e., setting
step (3) of ( 3.31 ) yields
Finally, approximating step (4) of ( 3.31 ) results in view of Prop. 3 in the update of p
Rights and permissions
Reprints and permissions
About this article
Åström, F., Petra, S., Schmitzer, B. et al. Image Labeling by Assignment. J Math Imaging Vis 58 , 211–238 (2017). https://doi.org/10.1007/s10851-016-0702-4
Download citation
Received : 25 March 2016
Accepted : 30 December 2016
Published : 12 January 2017
Issue Date : June 2017
DOI : https://doi.org/10.1007/s10851-016-0702-4
Share this article
Anyone you share the following link with will be able to read this content:
Sorry, a shareable link is not currently available for this article.
Provided by the Springer Nature SharedIt content-sharing initiative
- Image labeling
- Assignment manifold
- Fisher–Rao metric
- Riemannian gradient flow
- Replicator equations
- Information geometry
- Neighborhood filters
- Nonlinear diffusion
Mathematics Subject Classification
- Find a journal
- Publish with us
- Track your research
NOTIFICATIONS
Labelling the eye.
- + Create new collection
The human eye contains structures that allow it to perceive light, movement and colour differences.
In this activity, students use online or paper resources to identity and label the main parts of the human eye.
By the end of this activity, students should be able to:
- identify the main parts of the human eye
- describe the functions of the different parts of the human eye.
Download the Word file (see link below).
Related content
Find out more about our light and sight resources here .
Examine the components and functions of the eye with this dissection activity .
This article lists key terms and definitions about light and sight.
Build your knowledge and understanding of the components and function of the eye with this article .
Interested about a programme that uses peer-to-peer vision testing in classrooms? Have a look at this teacher case study .
Useful links
View a BBC animation showing how human sight occurs .
Information on a cow’s eye dissection , eye lenses and eye structure.
See our newsletters here .
Would you like to take a short survey?
This survey will open in a new tab and you can fill it out after your visit to the site.
Pardon Our Interruption
As you were browsing something about your browser made us think you were a bot. There are a few reasons this might happen:
- You've disabled JavaScript in your web browser.
- You're a power user moving through this website with super-human speed.
- You've disabled cookies in your web browser.
- A third-party browser plugin, such as Ghostery or NoScript, is preventing JavaScript from running. Additional information is available in this support article .
To regain access, please make sure that cookies and JavaScript are enabled before reloading the page.

IMAGES
VIDEO
COMMENTS
Term. Vitreous humor. Location. Sign up and see the remaining cards. It's free! Continue with Google. Start studying Med Term Chapter 11 Labeling Eye. Learn vocabulary, terms, and more with flashcards, games, and other study tools.
Anatomy and Physiology. Anatomy and Physiology questions and answers. 11.1 Image Labeling Drag and Drop 1 Choroid 2 Optic nerve 3 Aqueous fluid 4 Lens 5 Retina 6 Sclera Fovea centralis 8 Macula 9 Cornea (A) Anterior Segment (B) Posterior Segment 10 Iris 11 Vitreous fluid 12 Optic disk edback.
Terms in this set (85) Study with Quizlet and memorize flashcards containing terms like , , and more.
proximal convoluted tube. (4) descending nephron loop. distal convoluted tubule. collection tubule. ascending nephron loop. peritubular capillaries. Study with Quizlet and memorize flashcards containing terms like Kidney, urinary bladder, female urethra and more.
pre-class assignment 11.1 ch.11 nervous system (pp. 268 288) label the images below. when possible, include the combining form or root of the word used. corpus. Skip to document. University; ... PCA 8 (Urinary System) - pre-class assignment 8; PCA 7 (Digestive System) Related documents. PCA 6 (Blood, Lymph, Immune Systems) PCA 5.1 ...
9.1 image Labeling; Your solution's ready to go! Our expert help has broken down your problem into an easy-to-learn solution you can count on. See Answer See Answer See Answer done loading. Question: 9.1 image Labeling. need help lableing . Show transcribed image text. There's just one step to solve this. Solution. Step 1.
Label the Eye. This worksheet shows an image of the eye with structures numbered. Students practice labeling the eye or teachers can print this to use as an assessment. There are two versions on the google doc and pdf file, one where the word bank is included and another with no word bank for differentiation.
Figure 11.1 Label Art Activity - g-wlearning.com ... prev ...
85. The all-or-none phenomenon as applied to nerve conduction states that the whole nerve cell must be stimulated for conduction to take place. False. Study A&P Chapter 11 Fundamentals of the Nervous System and Nervous Tissue flashcards taken from chapter 11 of the book Human Anatomy & Physiology.
1.1 Motivation. at some global maximum, each of which corresponds to an image labeling that uniquely assigns the prior data. Our geo- Image Labeling is a basic problem of variational low-level metric variational approach constitutes a smooth non-convex image analysis.
University: Clemson University. Course: 16Documents. Students shared 16 documents in this course. Info More info. filled in pages instructor resource material for 11 respiratory system handout labeling label the images with their anatomic terms and combining forms where.
delicate membrane lining the inside of the eyelids and covering the eyeball. Location. Term. cornea. Definition. fibrous transparent layer of clear tissue like a dome that covers the anterior portion of the eyeball (the iris and pupil). It is the first structure to refract (bend) light that enters the eye. Location.
1.1 Motivation. Image Labeling is a basic problem of variational low-level image analysis. It amounts to determining a partition of the image domain by uniquely assigning to each pixel a single element from a finite set of labels. Most applications require such decisions to be made depending on other decisions. This gives rise to a global objective function whose minima correspond to favorable ...
Labelling the eye. Use this interactive to label different parts of the human eye. Drag and drop the text labels onto the boxes next to the diagram. Selecting or hovering over a box will highlight each area in the diagram. The human eye has several structures that enable entering light energy to be converted to electrochemical energy.
🌟 Left to win $100!Don't miss out, enter now! 🌟 This giveaway is our way of saying thanks for your invaluable contribution to the growth of ihatecbts.com.
Resource. The human eye contains structures that allow it to perceive light, movement and colour differences. In this activity, students use online or paper resources to identity and label the main parts of the human eye. By the end of this activity, students should be able to: describe the functions of the different parts of the human eye.
labeling the body. 4.5 (2 reviews) myel/o-. Click the card to flip 👆. spinal cord. Click the card to flip 👆. 1 / 6.
aortic valve. aorta. arterioles. arteries transfer blood to _. veins. _ are blood vessels that return blood to the heart. red blood cell. erythrocyte is another term for _. Study with Quizlet and memorize flashcards containing terms like , , and more.
Each assignment will be graded Materials for Instruction Medical Terminology for Health Professionals, 8th Edition by Ehrlich and ... 2 Building Terms 1.3 Vocabulary Instructions 1.4 Spelling Terms 1.1 Image Labeling Introductions 1.5 Spelling Terms Introduction to 1.6 Vocabulary 1.8 Building Terms Medical 1.9 Vocabulary Terminology 1.10 ...