

Rethink Biology Notes
Ace the biology exams with expert notes
FRAP (Fluorescence Recovery After Photobleaching)
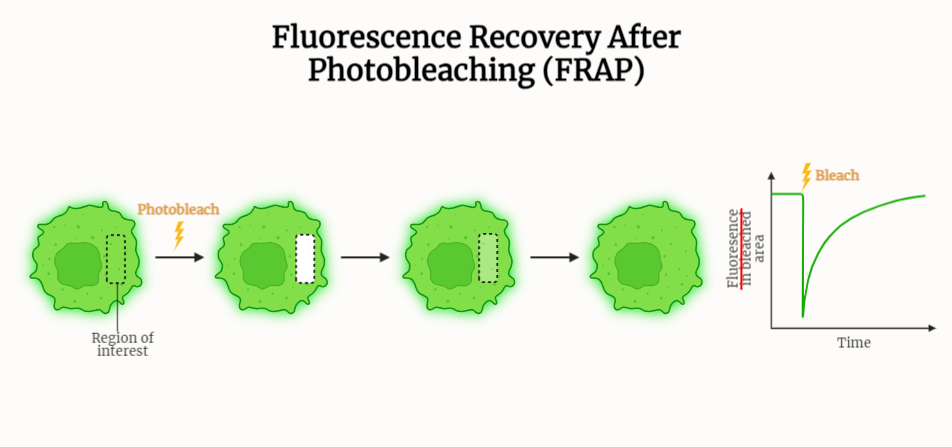
Table of Contents
Introduction to FRAP:
FRAP or Fluorescence Recovery After Photobleaching is a powerful technique that is widely used to study the dynamics of biomolecules within cells and other biological systems. The technique is based on the selective bleaching of a small region of a sample using a high-intensity laser, followed by the monitoring of fluorescence recovery in the bleached region.
Principles of FRAP:
- The main principle behind FRAP is that when a small region of a sample is selectively bleached, the fluorescence in that region will decrease.
- If the molecules in the bleached region are mobile, they will diffuse back into the bleached region, thereby restoring the fluorescence.
- The rate at which fluorescence is restored can be used to determine the mobility of the molecules in the bleached region.
- The first step in a FRAP experiment is to select a small region of the sample to be bleached. This can be done using a laser to selectively bleach a small region of the sample.
- Next, the fluorescence recovery in the bleached region is monitored over time.
- The data collected from the experiment is analyzed to determine the mobility of the molecules in the bleached region.
Advantages:
- One of the major advantages of FRAP is that it can be used to study the mobility of molecules in living cells, which allows researchers to study biological processes in their natural state.
- Additionally, the technique is relatively simple to perform and can be used to study a wide range of biomolecules.
Limitations:
- One of the major limitations of FRAP is that it is limited to studying the mobility of molecules in a small region of the sample.
- Additionally, the technique is not suitable for studying the mobility of very large structures, such as whole organelles.
Applications:
- Studying protein dynamics: FRAP can be used to study the movement and diffusion of proteins within cells, such as the diffusion of receptors on the cell surface.
- Intracellular organelles dynamics: This technique can be used to study the dynamics of intracellular organelles, such as the movement of mitochondria within a cell.
- Cytoskeleton organization: It can be used to study the organization and dynamics of the cytoskeleton, including the movement of microtubules and actin filaments.
- Membrane dynamics: FRAP can be used to study the dynamics of cell membranes, including the movement of lipids and the organization of membrane proteins.
- Intracellular transport: FRAP can be used to study the movement of molecules within cells, including the transport of molecules from the cell surface to the interior of the cell.
- Study of cellular interactions: FRAP can be used to study the interactions between cells, including the movement of molecules between cells.
- Study of biomaterials: FRAP can be used to study the dynamics of biomaterials, including the movement of molecules within hydrogels and other biomaterials.
Conclusion:
FRAP is a powerful technique that is widely used to study the dynamics of biomolecules within cells and other biological systems. The technique is based on the selective bleaching of a small region of a sample using a high-intensity laser, followed by the monitoring of fluorescence recovery in the bleached region.
FRAP has a wide range of applications and can be used to study a wide range of biological processes, including protein and membrane dynamics, cytoskeleton organization, and intracellular transport.

Check out our Latest Posts
- Mammalian Fertilization: Capacitation
- Isolation and Purification of RNA
- Skeletal muscle organization
- Objective Lenses of Microscopes
- Membrane Potential
Loading metrics
Open Access
Peer-reviewed
Research Article
Using Fluorescence Recovery After Photobleaching data to uncover filament dynamics
Roles Conceptualization, Data curation, Formal analysis, Investigation, Methodology, Project administration, Resources, Software, Supervision, Validation, Visualization, Writing – original draft, Writing – review & editing
* E-mail: [email protected]
Affiliation Department of Mathematics, Brigham Young University, Provo, Utah, United States of America

Roles Conceptualization, Data curation, Formal analysis, Investigation, Methodology, Validation, Visualization, Writing – original draft, Writing – review & editing
Affiliations Institut Pasteur, Université de Paris, UMR3691 CNRS, Cell Polarity, Migration and Cancer Unit, Université de Paris, Equipe Labellisée Ligue Contre le Cancer, Paris, France, Université Paris Cité, CNRS, Institut Jacques Monod, Paris, France
Roles Conceptualization, Formal analysis, Investigation, Methodology, Visualization, Writing – original draft, Writing – review & editing
Roles Conceptualization, Funding acquisition, Methodology, Project administration, Resources, Supervision, Visualization, Writing – original draft, Writing – review & editing
Affiliation Institut Pasteur, Université de Paris, UMR3691 CNRS, Cell Polarity, Migration and Cancer Unit, Université de Paris, Equipe Labellisée Ligue Contre le Cancer, Paris, France
Roles Conceptualization, Funding acquisition, Methodology, Project administration, Supervision, Visualization, Writing – original draft, Writing – review & editing
Affiliation Department of Mathematics, University of Manitoba, Winnipeg, Manitoba, Canada
- J. C. Dallon,
- Cécile Leduc,
- Christopher P. Grant,
- Emily J. Evans,
- Sandrine Etienne-Manneville,
- Stéphanie Portet
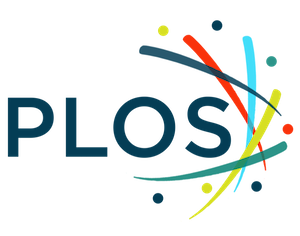
- Published: September 26, 2022
- https://doi.org/10.1371/journal.pcbi.1010573
- Reader Comments
Fluorescence Recovery After Photobleaching (FRAP) has been extensively used to understand molecular dynamics in cells. This technique when applied to soluble, globular molecules driven by diffusion is easily interpreted and well understood. However, the classical methods of analysis cannot be applied to anisotropic structures subjected to directed transport, such as cytoskeletal filaments or elongated organelles transported along microtubule tracks. A new mathematical approach is needed to analyze FRAP data in this context and determine what information can be obtain from such experiments. To address these questions, we analyze fluorescence intensity profile curves after photobleaching of fluorescently labelled intermediate filaments anterogradely transported along microtubules. We apply the analysis to intermediate filament data to determine information about the filament motion. Our analysis consists of deriving equations for fluorescence intensity profiles and developing a mathematical model for the motion of filaments and simulating the model. Two closed forms for profile curves were derived, one for filaments of constant length and one for filaments with constant velocity, and three types of simulation were carried out. In the first type of simulation, the filaments have random velocities which are constant for the duration of the simulation. In the second type, filaments have random velocities which instantaneously change at random times. In the third type, filaments have random velocities and exhibit pausing between velocity changes. Our analysis shows: the most important distribution governing the shape of the intensity profile curves obtained from filaments is the distribution of the filament velocity. Furthermore, filament length which is constant during the experiment, had little impact on intensity profile curves. Finally, gamma distributions for the filament velocity with pauses give the best fit to asymmetric fluorescence intensity profiles of intermediate filaments observed in FRAP experiments performed in polarized migrating astrocytes. Our analysis also shows that the majority of filaments are stationary. Overall, our data give new insight into the regulation of intermediate filament dynamics during cell migration.
Author summary
Fluorescence Recovery After Photobleaching (FRAP) is a commonly-used technique to analyze the dynamics of fluorescently-tagged proteins or structures in biology. After photochemical altering the fluorophor in a specific region, fluorescent material from the surrounding region moves into the photobleached region. Usually applied to the diffusion of soluble or membrane associate proteins, the existing models of analysis are not suitable for the elucidation of directional transport of elongated structures. Different modes of motions for the elongated structures with distributed lengths and velocities in cells are considered. First, we observe that filament lengths can be inferred from the level of noisiness. We further show the characteristics of fluorescence profile curves mainly depend on the occurrence of changes in velocities and distributions of velocities; whereas length distributions have negligible impact. Analysis of experimental data using this new framework indicates intermediate filaments transported by kinesins along microtubules in polarized migrating cells have gamma distributed velocities changing over time between pausing. Most filaments are found to be very slow or stationary with a few moving fast. This new computational approach should permit the interpretation of FRAP experimental data obtained with any directionally moving elongated structures of various lengths.
Citation: Dallon JC, Leduc C, Grant CP, Evans EJ, Etienne-Manneville S, Portet S (2022) Using Fluorescence Recovery After Photobleaching data to uncover filament dynamics. PLoS Comput Biol 18(9): e1010573. https://doi.org/10.1371/journal.pcbi.1010573
Editor: Melissa L. Kemp, Georgia Institute of Technology and Emory University, UNITED STATES
Received: February 25, 2022; Accepted: September 14, 2022; Published: September 26, 2022
Copyright: © 2022 Dallon et al. This is an open access article distributed under the terms of the Creative Commons Attribution License , which permits unrestricted use, distribution, and reproduction in any medium, provided the original author and source are credited.
Data Availability: All relevant data are within the manuscript and its Supporting information files.
Funding: SE and CL - This work was supported by the La Ligue contre le cancer (S-CR17017), Centre National de la Recherche Scientifique and Institut Pasteur and the agence nationale de la recherche (ANR 16-CE13-009, CL). SP is supported by a Discovery Grant of the Natural Sciences and Engineering Research Council of Canada (RGPIN-2018-04967) and a Burroughs Wellcome Fund 2020 Collaborative Research Travel Grant. The funders had no role in study design, data collection and analysis, decision to publish, or preparation of the manuscript.
Competing interests: The authors have declared that no competing interests exist.
1 Introduction
Living organisms are in constant dynamic equilibrium. In cells, many structures appear generally static but are, in fact, formed of molecules continuously moving and exchanging with the surrounding. Fluorescence Recovery After Photobleaching (FRAP), developed in the 1970s, is an essential tool for understanding molecular dynamics within a cell [ 1 – 3 ]. The typical setup for a FRAP experiment involves a fluorescent probe, a microscope, and some method of photobleaching [ 4 ]. A portion of the domain where the molecule of interest is present is bleached and the recovery of fluorescence in that region is imaged over time. In order to gain quantitative information on molecular dynamics, mathematical models of diffusion are typically used. These include models of diffusion in inhomogeneous media [ 5 ], models of diffusion and binding using reaction-diffusion equations [ 6 – 8 ], and advection-reaction-diffusion models of active transport and diffusion [ 9 ]. In some instances, when diffusion parameters are not of interest, simpler ordinary differential equation models are used to elicit information [ 10 ]. All these models deal with analysis of soluble, generally globular, molecules. Until now there has been no analysis of FRAP data regarding the dynamics of filamentous structures.
The main example we have in mind is that of short term transport of mature intermediate filaments (IFs), one of three major fibrous structural components of the cytoskeleton. They form a filamentous network spreading throughout the cell cytoplasm and this network together with actin filaments and microtubules, plays a key role in cell polarity and migration [ 11 ]. In migrating astrocytes (the type of glial cell used in our migration experiments), the dynamics of the IF network is mainly driven by microtubule and actin mediated transport [ 12 , 13 ]. Deterministic and stochastic mathematical models have been developed to describe the motion of IF driven by antagonistic molecular motors along microtubules [ 14 , 15 ]. In [ 12 ], FRAP experiments of IFs were used to better understand how the IF network global dynamics are regulated in migrating and non-migrating glial cells. They showed that, during cell polarization, IF transport is mainly anterograde, oriented from the cell center to the cell periphery, and this bias was due to the inhibition of the retrograde transport of IFs by CDC42-driven polarity signaling. However, due to the high density of the IF network, it was not possible to quantify the dynamics of IFs at the single filament level. Hence, there is a need for a mathematical model to better understand collective IF transport using FRAP data.
- PPT PowerPoint slide
- PNG larger image
- TIFF original image
https://doi.org/10.1371/journal.pcbi.1010573.g001
Our goal here is to determine what information can be obtained from the profile curves obtained from FRAP data. In particular, is it possible to infer the mode of filament motion (constant or variable velocity, with or without pausing), or information about filament velocity and length?
The time scale of the experiments is less than 30 minutes and the fluorescence only comes back from the edges of the bleached region. Hence we assume diffusion and remodelling of the filaments due to polymerization/depolymerization, subunit exchange (which occurs on a timescale of hours), fusion or severing are negligible [ 12 , 17 ]. Thus, the length of the filaments is assumed to be fixed during the observation time and the active transport of filaments moving from the cell center to the cell front is the major mechanism causing fluorescence recovery. In this model crowding effects or interactions with other filaments or organelles are not taken into consideration.
We will use mathematical modeling to determine what information can be obtained from FRAP data in the context of directional transport of elongated structures. In particular, we will focus on what characteristics of the velocity, length, and pause of filaments can be deduced from the experimental data. Due to the one directional transport, velocity and speed are synonymous in this work.
2.1 The mathematical model
We compare our results with experiments from polarized migrating astrocytes showing asymmetric fluorescence intensity profile curves with reduced retrograde transport [ 12 ]. Thus we consider only filaments which move to the right (having non-negative velocities). Following the observations that neurofilaments, a type of IFs observed in neurons, display a stop-and-go motion [ 13 , 18 ], we assume that the filaments can have a stop-and-go behavior, moving for a period of time T m at a velocity V and then stopping for a period of time T s . The cycle repeats, with the motion time, velocity, and stop time for each cycle being independent of those for the other cycles.
2.2 Simulations
There are five random variables with their associated distributions in the model. The initial setup of the filaments is determined by two random variables: the initial position of the right endpoint X 0 and the fiber length L . The movement of filaments is governed by V the filament velocity, T s the pausing time of the filament, and T m the time the filament is moving. We denote the distributions for all the random variables as follows:
- μ L governs the length L of each filament,
- μ V governs the velocity V of the filament during each period of motion,
- μ on governs the duration of time each filament moves before pausing or changing velocity, and
- μ off governs the duration of time each filament remains stationary (or pausing) before moving again.
We numerically simulated the FRAP experiments by moving the filaments and calculating how many filaments are in the bleached region. We did this in three different ways: 1) each filament gets a different velocity determined by μ V but it remains constant throughout the simulation; 2) after T on time units have elapsed, where T on is determined by μ on , velocities change but always come from the same distribution, μ V ; and 3) filaments have velocities which change, again determined by μ V , but they stop in between velocity changes. Thus, there is a cycle for each filament of duration T on + T off where T on is a μ on distributed random variable and is the time the filament is moving (the motion is on) and T off is a μ off distributed random variable and is the time the filament is stationary (the motion is off). We refer to these simulations as type 1, 2, and 3 simulations. Fig 2 depicts three filaments for each type of simulation. Depending on the simulation the length of the filaments is either fixed or uniformly, normally, or gamma distributed. Similarly, the velocity is either fixed or uniformly, normally, or gamma distributed. For the velocity the normal and gamma distributions are truncated so no velocities are greater than 40 microns per minute (and for the normal distribution the velocities are all positive). For type 2 simulations the lengths of time during which velocity is fixed are uniformly distributed with a specified mean τ on . For type 3 simulations the stop and run times are uniformly distributed with specified means τ off and τ on .
In each panel, kymographs of three typical filaments are shown. Panels (a)-(c) depict the three types of simulations where all the filaments have random velocities and random lengths. Panel (a) shows type 1 where the filaments have random velocities which are constant for the duration of the simulation. Panel (b) shows type 2 simulations where the filaments have random velocities which instantaneously change at random times. Finally, (c) shows type 3 simulations where filaments have random velocities and exhibit a pausing behavior between velocity changes. Panel (d) shows the particular situation/case of Type 1 simulations theoretically solved in Eq (4) where all filaments have the same fixed velocity but random (time-independent) lengths. Panel (e) the particular situation/case of type 1 simulations theoretically resolved in Eq (5) where all the filaments have the same length but different (time-independent) velocities that are randomly selected.
https://doi.org/10.1371/journal.pcbi.1010573.g002
We use MATLAB to perform the simulations. This work is driven by experimental data, however the variables of interest (velocity, length, pausing, and moving time) are not observable. Hence we model the variables of interest as random variables with underlying distributions. As previously motivated we use 4 types of distributions. We estimate only the parameters (mean and variance) of these distributions. Hence we fit the simulated fluorescence intensities to FRAP data by calibrating these distributions using the MATLAB function fmincon , a nonlinear optimizer which finds the minimum of a constrained nonlinear multivariable function. The result of our fitting specifies which type of distribution to use and its relevant free parameters for each variable of interest (velocity, pausing, and moving times). We found that information about length is not encoded in the profile curves, thus, to reduce the model complexity during the fitting process, we fixed the length to be a uniformly distributed random variable with μ = 5.025.
2.3 Experiments
2.3.1 cell culture..
Primary rat astrocytes were prepared as previously described [ 21 ] according to the guidelines approved by the French Ministry of Agriculture and following European standards. For scratch-induced migration assays, cells were seeded on poly-L-ornithine-precoated coverslips for immunofluorescence or 35-mm glass-bottomed culture dishes (MatTek Corporation) for videomicroscopy. Cells were grown to confluence in DMEM with 1 g/l glucose and supplemented with 10% FBS (Invitrogen), 1% penicillin–streptomycin (Thermo Fisher Scientific), and 1% amphotericin B (Thermo Fisher Scientific). On the day of the experiment, cells were scratched with a blunt-ended microinjection needle, creating a 300- μ m-wide wound to trigger cell migration.
2.3.2 Cell transfection.
Starting from a 10 cm diameter petri dish, primary astrocytes grown to confluence were trypsinized and electroporated with a Nucleofector machine (Lonza) using 5 μ g of vimentin-EGFP DNA. We have previously shown that EGFP-tagged vimentin co-polymerizes with the endogenous IF proteins and fluorescently labels the whole astrocytic IF network. Therefore labeling vimentin fluorescently is enough to follow the dynamics of the complete/whole IF network [ 12 ]. Medium was changed the day after transfection.
2.3.3 Live-cell imaging.
Nucleofected primary astrocytes were seeded on 35-mm glass-bottomed dishes and grown to confluence for 4 days. On the day before wounding, the medium was changed to a phenol red–free DMEM supplemented with 10% serum. The monolayer was wounded and cells were monitored between 1 and 2 hours after wounding, allowing them to grow a polarized protrusion [ 22 ]. Videos were acquired on a spinning-disk confocal microscope (PerkinElmer) equipped with an electron-multiplying charge-coupled device camera and either a 63×, 1.4 NA objective or a 100×, 1.4 NA objective.
In this section we give the results of the mathematical theory, the three types of simulations, and the experimental data. We divide it into five main subsections: first, we explain the theoretical results; second, we consider what can be learned from the initial setup; third, we compare type 1 simulations (where the velocity for each filament is fixed for the duration of the simulation but each filament’s velocity can be different) with the theoretical results derived in Eqs (4) and (5) ; fourth, we compare results from type 1 simulations (where each filament can have a different but fixed velocity) with type 2 simulations (where the velocity can abruptly change to a new value during the simulation) and with type 3 simulations (where the filament pauses before changing velocity); and finally, we compare the theory and results from simulations of type 1 and type 3 with experimental data.
3.1 Theoretical results
Based on the filament motion model assumed in this work and the description of the experimental setup described above, we are now deriving closed forms for the profile curves. Two simplifications allow the derivation of two equations for the profile curves valid under the corresponding assumptions. First, we assume that all the filaments have the same fixed velocity (a special case of type 1 simulations where all the filaments have a fixed velocity which is the same, i.e., a Dirac delta distribution which gives all the filaments the same velocity, see Fig 2d ). Thus the velocity is deterministic and no longer random. We then derive the corresponding profile curves in Eq (4) . Second, we used a random non-fixed velocity and we fix an identical filament length for all filaments, see Fig 2e . This allows us to derive Eq (5) .
Note that H depends on ( t , y ) through the traveling wave coordinate w .
Hence Eq (4) represents the density of fluorescent filaments at time t and location y , or the theoretical profile curves, when the velocity is fixed, and Eq (5) represents the density of the fluorescent filaments (or profile curves) when the length is fixed. The details of these calculations are found in S1 Appendix .
3.2 Initial setup
As will be shown in Section 3.3, the mathematical theory indicates that data from FRAP experiments reveals little information about filament length distributions, however some information can be obtained. By knowing how the density of the filaments changes in time and space some limited information about filament length can be deduced. In order to explain this we consider the initial setup for the system and distinguish between the initial distribution of the right endpoints of filaments and the distribution of filament densities. The first is independent of filament length ℓ and the second is not. For our mathematical setup we consider the filaments where the right endpoints are uniformly distributed in the interval [0, F ] (see Fig 1a ). The normalized density of filaments as a function of space depends on the length of filaments; two examples are shown in Fig 3 . The normalized density of filaments as a function of space will increase until it reaches 1 and then remain constant until some point before F where it will decrease to 0 at F and remain 0 from there on. The regions of increase and decrease are determined by the length distribution of the filaments. These types of regions may be found near the cell membrane. In panel (a) of Fig 3 the filament lengths have a larger mean (50, ±5 μ m (SD) from a Gaussian distribution) and thus have a gentle slope. In contrast, in panel (b) of Fig 3 the lengths have a smaller mean and standard deviation (0.5, ±0.05 μ m (SD) from a Gaussian distribution), resulting in a much sharper transition. In addition, if the filaments are long, the density measured from data will be smoother (less variability, with fewer filaments) but if the filaments are short, the measured density will be noisy (more variability which will require more filament measurements to smooth the density signal) as seen in panel (b).
This figure shows how filament length affects density measurements of the initial setup (before bleaching, all filaments are fluorescent). The mean length of filaments in (a) is 50 microns and in (b) 0.5 microns. One filament is highlighted in cyan in (a). The red lines show the density of filaments computed from the data shown above (calculated using bin sizes of 0.1 in (a) and 0.09 in (b)), normalized using the maximum bin value (a) and average non-zero bin value (b), and the dashed blue line shows the theoretical density of filaments using Eq (4) , where y 0 is set to be greater than F (this is the one exception to the assumption that y 0 ≤ F ), thus there is no bleached region in the panels. The filaments’ right endpoints are uniformly distributed on [0, 200] and the filaments have lengths which come from a Gaussian distribution with standard deviation 5 in (a) and 0.05 in (b). Eq (4a) is for w -values less than 0, where the density is lower since the right endpoints of filaments are not initially placed to the left of 0 (see panel (a)). For panel (a), if F − w > 60 (the mean length plus 2 standard deviations), the w coordinate is far enough to the left of F = 200 that the boundary effects (due to placement of the right endpoints) do not affect density. If y 0 is in the plateau region, the front of the traveling wave will be sharp. Different length distributions show the same qualitative features. Panel (c) shows the standard deviation of the filament density for different lengths of filaments and for four different values of total number of filaments. The simulations in (c) have filament lengths which are Gaussian distributions with varying length and the standard deviation is one tenth the length. The standard deviation is taken only for data on the plateau.
https://doi.org/10.1371/journal.pcbi.1010573.g003
To summarize, filaments with a short average length will give density measurements with greater variability and spatial derivatives. Measurements near the cell membrane may give an idea of average filament length due to the boundary.
3.3 Type 1 simulations and theory
In type 1 simulations the velocity for each filament does not change with time (see Fig 2 ). First we consider a special case of this, namely when the velocity is the same for all the filaments and the filament length, which does not change during the course of the experiment (here we do not model filament assembly or disassembly), is random. Then we consider fixed lengths and random (but constant) velocities. Finally we consider both random velocities and random lengths.
3.3.1 All filaments have the same constant velocity.
The solid curves in (a)-(c) are plots of a scaled version of Eq (4) and in (d)-(l) they are a scaled version of Eq (5) such that ‖ f ‖ ∞ = 1. The x’s are simulated results. The mean velocity for all simulations is 1 micron per minute and for (g)-(l) the standard deviation of the velocity is 0.25. In (a)-(c) the velocity is fixed and the insets show the simulated profile curves for the entire domain before bleaching and the region to be bleached is shown in grey. The bleached region is 50–60, 120–130, and 150–160 respectively. In (d)-(f) the velocity is uniformly distributed, (g)-(i) have Gaussian distributions, and (j)-(l) have gamma distributions. Panels (d), (g), and (j) have filaments with length 10 microns; (e), (h), and (k) have filaments with length 0.5 microns; and (f), (i) and (l) shows the average of 50 simulations (each a different realization) with filament length 0.5 microns. In these simulations, each filament has a velocity which does not change for the duration of the simulation. Each simulation in (a)-(c) has 200,000 filaments and in (d)-(l) 20,000. The right endpoints are uniformly distributed in (a)-(c) from 0 to 100 and in (d)-(l) from 0 to 470. The bleached region in (d)-(l) is from 200 to 210. The curves and x’s represent times 0.5 (blue and red), 1 (light blue and orange), 3 (lighter blue and light orange), and 5 minutes (cyan and yellow). The y axis is fluorescence intensity (a.u.) in all panels. Length : Row one—Gaussian, μ = 50, σ = 5 microns. Rows 2,3, and 4—fixed.
https://doi.org/10.1371/journal.pcbi.1010573.g004
As an example see the first row of Fig 4 . In Fig 4a , curves of a scaled version of Eq (4) are shown with data from simulations where the bleached region is in the region where the filament density is uniformly distributed (not just the right endpoints). The filament density is shown for the full domain before bleaching in the inset. The region to be bleached, between y 0 = 50 and y 1 = 60, is shown as grey. The profile curves are constant with a jump at the transition to the bleached region. In Fig 4b the bleached region is in a region near the right end of the interval where the filaments are located. In this region the filament density is not uniform and the profile curves are not constant before they drop down to zero. In Fig 4c the bleached region is at the right end of the region where the filaments are initially located y 0 = F = 150. The profile curves here are continuous and have no abrupt transition to zero. Regardless of the length distribution, if the bleached zone is in the region of the domain where the filament density is constant, the profile curves are constant with an abrupt change to zero.
To summarize, typically, if the filament velocities are constant the wave profile will have a front at y 0 which moves forward into the bleached zone without changing shape and there is almost no information about the length distribution. It may be possible to learn something about the filament lengths if the bleach region is near the cell membrane where the filament density may not be constant due to the boundary imposed by the membrane.
The fluorescence intensity profiles obtained experimentally are not traveling waves (see Fig 1c and 1d ). They have an abrupt transition at the time of bleaching from the fluorescent region to the bleached region, but as time evolves the transition becomes smoother and less abrupt. In order to explain the experimental data, we explored the effects of random filament velocity on the curves. From now on, the bleached region will always be in the “plateau” region where both the right endpoints of filaments and the filament density are uniformly distributed to avoid boundary effect in the theoretical and simulated results. Since we only consider filaments moving to the cell periphery, the right side of the profile curves and simulations do not give any additional information.
The rest of section 3.3 will show a primary result of this work—that the fluorescence intensity profile is affected only by filament velocity, and not by filament length .
3.3.2 Filaments with random velocity and fixed length.
The filament velocity distribution affects the fluorescence intensity profiles profoundly. The equation derived for fluorescent filament density with random velocities and fixed length filaments ( Eq (5) ) is compared to numerical simulations of type 1 in Fig 4d–4l . When the velocity is uniformly distributed the profiles are piecewise linear with the slope changing with time. For Gaussian distributed velocities the profiles are smooth curves with more abrupt transitions than the uniformly distributed velocities. The profile curves are also smooth curves in the case of gamma distributed velocity but the transitions are not as abrupt as when the Gaussian distribution is used.
We then turned our attention to how changes in the fixed length parameter affects the results of type 1 simulations. It is clear from Fig 4d–4l that as the length of the filaments increases the profiles from simulations approach the theoretical curve which is determined by the velocity distribution. If the length is small, there is more variation in the simulated results due to the random nature of the simulations but that variation can be averaged out giving profiles which are similar to those with filaments of longer lengths.
Fig 5c–5e , shows how the standard deviation of type 1 simulations vary with the filament lengths. In these simulations (similar to those shown in Fig 4 ) the averages of 50 simulations are plotted with error bars indicating the standard deviation for simulations with filaments of varying lengths and with uniformly distributed velocities. Clearly the variation increases as the filament length decreases. Depending on the quality of the data it may be able to surmise length information based on the noise in the data.
This figure compares the simulated results with random velocity and different length distributions. In panel (a) the type of length distribution changes, in panels (c)-(e) the length changes, and in (f)-(h) the mean length changes. Panel (b) is a blowup of the boxed region in panel (a). In panel (a) the average length for all distributions is 10 microns and for the Gaussian and gamma distributions the standard deviation is 0.2. In panels (c)-(e) the solid curves are plots of scaled Eq (5) such that ‖ f ‖ ∞ = 1 and the error bars are type 1 simulated results. The length of the error line is twice the standard deviation of the 50 realizations centered at the average of the realizations. The curves and error bars in (c)-(e) are profiles at times 0.5 (blue and red), 1 (light blue and orange), 3 (lighter blue and light orange), and 5 minutes (cyan and yellow). The y axis is fluorescence intensity (a.u.) in all panels. Length : (a)—varied distributions, (c)-(e)—fixed, (f)-(h)—uniform. Velocity : (a),(b), (f)-(h) gamma μ = 1, σ = 0.25; (c)-(e)—uniform on interval [0, 2].
https://doi.org/10.1371/journal.pcbi.1010573.g005
3.3.3 Filaments with random velocity and random length.
Next, we allowed the length to vary according to different distributions with the same mean while keeping the velocity distribution fixed as a gamma distribution. We do not have a theoretical curve to compare with these type 1 simulations because both velocity and length are random. Fig 5a , curves obtained with the three different distributions (uniform, Gaussian and gamma with the same mean length) for lengths are almost superimposed. In panels (f)-(h) the length distribution was left the same (a uniform distribution) but the mean length was altered from 0.5, 2, 4 microns. Again when the filaments have shorter lengths the data is noisier but still follows the same basic curve (determined by the velocity distribution).
3.4 Comparing type 1, 2, and 3 simulations
Here we compare results from type 1, 2, and 3 simulations with fixed filament length and various random velocities. Recall we simulated the velocity in three ways: type 1 each filament gets a different velocity but it remains constant throughout the simulation, type 2 velocities change after T on time units have elapsed but always come from the same distribution, and type 3 filaments have velocities which change after T on time units but then they stop in between velocity changes for T off time units.
https://doi.org/10.1371/journal.pcbi.1010573.g006
3.5 Fitting theory and simulations to the data
We have a theoretical formula ( Eq 5 ), and three types of simulations to compare to experimental data. We do not compare type 2 simulations to the data for two reasons. First, the results from the last section show that simulations of type 2 and type 3 are similar. Second, for long τ on (i.e., longer than the duration of the experiment) type 2 and type 3 simulations are the same. We now optimize the parameters in the theory, type 1, and type 3 simulations to fit the experimental data.
We fit thirteen data sets from five different experiments. All the data was from polarized migrating astrocytes showing asymmetric profiles of fluorescence recovery indicative of the polarization of the microtubule driven transport of IFs [ 12 ].
https://doi.org/10.1371/journal.pcbi.1010573.g007
Although, both type 1 and type 3 simulations fit the data well, the best fits come from type 3 simulations as can be seen, for one data set, in Fig 8 . Furthermore, in all cases we successfully simulated most of the fluorescence curves using a truncated gamma distribution for the velocities. This is not surprising since the videos of FRAP experiments clearly show a strong disparity in the filament speeds, with only a few filaments moving very fast and a large majority moving very slowly (see videos [ 12 ]). The gamma distribution is the only asymmetric distribution considered thus allowing for a fat tail.
This figure compares the simulated results (red shades) with experimental data (blue shades) for one data set. The figure has been separated into three regions showing results from the theoretical model, type 1 simulations, and type 3 simulations. Panels (a)-(e), (j), and (k) show all the time data together, whereas the rest show the time data in individual panels. When optimizing only the data in the unshaded area is used. The minimum value of the objective function is 0.0511, 0.0525, and 0.0139 for type 1 and for type 3 0.0415, 0.0404, and 0.0131 for uniform, Gaussian, and gamma distributed velocity respectively. The y axis is fluorescence intensity (a.u.) in all panels. Length : All—uniform with mean 5.025 microns.
https://doi.org/10.1371/journal.pcbi.1010573.g008
https://doi.org/10.1371/journal.pcbi.1010573.g009
4 Discussion
The work here builds a theoretical framework to quantitatively analyse the directed transport of anisotropic structures and allows the reconstruction of fluorescent profile curves generated by FRAP experiments. Here the focus is on IFs but the work is more generally applicable to all kind of anisotropic structures, such as mitochondria which are also actively transported along microtubules [ 23 ]. We show that data from FRAP experiments on IF, namely fluorescence intensity profile curves, reveal important information for determining the velocity of the filaments including mean velocity and shape of the distribution. In fact, the most important distribution governing the shape of the intensity profile curves is the distribution of the filament velocity, μ V . Profile curves which are observed to be traveling waves would suggest that all filaments are moving with the same constant velocity. On the contrary, profile curves which are piecewise linear with the slope changing in time suggest that the filament velocity is constant in time and uniformly distributed. Profile curves which are sigmoidal indicate velocities are normally or gamma distributed. Finally, we found that the filament length distribution has no impact on the global dynamics of filaments.
Our results show that for polarized migrating astrocytes with reduced retrograde transport, a gamma distribution for the velocity of the filaments best matches the data. Allowing the filaments to pause and restart with new velocities, gives the best experimental fit. In fact, in most of the data sets over half the filaments are stationary and most of the moving filaments have a small velocity. However, some filaments have a large velocity as indicated by the long tails in the velocity probability densities in Fig 9a and the long tails in the profile curves in Fig 8 . This is consistent with IF transport by one directional motor molecules with friction and with IF experiments where velocities were described to be non Gaussian with a high propensity of slow filaments in previous work [ 19 , 20 , 24 ]. Moreover, average velocities extracted from the fit of the profile curves with gamma distributions are consistent with values published in the literature [ 25 ], but lower than the range described for the transport of isolated small filaments called squiggles [ 25 , 26 ] and longer filaments measured in [ 17 ]. The distributions are consistent with filaments having a large range of velocities which is seen experimentally [ 25 ]. All of these velocities are lower than the maximum velocity of an individual kinesin-1 molecule of 0.668 microns per second [ 27 ] but which depends on the concentration of ATP and can be as low as 0.045 microns per second for low concentrations. Additionally, increasing the load on the kinesin molecule will decrease the velocity [ 27 ]. Long pauses have also been observed to last up to 25–80 percent of the time [ 25 ]. This matches our model predictions that at any one time fewer than half of the filaments are moving and the average percentage of time the filaments are paused ranges from 25 to 73 (with an average of 53.5).
The very slow speeds are not surprising because there are a lot of sources of friction for the transport of IF mainly driven by kinesin motors: interaction with other organelles, crosslinking proteins between microtubules and intermediate filaments such as plectin, or dyneins, although dynein activity has been shown to be inhibited [ 12 ]. Maintaining a network of filaments that is constantly being restructured requires a delicate balance where a portion of the network is stable, a portion is being dismantled, and a portion is being constructed.
Our results in the case of one directional transport also predict that other types of profile curves can be recovered with velocities which are not gamma distributed. These predictions could be used in other types of experiments investigating different cell conditions not described in [ 12 ]. For example, when the friction in the system is reduced, we expect the velocity distributions to be more symmetrically distributed (Gaussian) because only one motor is involved. This could be achieved by reducing the cross-linking of the filaments. Two possible methods would be to inhibit plectin, or completely inhibit dynein. Uniform and Dirac velocity distributions are ideal cases which were examined for the sake of comparison and are less biologically motivated.
There are several possible causes that would inhibit filament motion. Physical obstacles could hinder filament transport including the crowding from other filaments or crosslinking to other filaments. Stalled velocity due to the tug-of-war caused by motor molecules of opposing types could be another possible reason. Mathematical modeling shows that there are several scenarios where the majority of filaments remain in a state where the tug-of-war is unresolved resulting in stalled filaments [ 15 ]. Filaments detaching and staying detached from the microtubule would also be stalled. Finally there are direct and indirect interactions with actin which could cause anchoring of the filaments [ 17 ]. Overall, the data suggests that many of the fluorescent filaments are in the static portion of the network or in the process of being disassociated or associated with it. Thus the majority of filaments are stationary or moving with very slow velocities. This does not preclude the possibility that many filaments which are not associated with the stationary portion of the network are also pausing for long periods of time.
Assembly and disassembly of IF occurs on a time scale of hours in neurons [ 28 ]; whereas, the FRAP experiments take place on the time scale of minutes. Yet in epithelial cells, keratin assembly/disassembly occurs at the time scale of minutes [ 29 ]. But in our experiments the profile curves remain low in the middle of the bleached region indicating that assembly is not playing an important role. Preliminary results of simulations with length changes suggest that the results presented here are robust. This is not surprising since length does not substantially affect the profile curves. Of course, in systems where filament length can change at a time scale comparable to that of active transport, our analysis is not appropriate and the profile curves will have different characteristics.
Let’s consider three possible refinements to model. First, we could allow filament transport in two directions. This would be important when considering symmetric profile curves, for example those observed in astrocytes 8 hours after wounding, when cell polarity is well established [ 12 ]. Two directional analysis would indicate whether the filament velocity has the same characteristics in both directions. When there are no non-fluorescent moving filaments, the bleached region is symmetric, and the velocities are equal in magnitude but opposite in direction no new information is gained. If there are non-fluorescent, moving filaments the profile curves will be distorted but in a symmetric manner. If the velocity distributions are the same but with different mean velocities (still in opposite directions) the moving non-fluorescent filaments could break the symmetry. Second, we could allow the filament velocity to be correlated with filament length [ 14 ]. How this correlation would alter the profile curves is hard to predict without knowing how the length and speed are related. Third, we could consider the elastic nature of IFs. The elasticity of the filaments has two possible relevant effects: length change and speed change [ 14 ]. The first should not affect the profile curves but the second could. Finally, by combining the information about filament velocities found here with models of filament transport [ 14 , 15 ], it should be possible to elucidate properties of motors involved in the transport. Additionally, when the cell is stationary, while the filament network is very dynamic, there is no net change in filament density.
In future mathematical models, we plan to investigate conditions which would allow a steady state density and give insight into how the cell maintains a dynamic network with no net filament transport. Further mathematical analysis could be done using other mathematical formalization. A standard method would be to use the Chapman-Kolmogorov equation, but for our problem that requires simplification. All three types of simulations can naturally be thought of as realizations of stochastic processes. With some work and simplifying assumptions, they can be framed as Markov processes. Type 1 simulations, while certainly stochastic, have all of the randomness front-loaded at time 0. Consequently, they are trivially Markovian because the state of the system at each strictly positive time depends deterministically on the state at any previous time. The easiest way to frame type 2 and type 3 simulations as Markovian is to consider only the velocity process and to require the elapsed time between velocity jumps to be exponentially-distributed (rather than uniform), leaving us with classical examples of continuous-time, time-homogeneous Markov processes. For interested readers the resulting Chapman-Kolmogorov equation and other related formulas are given in S2 Appendix .
In summary, the modeling framework proposed in this work provides an in-silico platform to study the impact of IF protein post-translational modifications [ 30 ] or mutations [ 31 ], depleting or silencing one motor type, or altering the IF network composition on the IF transport and organization in cells.
Supporting information
S1 appendix. appendix 1..
https://doi.org/10.1371/journal.pcbi.1010573.s001
S2 Appendix. Appendix 2.
https://doi.org/10.1371/journal.pcbi.1010573.s002
S1 Datasets. The FRAP data.
https://doi.org/10.1371/journal.pcbi.1010573.s003
- View Article
- PubMed/NCBI
- Google Scholar

An official website of the United States government
The .gov means it’s official. Federal government websites often end in .gov or .mil. Before sharing sensitive information, make sure you’re on a federal government site.
The site is secure. The https:// ensures that you are connecting to the official website and that any information you provide is encrypted and transmitted securely.
- Publications
- Account settings
- My Bibliography
- Collections
- Citation manager
Save citation to file
Email citation, add to collections.
- Create a new collection
- Add to an existing collection
Add to My Bibliography
Your saved search, create a file for external citation management software, your rss feed.
- Search in PubMed
- Search in NLM Catalog
- Add to Search
Fluorescence recovery after photobleaching
Affiliation.
- 1 Faculty of Life Sciences, The University of Manchester, Manchester, UK.
- PMID: 21748690
- DOI: 10.1007/978-1-61779-207-6_26
This chapter describes the use of microscope-based fluorescence recovery after photobleaching (FRAP). To quantify the dynamics of proteins within a subcellular compartment, we first outline the general aspects of FRAP experiments and then provide a detailed protocol of how to measure and analyse the most important parameters of FRAP experiments such as mobile fraction and half-time of recovery.
PubMed Disclaimer
Similar articles
- Analysis of integrin dynamics by fluorescence recovery after photobleaching. Wehrle-Haller B. Wehrle-Haller B. Methods Mol Biol. 2007;370:173-202. doi: 10.1007/978-1-59745-353-0_13. Methods Mol Biol. 2007. PMID: 17416995
- Quantitative FRAP in analysis of molecular binding dynamics in vivo. McNally JG. McNally JG. Methods Cell Biol. 2008;85:329-51. doi: 10.1016/S0091-679X(08)85014-5. Methods Cell Biol. 2008. PMID: 18155469
- Mechanical forces alter zyxin unbinding kinetics within focal adhesions of living cells. Lele TP, Pendse J, Kumar S, Salanga M, Karavitis J, Ingber DE. Lele TP, et al. J Cell Physiol. 2006 Apr;207(1):187-94. doi: 10.1002/jcp.20550. J Cell Physiol. 2006. PMID: 16288479
- Measuring transcription dynamics in living cells using a photobleaching approach. Hochberg H, Brody Y, Shav-Tal Y. Hochberg H, et al. Methods. 2017 May 1;120:58-64. doi: 10.1016/j.ymeth.2017.04.007. Epub 2017 Apr 21. Methods. 2017. PMID: 28434903 Review.
- Autofluorescent proteins. Dobbie IM, Lowndes NF, Sullivan KF. Dobbie IM, et al. Methods Cell Biol. 2008;85:1-22. doi: 10.1016/S0091-679X(08)85001-7. Methods Cell Biol. 2008. PMID: 18155456 Review.
- A dynamic role for transcription factors in restoring transcription through mitosis. Budzyński MA, Wong AKL, Faghihi A, Teves SS. Budzyński MA, et al. Biochem Soc Trans. 2024 Apr 24;52(2):821-830. doi: 10.1042/BST20231022. Biochem Soc Trans. 2024. PMID: 38526206 Free PMC article. Review.
- RACK1 promotes Shigella flexneri actin-mediated invasion, motility, and cell-to-cell spreading. Valenzuela-Valderas KN, Farrashzadeh E, Chang YY, Shi Y, Raudonis R, Leung BM, Rohde JR, Enninga J, Cheng Z. Valenzuela-Valderas KN, et al. iScience. 2023 Oct 14;26(11):108216. doi: 10.1016/j.isci.2023.108216. eCollection 2023 Nov 17. iScience. 2023. PMID: 37953961 Free PMC article.
- Light Microscopy and Dynamic Light Scattering to Study Liquid-Liquid Phase Separation of Tau Proteins In Vitro. Hochmair J, Exner C, Betzel C, Mandelkow E, Wegmann S. Hochmair J, et al. Methods Mol Biol. 2023;2551:225-243. doi: 10.1007/978-1-0716-2597-2_15. Methods Mol Biol. 2023. PMID: 36310206
- Cancer Cells Evade Stress-Induced Apoptosis by Promoting HSP70-Dependent Clearance of Stress Granules. Wang A, Abulaiti X, Zhang H, Su H, Liu G, Gao S, Li L. Wang A, et al. Cancers (Basel). 2022 Sep 25;14(19):4671. doi: 10.3390/cancers14194671. Cancers (Basel). 2022. PMID: 36230594 Free PMC article.
- Inferences from FRAP data are model dependent: A subdiffusive analysis. Alexander AM, Lawley SD. Alexander AM, et al. Biophys J. 2022 Oct 18;121(20):3795-3810. doi: 10.1016/j.bpj.2022.09.015. Epub 2022 Sep 19. Biophys J. 2022. PMID: 36127879 Free PMC article.
Publication types
- Search in MeSH
Grants and funding
- BB/G004552/1/Biotechnology and Biological Sciences Research Council/United Kingdom
- Wellcome Trust/United Kingdom
LinkOut - more resources
Full text sources, miscellaneous.
- NCI CPTAC Assay Portal
- Citation Manager
NCBI Literature Resources
MeSH PMC Bookshelf Disclaimer
The PubMed wordmark and PubMed logo are registered trademarks of the U.S. Department of Health and Human Services (HHS). Unauthorized use of these marks is strictly prohibited.
Suggestions
- Mateo Digital Microscopes
- MyVeo All-In-One Surgical Visualization Headset
- UC Enuity Ultramicrotome
- Ivesta 3 Greenough Stereo Microscopes
- Service & Support
Select your country and language
Not all products or services are approved or offered in every market, and approved labelling and instructions may vary between countries. Please contact your local representative for further information.
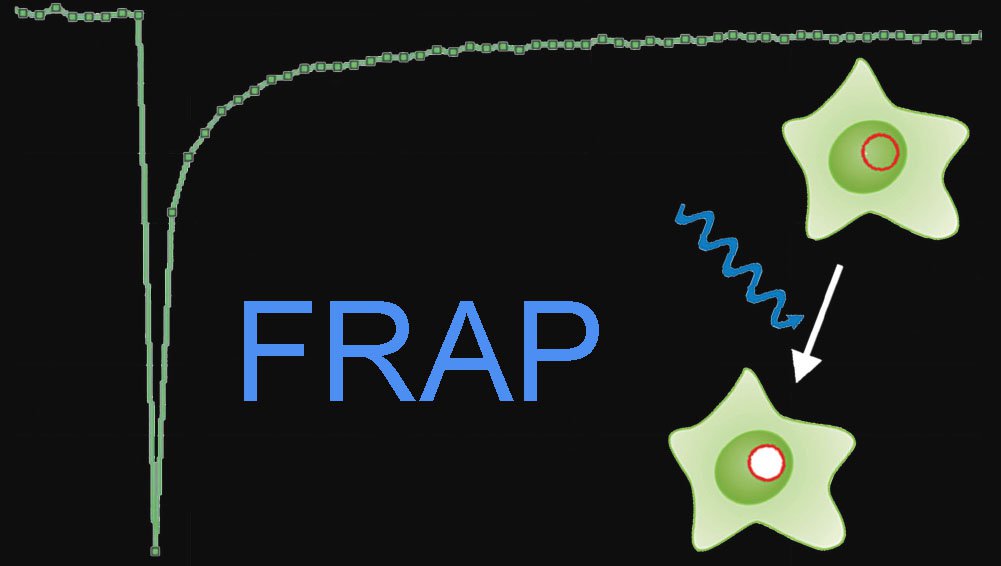
Fluorescence Recovery after Photobleaching (FRAP) and its Offspring
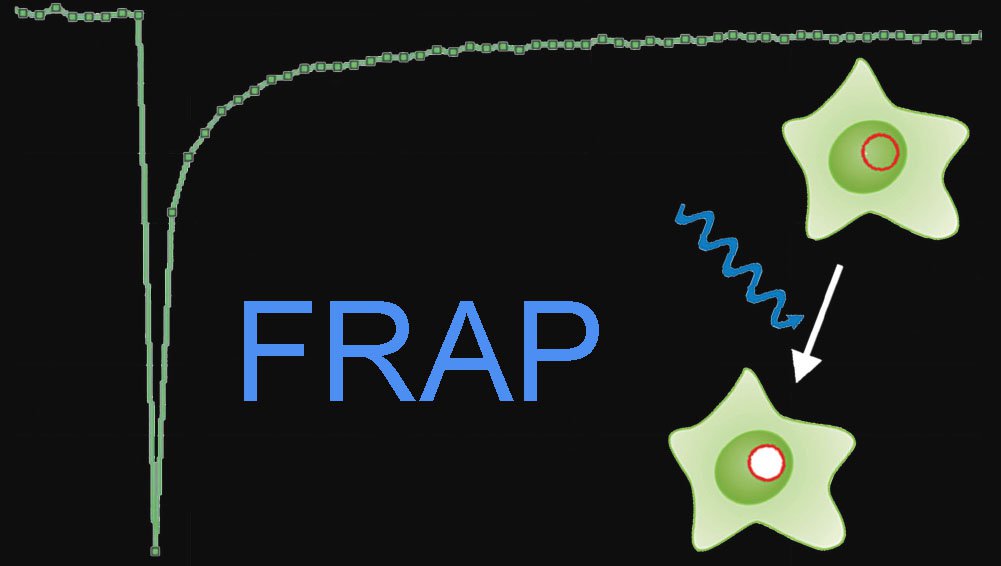
FRAP (Fluorescence recovery after photobleaching) is used to characterize the mobility of cellular molecules. The experimental setup comprises a microscope, a light source and a fluorescent probe coupled to the molecule of interest. Several images using a low light level are acquired to determine the initial fluorescence, and then a high level of light for a short time inside a region of interest is applied to bleach the fluorescence. Finally, another set of images using a light level sufficiently low to prevent further bleaching is acquired to gain insight into the redistribution of molecules via recovery of fluorescence.
Fluorescence Recovery after Photo Bleaching (FRAP)
The FRAP technique was first used to analyze the mobility of individual lipid molecules within a cell membrane. FRAP can also be used to study protein dynamics outside the membrane: a region of interest within the cytoplasm or cellular structures within the cell can be monitored.
Photobleaching experiments can be conducted with confocal laser scanning microscopes where the laser is used at high intensity for bleaching and low intensity for image recording. It employs irradiation of a fluorophore in a living sample with a short laser to erase fluorescence followed by time-resolved image recording of the sample. In consequence there are three different image sequences: The prebleach sequence with low laser power setting to have a reference value, the bleach sequence with high laser power inside the ROI and the postbleach setting with low laser power setting to examine the recovery of fluorescence. All other bleaching methods are derived from this basic principle (Figure 1).
Fig. 1: Top: Fluorescence intensities of the Bleach-ROI in a time series, the bleach interval is marked red. Bottom: The same cell in a schematic drawing within a time series. At time point two, a region of interest (ROI) within a cell is bleached (arrow). Non bleached molecules are moving into the ROI. The speed of redistribution can be determined.
Typically, the protein of interest is made fluorescent by expression as a GFP (green fluorescent protein cloned from the jellyfish A. victoria) fusion protein or by tagging the protein of interest with reactive ligands which then binds a fluorescent dye. The most common way to fluorescently tag proteins in living cells is GFP technology. Of the different spectral GFP mutants EGFP is the best because of its high quantum yield, its low tendency of photobleaching as well as its relative photostability during post-bleach image acquisition. The speed of redistribution is dependent on molecule size or viscosity of environment or degree of interaction with other molecules or intracellular transport processes. Additionally, the ratio between mobile and immobile molecules can be determined.
As there are numerous possibilities of experiments depending on the application, there is no general recipe for types of experiments. Please refer to the literature cited in the references part.
Fluorescence loss in photo bleaching (FLIP)
Typically, FLIP is used to examine whether cellular organelles like ER or Golgi apparatus are interconnected. A FLIP experiment can gain insight into whether molecules are mobile, immobile or restricted to compartments.
Fig. 2: shows the same cell in a schematic drawing within a time series. Starting at time point two, a region of interest (ROI) within a cell is bleached (arrow) repeatedly. Bleached molecules are spreading out. The loss of fluorescence indicates, whether cell organelles are physically connected.
Inverse FRAP
In contrast to FRAP , inverse FRAP (i- FRAP ) allows direct analysis of the fluorescent molecules. The fluorescent molecules outside an organelle are bleached. Thus, the efflux of fluorescent molecules out of organelles can be monitored directly without bleaching them. A disadvantage is that this method requires lot of light intensity to bleach the whole cell.
Fig. 3: shows the same cell in a schematic drawing within a time series. At time point two, the whole cell area is bleached (arrow). Non bleached molecules are spreading out. The speed of fluorescence recovery in the cell can be measured.
Fluorescence localization after photobleaching
Fluorescence Localization After Photobleaching (FLAP) is a ratiometric method which can be applied to two channels. The experiment requires two different fluorescent labels and only one of the two labels is bleached. The two labels can be tagged to two proteins or one. The non bleached population is the reference measure. The ratio of the bleached and of a second non-bleached area then gives insight into mobility of the proteins. In contrast to FRAP and FLIP, it is a direct measurement method and can be applied to structures which change their morphology rather fast.
Fig. 4: shows the same cell in a schematic drawing within a time series. At time point two, the ROI is bleached (arrow) with a suitable wavelength which only bleaches the red fluorescence, for example.
Useful links
- Analysis of FRAP Curves, Kota Miura: www.embl.de/eamnet/frap/FRAP6.html
- Introduction to FRAP , Timo Zimmermann: www.embl.de/eamnet/downloads/courses/FRAP2005/tzimmermann_frap.pdf
- Video on FRAP Technique from Essential Cell Biology, 3 rd Edition Alberts, Bray, Hopkin, Johnson, Lewis, Raff, Roberts, & Walter ISBN: 978-0-8153-4129-1: www.youtube.com/watch?v=LicQb_SnCSI
- Lecture of Jennifer Lippincott-Schwartz, Part 2: Photobleaching and Photoactivation: www.youtube.com/watch?v=bhjP9PqfJRE
- Online Manual fot the MBF-ImagsJ Collection: www.macbiophotonics.ca/imagej/intensity_vs_time_ana.htm
- Axelrod D, Koppel DE, Schlessinger J, Elson E, Webb WW: Mobility measurement by analysis of fluorescence photobleaching recovery kinetics. Biophys J 16:9 (1976) 1055–69.
- Phair RD, Misteli T: Kinetic modelling approaches to in vivo imaging. Nat Rev Mol Cell Biol 2:12 (2001) 898–907.
- Snapp E, Altan N, Lippincott-Schwartz J: Measuring protein mobility by photobleaching GFP -chimeras in living cells. Current Protocols, Online Posting Date: August 2003.
- Lippincott-Schwartz J, Altan-Bonnet N, Patterson GH: Photobleaching and photoactivation: following protein dynamics in living cells.
- Phair RD, Misteli T: High mobility of proteins in the mammalian cell nucleus. Nature 404:6778 (2000) 604–9.
- Dundr M, Misteli T: Measuring Dynamics of Nuclear Proteins by Photobleaching. Current Protocols, Online Posting Date: August 2003.
- Dunn GA, Dobbie IM, Monypenny J, Holt MR, Zicha D: Fluorescence localisation after photobleaching (FLAP): a new method for studying protein dynamics in living cells. J Microscopy 205 (2002) 109–112.
Related Articles
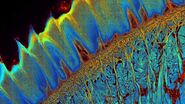
A Guide to Fluorescence Lifetime Imaging Microscopy (FLIM)
The fluorescence lifetime is a measure of how long a fluorophore remains on average in its excited…
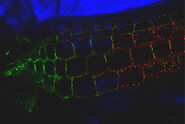
Live-Cell Imaging Techniques
The understanding of complex and/or fast cellular dynamics is an important step for exploring…
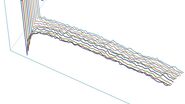
Step by Step Guide for FRAP Experiments
Fluorescence Recovery After Photobleaching ( FRAP ) has been considered the most widely applied method…
Interested to know more? Talk to our experts.
I need a configuration or price info.
I need an on site or remote demo.
Service & Repair
I need repair, technical service, spare parts or service contract.
Application Support
I need assistance/training in how to operate my system properly, or how to run a specific application with my system.
Do you prefer personal consulting? Show local contacts
Are you sure you want to close the form?
no yes

An official website of the United States government
The .gov means it’s official. Federal government websites often end in .gov or .mil. Before sharing sensitive information, make sure you’re on a federal government site.
The site is secure. The https:// ensures that you are connecting to the official website and that any information you provide is encrypted and transmitted securely.
- Publications
- Account settings
The PMC website is updating on October 15, 2024. Learn More or Try it out now .
- Advanced Search
- Journal List
- v.115(7); 2018 Oct 2
The Development and Enhancement of FRAP as a Key Tool for Investigating Protein Dynamics
Jennifer lippincott-schwartz.
1 Janelia Research Campus, Howard Hughes Medical Institute, Ashburn, Virginia
Erik Lee Snapp
Robert d. phair.
2 Integrative Bioinformatics, Inc., Mountain View, California
The saga of fluorescence recovery after photobleaching (FRAP) illustrates how disparate technical developments impact science. Starting with the classic 1976 Axelrod et al. work in Biophysical Journal , FRAP (originally fluorescence photobleaching recovery) opened the door to extraction of quantitative information from photobleaching experiments, laying the experimental and theoretical groundwork for quantifying both the mobility and the mobile fraction of a labeled population of proteins. Over the ensuing years, FRAP’s reach dramatically expanded, with new developments in GFP technology and turn-key confocal microscopy, which enabled measurement of protein diffusion and binding/dissociation rates in virtually every compartment within the cell. The FRAP technique and data catalyzed an exchange of ideas between biophysicists studying membrane dynamics, cell biologists focused on intracellular dynamics, and systems biologists modeling the dynamics of cell activity. The outcome transformed the field of cellular biology, leading to a fundamental rethinking of long-held theories of cellular dynamism. Here, we review the pivotal FRAP studies that made these developments and conceptual changes possible, which gave rise to current models of complex cell dynamics.
“Biology is wet and dynamic. Molecules, subcellular organelles and cells, immersed in an aqueous environment, are in continuous riotous motion” ( 1 ). Today, we know this is true largely through the application of a single, powerful technique—fluorescence recovery (or redistribution) after photobleaching (FRAP). The development of FRAP in the 1970s permitted the detection and measurement of protein motility in vivo by investigating the return of fluorescence to areas of the cell subject to targeted photobleaching ( 2 ). This potent technique has been steadily enhanced to address whether a protein (or other biomolecules) diffuses freely, has directed motion, or undergoes binding events in its native cellular environment ( 3 , 4 , 5 ). New analytical tools have permitted the kinetics of diffusion to be distinguished from the kinetics of binding under various conditions using FRAP ( 6 ). And, as use of FRAP has further transitioned from studies of diffusion/binding to studies of endomembrane trafficking on time and space scales much greater than those for which diffusion is a concern, ordinary differential equations of compartmental analysis have been applied to FRAP results ( 7 ). This has allowed the study of complex models of cell dynamics, including membrane trafficking pathways and regulatory feedback mechanisms invoked by non-steady-state experimental perturbations.
The history and future potential of FRAP, discussed here, represent convergence in science, in which intermingling of different fields opens up a fruitful new approach. FRAP was initially developed by biophysicists over 40 years ago to study the diffusive properties of fluorescent dyes using custom-built devices. Because of technical developments in microscopy and fluorescent proteins, FRAP transitioned 20 years later into the most widely used technique for quantifying protein and organelle dynamics in live cells. As with any new approach, there were challenges in applying it reliably to a range of cellular processes. But improved fluorescent probes, innovative applications, and new computational methods have allowed FRAP to usher in a new era of cell biology and biophysics. Today, FRAP makes it possible to quantify the kinetics of virtually any biological process and investigate its underlying mechanisms.
Brownian motion and the origins of FRAP
Diffusive, random, or Brownian molecular motion was first described by the botanist Robert Brown in 1827 when he observed the free motion of plant organelles ejected from pollen grains into a surrounding aqueous solution. Einstein proposed this type of diffusive motion resulted from random thermal movement of water molecules displacing the structures. He mathematically described such diffusive motion as a mean-squared displacement (dependent on the temperature of the medium), which was inversely proportional to the object's hydrodynamic radius ( r h ) and the viscosity of the medium ( η ) (the Stokes-Einstein relationship D = kT /6 πηr h ) ( 8 ). It took another 65 years before proteins within cells could be visualized to test whether they undergo Brownian motion or whether they are propelled, bound to immobile scaffolds, or confined by barriers.
The classic Frye and Edidin heterokaryon surface-protein-mixing experiment using fluorescent-dye-labeled antibodies ( 9 ) provided the first indication that proteins on membranes can be mobile. Their study revealed that plasma membrane proteins from two different cells exchange and mix within the fused plasma membrane of the two formerly distinct cells. The dye-labeled antibodies used in their study recognized surface proteins unique to each cell. When applied at different time points after fusion, increasing degrees of mixing were observed. Their findings indicated that at least some proteins residing on the surface of cells were not fixed and could move between surfaces. A complementary study by Edidin and Fambrough ( 10 ) cemented this principle. They observed diffusive movement when cell surface proteins of a muscle fiber were labeled in a small spot with fluorescent-dye-labeled monovalent antibodies.
The following year, Poo and Cone ( 11 ) and Liebman and Entine ( 12 ) independently carried out some of the first photobleaching experiments, applying laser bursts to endogenous rhodopsin in amphibian disc membranes. They used a custom-built microspectrophotometer fitted with a high-quantum-efficiency photomultiplier to photobleach the proteins and then measured movement of bleached molecules to the unbleached area in a time series of line traces of the adjacent bleached and unbleached regions with a 4 μ m scanning beam. Their approach worked because intense light causes the rhodopsin chromophore to undergo cis - trans isomerization into an effectively dark state. The results demonstrated that these molecules moved diffusively. Each group solved a distinct two-dimensional diffusion equation for a closed boundary disk and then calculated a D eff value of ∼0.35–0.39 μ m 2 /s for the integral membrane protein. Together, the work demonstrated that rhodopsin proteins inside cells undergo random motion and are not fixed in place. A related study by Peters et al. photobleached erythrocyte membrane proteins randomly labeled with a fluorescein isothiocyanate fluorescent dye ( 13 ). However, no recovery was observed, as the major erythrocyte membrane proteins are constrained by tight binding to the actin cytoskeleton. Interestingly, Peters et al. coined the FRAP acronym. The earlier studies from Liebman and Entine and from Poo and Cone did not use any acronyms for photobleaching.
Daniel Axelrod, working with Watt Webb and others, made a quantum leap forward by discovering how to transform photobleaching into a general research tool ( 2 ). The team devised a setup ( Fig. 1 A ) for making reliable photobleaching measurements of fluorescent dye solutions and thus were not confined to systems that endogenously expressed the rhodopsin protein. Moreover, Axelrod et al. ( 2 ) introduced a key modification to the photobleaching method. Instead of a prolonged exposure to a distinct light source, a neutral density filter was placed after a laser to enable high illumination for rapid photobleaching of a spot and low intensity illumination during acquisition of fluorescence recovery to minimize further photobleaching and consequent signal loss. This permitted imaging of dim populations of molecules that moved quickly. Combining these results with careful analysis laid the experimental and theoretical groundwork for using FRAP to quantify both the mobility and the mobile fraction of a fluorescently labeled population of molecules within cells. Note that Axelrod et al. used the acronym FPR (fluorescence photobleaching recovery). This acronym did not gain much traction, as other photobleaching studies later in 1976 opted for the use of FRAP ( 14 , 15 ).

( A ) Original photobleaching microscope layout. ( B ) Plotting of the normalized initial post-bleach fluorophore concentration with a Gaussian beam. ( C ) Graphic illustration of the method used for “best fit” of theoretical curves to experimental data. Selected figures from Axelrod et al. ( 2 ).
In their analysis of recovery kinetics, Axelrod et al. measured concentrations of bleached and unbleached molecules in relation to the Gaussian laser beam radius and intensity (see Fig. 1 B ; ( 2 )). The amount of bleaching induced in a discrete time equaled “ K ,” which established the initial postbleach condition. Using this value along with the initial and boundary concentrations, differential equations were applied to derive the diffusion and convection contributions to molecular mobility. This was one of the earliest efforts to examine the respective contributions of pure diffusion and directed motion or “flow.” Axelrod et al. posited that the shape of the recovery plot and extent of recovery of unbleached molecules into the photobleached region could reveal the relative contributions of flow and diffusion to molecular mobility. The group further noted that not all unbleached molecules necessarily move into the photobleached region during the time course of the experiment and thus comprised an “immobile fraction” that coexisted with the “mobile fraction” in the sample. The group appreciated that measuring these fractions could provide potential biological insight into whether a population of molecules is freely mobile, trapped in a physically distinct compartment, or part of a comparatively large complex that moves slowly during the time course of the experiment.
The Axelrod et al. experimental FRAP setup was tested on rhodamine 6G dye in water and in a viscous 1:1 mix of water and glycerol. Dye mobility was 4.5× higher in water than in the glycerol water mix, and the values were extremely close to those predicted by theory ( Fig. 1 C ). In making these measurements, the relative contributions of laser power, time, bleach depth, and bleach width were carefully considered, making the approach generalizable. The theory and analytical tools were immediately adopted by other groups using photobleaching to study molecular mobility in cells ( 14 , 15 ). In the first four years after the publication of this study, over 50 studies applied this spot-photobleaching approach to study the mobility of dye-labeled proteins on the plasma membrane of cells. Today, over 2400 publications have cited the contribution of the Axelrod et al. study for clarifying how to analyze diffusion and motion of proteins in cells.
Transition to confocal FRAP with GFP-tagged proteins
The results using Axelrod et al.’s approach were striking, but largely limited to dyes and dye-labeled antibodies that could be attached to cell-surface molecules. This restricted the application of FRAP methodology for characterizing protein diffusion rates because fluorescent dyes 1) can produce toxic free radicals that can impact protein behavior and cell health and 2) have to be attached to a protein by a cell impermeant antibody or lectin. In the mid-1990s, two exciting developments in cell biology and imaging resolved these problems, making it possible to use Axelrod et al.’s fundamental techniques in a way that was truly generalizable. These key developments were the creation of green fluorescent protein (GFP) technology and the rise of commercially available, laser scanning confocal microscopes. Together, these innovations turned FRAP into one of the most powerful tools for investigating intracellular protein and organelle dynamics.
GFP technology ( 16 , 17 ) made it possible to visualize the steady state distribution of tagged proteins in living cells, often in subcellular regions inaccessible to microinjection (e.g., the endoplasmic reticulum (ER) and mitochondria). Thousands of published studies have amply demonstrated the functional importance of this information. Central to this success was the laser scanning confocal microscope. Turn-key confocal systems enabled imaging of GFP constructs with high signal/noise, providing useful information about changes in steady state distributions of proteins over time. Yet, steady-state distributions of molecules rarely inform whether proteins are static, moving within a cellular compartment, exchanging in and out of a compartment, or undergoing progressive movement from one site or compartment to another. Investigation of such questions had been primarily restricted to the realm of biochemistry with radioactive pulse-chase labeling or enzymatic processing of molecules. Although these methods are powerful cornerstones of cell biology, temporal analyses are usually on the scale of minutes, and spatial information is absent. The availability of GFP and laser scanning confocal microscopes made it possible to follow protein dynamics in cells with unprecedented spatiotemporal resolution.
Initially, GFP was particularly valued because of its resistance to photobleaching during prolonged imaging ( 18 ). Fluorescein isothiocyanate and other fluorescent dyes at the time were notoriously prone to photobleaching. Then, investigators using confocal microscopes realized that photobleaching of GFP-tagged proteins was possible if they used a high-intensity laser pulse from their microscopes ( 19 ). Indeed, GFP was found to be an ideal confocal FRAP probe: it could be irreversibly photobleached using a high-intensity confocal laser pulse and be imaged without significant photobleaching using low-intensity illumination. With GFP-expressing cells and a confocal microscope for FRAP, questions posed by Axelrod et al. about cell surface molecules could now be answered for any set of proteins within the cell. Soon, it was possible to determine diffusion coefficients not just on the surface of plasma membranes but also in cytoplasm and organelles. Using known targeting sequences, GFP was, in the space of two or three years, expressed and photobleached in every organelle ( 20 , 21 , 22 ).
The key attraction of confocal FRAP using GFP-tagged proteins was its simplicity: bleach a region of interest and then monitor the time course of the fluorescence recovery ( Fig. 2 ). Another appeal of FRAP using GFP-tagged proteins was that it was minimally invasive: photobleaching simply makes a fraction of the fluorescently labeled molecules invisible without perturbing their physical and chemical interactions. This can be seen as a restatement of the basic indistinguishability principle of tracer kinetics. After FRAP, two important parameters of protein motion could be readily deduced: the mobile fraction of fluorescence molecules and the rate of their mobility (e.g., effective diffusion coefficient, D eff ). Calculations of the mobile fraction only required comparing the fluorescence in the bleached region after full recovery with the fluorescence before bleaching and just after bleaching, whereas D eff could be calculated by applying various diffusion equations and simulations, e.g., ( 23 , 24 , 25 , 26 ). It is important to note that the Axelrod et al. formula has been frequently misapplied in the cell biology literature and produces grossly inaccurate diffusion calculations when used with laser scanning confocal microscopy FRAP. The original equation was developed for a single-spot photobleach in a continuous sheet. Photobleaching of different geometric regions of interest (ROIs) using a laser scanning confocal involves a raster scan bleach that has different spatial and temporal properties and assumptions relative to the conditions calculated by Axelrod. Therefore, confocal-specific diffusion equations and simulations need to be applied ( 20 , 21 , 22 , 23 ).

FRAP of COS-7 cell coexpressing an ER luminal and an ER membrane probe. Imaging was performed on a laser scanning confocal microscope. Note that the ER luminal probe (i.e., calreticulin-signal-sequence-mCherry-KDEL) recovers significantly faster than the ER membrane probe (i.e., mEmerald-Sec61 β ), which is characteristic of proteins localized to the lumen versus the membrane of an organelle. The whole cell is imaged (although only part of it is shown here), which enables visualization of the source(s) of fluorescence recovery and robust direct measurement of mobile fractions of unbleached proteins. Scale bars, 5 μ m (courtesy of Christopher Obara, Janelia Research Campus).
Rethinking long-held theories of cellular dynamics
The new experimental observations derived from FRAP experiments were often incompatible with the knowledge at the time. This was especially true because most biological understanding up to this time was derived from static measurements (e.g., electron microscopy and immunofluorescent microscopy) or from in vitro systems (e.g., biochemical measurements in cell lysates). Not surprisingly, these approaches led to the view of cellular compartments and their associated proteins being static or nonmotile. FRAP results revolutionized this thinking by demonstrating that virtually all subcellular compartments are highly dynamic, with their lipid and protein content undergoing extensive diffusion and sorting.
One of the first subcompartments whose understanding was profoundly impacted by FRAP results was the Golgi apparatus. The classical view held that integral membrane enzymes within the Golgi apparatus undergo extensive interactions that “fix” these proteins within particular cisternae ( 27 ). This would prevent these enzymes from being carried along with secretory cargo out of the Golgi apparatus toward the plasma membrane or other destinations. FRAP results, however, did not support this model. GFP-tagged Golgi apparatus enzymes were observed to undergo rapid lateral diffusion, seemingly unhindered by any immobilizing interactions ( 19 ). Additional FRAP experiments showed that Golgi enzymes slowly cycle between the ER and Golgi, following a retrograde pathway back to the ER ( 28 , 29 , 30 ). This recycling pathway was shown to be stimulated during mitosis, resulting in Golgi enzymes relocating into the ER in mitotic cells ( 28 , 29 ). At the end of mitosis, Golgi enzymes left the ER along with other secretory cargo, reforming new Golgi structures de novo ( 28 ). These observations prompted major rethinking regarding how the Golgi complex is organized and functions.
Similarly, in the ER, FRAP studies showed most resident membrane and luminal protein species to be highly mobile, with GFP-labeled proteins diffusing throughout the ER on a timescale of minutes ( 19 , 24 , 25 ). These observations led to alternative models for how these proteins avoided being exported, like secretory proteins, from the ER ( 31 ). Equally unanticipated were findings about misfolded proteins and their chaperones in the ER. Retention of misfolded secretory proteins in the ER is a cornerstone of the principle of protein quality control. Based on analogies to affinity chromatography, the prevailing model of quality control in the 1990s was that misfolded secretory proteins are retained in the ER because they bind to and are immobilized by a matrix of chaperone proteins. However, photobleaching of a GFP-tagged misfolded protein reporter—the temperature-sensitive vesicular stomatitis virus G protein, ts045—revealed that the reporter remained equally mobile in the ER whether correctly folded or misfolded under a variety of misfolding conditions ( 32 ). These results revealed that ER retention of misfolded proteins is not due to the proteins being held in place in the ER. Subsequent photobleaching studies investigating the mobilities of GFP-tagged ER chaperones including calreticulin, GRP94, BiP, and BiP cofactors established that ER chaperones are also mobile and not part of an immobile matrix in homeostatic cells ( 33 , 34 , 35 , 36 , 37 , 38 ). Even during stressful conditions that induce global misfolding of secretory proteins in the ER, these chaperones remain mobile throughout the ER ( 33 , 34 , 35 , 37 , 38 ). Importantly, BiP-mGFP mobility was found to decrease when bound to misfolded proteins. In fact, this property was exploited to use photobleaching of the tagged chaperone to create the first live-cell quantitative biosensor of the unfolded secretory protein burden ( 37 ).
Although many membrane proteins are highly mobile in different organelles, some exhibit dramatic changes in their mobility depending on where they are localized. The nuclear envelope (NE)-localized lamin-B receptor is perhaps the most striking example. This transmembrane protein is highly mobile when localized in the ER but becomes immobile upon redistribution into the NE in interphase cells ( Fig. 3 ) ( 33 ). During mitosis, once the NE fuses with the ER, the receptor diffuses freely and rapidly throughout the ER ( 39 ). This suggests that in interphase, lamin-B receptor binds tightly to an immobile nuclear scaffold of lamins near the NE, whereas during mitosis, the receptor is released. Similar behavior was observed for nuclear pore complex components ( 40 ). At the plasma membrane (PM), GFP-E-cadherin proteins in polarizing epithelial cells were observed to be highly mobile in the PM but shifted to being largely immobile upon recruitment to cell-cell contact sites ( 41 ). In general, proteins embedded in the NE and PM were found to diffuse considerably more slowly than proteins in the ER and Golgi apparatus. This is likely due to constraints from interactions with nearby structural components (e.g., cytoskeleton, extracellular matrix, or nuclear matrix).

Lamin B receptor-GFP fusion protein (LBR-GFP) exhibits differential mobility when localized in the ER versus the NE. The left panel shows photobleach recovery within ER membranes, and the right panel shows photobleach recovery within the NE. Bars, 10 μ m (adapted from ( 22 )).
As indicated above, the dynamics of GFP-tagged proteins measured by confocal FRAP repeatedly challenged widely held assumptions about the static distribution of intracellular proteins in membranes, but inducing researchers to fundamentally rethink their long-held theories of cellular dynamics was often difficult. Indeed, many of the new findings derived from FRAP were initially outright dismissed or ignored or gave rise to heated debates at conferences. With time, however, as more studies confirmed the rapid diffusion of proteins in membranes and also, as discussed next, showed rapid diffusion of proteins in the cytoplasm and nucleus, protein dynamism in cells became widely accepted.
FRAP studies examining protein diffusion in the cytoplasm and nucleus revealed that although many nuclear and cytoplasmic proteins diffuse freely, their diffusion rates were often slower than what free diffusion predicts in water ( 42 ). This retardation compared to pure diffusion most likely arises from reversible binding of the proteins to large scaffolds like chromatin, actin, or microtubules ( 43 ). For nuclear proteins such as the transcription factor NF κ B (i.e., Dorsal in flies), FRAP studies revealed that rather than being retained in the nucleus, the protein continuously shuttles between the nucleus and cytoplasm ( 44 ). Together, these FRAP findings opened up new directions of research focused on delineating the various mechanisms involved in protein shuttling between nucleus and cytoplasm, as well as those involved in sorting and retention of proteins in different subcellular compartments.
FRAP variations to measure organelle connectivity and membrane trafficking pathways
Various technical modifications expanded FRAP’s reach for investigating the kinetics of biological systems. One powerful variant is fluorescence loss in photobleaching (FLIP) ( Fig. 3 ), which involves repeated photobleaching of one subregion while collecting images of the entire cell ( 19 ). In FLIP, if the photobleached ROI through which the reporter translates is either connected to other cell domains or the fluorescent reporter can translocate between domains, continuous fluorescence loss is observed because over time, the fluorescently labeled proteins throughout the system pass through the photobleaching ROI. Similarly, if a membrane-bound organelle containing a GFP-tagged protein translocates between the photobleached ROI and nonbleached areas, fluorescence loss is observed. Analysis of the process of fluorescence loss can reveal the translocation speed of an organelle or its transport intermediates ( 32 ). Among the findings using FLIP were that the elaborate tubular system of the ER is continuous throughout the cell ( 19 ) and that during mitosis, both the Golgi apparatus and NE membrane proteins redistribute into the continuous membranes of the ER ( 22 , 28 , 45 ).
Another FRAP variation, termed inverse FRAP (iFRAP), involves optical highlighting of an ROI by photobleaching the areas surrounding it ( 46 ). Photoactivating molecules in an ROI and following their dispersion/fate accomplishes the same experimental goal ( 47 ) and has been used interchangeably with iFRAP. iFRAP and photoactivation methods have been particularly valuable for studying membrane trafficking pathways for protein transport between discrete organelles. The techniques have revealed, for example, the speed and directionality of secretory transport carriers moving from the Golgi apparatus to the PM ( 46 , 48 ) and the cycling rates for trafficking intermediates carrying proteins moving between the ER-Golgi and Golgi-PM domains ( 29 , 45 , 46 ). In a recent study, both iFRAP and photoactivation methods established that nuclear-localized LC3 moves into the cytoplasm and binds to autophagosomes in starved cells ( 49 ) ( Fig. 4 ). In contrast, cytoplasmic pools of LC3 did not bind to autophagosomes. LC3 only bound autophagosomes if the protein was first deacetylated, which occurs in the nucleus catalyzed by nuclear-localized deacetylases.

iFRAP and photoactivation experiments reveal that a nuclear, not cytoplasmic, pool of LC3 redistributes onto newly formed autophagosomes under starvation conditions. In ( A ), note that GFP-LC3 puncta (representing autophagosomes) can form upon photobleaching the cytoplasm, whereas after photobleaching the nucleus, no GFP-LC3 puncta are seen. ( B ) A similar result is obtained using photoactivation to specifically highlight either the nuclear or cytoplasmic pool of PAGFP-LC3 (from ( 49 )).
Enhancing FRAP’s scope through computational modeling
Most macromolecules in cells do more than simply diffuse. Macromolecules also bind transiently or long-term to moving or immobile substrates. FRAP recovery measurements offer the opportunity to characterize these mobility characteristics with kinetic analysis tools. For example, it is possible to determine whether an observed recovery curve arises from random diffusion, directed motion, binding events, or some combination of these ( 5 , 6 , 50 , 51 ). To distinguish among these possibilities, the researcher first generates distinct hypotheses about the various biophysical parameters for the molecule of interest (e.g., its binding and release constants, probable residence time for binding to a substrate, and diffusion coefficient). These parameters are then incorporated into a mathematical model describing the behavior of the protein that is simulated and tested against the actual recovery data. The model is supported if the simulated data fit the recovery data. If the simulation does not fit it, additional hypotheses and mathematical simulations are used to find ones that do. The model can then be tested in further experiments to assess its overall ability to account for different classes of experimental data.
Results from this type of computational modeling have revealed unexpected dynamic properties of many subcellular structures previously assumed to be stable. For example, protein assemblies comprising the nucleolus and other nuclear subcompartments have been found to consist primarily of dynamic components undergoing continuous and rapid association/dissociation ( 52 , 53 , 54 ). Kinetic modeling approaches revealed the on/off rate constant for a specific component’s subcompartment association and the component’s residency time there ( 55 ). Another study examined binding of cytosolic “coat” proteins to membranes during membrane trafficking (including COPI, clathrin, Arf1, ArfGAP1, GBF1, and Sec13). These coat proteins play an important role in shaping a secretory vesicle as the vesicle buds off a target membrane. The coat proteins were previously thought to stably associate with the vesicle membrane until the vesicle released from the target membrane. Selective photobleaching of these coat proteins when bound to their originating compartment instead revealed that the coat proteins all undergo fast continuous cytosol/membrane exchange irrespective of vesicle budding ( 56 , 57 , 58 , 59 , 60 ). This finding established that budding was a downstream event of multiple binding/release events by these proteins and not directly coupled to coat dissociation. Kinetic modeling of the observed dynamics established specific rates of binding and release for each type of coat protein ( 57 , 59 ). These studies enabled the development of testable predictive models for their coordinated activities with other transport machinery.
Uncertainties and challenges in analyzing FRAP experiments
There are several possible sources of uncertainty in extracting physicochemical parameters of molecular mobility measurements in FRAP experiments ( 5 , 61 ). If not addressed, these uncertainties can lead to incorrect estimates for diffusion coefficients and binding/dissociation constants. For example, clarifying the geometry of the membrane (e.g., a flat plane or three-dimensional meshwork) is critical for accurate determination of a protein’s diffusion coefficient within a membrane ( 62 ). Another challenge arises when using the wide array of fluorescent proteins that have been developed. Not all fluorescent proteins irreversibly photobleach, and such behavior could lead to spurious results. When establishing an FRAP protocol, it is critical for the investigator to establish conditions that achieve adequate bleach depth and confirm that bleaching is irreversible during the time course and conditions of the experiment ( 63 ). In addition, photobleaching cannot be assumed to be instantaneous, especially when monitoring a rapidly diffusing molecule. Molecular translation does not stop while an ROI is photobleached. Indeed, if photobleaching is slow relative to a fast-diffusing protein, then unbleached molecules will enter the ROI from the rims and become bleached, forming a “corona” of bleached particles around the ROI similar to an FLIP experiment ( 64 ). The corona leads to an underestimate of the diffusion coefficient. To overcome this problem, additional FRAP measurements gathered with different bleach iterations can be made, and the “corona” effect can be corrected through computer modeling simulations ( 64 , 65 ).
Another source of uncertainty arises from exceptionally transient interactions that are nonuniformly distributed. Environments where this occurs include the nucleus and other highly crowded compartments in the cell. In such environments, FRAP recoveries can be retarded and represent a combination of both diffusion and binding reactions, requiring solutions to the complex kinetics of reaction-diffusion systems. Using simulations that incorporate features of reaction-diffusion systems, researchers have successfully modeled FRAP recoveries of several types of chromatin binding proteins in the nucleus ( 66 ). The results revealed that diffusion can limit the fluorescence redistribution of proteins in cases in which the distribution of binding sites is inhomogeneous and the binding time is very transient. The tools from this study provide a general analytical framework for analyzing the contribution of diffusion and interaction to fluorescence redistributions for any compartment in the cell.
Using FRAP for mechanistic analysis of non-steady-state systems
Most methods for extraction of quantitative information from FRAP data use the mathematics of diffusion as well as tracer kinetics. Classical tracer kinetic methods assume that all molecular concentrations and fluxes are constant for the duration of the experiment—i.e., the system being studied is in a steady state. This assumption has generally been applied, either implicitly or explicitly, in most photobleaching (FRAP, FLIP) and photoactivation experiments that use GFP as a tracer with no other perturbation than turning the tracer off or on by photobleaching and photoactivation. However, when the experiment involves perturbations of the steady state by the addition of drugs, growth factors, change of pH, or other modifications to activate or inhibit processes of interest, alternative methods for simulating and analyzing the data are needed.
One approach ( 67 ) that explicitly accounts for non-steady states while retaining the power of GFP tracer kinetics (including FRAP and photoactivation) is based on the derivation of one set of differential equations for the non-steady-state biological system and a separate set of differential equations for each fluorescent tracer. Importantly, these two systems of equations are linked through a straightforward application of the basic indistinguishability postulate that is the foundation of all tracer kinetic applications. This approach of linking two systems has been applied to the analysis of non-steady-state tracer experiments at levels of biological organization from single cells ( 7 , 57 ) to human metabolic physiology ( 68 ) and pharmaceutical development ( 69 ).
The above “linking” approach differs from classic methods of estimating fluxes in non-steady states, which employ additional tracers of the same molecule to supply the required information ( 70 ) or employ hybrid algebraic and stochastic methods ( 71 ). These classic methods consider only tracer dynamics and do not directly account for the impact of the underlying nonlinear dynamics in non-steady state experiments. The linking approach extends differential-equation-based methods to non-steady-state systems so that it can be applied to any GFP-reporters used as tracers ( 67 ).
An advantage of the linking approach is that all aspects of the experiment can be modeled from the tracer distribution phase to the photobleaching and recovery phases, as well as concentration data acquired by biochemical methods. Importantly, this approach automatically reduces to classical linear tracer kinetics whenever the biological system returns to a steady state.
With the transition from the mathematics of diffusion to the mathematics of tracer kinetics and the extension of tracer kinetics to non-steady-state situations, it has become possible to test fully mechanistic hypotheses against GFP tracer dynamics and chemical dynamics simultaneously.
The history of FRAP methods and analysis thus exemplifies the power of convergence in science; collaborative innovations since Axelrod et al.’s original breakthroughs have yielded a modern FRAP technology that is now indispensable for appreciating and analyzing the dynamic properties of life at the cellular level. Although many long-standing conundrums revealed by FRAP still remain to be addressed, answers may come through the ability of FRAP measurements to be integrated into complex models of cell dynamics, including membrane trafficking pathways and regulatory feedback mechanisms invoked by non-steady-state experimental perturbations.
Editor: Julie Biteen.

- Lab Basics- , Science
Fluorescence recovery after photobleaching (FRAP)
As an Amazon Associate Conductscience Inc earns revenue from qualifying purchases
Introduction
Fluorescence recovery after photobleaching (FRAP) is an imaging technique for the investigation of molecular dynamics and cellular events within living cells. The FRAP elucidates the dynamics and kinetics of the protein-protein interaction and cellular signaling. In a FRAP experiment, the laser beam is focused on a small area of cell membrane labeled with a fluorescent probe, and then the fluorescence intensity excited by the beam is observed as a function of time. For a moment, the optical attenuator is removed to bleach some of the fluorescent probe molecules in the region of interest. This reduces the fluorescence intensity by the bleaching pulse, but the intensity recovers through the diffusion of unbleached molecules from the surrounding. FRAP studies yield qualitative data that provides with an insight into the binding characteristics, relative binding affinity, and the effects of pharmaceutical agents or mutations on protein mobility. Improvements in fluorophores and labeling techniques, and the identification of fluorescent proteins such as green fluorescent protein (GFP), have further advanced the use of FRAP in biomedical science ( Carisey., et al., 2011 ).
The underlying principle of the fluorescence recovery after photobleaching involves the discontinuation of fluorescence caused by the conversion of the fluorophore to a chemically non-fluorescent compound. Photobleaching needs an incident light and molecular oxygen for most fluorophores. Initially, a series of fluorescence intensity images are collected to record the value for intensity in both the region of interest and the surrounding. Following this, a small area of interest is illuminated with high-intensity light bleaching the fluorophore within that region to create a darker, bleached region in the sample. Photobleached molecules are replaced with nonbleached molecules, diffused from the surrounding, increasing in the fluorescence intensity of the bleached region. FRAP is a quantitative fluorescence technique that is used to measure the dynamics of molecular mobility two-dimensionally by utilizing the irreversibly bleached fluorophores ( Bizzarri et al., 2012 ).
The fluorescence recovery after photobleaching (FRAP) equipment consists of a fluorescence microscope equipped with light sources, filter sets, the photobleaching light source, and fluorescent probes. The fluorescence emission is dependent on the absorption of a specific wavelength restricting the choice of lamps. For this, broad-spectrum mercury or xenon source with a color filter is used. A background image of the sample is captured before photobleaching. Then, the light source is focused onto a small area with laser light of the appropriate wavelength. The fluorescent molecules in this region receive high-intensity illumination which causes their fluorescence lifetime to elapse quickly. This makes the background image of a uniformly fluorescent field with a prominent dark spot. As the diffusion proceeds, the fluorescing probes from the surroundings will diffuse throughout the sample and replace the non-fluorescent probes in the bleached region ( Lopez et al., 1988 ).
Cell preparation and transfection
- Prepare the mEGFP fusion proteins using the standard procedures.
- Transfect the cells with the fusion protein construct in a six-well plate using Lipofectamine Plus reagent .
- Coat the dishes with 10 mg/ml fibronectin for 1 hour at room temperature.
- After 4 hours of incubation, wash the cells with sterile phosphate buffer solution for two times.
- Trypsinize the cells and rinse them twice with Dulbecco’s modified Eagle’s medium (DMEM) supplemented with fetal calf serum (FCS).
- Plate the cells (5 × 104 cells/dish) in the glass bottom dish.
- Incubate the cells for 12-48 hours until the expression of the transfected plasmid reaches its maximum.
- Replace the Dulbecco’s modified Eagle’s medium with pre-warmed Ham’s F12 medium .
Data acquisition
- Select the cells expressing the fluorescent protein in the region of interest using the eyepiece/objective of the microscope.
- Adjust the microscope parameters for monitoring the fluorescence.
- Adjust the focus and select the required desired region of interest.
- Start the time-lapse.
- Proceed with another cell and acquire multiple cells.
Intensity measurements
- Transfer the collected images of the FRAP experiment to the analysis workstation.
- Re-adjust the alignment of the images to avoid any stage drift.
- Draw the boundaries of the region of interest using the circular tool.
- Obtain the fluorescence intensity values for:
- The bleached region of interest.
- Unbleached areas showing the background fluorescence.
- Unbleached structures similar to the bleached target.
- Label the data and store it for the experimental record.

Protocol for FRAP of dendritic spines in cultured hippocampal neurons
Neuronal transfection
- Culture the hippocampal neurons of rat (embryonic day 18) on poly-d-lysine -coated 35-mm glass-bottom dishes. On 16-18 days in vitro, transfect the neurons using the transfection Kit. Replace the original culture medium with 1.5 ml of Dulbecco’s Modified Eagle Medium (DMEM) half an hour before the transfection.
- Mix 10 μg of enhanced green fluorescent protein (pEGFP-N1) plasmid DNA with sterile water and 12.4 μl of 2M calcium solution to make a total volume of 100 μl.
- Add 100 μl 2×HBS dropwise to the mixture while vortexing 2×HBS at medium speed.
- Leave the mixture at room temperature and let it sit for 20 minutes.
- Add the final mixture into the DMEM -incubated neurons.
- Incubate the neurons at 37 °C for 1-1.5 hours.
- Remove the calcium phosphate-containing medium and wash the cells with DMEM for three times.
- Replace the DMEM medium with the culture medium.
Spine FRAP experiment
- After 2-4 days of transfection, replace the culture medium with pre-warmed Tyrode Solution containing NaCl 145, KCl 5, HEPES 10, Glucose 10, Glycine 0.005, CaCl2 2.6, and MgCl2 1.3.
- Find the transfected mature dendrite by adjusting the eyepiece of the microscope.
- Select the region of interest and capture the images every 1 second for 15 seconds after bleaching. Save the captured images for analysis.
Applications
Visualizing active transport across the cell membrane ( zheng., et al., 2011 ).
Fluorescence recovery after photobleaching (FRAP) is a widely used imaging technique to study binding and diffusion of biomolecules in cells. The study was conducted to estimate diffusion, binding/unbinding rates, and active transport velocities using the FRAP data by capturing intracellular dynamics. The transport and localization of mRNA molecules in Xenopus laevis oocytes were observed. The results suggested that the RNA movement in both the animal and vegetal directions could impact the duration and the time of RNA localization in Xenopus oocytes. It was also presented that the initial model conditions extracted from FRAP postbleach intensities prevent the underestimation of diffusion, which could arise from instantaneous bleaching. The FRAP technique is a broadly applicable tool to analyze the systems where intracellular transport is a critical molecular mechanism.
Demonstrating the assembly dynamics of E.coli FtsZ-ring ( Stricker et al., 2002 )
FtsZ is an important cytoskeletal component of the bacterial cell division machinery. It assembles into a ring called the Z-ring which contracts at septation. FtsZ is involved in guanosine triphosphate (GTP) hydrolysis and in vitro assembly. In the FRAP experiment, green fluorescent protein-labeled FtsZ was used to show the dynamics of E. coli Z-ring which continually remodels itself with a half-time of 30 s. It was found that the ZipA, a membrane protein involved in cell division, is also dynamic. The Z-ring of the mutant ftsZ84 showed a 9-fold slower turnover in vivo, which in the wild-type has 1/10 the guanosine triphosphatase activity in vitro. The study indicated that the assembly dynamics could be determined by GTP hydrolysis. The FRAP technique was found to be a powerful imaging tool to observe the dynamics of membrane proteins in bacterial species.
Detection of the lateral mobility in membranes ( Yguerabide., Schmidt., & Yguerabide., 1982 )
The fluorescence recovery after photobleaching (FRAP) can demonstrate the lateral diffusion coefficients of membrane components. The method was developed for precise analysis of FRAP data to minimize uncertainties arising from the photobleaching in the experiment. For this, thin, multi-bilayer films containing a fluorescent probe were used with a molar-lipid-to-probe ratio of 500:1. The thin, clear multi-bilayer film was hydrated using the fully hydrated agarose gel, and the cover glass was sealed onto a microscope slide for the observation of FRAP experiment. The results indicated that the recovery of fluorescence could be represented over a broad range of percent bleach and recovery time. It was also shown that the linear reciprocal plot provides the researchers with a simple method to detect flow or multiple diffusion coefficients and to establish different parameters such as data precision, differences in multiple diffusion coefficients, the magnitude of flow rate compared to lateral diffusion. It was concluded that the FRAP method is applicable to obtain data from biological cells, tissues, and living systems when the optics are properly aligned, and the cellular movement is masked.
Analyzing the thylakoid membrane proteins ( Rayan et al., 2010 )
The diffusion of grana thylakoid membrane chlorophyll-protein complexes was observed using the fluorescence recovery after photobleaching (FRAP). The samples were obtained from isolated spinach ( Spinacia oleracea ) grana membranes. Grana are the clusters of thylakoid membrane domains and are protein hubs with around 70% to 80% of the membrane occupied by proteins. It was found that around 75% of chlorophyll-protein complexes remain immobile up to 9 minutes, while the remaining proteins diffused rapidly after photobleaching. These “rapidly” diffusing proteins were the mobile proteins, which exchange between the grana and stroma lamellae. The FRAP technique is a promising imaging technique for the observation of protein diffusion across the cell membrane.
Precautions
- The cells should be properly aligned before the experiment because living cells often move during the experiment.
- Bleaching event reduces the total amount of excitable fluorochromes in the cell, so it is recommended to measure a control region to have background data for the overall loss in fluorescence.
- In some experiments the final FRAP result is determined by the size of the region of interest; therefore, it is important to include a control to avoid this.
- It is essential to check the recovery rate for different bleaching intensities because photo-induced cross-linking may occur.
- If the study involves living cells then the removal of oxygen cannot be done; therefore there is a need to carefully control the dose of light received by the sample in fluorescence imaging to avoid bleaching.
- If long-term experiments are planned, then it is advisable to use Photoactivatable GFP because proteins may degrade or denature at extended periods.
Strengths and limitations
- Fluorescence recovery after photobleaching (FRAP) is a standard method that unveils subtle dynamic and biochemical properties of intracellular components and cellular events.
- The FRAP imaging provides valuable information about the diffusion and mobility of cellular protein population.
- The photobleaching effect of the FRAP provides with high fluorescence signal enabling the detection of strongly associated components.
- FRAP allows the analyses of binding dynamics of the protein molecules.
- The data obtained from the FRAP studies are qualitative and provides an insight into the binding characteristics, binding affinity, and the effect of drugs or mutations on protein mobility and diffusion.
- , M. Stroud., R. Tsang., & Ballestrem., C. (2011). Fluorescence recovery after photobleaching. Methods Mol Biol, 769, 387-402.
- , L. Dupou., A. Altibelli., J. Trotard., & Tocanne., J. F. (1988). Fluorescence recovery after photobleaching (FRAP) experiments under conditions of uniform disk illumination . Biophys J, 53(6), 963-70.
- , R. S. Petralia., Y. Wang., & Kachar., B. (2011). Fluorescence Recovery After Photobleaching (FRAP) of Fluorescence Tagged Proteins in Dendritic Spines of Cultured Hippocampal Neurons. J Vis Exp, 50, 2568.
- Rayan., J. Guet., N. Taulier., F. Pincet., & Urbach., W. (2010). Recent applications of fluorescence recovery after photobleaching (FRAP) to membrane bio-macromolecules. Sensors (Basel), 10(6), 5927-48.
- J Yguerabide., J. A. Schmidt., & Yguerabide., E. E. (1982). Lateral mobility in membranes as detected by fluorescence recovery after photobleaching. Biophys J, 40(1), 69-75.
- Stricker., P. Maddox., E. D. Salmon., & Erickson., H. P. (2002). Rapid assembly dynamics of the Escherichia coli FtsZ-ring demonstrated by fluorescence recovery after photobleaching . Proc Natl Acad Sci U S A, 99(5), 3171-5.
- Bizzarri., F. Cardarelli., M. Serresi., & Beltram., F. (2012). Fluorescence recovery after photobleaching reveals the biochemistry of nucleocytoplasmic exchange . Anal Bioanal Chem, 403(8), 2339-51.
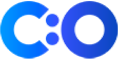
Our Location
- 5250 Old Orchard Rd Suite 300 Skokie, IL 60077
- +1 847 983 3672
Monday – Friday 9 AM – 5 PM EST
Conduct Science
- Become a Partner
- Social Media
- Career/Academia
- Privacy Policy
- Shipping & Returns
- Request a quote
Customer service
- Account Details
- Lost Password
DISCLAIMER: ConductScience and affiliate products are NOT designed for human consumption, testing, or clinical utilization. They are designed for pre-clinical utilization only. Customers purchasing apparatus for the purposes of scientific research or veterinary care affirm adherence to applicable regulatory bodies for the country in which their research or care is conducted.
Fluorescence recovery after photobleaching: direct measurement of diffusion anisotropy
- Original Paper
- Published: 19 June 2020
- Volume 19 , pages 2397–2412, ( 2020 )
Cite this article
- Kotaybah Hashlamoun 1 , 2 , 3 ,
- Ziad Abusara 2 nAff5 ,
- Ariel Ramírez-Torres 4 ,
- Alfio Grillo 4 ,
- Walter Herzog 1 , 2 &
- Salvatore Federico ORCID: orcid.org/0000-0003-0866-1121 1 , 2
1056 Accesses
6 Citations
Explore all metrics
Fluorescence recovery after photobleaching (FRAP) is a widely used technique for studying diffusion in biological tissues. Most of the existing approaches for the analysis of FRAP experiments assume isotropic diffusion, while only a few account for anisotropic diffusion. In fibrous tissues, such as articular cartilage, tendons and ligaments, diffusion, the main mechanism for molecular transport, is anisotropic and depends on the fibre alignment. In this work, we solve the general diffusion equation governing a FRAP test, assuming an anisotropic diffusivity tensor and using a general initial condition for the case of an elliptical (thereby including the case of a circular) bleaching profile. We introduce a closed-form solution in the spatial coordinates, which can be applied directly to FRAP tests to extract the diffusivity tensor. We validate the approach by measuring the diffusivity tensor of \(3~\mathrm {kDa}\) FITC-Dextran in porcine medial collateral ligaments. The measured diffusion anisotropy was \(1.42 \pm 0.015\) (SE), which is in agreement with that reported in the literature. The limitations of the approach, such as the size of the bleached region and the intensity of the bleaching, are studied using COMSOL simulations.
This is a preview of subscription content, log in via an institution to check access.
Access this article
Subscribe and save.
- Get 10 units per month
- Download Article/Chapter or eBook
- 1 Unit = 1 Article or 1 Chapter
- Cancel anytime
Price includes VAT (Russian Federation)
Instant access to the full article PDF.
Rent this article via DeepDyve
Institutional subscriptions
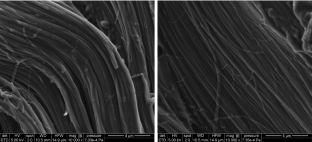
Similar content being viewed by others
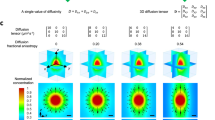
A noninvasive fluorescence imaging-based platform measures 3D anisotropic extracellular diffusion
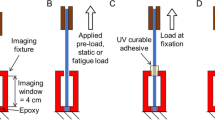
Assessment of Mechanically Induced Changes in Helical Fiber Microstructure Using Diffusion Tensor Imaging
A validated software application to measure fiber organization in soft tissue.
Axelrod D, Koppel D, Schlessinger J, Elson E, Webb WW (1976) Mobility measurement by analysis of fluorescence photobleaching recovery kinetics. Biophys J 16(9):1055–1069
Article Google Scholar
Brezis H (2010) Functional analysis. Sobolev spaces and partial differential equations. Springer, New York
Book Google Scholar
Brinkman H (1949) A calculation of the viscous force exerted by a flowing fluid on a dense swarm of particles. Appl Sci Res 1(1):27–34
Burton-Wurster N, Lust G (1990) Fibronectin and proteoglycan synthesis in long term cultures of cartilage explants in Ham’s F12 supplemented with insulin and calcium: effects of the addition of TGF- \(\beta\) . Arch Biochem Biophys 283(1):27–33
Curnier A, He Q-C, Zysset P (1995) Conewise linear elastic materials. J Elast 37:1–38
Article MathSciNet Google Scholar
DiDomenico CD, Lintz M, Bonassar LJ (2018) Molecular transport in articular cartilage—what have we learned from the past 50 years? Nat Rev Rheumatol 14(7):393–403
Greene GW, Zappone B, Zhao B, Söderman O, Topgaard D, Rata G, Israelachvili JN (2008) Changes in pore morphology and fluid transport in compressed articular cartilage and the implications for joint lubrication. Biomaterials 29(33):4455–4462
Jönsson P, Jonsson MP, Tegenfeldt JO, Höök F (2008) A method improving the accuracy of fluorescence recovery after photobleaching analysis. Biophys J 95(11):5334–5348
Kang M, Day CA, DiBenedetto E, Kenworthy AK (2010) A quantitative approach to analyze binding diffusion kinetics by confocal frap. Biophys J 99(9):2737–2747
Kang M, Day CA, Kenworthy AK, DiBenedetto E (2012) Simplified equation to extract diffusion coefficients from confocal frap data. Traffic 13(12):1589–1600
Le Bihan D, Mangin J-F, Poupon C, Clark CA, Pappata S, Molko N, Chabriat H (2001) Diffusion tensor imaging: concepts and applications. J Magn Reson Imaging 13(4):534–546
Leddy HA, Guilak F (2003) Site-specific molecular diffusion in articular cartilage measured using fluorescence recovery after photobleaching. Ann Biomed Eng 31(7):753–760
Leddy HA, Haider MA, Guilak F (2006) Diffusional anisotropy in collagenous tissues: fluorescence imaging of continuous point photobleaching. Biophys J 91(1):311–316
Lee JI, Sato M, Ushida K, Mochida J (2011) Measurement of diffusion in articular cartilage using fluorescence correlation spectroscopy. BMC Biotechnol 11(1):19
Lori N, Akbudak E, Shimony J, Cull T, Snyder A, Guillory R, Conturo T (2002) Diffusion tensor fiber tracking of human brain connectivity: aquisition methods, reliability analysis and biological results. NMR Biomed 15(7–8):494–515
Maroudas A (1968) Physicochemical properties of cartilage in the light of ion exchange theory. Biophys J 8(5):575–595
Mazza D, Braeckmans K, Cella F, Testa I, Vercauteren D, Demeester J, De Smedt SS, Diaspro A (2008) A new FRAP/FRAPa method for three-dimensional diffusion measurements based on multiphoton excitation microscopy. Biophys J 95(7):3457–3469
Qian H, Sheetz MP, Elson EL (1991) Single particle tracking. Analysis of diffusion and flow in two-dimensional systems. Biophys J 60(40):910–921
Shi C, Cisewski SE, Bell PD, Yao H (2014) Measurement of three-dimensional anisotropic diffusion by multiphoton fluorescence recovery after photobleaching. Ann Biomed Eng 42(3):555
Shi C, Kuo J, Bell PD, Yao H (2010) Anisotropic solute diffusion tensor in porcine TMJ discs measured by frap with spatial fourier analysis. Ann Biomed Eng 38(11):3398–3408
Sprague BL, Pego RL, Stavreva DA, McNally JG (2004) Analysis of binding reactions by fluorescence recovery after photobleaching. Biophys J 86(6):3473–3495
Verkman AS (2002) Solute and macromolecule diffusion in cellular aqueous compartments. Trends Biochem Sci 27(1):27–33
Xia Y, Farquhar T, Burton-Wurster N, Ray E, Jelinski LW (1994) Diffusion and relaxation mapping of cartilage-bone plugs and excised disks using microscopic magnetic resonance imaging. Magn Reson Med 31(3):273–282
Xia Y, Farquhar T, Burton-Wurster N, Vernier-Singer M, Lust G, Jelinski L (1995) Self-diffusion monitors degraded cartilage. Arch Biochem Biophys 323(2):323–328
Download references
Acknowledgements
The Authors gratefully acknowledge the help of Ruth Seerattan for the help with sample preparation and of Elyar Asl Sabbaghian Hokm Abadi for useful discussions. This work was supported in part by the Natural Sciences and Engineering Research Council of Canada, through the NSERC Discovery Programme [SF, WH], the Canadian Institutes of Health Research (CIHR) [WH], the Canada Research Chair Programme [WH], the Killam Foundation [WH], Dipartimento di Eccellenza 2018–2022, Politecnico di Torino, Project No. E11G18000350001 [AG, ART], the Biomedical Engineering Graduate Programme of the University of Calgary (Canada), through the BME GP Academic Award [KH] and the BME Research Scholarship Award [KH], the University of Calgary Eyes High Doctoral Programme [KH] and the Canadian Society for Biomechanics, through the CSB PhD Student Travel Grant [KH]. Part of this work was conducted during the visit of KH at the Department of Mathematical Sciences (DISMA) “G.L. Lagrange”, Politecnico di Torino, under the supervision of AG and with the support of the CSB PhD Student Travel Award.
Author information
Ziad Abusara
Present address: Advanced Imaging and Histopathology Core, Qatar Biomedical Research Institute, Hamad Bin Khalifa University, Qatar Foundation, P.O. Box 34110, Doha, Qatar
Authors and Affiliations
Department of Mechanical and Manufacturing Engineering, The University of Calgary, Calgary, Canada
Kotaybah Hashlamoun, Walter Herzog & Salvatore Federico
Human Performance Laboratory, The University of Calgary, Calgary, Canada
Kotaybah Hashlamoun, Ziad Abusara, Walter Herzog & Salvatore Federico
Graduate Programme in Biomedical Engineering, The University of Calgary, Calgary, Canada
Kotaybah Hashlamoun
Department of Mathematical Sciences “G.L. Lagrange”, ‘Dipartimento di Eccellenza 2018-2022’, Politecnico di Torino, Torino, Italy
Ariel Ramírez-Torres & Alfio Grillo
You can also search for this author in PubMed Google Scholar
Corresponding author
Correspondence to Salvatore Federico .
Ethics declarations
Conflict of interest.
The authors declare that they have no competing interests.
Rights and permissions
Reprints and permissions
About this article
Hashlamoun, K., Abusara, Z., Ramírez-Torres, A. et al. Fluorescence recovery after photobleaching: direct measurement of diffusion anisotropy. Biomech Model Mechanobiol 19 , 2397–2412 (2020). https://doi.org/10.1007/s10237-020-01346-z
Download citation
Received : 29 October 2019
Accepted : 12 May 2020
Published : 19 June 2020
Issue Date : December 2020
DOI : https://doi.org/10.1007/s10237-020-01346-z
Share this article
Anyone you share the following link with will be able to read this content:
Sorry, a shareable link is not currently available for this article.
Provided by the Springer Nature SharedIt content-sharing initiative
- Anisotropic diffusion
- Fibrous tissues
- Direct measure
- Find a journal
- Publish with us
- Track your research
Thank you for visiting nature.com. You are using a browser version with limited support for CSS. To obtain the best experience, we recommend you use a more up to date browser (or turn off compatibility mode in Internet Explorer). In the meantime, to ensure continued support, we are displaying the site without styles and JavaScript.
- View all journals
- Explore content
- About the journal
- Publish with us
- Sign up for alerts
- Open access
- Published: 20 April 2018
Quantitative diffusion measurements using the open-source software PyFRAP
- Alexander Bläßle 1 ,
- Gary Soh 1 ,
- Theresa Braun 1 nAff3 ,
- David Mörsdorf 1 ,
- Hannes Preiß 1 ,
- Ben M. Jordan 2 &
- Patrick Müller 1
Nature Communications volume 9 , Article number: 1582 ( 2018 ) Cite this article
7187 Accesses
27 Citations
53 Altmetric
Metrics details
- Biophysical methods
- Computational biology and bioinformatics
- Developmental biology
Fluorescence Recovery After Photobleaching (FRAP) and inverse FRAP (iFRAP) assays can be used to assess the mobility of fluorescent molecules. These assays measure diffusion by monitoring the return of fluorescence in bleached regions (FRAP), or the dissipation of fluorescence from photoconverted regions (iFRAP). However, current FRAP/iFRAP analysis methods suffer from simplified assumptions about sample geometry, bleaching/photoconversion inhomogeneities, and the underlying reaction-diffusion kinetics. To address these shortcomings, we developed the software PyFRAP, which fits numerical simulations of three-dimensional models to FRAP/iFRAP data and accounts for bleaching/photoconversion inhomogeneities. Using PyFRAP we determined the diffusivities of fluorescent molecules spanning two orders of magnitude in molecular weight. We measured the tortuous effects that cell-like obstacles exert on effective diffusivity and show that reaction kinetics can be accounted for by model selection. These applications demonstrate the utility of PyFRAP, which can be widely adapted as a new extensible standard for FRAP analysis.
Similar content being viewed by others
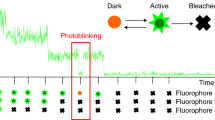
Diffraction-limited molecular cluster quantification with Bayesian nonparametrics
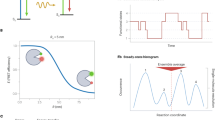
Fluorescence resonance energy transfer at the single-molecule level
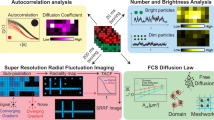
Simultaneous spatiotemporal super-resolution and multi-parametric fluorescence microscopy
Introduction.
The diffusion of molecules is important for almost any process across all scales of biological organisation, from transcription factors finding their targets on DNA to signalling molecules spreading through tissues during development and homoeostasis 1 , 2 , 3 . The biological function of a molecule is affected by its action range and therefore its mobility; however, effective diffusion of molecules moving through complex tissues is difficult to measure quantitatively. More than 40 years ago, Poo & Cone 4 and Liebman & Entine 5 developed a method to assess the diffusivities of fluorescent molecules. In these fluorescence recovery after photobleaching (FRAP) experiments, the fluorescence of molecules in a small region of the sample is bleached by exposure to a strong laser pulse 6 . The dynamics of fluorescence recovery in the bleached region can then be used to infer the mobility of the fluorescent molecules (Fig. 1 a). Inverted FRAP (iFRAP) assays have recently been developed as an extension of FRAP experiments 7 , 8 , 9 , 10 , which eliminate the often harsh bleaching conditions used in FRAP experiments. iFRAP assays utilise photoconvertible molecules that can be induced to alter their fluorescence excitation/emission properties after exposure to ‘photoconverting’ light. In iFRAP experiments, the spread of signal from a small photoconverted domain into the neighbouring regions of the sample is monitored over time and thus represents an experimental mirror image of FRAP (Fig. 1 b).
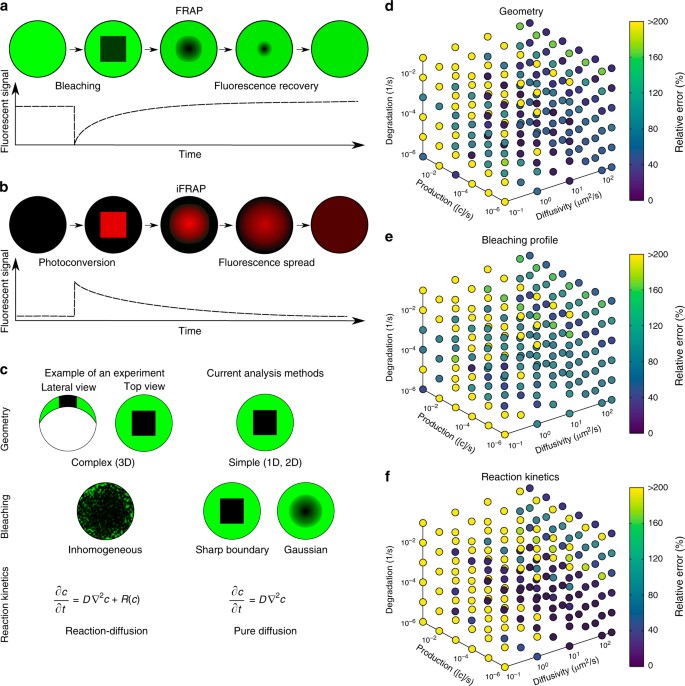
Fluorescence perturbation techniques used for effective diffusion measurements, and drawbacks of current analysis methods. a In fluorecence recovery after photobleaching (FRAP) experiments, a small region in the sample is bleached. After bleaching, the diffusion-driven recovery in the bleached region is monitored. b Inverse FRAP (iFRAP) is an experimental mirror image of FRAP: Molecules in a given region are photoconverted and then spread throughout the sample, resulting in the loss of fluorescent signal in the region of photoconversion. c Drawbacks of current analysis methods exemplified with zebrafish development at late blastula stages. Current analysis methods simplify sample geometry, idealise bleaching profiles, or ignore underlying reaction kinetics. d – f Possible relative error in diffusion coefficient estimates that can occur if false assumptions are made about sample geometry ( d ), bleaching conditions ( e ), or reaction kinetics ( f ), respectively. The maximum displayed error was capped to a value of 200%, but can be up to 1000%
Diffusion coefficients are commonly extracted from FRAP experiments by fitting analytical solutions computed from theoretical models to the measured recovery curves 11 , 12 , 13 , 14 , 15 , 16 , 17 , 18 , and a few simulation-based analysis methods have been developed 19 , 20 , 21 . Although this allows for a rapid assessment of qualitative mobility differences in identical experimental settings, current approaches rely on several assumptions that can affect the accuracy of the analysis. First, most current methods reduce the FRAP analysis to one-dimensional or two-dimensional simplifications 11 , 12 , 13 , 14 , 15 , 16 , 17 , 18 , 19 , 20 , 21 , often assuming that the fluorescent pool is infinitely large 11 , 12 , 13 , 14 , 16 , 17 , or ignoring more complex geometries of biological samples that could play important roles in molecule movement (Fig. 1c ). Recent studies have argued that geometry is crucial for dynamic biological processes 22 , 23 , and must be taken into account for accurate analysis of FRAP data. Indeed, false assumptions about the FRAP sample geometry can drastically affect diffusion coefficient estimates (Fig. 1d ).
Second, the bleaching process in FRAP experiments is often inaccurately modelled. Bleaching is posited to be homogeneous or to follow a Gaussian distribution throughout bleached circular or rectangular regions, while the molecules outside of the bleached region are assumed to remain unbleached 11 , 12 , 13 , 15 , 16 , 17 , 18 . However, molecules diffusing during the bleaching process can create inhomogeneities both inside and outside of the bleached region; moreover, a delay between bleaching and the start of the recovery measurement can lead to further inhomogeneities (Fig. 1c ). Incorrect assumptions about the bleaching process can thus lead to a severe misestimation of diffusion coefficients 14 , 24 , 25 , 26 , 27 (Fig. 1e ).
Third, in vivo FRAP experiments can be strongly influenced by reaction kinetics such as production or degradation of fluorescent molecules, which can contribute to the observed recovery curve (Fig. 1c ). However, this is mostly neglected in classical FRAP analysis models and can lead to erroneous diffusion estimates (Fig. 1f ) 11 , 12 , 13 , 14 , 15 , 16 , 17 .
To address these shortcomings, we developed the versatile Python-based FRAP analysis software PyFRAP (available at https://mueller-lab.github.io/PyFRAP ). To facilitate data analysis, PyFRAP is equipped with an intuitive graphical user interface (GUI, Fig. 2a ), which gives users without a computational background access to a sophisticated FRAP data analysis work flow from image analysis to statistical model comparison methods (Fig. 2b ). PyFRAP applies the first post-bleach image as initial condition (Fig. 2c ), and numerically simulates the FRAP experiment in realistic two-dimensional or three-dimensional experiment geometries (Fig. 2d, e ); the solution from this simulation is then fitted to the experimental data. Furthermore, PyFRAP can accurately account for both uniform production and degradation during FRAP experiments. PyFRAP saves all analysed data and settings in a logical data structure that can be shared with collaborators or re-used for later analyses (Fig. 2f ). The software is freely available, and the open-source environment allows for rapid expansion through collaborative work 28 to adjust analysis methods to the users’ needs.
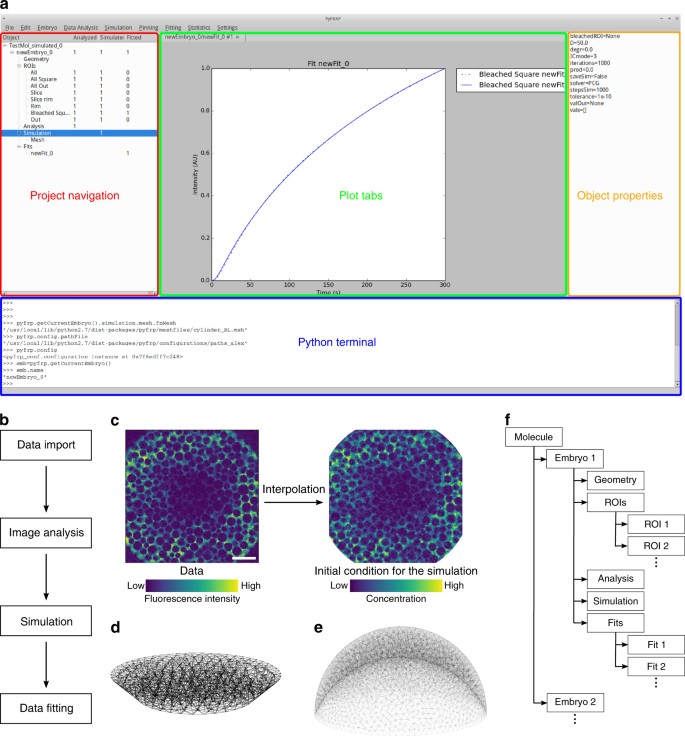
The PyFRAP software package. a Annotated snapshot of the PyFRAP main GUI with project navigation tree (red), plot tabs (green), object property display (orange), and integrated Python terminal (blue). b PyFRAP work flow. c PyFRAP’s interpolation of the first post-bleach image as initial condition for FRAP simulations. The length of the white scale bar represents 100 μm. d , e Spatial discretisation of geometries resembling d a frustum and e a zebrafish embryo at late blastula stages (dome stage). f PyFRAP’s data structure
To demonstrate the utility of PyFRAP, we conducted several typical in vitro and in vivo FRAP experiments (Supplementary Fig. 1 ). PyFRAP accurately determines the diffusion coefficients of fluorescent molecules ranging from 3 to 500 kDa in both artificial and biological contexts. In contrast to currently available software, PyFRAP’s flexible initial conditions also allow analysis of iFRAP experiments, producing results comparable to FRAP. We used PyFRAP to measure the influence that obstacles such as cells exert on the movement of diffusing molecules, and found that such geometric hindrance decreases diffusivity by about one-third. Moreover, PyFRAP provides accurate modelling of reaction kinetics, including production and degradation. Finally, to test the impact of extracellular binding on protein diffusivity, we measured the diffusion of signalling molecules in living zebrafish embryos. We found that the effective diffusivity of a signalling molecule in developing zebrafish was reduced to about one-tenth of its predicted value, in agreement with hindered diffusion models postulating interactions of embryonic signals with diffusion regulators 22 , 29 . Altogether, our analyses highlight how detailed examination of FRAP data can be used to determine the contribution of individual factors to the movement of molecules in controlled artificial and biological contexts 30 .
PyFRAP is a versatile FRAP/iFRAP analysis package
Current FRAP analysis methods often make simplified assumptions about FRAP experimental conditions to aid in the derivation of analytical solutions 11 , 12 , 13 , 14 , 15 , 16 , 18 , and to facilitate numerical simulations 20 , 21 . Such assumptions include reducing complex sample geometries to lower dimensions, idealising the initial bleaching profile, or ignoring additional reaction kinetics potentially underlying fluorescence recovery (Fig. 1c ). Unless the experiment is well approximated by these assumptions (e.g., simple geometry, small bleach spot compared to a large sample volume, sharp bleach profile, no reactions), this can lead to erroneous diffusion estimates (Fig. 1d–f ). To address these shortcomings, we developed PyFRAP. PyFRAP numerically simulates FRAP experiments in realistic three-dimensional geometries using an interpolation of the first post-bleach image as initial condition. This simulation is then fitted to the experimental data, incorporating reaction kinetics such as uniform production and degradation.
PyFRAP is an open-source Python-based FRAP analysis software that runs on the major operating systems Microsoft Windows, Mac OSX and Linux. Over the past 20 years, Python has become the standard programming language for scientific research because of the availability of versatile add-on packages and its intuitive and simple syntax 31 . Building on the resourcefulness of Python, PyFRAP is based on commonly used packages such as PyQT, SciPy and FiPy 32 , 33 , 34 , 35 , 36 . PyFRAP comes with an intuitive graphical user interface (GUI, Fig. 2a ) and a fully documented application programming interface (API) allowing quick development of scripts or modifications of the PyFRAP code. PyFRAP’s functionalities include sophisticated image processing functions useful for FRAP analysis, customisable geometry and analysis region definitions, a finite element partial differential equation (PDE) solver that simulates FRAP/iFRAP experiments with adjustable options, statistical tools for averaging and model comparison, and multiple plotting and input/output functions (see Methods section and Supplementary Note 1 for details). To make the software easily accessible, dialogue boxes (software wizards) guide the user step-by-step through data import, image analysis, simulation and fitting.
We programmed PyFRAP to import image data from most common microscope formats, such as .tif, .lsm and .czi. Users can define arbitrary regions of interests (ROIs) that are then used for image analysis, simulation and fitting (Supplementary Fig. 2a ). For some experimental setups, the imaged sample might be larger than the field of view. In these cases, the concentration of molecules in regions outside of the image can be estimated from selected areas in the first image of the recovery image series (Supplementary Fig. 2b ). Uneven illumination is a common artefact in FRAP experiments. PyFRAP can correct this artefact by normalisation using pre-bleach images or using a correction matrix computed from a secondary data set generated with a homogeneously distributed fluorophore 37 , 38 , 39 (see Methods section and Supplementary Fig. 2c for details). To avoid numerical instabilities, PyFRAP allows the user to smooth or denoise the image data using a Gaussian or median filter (see Methods section, Supplementary Note 1 , Supplementary Fig. 3 , and Supplementary Table 1 for details).
FRAP and iFRAP experiments have been performed in a variety of contexts, from the cigar-shaped Drosophila embryo and the relatively flat Drosophila wing disc to the dome-shaped pre-gastrula stage zebrafish embryo 10 , 22 , 29 , 40 , 41 , 42 . These structures have distinct geometries that could impact fluorescence recovery. In fact, we found that simplifying the three-dimensional zebrafish embryo to a two-dimensional disc can frequently lead up to a >200% error in estimated diffusion coefficients (Fig. 1d ). In PyFRAP, users can define arbitrary two-dimensional and three-dimensional geometries using Gmsh 43 or CAD STereoLithography (.stl) files that are then spatially discretised into tetrahedral meshes by Gmsh in combination with TetGen 44 . PyFRAP provides various meshing options, such as local mesh refinements, boundary layer meshes and attractor meshes, allowing users to adapt the mesh to experimental details (see Fig. 2d, e and Supplementary Fig. 4c for example geometries and meshes).
In current FRAP analysis methods, the initial condition of the FRAP experiment is often simplified to a simple rectangular function or a Gaussian profile to approximate sharp or blurred bleach boundaries, respectively 11 , 12 , 14 , 15 , 16 , 17 , 18 , 45 , 46 , 47 . However, light scattering, imperfect bleaching and diffusion during the bleaching process can lead to more complex bleaching profiles and thus need to be considered during FRAP analysis to avoid misestimation of diffusion coefficients 24 , 25 , 30 , 48 . To overcome this issue, PyFRAP uses a bilinear interpolation between pixels of the first post-bleach image to estimate the initial condition for mesh cells. This initial condition closely resembles initial experimental bleaching profiles and concentration distributions (Fig. 2c ). Moreover, in contrast to most current FRAP analysis methods 11 , 12 , 13 , 14 , 15 , 16 , 17 , 18 , 46 , 47 , PyFRAP does not fit a mathematical expression based on simplified assumptions to the data; instead, PyFRAP uses FiPy 32 to simulate the experiment numerically, resulting in a solution that incorporates the realistic three-dimensional geometry and initial conditions. The numerical simulation is then fitted to the FRAP data by minimising the sum of squared differences using classical optimisation algorithms 49 , 50 , 51 (see Methods section for details).
In typical FRAP and iFRAP experiments, a protein of interest is tagged with a fluorescent protein and expressed within a tissue. In such an experiment, the fusion protein is often actively produced at the same time that FRAP is carried out; additionally, fusion proteins undergo degradation over time. Depending on how the fusion protein is expressed (promoter-driven expression, mRNA injection, etc.), its degradation kinetics, and the timescale of the FRAP/iFRAP experiment, production and degradation can dramatically influence recovery curves. Ignoring reaction kinetics in FRAP experiments could therefore lead to erroneous diffusion coefficient estimates. Indeed, recovery curves with pure diffusion fitted to a simulated reaction-dominant data set often resulted in a >200% error in the estimated diffusion coefficients (Fig. 1f ). To ensure that the appropriate reaction kinetics are considered when analysing FRAP data, PyFRAP is equipped with four models: (1) Pure diffusion, (2) diffusion with production, (3) diffusion with degradation and (4) diffusion with production and degradation (see Methods section for details). The model can be constrained with previous reaction rate measurements from assays such as fluorescence decay after photoconversion (FDAP) 52 , 53 ; alternatively, production and degradation rates can be directly obtained from fitting the FRAP data. Below, we discuss methods to determine which approaches are most appropriate for a given data set.
An advantage of PyFRAP is its ability to assess FRAP data using multiple models of varying complexity, from pure diffusion to combined reaction-diffusion kinetics. However, determining which model is appropriate for a given data set can be challenging. Choosing the incorrect model can lead to overfitting and potentially false diffusion coefficients 54 . The Akaike information criterion (AIC) is a statistical tool that can aid in model selection 55 . PyFRAP’s implementation of the AIC allows users to compare the models mentioned above and determines the most likely model based on a relative weighted measure that includes both the model’s log-likelihood and its degrees of freedom, i.e., the number of model parameters. Moreover, PyFRAP provides several statistical tests (Supplementary Table 2 ) to assess differences between measurements and obtained fits, such as Student’s t -test 56 for normally distributed data or the Mann–Whitney-U-test 57 , which does not require normally distributed data. The Shapiro–Wilk-test can be used to assess whether the measured diffusivities follow a normal distribution 58 and whether application of Student’s t -test or the Mann–Whitney-U-test is justified.
PyFRAP’s object-oriented data structure (Fig. 2f ) can be saved into serialised objects and easily loaded for further analysis or shared with collaborators. In addition, PyFRAP lets users visualise every aspect of PyFRAP’s analysis work flow and save plots and images into publication-ready figures.
Benchmarking PyFRAP
To validate PyFRAP, we first determined whether it can recover true diffusion coefficients and reaction kinetics from simulated data. We used our previous in-house solution 22 , 29 , 42 based on the commercial programs MATLAB and COMSOL multiphysics to simulate 24 FRAP experiments with different reaction kinetics and diffusion coefficients. Using PyFRAP, the simulated data sets were fitted with all four possible reaction-diffusion models (see above). We determined a maximal error of 10% (average error: 2%, Supplementary Table 3 ) between simulated and estimated diffusion coefficients, demonstrating that PyFRAP recovers correct diffusion coefficients within the error tolerance of the numerical simulations.
Next, we tested whether PyFRAP’s implementation of the AIC allows identification of the models used to create the simulated data. When the data were simulated with models describing either pure diffusion, diffusion and degradation, or diffusion and production, the AIC predicted the correct underlying model (Supplementary Table 3 ). However, the model selection based on the AIC did not favour the correct model for data sets that included diffusion combined with both production and degradation, since models with fewer degrees of freedom provided smaller Akaike weight values. Simulations involving diffusion, production and degradation can generate data effectively indistinguishable from data simulated with only diffusion and production or diffusion and degradation, explaining why the AIC cannot predict the correct model in this case.
To assess PyFRAP’s performance in comparison with other available software packages based on analytical 17 , 46 , 47 , 59 or numerical 20 , 21 , 60 approaches (Supplementary Table 4 ), we used easyFRAP 47 , Virtual FRAP 20 , FrapCalc 46 , simFRAP 21 and PyFRAP itself to analyse simulated FRAP experiments (Supplementary Note 2 , Fig. 3 ). We simulated 18 experiments in which geometry, relative bleach window size, and diffusion coefficients differed. Simulations were conducted either in a simple circular two-dimensional domain or a complex three-dimensional zebrafish embryo-like geometry (Fig. 2e ). FrapCalc and easyFRAP assume circular bleach windows 12 , 46 , 47 ; to facilitate comparison, we therefore simulated FRAP experiments with circular bleach windows. Bleach window sizes comprised 5, 10 or 50% of the slice diameter, representing different proportions between fluorescent and bleached pools (Fig. 3b ). Simulations were performed with three biologically relevant diffusion coefficients: 10, 50 and 200 μm 2 /s.
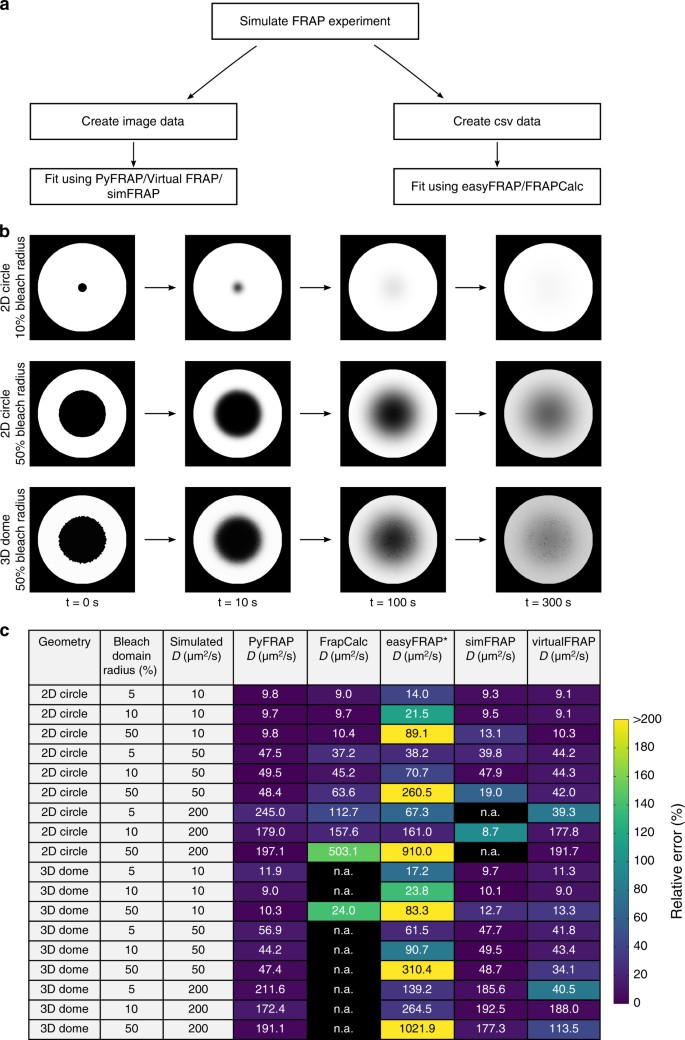
PyFRAP benchmarking simulation experiment. a Workflow of PyFRAP benchmarking. b Examples of simulated data sets for different bleach spot sizes and geometries. c Benchmarking results of PyFRAP against currently available software packages using simulated FRAP experiments. Simulation experiments varied in bleached region size, diffusion coefficient and experiment geometry. All diffusion coefficients and estimates are given in units of μm 2 /s. n.a. indicates that the software was not able to fit the simulated data. Colours indicate relative estimation error in %. Diffusion coefficients determined by easyFRAP (asterisk) were computed in combination with an equation providing a relationship between recovery rate, bleached domain size and diffusivity 45
Simulation-based programs (PyFRAP, virtualFRAP and simFRAP) generally provided better results than analytical solutions (easyFRAP and FrapCalc): FrapCalc and easyFRAP were either unable to determine diffusion coefficients, or provided diffusivities that were off by at least 20% for most experiments (Fig. 3c ). Fast recovery dynamics were challenging for all tested software. One reason for this is that fewer data points were recorded during the actual recovery process of highly diffusive molecules due to a fixed frame rate of 1 frame/s in the simulated test data sets, leading to larger errors; moreover, for fast recovery dynamics errors from interpolating simulations onto images are more severe. The analytical software packages provided better results for the two-dimensional compared to three-dimensional geometries, while simulation-based approaches showed no clear trend regarding geometry. In terms of bleach window radius, the analytical solutions performed worst if the window diameter was 50% of the slice diameter. This effect might be due to the assumption of an infinite pool of fluorescent molecules outside of the bleached region 12 —when the bleach window is very large, the pool of unbleached fluorescent molecules is small, which conflicts with the assumption of an infinite pool. In contrast, PyFRAP outperformed all current software packages and exhibited the smallest error between predicted and simulated diffusion coefficients (Fig. 3c ).
Applications of PyFRAP to measure diffusion hindrance
In vivo, it is thought that the overall movement of molecules is affected by binding interactions and by the presence of obstacles such as cells, resulting in a reduced effective diffusion coefficient of secreted proteins that move through tissues 22 . However, the effects of these interactions have not been rigorously tested experimentally. We therefore employed PyFRAP to examine the effects of obstacles and binding partners on the effective diffusivity of dextrans and proteins in experimentally controlled in vitro geometries and in living zebrafish embryos.
First, we measured diffusion coefficients of a wide range of differently sized molecules (Supplementary Table 5 ) in a simple in vitro context in the absence of binding partners or obstacles. We performed FRAP experiments with different bleach geometries using fluorophore-coupled dextrans ranging from 3 to 500 kDa in molecular weight (Fig. 4a–d , Supplementary Figs. 5 and 6 ), and compared the results with theoretical predictions and literature values. Fluorescence recovery in these in vitro experiments should be purely defined by diffusion, and the theoretical diffusivities D of spherical molecules can be calculated from their radii r based on the relationship D ~ 1/ r as postulated by the Einstein–Stokes equation (Supplementary Note 3 ). The diffusion coefficients determined by PyFRAP were in good agreement with literature values and theoretical predictions (Fig. 5a , Supplementary Tables 6 and 7 ).
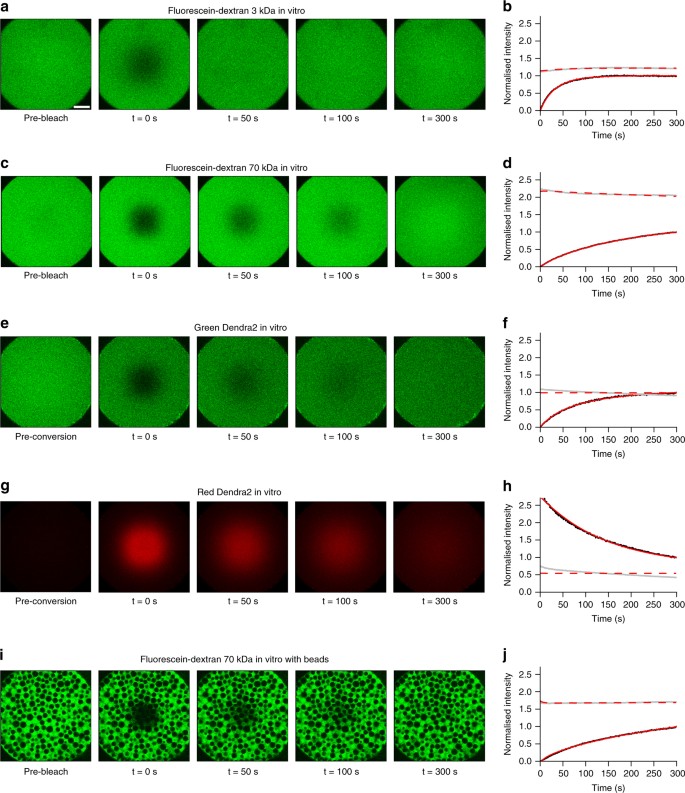
Examples of in vitro FRAP and iFRAP experiments and the resulting fits to measure free diffusion. a , c , e , g , i In vitro FRAP and iFRAP experiment images and b , d , f , h , j fits with PyFRAP. Black and grey dots represent data points of bleached and slice ROI, respectively. Red solid and dashed lines show the respective fits. a – d FRAP experiments with 3 and 70 kDa fluorescent dextrans (see Supplementary Fig. 5 for the full data set with fluorescent dextrans between 3 and 500 kDa). e – h iFRAP experiment with photoconverted Dendra2 protein showing data for the green ( e , f ) and the red ( g , h ) channel. i , j FRAP experiment with 70 kDa fluorescent dextran in the presence of polyacrylamide beads. Recovery curves were normalised between 0 (intensity in the bleached ROI at the first post-bleach time point) and 1 (intensity in the bleached ROI at the last post-bleach time point) to allow comparison across data sets. The length of the white scale bar in a represents 100 μm, and all images were acquired with the same magnification
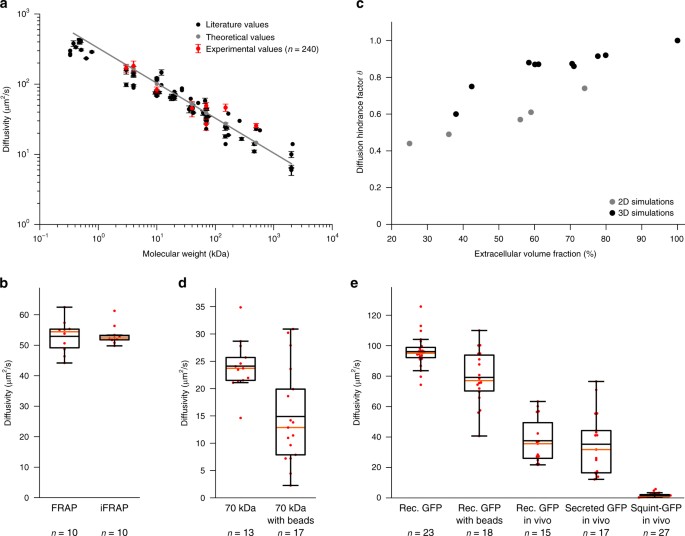
Effective diffusion coefficients determined by PyFRAP. a Results of in vitro experiments and PyFRAP analysis for freely diffusing fluorescent dextrans of different molecular weights. Black markers indicate literature values for fluorescent dextrans, red markers the mean effective diffusion estimates obtained by in vitro experiments and PyFRAP analysis, and grey markers the theoretical estimates derived from the Einstein-Stokes equation (see Supplementary Note 3 ). Red error bars show the standard deviation of PyFRAP’s effective diffusion estimates, and black error bars show the standard deviation of the literature values listed in Supplementary Table 7 . The grey line represents a linear regression fit to the theoretical values. b Results of FRAP/iFRAP experiments for the photoconvertible protein Dendra2. c Results of simulations investigating the influence of tortuosity on effective diffusion for differently packed bead experiments. Grey and black markers indicate 2D and 3D simulation results, respectively. d Results of fluorescent dextran experiments demonstrating the impact of tortuosity on effective diffusivities. e Results of GFP experiments to analyse the impact of tortuosity, embryonic extracelluar environment, protein production, and extracellular binding on effective diffusion estimates. Box plots in b , d , e show median (orange line), mean (black horizontal line inside box), 25% quantiles (box), and all included data points (red markers). Whiskers extend to the smallest data point within the 1.5 interquartile range of the lower quartile, and to the largest data point within the 1.5 interquartile range of the upper quartile
A variant of FRAP that allows exclusion of reaction kinetics, such as production, and thus decrease the number of unknown experimental parameters is iFRAP (Fig. 1b ). To perform in vitro iFRAP experiments, we used the green-to-red photoconvertible protein Dendra2 61 . Since photoconverting Dendra2 from green to red can also be interpreted as bleaching the original green fluorescence, measuring unconverted and converted protein distributions produces both FRAP and iFRAP experiments at the same time. To test whether PyFRAP correctly analyses iFRAP data, we used the experimental FRAP and iFRAP sets independently and assessed whether the obtained diffusion values are equal (Fig. 4e–h ). Using FRAP we measured a Dendra2 diffusivity of 52.9 ± 5.2 (standard deviation) μm 2 /s, and using iFRAP we obtained a similar value of 53.3 ± 3.1 μm 2 /s (Fig. 5b , average difference between the two diffusivities per data set: 2.6 ± 1.5 μm 2 /s).
Next, we examined the effect of tortuosity on diffusion. In biological samples, the path length that molecules take increases as they move around obstacles such as cells. The effect of this tortuous movement can be described by the diffusion hindrance factor (also known as diffusion permeability 62 ) θ = 1/ λ 2 = D * / D , where λ is the tortuosity, D * is the effective diffusion coefficient (with obstacles), and D is the free diffusion coefficient (without obstacles). To assess the expected magnitude of tortuosity on altering effective diffusivity, we first performed numerical simulations of FRAP experiments with and without radial obstacles in two- and three-dimensional geometries. Radial obstacles were either placed regularly, randomly, or following a nearly-ideal packing scheme, resulting in an extracellular volume fraction (EVF, i.e., the space available for molecules to diffuse) ranging from 78% down to 25% (Supplementary Fig. 7 ). These simulations demonstrated that recovery rates are slowed down as the EVF decreases (Fig. 5c , Supplementary Table 8 ). If the geometry is two-dimensional, an EVF of 25% results in an expected reduction in effective diffusivity of approximately 66%. In three-dimensional simulation experiments, we obtained a reduction of effective diffusion coefficients by 40% when the EVF was decreased to 38% (Supplementary Note 3 ).
To determine whether the presence of obstacles decreases effective diffusivity as predicted by our simulations, we performed FRAP assays in vitro with a fluorescein-coupled 70 kDa dextran (Fig. 4i, j ) or recombinant GFP (Supplementary Fig. 8 ) in the presence of polyacrylamide beads. Consistent with our predictions, recovery was slower in the presence of beads, and the effective diffusivity of fluorescein-coupled 70 kDa dextran dropped from 24.1 ± 0.4 (standard error) μm 2 /s to 14.9 ± 0.5 μm 2 /s, suggesting an EVF of 39% ( θ = 0.61) (Fig. 5c, d , Supplementary Tables 8 and 9 ). Similarly, for recombinant GFP effective diffusivity dropped by 18% (Fig. 5e , Supplementary Table 10 , Supplementary Fig. 8a–d ).
To assess diffusion hindrance in vivo, we injected recombinant GFP protein into the extracellular space of living zebrafish embryos. We found that the effective diffusivity in vivo was 60% lower than for freely diffusing GFP, and 53% lower than in in vitro experiments with beads (Fig. 5e , Supplementary Table 10 , Supplementary Fig. 8e, f ). This suggests that tortuosity in zebrafish embryos is higher than in the in vitro bead assay. Importantly, we found similar diffusion coefficients of 36 μm 2 /s in vivo for extracellularly injected recombinant GFP and secreted GFP constantly produced from injected mRNA, showing that PyFRAP can properly account for both diffusion and production (Fig. 5e , Supplementary Table 10 , Supplementary Fig. 8g, h ).
Finally, we examined the effects of binding interactions on effective diffusivity. GFP presumably does not experience significant binding interactions with extracellular molecules in zebrafish embryos, although its movement is affected by obstructions like cells and cellular extensions. In contrast, secreted signalling molecules are expected to interact with extracellular molecules such as receptors and extracellular matrix components 22 . To assess the effect that interactions with extracellular molecules might have on secreted signalling molecules, we injected mRNA encoding the TGF β -superfamily member Squint fused to GFP into zebrafish embryos 29 . Squint-GFP is approximately 1.5 times larger than GFP and according to the Einstein-Stokes equation (Supplementary Note 3 ) would be predicted to have an approximately 1.14 times smaller diffusion coefficient than GFP (effective diffusivity D (GFP) = 36 μm 2 /s, expected effective diffusivity D (Squint-GFP) = 31 μm 2 /s). However, we measured an effective diffusion coefficient of approximately 2 μm 2 /s for Squint-GFP in living zebrafish embryos, ~90% lower than the predicted diffusion coefficient (Fig. 5e , Supplementary Table 10 , Supplementary Figs. 8i , j and 9 ). These findings are consistent with previous measurements 29 and with the idea that interactions with so far unidentified binding partners slow down the effective diffusion of embryonic signalling molecules like Squint-GFP 22 , 29 .
Although FRAP analyses have long been used to measure relative differences in mobilities between macromolecules, analysis tools to accurately and quantitatively determine effective diffusion coefficients from FRAP data are lacking. Current analysis tools impose several simplifications including one-dimensional or two-dimensional reductions of complex three-dimensional geometries, idealised bleaching conditions, and the absence of important reaction kinetics. When the experimental conditions closely resemble the simplified assumptions, e.g., small bleach domains and negligible reaction kinetics, these tools can rapidly provide reasonable diffusion estimates (Fig. 3c ). However, experimental conditions are often more complex, and the use of simplified assumptions may yield drastically divergent diffusion coefficients (Fig. 1d–f ). PyFRAP addresses these shortcomings by providing a simulation-based analysis that incorporates realistic geometries, bleaching conditions and reaction kinetics.
We found that PyFRAP’s data analysis pipeline is numerically reliable, recovered the correct diffusion coefficients and reaction kinetics, and additionally predicted the correct underlying reaction-diffusion models for simulated test data sets with known diffusion, production, and degradation parameters. PyFRAP consistently outperformed all other tested software packages, demonstrating its strength as a novel FRAP analysis method. Furthermore, PyFRAP was able to determine diffusion coefficients comparable to both theoretical and previously experimentally measured estimates for macromolecules with molecular weights ranging over two orders of magnitude. Since PyFRAP can analyse data independently of any assumptions about the initial conditions, it is suitable to analyse both FRAP and iFRAP experiments. iFRAP has recently been developed as an alternative to FRAP due the increasing availability of photoconvertible proteins and allows ignoring reaction kinetics such as production. We performed tandem FRAP/iFRAP experiments to analyse the diffusion of the photoconvertible protein Dendra2 and found equal diffusion coefficients in vitro with both methods.
FRAP experiments are typically performed in tissues in which macromolecules need to move around cellular obstacles, resulting in slower fluorescence recovery. To determine how this tortuosity might affect diffusion coefficients estimated from FRAP experiments, we first simulated FRAP experiments in two- and three-dimensional geometries introducing radial beads at different densities to vary the extracellular volume fraction (EVF). Our simulations showed a strong correlation between tortuosity and effective diffusivity and agree with previous theoretical work including Monte-Carlo simulations and homogenisation theory 62 , 63 , 64 , 65 . We then tested the predictions from these simulations with in vitro experiments using polyacrylamide beads to mimic cells. Compared to experiments without beads, the effective diffusion coefficient decreased by 39% (diffusion hindrance factor θ = 0.61) for 70 kDa fluorescein-dextran and 18% ( θ = 0.82) for recombinant GFP. In living zebrafish embryos, effective diffusivity is much further reduced (Fig. 5e ). It is unlikely that this is due to different viscosity of the extracellular medium in vivo, since free GFP diffusion is only marginally reduced in zebrafish embryos 22 . Instead, it is plausible that the complex geometries of real extracelluar environments—which include filopodia, extracellular matrix, and cavities that might act as dead end pores—could further increase tortuosity 62 . Finally, most in vivo FRAP experiments are affected by biochemical reactions such as production and degradation of proteins, which must be taken into account for accurate diffusion coefficient estimates (Fig. 1c, f ). PyFRAP offers various models for different reaction kinetics and can accurately estimate diffusion coefficients from data sets that include constant production and degradation.
PyFRAP measures effective diffusion, but due to its built-in PDE solver it could be extended in the future to consider spatially inhomogeneous kinetics and advective fluxes and to perhaps even determine the diffusivities of individual species in polydisperse mixtures of fluorescent molecules 66 , 67 . While PyFRAP can simulate three-dimensional FRAP experiments, FRAP data is currently almost exclusively obtained from two-dimensional confocal microscopy. In recent years, the development of light-sheet microscopy made fast three-dimensional imaging with low phototoxicity feasible 68 . In the future, PyFRAP’s image analysis tools could be extended to fit light-sheet microscopy data, which might provide deeper insights into the three-dimensional dynamics of molecule movement including convective flows or spatially inhomogeneous diffusion.
FRAP/iFRAP experiments in vitro
FRAP experiments to measure pure diffusion and tortuosity effects were conducted in a frustum-like plexiglass hole. Holes around 700 μm in diameter and about 100 μm in depth were drilled into a plexiglass block using a dental drill. Due to the small depth, the resulting shape was frustum-like with an upper base of 510 μm diameter.
Holes were filled with aqueous solutions of FITC-/fluorescein-labelled dextrans of different sizes, recombinant GFP, or Dendra2 protein (Supplementary Table 5 ) using a micro-pipette. Dendra2 protein was centrifuged at 16,000 × g for 30 min at 4 °C to remove protein aggregates. Excess liquid was removed from the hole by pipetting under observation with a stereo microscope.
To model the effect of tortuosity in the in vitro FRAP experiments, polyacrylamide beads were added to the sample solution. The microbeads (Bio-Gel P-2 Gel, <45 μm wet bead size) were first soaked in distilled water overnight for hydration. The beads were then centrifuged at 300 × g , the supernatant removed, and the required quantity of beads transferred to another tube for resuspension in fluorescein-dextran or GFP+BSA solution. This was repeated and followed by removal of the supernatant, leaving a concentrated slurry of beads and fluorescent solution for the experiments. The beads were transferred into the plexiglass template and settled within 1–2 min.
To prevent evaporation, mineral oil (Sigma) was placed around the solution before sealing the hole with a cover slip (No 1.5). Supplementary Fig. 1a outlines the sample preparation process for in vitro experiments. The sample was upended carefully and mounted on an inverted confocal microscope. Images were taken using an LSM 780 NLO microscope (ZEISS) with an LD LCI Plan-Apochromat 25×/0.8 Imm Korr DIC objective (ZEISS) and immersion oil (Immersol TM W, n = 1.334 at 23 °C, ZEISS). First, a plane approximately in the middle of the hole was chosen and the z -position set to zero. Then, the position of the highest and lowest point was determined. Cuboid volumes (141.42 μm × 141.42 μm × 100 μm) were bleached by imaging a z -stack at highest laser power (488 nm) or photoconverted at moderate laser power. Time series of 300 images (512 pixels × 512 pixels) were taken with a speed of 1 frame/s (pixel dwell time: 3.15 μs) over a duration of 5 min. The zoom was set to 0.7, and the resulting images had a size of 566.79 μm × 566.79 μm.
After the FRAP experiment, the template was cleaned using distilled water, soap, and an interdental toothbrush.
FRAP experiments in vivo
Zebrafish embryos ( Danio rerio ) were collected 10 min after mating and proteolytically dechorionated 22 , 29 , 42 . For the experiments with recombinant GFP, 100 pg of recombinant GFP were injected into the extracelluar space when zebrafish embryos reached high stage 22 , 29 , 69 (Supplementary Table 10 ). For experiments with secreted GFP 29 , 100 pg of the mRNA encoding the fluorescent protein were injected at the one-cell stage. For experiments with Squint-GFP 29 , either 30 or 200 pg of mRNA were injected at the one-cell stage. At dome stage, embryos were mounted in drops of 1% low-melting-point agarose animal pole down onto a glass-bottom dish (MatTek Corp. P35G-1.5-20-C), and as soon as the drops solidified covered with Danieau’s medium 29 , 42 to prevent the embryos from drying out. Supplementary Fig. 1b outlines the in vivo sample preparation process.
Confocal images were taken roughly at a depth of 40 μm from the animal pole into the embryo. For data sets injected with 200 pg of Squint-GFP-encoding mRNA, images were acquired with the same settings as described for the in vitro experiments either with 1 frame/s for 300 s, or 1 frame/10 s for 3000 s. Images of embryos injected with 30 pg of Squint-GFP-encoding mRNA were taken with a spatial resolution of 340.08 μm × 340.08 μm and 1 frame/10 s for 3000 s. Data sets for recombinant GFP in vivo were acquired with the same microscope settings as the experiments conducted in vitro.
ROI selection
PyFRAP’s image analysis depends on defining specific ROIs for the experimental data and simulations. Users can define multiple different geometrical shapes of ROIs in three-dimensional space such as cylinders, prisms, and any kind of addition or subtraction between ROIs. The specified ROIs are then used for image analysis, estimating concentrations outside the field of view, evaluating the simulation, and fitting to the analysed data. PyFRAP is equipped with an ROI manager and wizards for several standard sets of ROIs.
Image analysis
Let Ω i (with i ∈ {1, 2, …, n Ω } and n Ω the number of ROIs) be the list of ROIs specified for PyFRAP’s analysis. The mean intensity over the ROI Ω i at time t j (with j ∈ {1, 2, …, n t } and n t the number of images) is then calculated by
where A i is the area of Ω i , and I ( x k , y l , t j ) is the intensity at pixel ( x k , y l ) (with k ∈ {1, 2, …, n x } and n x the number of rows in the images, and with l ∈ {1, 2, …, n y } and n y the number of columns in the images).
FRAP image data were analysed within the ROIs Ω bleached and Ω slice . Ω slice was defined as a circular domain with centre C slice and radius r slice . Since the imaging depth varied between experiments, both C slice and r slice were cropped for each data set. The bleached ROI Ω bleached was defined as a square with sidelength s bleached and left-lower corner at O bleached = C slice − \(\frac{1}{2}\) ( s bleached , s bleached ). The definition of both ROIs is shown in Supplementary Fig. 2a .
Accounting for uneven illumination
Uneven imaging due to inhomogeneous sample illumination is a common problem in microscopy 37 , 38 , 39 . We implemented two solutions in PyFRAP to address this problem: (1) Normalisation by an image acquired before bleaching, and (2) applying a flattening mask derived from imaging a homogeneous fluorescent sample. The pixel-wise mean image over n t images can be defined as
To avoid noise-induced singularities when normalising, PyFRAP computes a mean normalisation mask M pre over multiple pre-bleach images, and then divides each image of the recovery time series pixel-wise by the computed mask
where O norm is the optimal data offset computed via
Similarly, the flattening mask F is computed using the mean over multiple images of a fluorophore spread homogeneously across a cover slip, M flat :
Similar to the normalisation in Eq. ( 4 ), the optimal data offset O flat is obtained by taking the maximum over all minimum intensities of images in both recovery and flattening data sets. The recovery data set is obtained by pixel-wise multiplication of the recovery image with the flattening mask obtained in Eq. ( 5 ):
An outline of both correction methods is shown in Supplementary Fig. 2c .
In the present study, two pre-bleach images were acquired per sample for the normalisation mask, and two images of fluorescein conjugated to a 40 kDa dextran or recombinant GFP homogeneously spread on a cover slip were acquired for the flattening approach. The effects of flattening and normalisation on data analysis are described in Supplementary Note 1 .
Accounting for background fluorescence
Background subtraction is a standard procedure to extract the true signal of microscope images 38 , 39 . Similar to the flattening and normalisation masks, PyFRAP takes the average over multiple pixels to obtain a background mask and then subtracts it pixel-wise 38 , 39 :
The mean of two images without a sample was determined to compute a background mask. The effect of background subtraction is discussed in Supplementary Note 1 .
Application of filters for noise reduction
Microscope data sets are often noisy, causing problems for normalisation and simulation. PyFRAP smooths noisy pixels by either applying a Gaussian blur with standard deviation σ gauss , or a median filter with filter window radius r median . We found that σ gauss = 2 and r median = 5 provided good results for the data in the present study (see Supplementary Note 1 ).
Accounting for fluorescence outside of the imaging view
In some cases it is not possible to capture the whole sample in one field of view under the microscope, and the concentration in the non-imaged regions needs to be estimated. PyFRAP solves this by letting users define an ROI Ω rim to select an approximation of the average unbleached intensity from the first image of the recovery image series:
Ω rim is defined by Ω rim = Ω slice − Ω centre , where
with ( x c , y c ) the centre pixel coordinates of the image. Ω rim thus defines a small annulus comprising all pixels ( x k , y l ) inside Ω slice that have a distance of at least ρ rim r slice from the centre of the image (Supplementary Fig. 2b ). ρ rim = 0.66 and ρ rim = 0.4585 were found to provide good values for the in vitro and in vivo experiments, respectively.
Simulations
PyFRAP simulates FRAP experiments numerically. Ignoring reaction kinetics, a FRAP experiment can be described by the diffusion equation
where c ( x , t ) is the concentration of the measured molecule at position x = \(\left\langle {x,y,z} \right\rangle\) and time t inside the domain Ω, and D is its scalar diffusion coefficient. The diffusion coefficient is assumed to be constant and homogeneous.
Since the sample is assumed to be a closed system, no-flux Neumann boundary conditions were defined as
where n is the normal vector of the boundary ∂Ω at position x .
Initial conditions for simulations
The initial conditions are given by the bilinear interpolation P between pixels of the initial post-bleaching image:
I ( x k ′ , y l ′ ) with k ′, l ′ ∈ {1, 2} represents the intensities in the initial image of the four pixels surrounding ( x , y ). If ( x , y ) is outside of the visible ROI in the initial image (Ω 1 ), the rim concentration c rim given in Eq. ( 8 ) is combined piece-wise with Eq. ( 12 ) to give the initial condition
Simulation geometry
PyFRAP comes with its own geometry definition tool. Geometry definitions can then be converted into the Gmsh format 43 for meshing. PyFRAP can read Gmsh’s geometry definition files, use Gmsh’s mesh files, or import STereoLithography (.stl) files, allowing users to define arbitrary two- and three-dimensional geometries. This gives users the ability to describe a realistic FRAP experiment geometry with the necessary precision.
The simulation geometry Ω for the in vitro experiments was a conical frustum with upper radius r upper = 317.65 pixels, lower radius r lower = 224.25 pixels, and height h ≈ 90.33 pixels (Supplementary Fig. 4b ). For the in vivo experiments, the simulation geometry resembled a zebrafish embryo at dome stage, i.e., the intersection of two hemispheres intersecting each other at the equator of the outer hemisphere. Since the geometry depends on the radius of the embryo in the initial image, r imaging was calculated separately for each experiment 29 , 70 . Assuming that the radius of the inner hemisphere r inner is 10% larger than the one of the outer hemisphere, r outer , the geometry can be computed by
where d centre is the distance between the two centres of the hemispheres. Supplementary Fig. 4a shows a schematic of the zebrafish dome stage geometry.
Meshing for simulations
PyFRAP discretises simulation geometries using Gmsh 43 in combination with TetGen 44 into tetrahedral meshes. PyFRAP utilises almost all functionalities of Gmsh—such as boundary layer meshes, attractor meshes, mesh merging and mesh refinement—allowing users to apply fine meshes where they are needed.
The overall default element size in the present study was v = 25 pixels 3 . To overcome numerical instabilities, such as Gibbs phenomena at the boundary of Ω bleached , the mesh around the bleached area boundary was refined using a boundary layer mesh of thickness w BL = 30 pixels and element size v BL = 15 pixels 3 . Since only the simulation inside Ω slice and Ω bleached is used to fit the FRAP experiments, the mesh inside Ω slice was also refined to an element size of v slice = 15 pixels 3 . Supplementary Fig. 4c, e shows an example of a tetrahedral mesh with both slice refinement and boundary layer meshes for the zebrafish dome geometry described in the previous section.
All partial differential equations (PDEs) were simulated using the FiPy toolbox 32 . The LU factorisation algorithm or the Preconditioned-Conjugated-Gradient algorithm implemented in PySparse were used to solve the linear system at each time step.
Simulation parameters
All simulations were performed with a reference diffusion coefficient of D = 50 pixels 2 /s. To ensure that the simulations run long enough to capture the full recovery of the FRAP experiment, the end time point of the simulation was set to t sim,end = 1680 s for experiments conducted with an acquisition interval of Δ t = 1 s. Since the recovery is steepest at the beginning of the simulations, a logarithmic time-stepping scheme was used, making early time steps shorter to achieve greater accuracy. A summary of all simulation parameters used to analyse the FRAP data in the present study is given in Supplementary Table 11 .
To avoid the need to re-simulate the FRAP experiment for each choice of diffusion coefficient D , PyFRAP uses the self-similarity property of the solution to Eq. ( 10 ). For example, a simulated FRAP experiment with the diffusion coefficient D = 50 pixels 2 /s results in the same recovery behaviour as an experiment with the diffusion coefficient D = 200 pixels 2 /s, just four times slower. This can be described as
where D ref is the reference diffusion coefficient, i.e., the diffusion coefficient used for the simulation of Eq. ( 10 ). Supplementary Fig. 4d shows simulated recovery curves for various diffusion coefficients illustrating this self-similarity property.
PyFRAP allows users to fit four different models to FRAP data: (1) Pure diffusion, (2) diffusion and production, (3) diffusion and degradation, (4) diffusion with degradation and production, and each of these models with an additional set of equalisation parameters (see below). In case of pure diffusion, the solution for the diffusion coefficient D over a given ROI Ω i is simply given by the volume integral of the solution in Eq. ( 15 ):
A summary of all parameters used to fit the FRAP data in the present study is given in Supplementary Table 12 .
Extending the diffusion model with reaction kinetics
Spatially uniform production was added to the scaled FRAP model defined in Eq. ( 15 ) or in Eq. ( 20 ) by
where k 2 is the production rate. To add spatially uniform degradation, the resulting solution is given by
The parameter k 1 represents the degradation rate constant. Adding both degradation and production to the system results in the following superposition of solutions:
Accounting for varying fluorophore fractions by equalisation
FRAP experiments can vary in intensity during the experiment due to, for example, an increase or decrease in extracellular volume fraction, due to molecules moving in and out of the imaging plane, or due to an immobile fraction of fluorescent molecules. These effects are accounted for by equalisation, which normalises both simulation and data recovery curves to an equivalent scale between 0 and 1. During the fitting process, the simulated recovery curves are slightly lifted or lowered to better resemble overall fluorescence levels. This can be written as
where E i is the equalisation factor for ROI Ω i . The background \(\bar c_{{\mathrm{min}}}\) was chosen to be the smallest concentration of the bleached ROI inside the imaging region (Ω bleached ), over the whole time series
and the normalisation value \(\bar c_{{\mathrm{max}}}\) to be the maximum concentration inside the whole imaging ROI (Ω slice ), over the whole time series
Minimisation and parameter estimation
Choosing one of the models defined in Eqs. ( 15 ), ( 16 ), ( 17 ), ( 18 ) and ( 19 ), the sum of squared differences, SSD, was calculated by
where t j ∈ 0, .., T are all time points of the FRAP data set, and Ω i ∈ Ω bleached , Ω slice are the two ROIs of interest yielding a mean optimal fit between all fitted ROIs. The minimisation of Eq. ( 23 ) was carried out using a constrained Nelder–Mead algorithm 49 . Since especially for a larger number of degrees of freedom the minimisation algorithm tended to stop in local minima, initial guesses for the diffusion coefficient D were tested over two orders of magnitude, and the fit yielding the minimum SSD was considered optimal.
Analysis speed
Details of the method to determine PyFRAP’s performance in terms of analysis speed are described in Supplementary Note 4 and Supplementary Tables 13 and 14 .
PyFRAP offers four statistical tools (Supplementary Table 2 ) allowing users to test whether the estimated diffusion coefficient for one experimental group is significantly different from another one. The statistical tools include the two most prominent parametric significance tests, the Student’s t -test 56 and a modification of this test, Welch’s t -test 71 , which both assume normally distributed test groups. PyFRAP also provides the Shapiro–Wilk test, allowing PyFRAP users to quickly assess whether the estimated diffusion coefficients follow a normal distribution. The Shapiro–Wilk test was recently found to have the best sensitivity compared to other common normality tests 72 . If normality cannot be guaranteed, PyFRAP offers two non-parametric ranked hypothesis tests: The Wilcoxon signed-rank test 73 and the Mann–Whitney U test 57 .
Often, the underlying reaction kinetics of FRAP experiments or the relevance of their contribution might be unknown 54 . However, models with more parameters generally provide better fits than simpler models. The AIC 55 allows users to evaluate which model fits the data the best while keeping model complexity low. For this, let
be the vector of unknown diffusion coefficient D , reaction rates k 1 and k 2 , and E 1 , E 2 , … a list of equalisation factors. Moreover, let m = m (Θ) be the model prediction using Θ. Assuming that the data is distributed normally around the model
the log-likelihood function at data point i , L i becomes
and is thus identical with the sum of squared differences used for optimisation in Eq. ( 23 ):
The AIC is then given by
where k is the number of parameters of model m and
is the parameter configuration Θ minimising the log-likelihood function (Eq. ( 27 )), i.e., the parameter configuration returned from fitting the model to data. The best model according to the AIC is then m (argmin(AIC i − AIC min )). If the number of sample points is small, the corrected AIC (AICc) provides a more accurate model selection technique:
where n is the number of data points. A rule of thumb for when the AIC (Eq. ( 28 )) or its corrected version (Eq. ( 30 )) should be used is
PyFRAP automatically selects which statistical model is more appropriate if not specified differently.
PyFRAP also provides R 2 -values for each fit: An R 2 -value for each fitted ROI and the product and mean of these values. In general, PyFRAP computes an R 2 -value of an ROI by
where m i and d i are model and data at time i , and \(\bar d\) is the mean over all data points.
Data exclusion
We performed a rigorous screen of all data sets, and we excluded data sets that showed strong radial inhomogeneities in the first post-bleach image due to inhomogeneous distribution of fluorescent molecules. Moreover, we excluded in vitro data sets that showed unstable distributions in the overall fluorescence intensity levels, indicating incomplete bleaching through the depth of the sample.
Code availability
PyFRAP is freely available from https://mueller-lab.github.io/PyFRAP .
Data availability
All data is available from the corresponding author upon request.
Crick, F. Diffusion in embryogenesis. Nature 255 , 420–422 (1970).
Article ADS Google Scholar
Lander, A. D., Nie, Q. & Wan, F. Y. M. Do morphogen gradients arise by diffusion? Dev. Cell. 2 , 785–796 (2002).
Article CAS PubMed Google Scholar
Müller, P. & Schier, A. F. Extracellular movement of signaling molecules. Dev. Cell. 21, 145-158 (2011).
Poo, M. M. & Cone, R. A. Lateral diffusion of rhodopsin in Necturus rods. Exp. Eye Res. 17 , 503–507 (1973).
Liebman, P. A. & Entine, G. Lateral diffusion of visual pigment in photoreceptor disk membranes. Science 185 , 457–459 (1974).
Article ADS CAS PubMed Google Scholar
Loren, N. et al. Fluorescence recovery after photobleaching in material and life sciences: putting theory into practice. Q. Rev. Biophys. 48 , 323–387 (2015).
Lippincott-Schwartz, J., Altan-Bonnet, N. & Patterson, G. H. Photobleaching and photoactivation: following protein dynamics in living cells. Nat. Cell Biol. 5 , S7–S14 (2003).
Article Google Scholar
Bancaud, A., Huet, S., Rabut, G. & Ellenberg, J. Fluorescence perturbation techniques to study mobility and molecular dynamics of proteins in live cells: FRAP, photoactivation, photoconversion, and FLIP. Cold Spring Harb. Protoc. 12 , 1303–1325 (2010).
Google Scholar
Griffin, E. E., Odde, D. J. & Seydoux, G. Regulation of the MEX-5 gradient by a spatially segregated kinase/phosphatase cycle. Cell 146 , 955–958 (2011).
Article CAS PubMed PubMed Central Google Scholar
Zhou, S. et al. Free extracellular diffusion creates the Dpp morphogen gradient of the Drosophila wing disc. Curr. Biol. 22 , 668–675 (2012).
Axelrod, D., Koppel, D. E., Schlessinger, J., Elson, E. & Webb, W. W. Mobility measurement by analysis of fluorescence photobleaching recovery kinetics. Biophys. J. 16 , 1055–1069 (1976).
Article ADS CAS PubMed PubMed Central Google Scholar
Soumpasis, D. Theoretical analysis of fluorescence photobleaching recovery experiments. Biophys. J. 41 , 95–97 (1983).
Sprague, B. L. & McNally, J. G. FRAP analysis of binding: proper and fitting. Trends Cell Biol. 15 , 84–91 (2005).
Kang, M., Day, C. A., Drake, K., Kenworthy, A. K. & DiBenedetto, E. A generalization of theory for two-dimensional Fluorescence Recovery After Photobleaching applicable to confocal laser scanning microscopes. Biophys. J. 97 , 1501–1511 (2009).
Deschout, H. et al. Straightforward FRAP for quantitative diffusion measurements with a laser scanning microscope. Opt. Express 18 , 22886–22905 (2010).
Article ADS PubMed Google Scholar
Kang, M., Day, C. A., Kenworthy, A. K. & DiBenedetto, E. Simplified equation to extract diffusion coefficients from confocal FRAP data. Traffic 13 , 1589–1600 (2012).
Kraft, L. J., Dowler, J. & Kenworthy, A. K. Frap-toolbox: Software for the Analysis of Fluorescence Recovery after Photobleaching . http://www.fraptoolbox.com Accessed 12 Dec 2016 (2014).
Lin, L. & Othmer, H. G. Improving parameter inference from FRAP data: an analysis motivated by pattern formation in the Drosophila wing disc. Bull. Math. Biol. 79 , 448–497 (2017).
Article MathSciNet CAS PubMed PubMed Central MATH Google Scholar
Beaudouin, J., Mora-Bermúdez, F., Klee, T., Daigle, N. & Ellenberg, J. Dissecting the contribution of diffusion and interactions to the mobility of nuclear proteins. Biophys. J. 90 , 1878–1894 (2006).
Schaff, J. C., Cowan, A. E., Loew, L. M. & Moraru, I. I. Virtual FRAP-an experiment-oriented simulation tool. Biophys. J. 96 , 30a (2009).
Blumenthal, D., Goldstien, L., Edidin, M. & Gheber, L. A. Universal approach to FRAP analysis of arbitrary bleaching patterns. Sci. Rep. 5 , 11655 (2015).
Müller, P., Rogers, K. W., Yu, S. R., Brand, M. & Schier, A. F. Morphogen transport. Development 140 , 1621–1638 (2013).
Article PubMed PubMed Central Google Scholar
Umulis, D. M. & Othmer, H. G. The importance of geometry in mathematical models of developing systems. Curr. Opin. Genet. Dev. 22 , 547–552 (2012).
Weiss, M. Challenges and artifacts in quantitative photobleaching experiments. Traffic 5 , 662–671 (2004).
Mazza, D., Cella, F., Vicidomini, G., Krol, S. & Diaspro, A. Role of three-dimensional bleach distribution in confocal and two-photon fluorescence recovery after photobleaching experiments. Appl. Opt. 46 , 7401–7411 (2007).
Machán, R., Foo, Y. H. & Wohland, T. On the equivalence of FCS and FRAP: simultaneous lipid membrane measurements. Biophys. J. 111 , 152–161 (2016).
Article ADS PubMed PubMed Central Google Scholar
Braga, J., Desterro, J. M. & Carmo-Fonseca, M. Intracellular macromolecular mobility measured by Fluorescence Recovery After Photobleaching with confocal laser scanning microscopes. Mol. Biol. Cell. 10 , 4749–4760 (2004).
Blischak, J. D., Davenport, E. R. & Wilson, G. A quick introduction to version control with Git and GitHub. PLoS. Comput. Biol. 12 , e1004668 (2016).
Müller, P. et al. Differential diffusivity of Nodal and Lefty underlies a reaction-diffusion patterning system. Science 336 , 721–724 (2012).
Sigaut, L., Ponce, M. L., Colman-Lerner, A. & Dawson, S. P. Optical techniques provide information on various effective diffusion coefficients in the presence of traps. Phys. Rev. E 82 , 051912 (2010).
Millman, K. J. & Aivazis, M. Python for scientists and engineers. Comput. Sci. Eng. 13 , 9–12 (2011).
Guyer, J. E., Wheeler, D. & Warren, J. A. FiPy: partial differential equations with Python. Comput. Sci. Eng. 11 , 6–15 (2009).
Article CAS Google Scholar
van der Walt, S. et al. scikit-image: Image processing in Python. PeerJ 2 , e453 (2014).
Jones, E. et al. SciPy: Open source scientific tools for Python (2001). http://www.scipy.org/
van der Walt, S., Colbert, C. & Varoquaux, G. The numpy array: a structure for efficient numerical computation. Comput. Sci. Eng. 13 , 22–30 (2011).
Hunter, J. D. Matplotlib: a 2D graphics environment. Comput. Sci. Eng. 9 , 90–95 (2007).
Lindblad, J. & Bengtsson, E. in Proceedings of the 12th Scandinavian Conference on Image Analysis (SCIA) , 264–271 (2001).
Waters, J. C. Accuracy and precision in quantitative fluorescence microscopy. J. Cell. Biol. 185 , 1135–1148 (2009).
Schwarzfischer, M. et al. in Proceedings of Microscopic Image Analysis with Applications in Biology (2011).
Gregor, T., Bialek, W., de Ruyter van Steveninck, R. R., Tank, D. W. & Wieschaus, E. F. Diffusion and scaling during early embryonic pattern formation. Proc. Natl Acad. Sci. USA 102 , 18403–18407 (2005).
Kicheva, A. et al. Kinetics of morphogen gradient formation. Science 5811 , 521–525 (2007).
Pomreinke, A. P. et al. Dynamics of BMP signaling and distribution during zebrafish dorsal-ventral patterning. eLife 6 , e25861 (2017).
Geuzaine, C. & Remacle, J.-F. Gmsh: a 3-D finite element mesh generator with built-in pre- and post-processing facilities. Int. J. Numer. Methods Eng. 79 , 1309–1331 (2009).
Article MathSciNet MATH Google Scholar
Si, H. Tetgen, a delaunay-based quality tetrahedral mesh generator. ACM Trans. Math. Softw. 41 , 11:1–11:36 (2015).
Sprague, B. L., Pego, R. L., Stavreva, D. A. & McNally, J. G. Analysis of binding reactions by fluorescence recovery after photobleaching. Biophys. J. 86 , 3473–3495 (2004).
Miura, K. Frapcalc . http://wiki.cmci.info/downloads/frap_analysis Accessed 12 Dec 2016 (2016).
Rapsomaniki, M. A. et al. EasyFRAP: an interactive, easy-to-use tool for qualitative and quantitative analysis of FRAP data. Bioinformatics 28 , 1800–1801 (2012).
Castle, B. T., Howard, S. A. & Odde, D. J. Assessment of transport mechanisms underlying the Bicoid morphogen gradient. Cell. Mol. Bioeng. 4 , 116–121 (2011).
Nelder, J. A. & Mead, R. A simplex method for function minimization. Comput. J. 7 , 308–313 (1965).
Nash, S. G. Newton-type minimization via the Lanczos method. SIAM J. Numer. Anal. 21 , 770–788 (1984).
Article ADS MathSciNet MATH Google Scholar
Nocedal, J. & Wright, S. J. Numerical optimization . 2nd edn, (Springer, NY, 2006).
MATH Google Scholar
Bläßle, A. & Müller, P. PyFDAP: automated analysis of fluorescence decay after photoconversion (FDAP) experiments. Bioinformatics 6 , 972–974 (2015).
Rogers, K. W., Bläßle, A., Schier, A. F. & Müller, P. Measuring protein stability in living zebrafish embryos using fluorescence decay after photoconversion (FDAP). J. Vis. Exp . 95 , e52266 (2015).
Mai, J. et al. Are assumptions about the model type necessary in reaction-diffusion modeling? A FRAP application. Biophys. J. 100 , 1178–1188 (2011).
Akaike, H. A new look at the statistical model identification. IEEE Trans. Autom. Control 19 , 716–723 (1974).
Student. The probable error of a mean. Biometrika 6 , 1–25 (1908).
Mann, H. B. & Whitney, D. R. On a test of whether one of two random variables is stochastically larger than the other. Ann. Math. Stat. 18 , 50–60 (1947).
Shapiro, S. S. & Wilk, M. B. An analysis of variance test for normality (complete samples). Biometrika 52 , 591–611 (1965).
Aaron, J. FRAP https://de.mathworks.com/matlabcentral/fileexchange/47327-frap-zip Accessed 12 Dec 2016 (2016).
Ulrich, M. et al. Tropical-parameter estimation and simulation of reaction-diffusion models based on spatio-temporal microscopy images. Bioinformatics 22 , 2709–2710 (2006).
Gurskaya, N. G. et al. Engineering of a monomeric green-to-red photoactivatable fluorescent protein induced by blue light. Nat. Biotechnol. 24 , 461–465 (2006).
Hrabe, J., Hrabetová, S. & Segeth, K. A model of effective diffusion and tortuosity in the extracellular space of the brain. Biophys. J. 87 , 1606–1617 (2004).
Tao, L. & Nicholson, C. Maximum geometrical hindrance to diffusion in brain extracellular space surrounding uniformly spaced convex cells. J. Theor. Biol. 229 , 59–68 (2004).
Article MathSciNet CAS PubMed Google Scholar
Novak, I. L., Kraikivski, P. & Slepchenko, B. M. Diffusion in cytoplasm: effects of excluded volume due to internal membranes and cytoskeletal structures. Biophys. J. 97 , 758–767 (2009).
Donovan, P., Chehreghanianzabi, Y., Rathinam, M. & Zustiak, S. P. Homogenization theory for the prediction of obstructed solute diffusivity in macromolecular solutions. PLoS ONE 11 , e0146093 (2016).
Periasamy, N. & Verkman, A. S. Analysis of fluorophore diffusion by continuous distributions of diffusion coefficients: application to photobleaching measurements of multicomponent and anomalous diffusion. Biophys. J. 75 , 557–567 (1998).
Xiong, R. et al. Sizing nanomaterials in bio-fluids by cFRAP enables protein aggregation measurements and diagnosis of bio-barrier permeability. Nat. Commun. 7 , 12982 (2016).
Höckendorf, B., Thumberger, T. & Wittbrodt, J. Quantitative analysis of embryogenesis: a perspective for light sheet microscopy. Dev. Cell. 23 , 1111–1120 (2012).
Article PubMed Google Scholar
Yu, S. R. et al. Fgf8 morphogen gradient forms by a source-sink mechanism with freely diffusing molecules. Nature 461 , 533–536 (2009).
Kimmel, C. B., Ballard, W. W., Kimmel, S. R., Ullmann, B. & Schilling, T. F. Stages of embryonic development of the zebrafish. Dev. Dyn. 203 , 253–310 (1995).
Welch, B. L. The generalisation of Student’s problems when several different population variances are involved. Biometrika 34 , 28–35 (1947).
MathSciNet CAS PubMed MATH Google Scholar
Razali, N. M. & Wah, Y. B. Power comparisons of Shapiro-Wilk, Kolmogorov-Smirnov, Lilliefors and Anderson-Darling tests. J. Stat. Model. Anal. 2 , 21–33 (2011).
Wilcoxon, F. Individual comparisons by ranking methods. Biom. Bull. 1 , 80–83 (1945).
Download references
Acknowledgements
We thank Ekkehard Kröwerath (University Hospital Tübingen, Centre for Dentistry, Oral Medicine, and Maxillofacial Surgery) for the preparation of plexiglass holes, Katherine Rogers and Fabian Fröhlich for discussions and suggestions, and Sarah Keim and Katherine Rogers for testing PyFRAP. This work was supported by the Emmy Noether Programme of the Deutsche Forschungsgemeinschaft, the Max Planck Society, and an ERC Starting Grant to P.M.
Author information
Theresa Braun
Present address: University of Konstanz, Universitätsstraße 10, 78457, Konstanz, Germany
Authors and Affiliations
Friedrich Miescher Laboratory of the Max Planck Society, Max-Planck-Ring 9, 72076, Tübingen, Germany
Alexander Bläßle, Gary Soh, Theresa Braun, David Mörsdorf, Hannes Preiß & Patrick Müller
Department of Organismic and Evolutionary Biology, Harvard University, 26 Oxford Street, Cambridge, MA, 02138, USA
Ben M. Jordan
You can also search for this author in PubMed Google Scholar
Contributions
A.B., B.M.J. and P.M. conceived the study. A.B. and P.M. designed the software and performed data analysis and simulations. A.B. implemented the software. G.S., T.B., D.M. and H.P. conducted experiments. A.B. and P.M. wrote the manuscript.
Corresponding author
Correspondence to Patrick Müller .
Ethics declarations
Competing interests.
The authors declare no competing interests.
Additional information
Publisher's note: Springer Nature remains neutral with regard to jurisdictional claims in published maps and institutional affiliations.
Electronic supplementary material
Supplementary information, rights and permissions.
Open Access This article is licensed under a Creative Commons Attribution 4.0 International License, which permits use, sharing, adaptation, distribution and reproduction in any medium or format, as long as you give appropriate credit to the original author(s) and the source, provide a link to the Creative Commons license, and indicate if changes were made. The images or other third party material in this article are included in the article’s Creative Commons license, unless indicated otherwise in a credit line to the material. If material is not included in the article’s Creative Commons license and your intended use is not permitted by statutory regulation or exceeds the permitted use, you will need to obtain permission directly from the copyright holder. To view a copy of this license, visit http://creativecommons.org/licenses/by/4.0/ .
Reprints and permissions
About this article
Cite this article.
Bläßle, A., Soh, G., Braun, T. et al. Quantitative diffusion measurements using the open-source software PyFRAP. Nat Commun 9 , 1582 (2018). https://doi.org/10.1038/s41467-018-03975-6
Download citation
Received : 24 September 2017
Accepted : 26 March 2018
Published : 20 April 2018
DOI : https://doi.org/10.1038/s41467-018-03975-6
Share this article
Anyone you share the following link with will be able to read this content:
Sorry, a shareable link is not currently available for this article.
Provided by the Springer Nature SharedIt content-sharing initiative
By submitting a comment you agree to abide by our Terms and Community Guidelines . If you find something abusive or that does not comply with our terms or guidelines please flag it as inappropriate.
Quick links
- Explore articles by subject
- Guide to authors
- Editorial policies
Sign up for the Nature Briefing newsletter — what matters in science, free to your inbox daily.


IMAGES
VIDEO
COMMENTS
Fluorescence recovery after photobleaching (FRAP) is a method for determining the kinetics of diffusion through tissue or cells. It is capable of quantifying the two-dimensional lateral diffusion of a molecularly thin film containing fluorescently labeled probes, or to examine single cells. This technique is very useful in biological studies of ...
Learn how to use FRAP, a technique for measuring molecular diffusion in cells or tissues, with this YouTube video. Watch the demonstration and explanation.
Fluorescence Recovery After Photobleaching (FRAP) has been considered the most widely applied method for observing translational diffusion processes of macromolecules. The resulting information can be used to determine kinetic properties, like the diffusion coefficient, mobile fraction, and transport rate of the fluorescently labeled molecules. FRAP employs irradiation of a fluorophore with a ...
Principle of FRAP. FRAP is a technique developed by Axelrod et al. (1976) as a method to study protein mobility in living cells. In FRAP, a specific area of a cell or tissue is photobleached by intense laser light, removing fluorescence from this area. This area is typically a cell membrane or area where diffusion occurs, such as the nucleus ...
Principle of FRAP experiment and example. (a) Scheme depicting the photobleaching and fluorescence recovery of a region of interest (ROI) within a focal adhesion (FA) in a cell.Before the bleach event, the fluorescent proteins are uniformly distributed within the structure and are in dynamic equilibrium, immediately after photobleaching, the equilibrium is lost and the fluorescence intensity ...
Fluorescence recovery after photobleaching (FRAP) is a standard technique used to study the diffusion properties of biomolecules in artificial or cell membranes. Photobleaching is defined as the process of irreversible transition of a fluorophore from an active to a dark state. This occurs typically in the presence of oxygen and when the ...
To derive quantitative results from such experiments, several parameters and controls need to be considered and utilised in the analysis. There are several FRAP-related methods that have been developed for specific applications and biological questions. FRAP is a versatile and popular method in modern biomedical research.
FRAP is a powerful technique that is widely used to study the dynamics of biomolecules within cells and other biological systems. The technique is based on the selective bleaching of a small region of a sample using a high-intensity laser, followed by the monitoring of fluorescence recovery in the bleached region.
Abstract. In this chapter, we review the main principles of the fluorescence recovery after photobleaching (FRAP) technique, and elaborate on how to correctly design a FRAP experiment. We also explain a set of analytical solutions used to quantify the different types of biological motions in living organisms. License Information.
Fluorescence recovery after photobleaching (FRAP) is a standard method to study the movement of protein in live cells, measured by the recovery of the fluorescence on the bleached locations. In this technique, a fluorescently tagged protein, usually GFP tagged, is permanently photobleached by applying a highly intense pulse of a laser beam.
Fluorescence Recovery After Photobleaching (FRAP), developed in the 1970s, is an essential tool for understanding molecular dynamics within a cell [1-3]. The typical setup for a FRAP experiment involves a fluorescent probe, a microscope, and some method of photobleaching . A portion of the domain where the molecule of interest is present is ...
This chapter describes the use of microscope-based fluorescence recovery after photobleaching (FRAP). To quantify the dynamics of proteins within a subcellular compartment, we first outline the general aspects of FRAP experiments and then provide a detailed protocol of how to measure and analyse the most important parameters of FRAP experiments such as mobile fraction and half-time of recovery.
The raw data are collected by a light sensor which measures the light through the microscope. These data are displayed on a computer screen and by the end of the experiment, a graph will be produced that looks similar to figure 4. Figure 4. Graphical presentation of data collected during a FRAP experiment.
The FRAP technique was first used to analyze the mobility of individual lipid molecules within a cell membrane.FRAP can also be used to study protein dynamics outside the membrane: a region of interest within the cytoplasm or cellular structures within the cell can be monitored.. Photobleaching experiments can be conducted with confocal laser scanning microscopes where the laser is used at ...
Instrumentation. The hardware required for FRAP experiments com-prises a fluorescence microscope equipped with light sources (arc lamps, LEDs or lasers) and filter sets for imaging as well as a light source for bleaching (typi-cally lasers) with some A={ method k_of} of selectively over bleach- {k_on +.
This video explains the concept of FRAP in details .Fluorescence recovery after photobleaching (FRAP) is a method for determining the kinetics of diffusion t...
Abstract. A fluorescence recovery after photobleaching (FRAP) experiment is a time series with controlled bleaching. The mobility of diffusing fluorescent molecules back into the bleach region of interest depends upon the nature of the cell, its local environment, the size and binding of molecules to membranes and organelles within the cell. A ...
The saga of fluorescence recovery after photobleaching (FRAP) illustrates how disparate technical developments impact science. Starting with the classic 1976 Axelrod et al. work in Biophysical Journal, FRAP (originally fluorescence photobleaching recovery) opened the door to extraction of quantitative information from photobleaching experiments, laying the experimental and theoretical ...
Fluorescence recovery after photobleaching (FRAP) is an imaging technique for the investigation of molecular dynamics and cellular events within living cells. The FRAP elucidates the dynamics and kinetics of the protein-protein interaction and cellular signaling. In a FRAP experiment, the laser beam is focused on a small area of cell membrane ...
Fluorescence recovery after photobleaching (FRAP) is a widely used technique for studying diffusion in biological tissues. Most of the existing approaches for the analysis of FRAP experiments assume isotropic diffusion, while only a few account for anisotropic diffusion. In fibrous tissues, such as articular cartilage, tendons and ligaments, diffusion, the main mechanism for molecular ...
The saga of fluorescence recovery after photobleaching (FRAP) illustrates how disparate technical developments impact science. Starting with the classic 1976 Axelrod et al. work in Biophysical Journal, FRAP (originally fluorescence photobleaching recovery) opened the door to extraction of quantitative information from photobleaching experiments, laying the experimental and theoretical ...
FRAP experiments can vary in intensity during the experiment due to, for example, an increase or decrease in extracellular volume fraction, due to molecules moving in and out of the imaging plane ...
Indeed, either of these effects can give rise to FRAP recoveries that cannot be explained by simple diffusion models. With the recent interest in specifically extracting binding information from FRAP experiments, however, it has become important to distinguish between the contribution of obstructed diffusion and the contribution of binding to a ...