Academia.edu no longer supports Internet Explorer.
To browse Academia.edu and the wider internet faster and more securely, please take a few seconds to upgrade your browser .
Enter the email address you signed up with and we'll email you a reset link.
- We're Hiring!
- Help Center
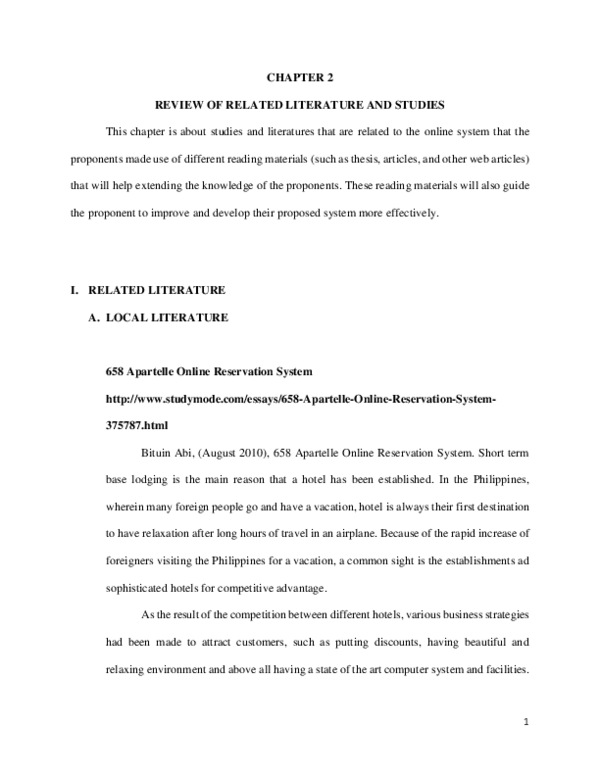
Download Free PDF

CHAPTER 2 REVIEW OF RELATED LITERATURE AND STUDIES Online Billing and Reservation System

This chapter is about studies and literature that are related to the online system that the proponents made use of different reading materials (such as thesis, articles, and other web articles) that will help extending the knowledge of the proponents. These reading materials will also guide the proponent to improve and develop their proposed system more effectively.
Related papers
Tribal Art 107, 2023
A bladed gunstock club sold in 2021 at an Auction in the Normandie without documented provenance is identified as having been collected in 1823 near Fort Lookout by Duke Paul Wilhelm of Württemberg. Another one, also without documented provenance, now in the Warnock Collection is identified as formerly having been in the Westerm Museum in Cincinnati, based on a drawing attributed to the Duke of Württemberg.
Lambert Academic Publication, 2024
This is only an attempt to understand what are various acts involved in spirit possession and exorcism. The analysis shows that most of these acts like prolonged silence, lewd and lurid words and actions, improper gestures, improper consumption of food and drink, facial expressions and so on are contrary to the mainstream social and cultural values and etiquette. Here one needs to be careful that these are not universal generalizations. Even though, author's attempt was to collect, put together and describe various acts from spirit possessions across the globe it is not a generalization study. Author completely believes that the interested scholars need to study spirit possession in its own local context. Moreover, Acts of spirit possession can only be analyzed and interpreted within its local cultural and social discourses.
Indo Nordic Authors’ Collective, 2024
The Metaphysics of Lotus Feet of KRISHNA - book
TRABALHOS COMPLETOS
Óðinn e os seus Guerreiros: Berserkir, Úlfheðnar e Einherjar, 2019
Óðinn e os seus Guerreiros: Berserkir, Úlfheðnar e Einherjar. Esse estudo procura mostrar que há evidencias de que o culto de Wodan/Woden/Óðinn pode ser muito mais antigo do que se pensa.
Sistem Hukum menurut Nur Khalifa Hazim, A.R Elham berarti susunan kesatuan dari bagian-bagin yang saling bergantung. Selain itu menurut Lili Rasyidi dan I.B Wyaasa Putra adalah Suatu kesatuan sitem yang tersusun atas integritas sebgai komponen sitem hukum hukum, yang masiang-masing memiliki fungsi tersendiri dan terikat dalam suatu kesatuan hubungan yang saling terkait, bergantung, mempengaruhi, bergerak dalamkesatuan proses sistem hukum untuk meweujudkan tujuan hukum. Selanjutnya menurut Lawrence M. Friedman, sistem hukum itu terdiri atas struktur, substansi, dan budaya hukum. Strukur merupakan halyang menyangkut lembaga-lembaga negara seperti eksekutif, legislatif, yudikatif. Kemudian Substansi Hukum itu pada hakekatnya mencangkup semeua peratura hukum baik tertulis maupun tidak tertulis seperti keputusan pengadilan yang dapat menjadi peraturan baru ataupun hukum baru. Disamping struktur dan substansi hukum ada pula budaya hukum yaitu merupakan sikap masyarakat, kepercayaan masyarkat, nilai-nilai masyarakat dan ide pengeharapan mereka terhadap hukum dan sistem hukum. Mengukur hukum sebagai suatu sitem, menurut Fuller yang dikutip oleh prof Satjipto Rahardjo harus meletakkan depan nilai-nilai sebagai berikut: 1. Harus ada peraturan terlebih dahulu, hal ini merupakan tidak ada tempat bagi keputusan secara ad hoc, atu tindakan yang berdifat arbiter. 2. Peraturan itu harus diumumkan secar layak kepada umum 3. Peraturan itu tidak boleh berlaku surut 4. Perumusan peraturan itu harus jelas dan terperinci serta dapat dimengerti oleh masyarakat. 5. Hukum tidak boleh meminta dijalankanya hal-hal yang tidak mungkin. 1 Tulisan ini dibuat untuk bahan bacaan dan untuk menambah wawasan dalam dunia hukum
Poteri al confine. Filarchi giudici re tra Impero romano e Barbaricum, 2023
Il volume propone una lettura in chiave socio-antropologica delle figure apicali del 'Barbaricum'. In uno scenario complesso e variegato, l’antico spazio dell’Impero romano risulta modellato in forme nuove proprio a seguito della caduta e della disgregazione dei confini politici (se non ancora culturali), epifenomeno di crisi interne e shock esogeni. Per queste e altre vie, un mondo dominato dall’unicità ‒ l’imperatore, il Dio dei cristiani ‒ sarebbe poi approdato alla molteplicità del Medioevo.
Hastings Race and Poverty Law Journal, 2019
Cities: The International Journal of Urban Policy and Planning, Vol. 29, Supplement 2, "Current Research on Cities", 2012
Religious Studies: An International Journal for the Philosophy of Religion, 2023
Journal of Innovative Research in Teacher Education, 2022
Journal of Proteome Research, 2014
Geophysical Research Letters, 2006
The American Journal of Cardiology, 2011
Energies, 2019
Praxis Filosófica, 2024
InVet, 2006
Journal of Experimental & Clinical Cancer Research, 2021
International Journal for Research in Applied Science and Engineering Technology - IJRASET, 2020
4º Congreso Internacional Ciudad y Territorio Virtual, Guadalajara-Jalisco, México, 1-5 Octubre 2007
- We're Hiring!
- Help Center
- Find new research papers in:
- Health Sciences
- Earth Sciences
- Cognitive Science
- Mathematics
- Computer Science
- Academia ©2024
An official website of the United States government
Official websites use .gov A .gov website belongs to an official government organization in the United States.
Secure .gov websites use HTTPS A lock ( Lock Locked padlock icon ) or https:// means you've safely connected to the .gov website. Share sensitive information only on official, secure websites.
- Publications
- Account settings
- Advanced Search
- Journal List

Forecasting resort hotel tourism demand using deep learning techniques – A systematic literature review
Noomesh dowlut, baby gobin-rahimbux.
- Author information
- Article notes
- Copyright and License information
Corresponding author. [email protected]
Received 2023 Jan 13; Revised 2023 Jul 15; Accepted 2023 Jul 17; Collection date 2023 Jul.
This is an open access article under the CC BY-NC-ND license (http://creativecommons.org/licenses/by-nc-nd/4.0/).
In the hospitality industry, revenue management is vital for the sustainability of the business. Powering this strategic concept is the occupancy rate (OR) forecast. Predicting occupancy of the hotel is essential for managers’ decision-making process as it gives an estimate of the future business performance. However, the fast-changing marketing demands in the tourism sector, boosted by the advent of online booking, generating accurate forecast figures is nowadays a tough task - needing personnel with advance technical skills and expensive software. The aim of the Systematic Literature review is to provide an insight of the use of Deep Learning techniques for OR prediction. The latest trends in this field over five years (from 2017 to 2022) are highlighted. Through this SRL, three research questions are answered. The questions are related to the variables, deep learning algorithms for prediction and the evaluation metrics used for evaluating the models developed. The Snowballing methodology was used to carry out the SLR. 50 papers were selected for the final analysis. Five categories of variables were identified. LSTM was found to be the most popular deep learning algorithm used to build prediction models. Seven performance metrics were found and among them MAPE was the most popular. To conclude it was found that the hybrid model, CNN-LSTM, to increase accuracy and required more investigation.
Keywords: Occupacy rate, Deep learning, Hospitality industry, LSTM
1. Introduction
Precise occupancy rate (OR) forecast is essential for the sustainability of hotels in this current complex, rapidly evolving market, restricted by many factors. Failing to identify those factors and the magnitude of their influence leads to wrong perception of the hotel performance and consequently inappropriate business decisions. OR is affected by many factors, such as the availability of safe infrastructures, the pricing strategy [ 1 ], political engagement for tourism development the air access [ 2 ] among others. Predicting the occupancy of a hotel is vital for the decision-making process of revenue managers. Managers can plan important activities, like stop sales, launch promotional offers or budgeting and even workforce management, with the ultimate goal of improving financial performance - maximise revenue and minimise costs. However, generating forecast reports with an acceptable accuracy is not a simple task and often requires specific data analytics expertise and statistical knowhow. However, generating forecast reports with an acceptable accuracy is not a simple task and often requires specific data analytics expertise and statistical knowhow. For instance, based on the traditional way of exploring historical data, the forecasting exercise carried out by the revenue managers is time consuming and tedious. Data needs to be extracted from numerous sources and the necessary analytical skills are required to come up with results. Despite having proven its reliability in the past, the conventional OR forecast process based on some customary set of data – commonly used across the hospitality industry, the inherent variability of nowadays market, gradually reduces its efficacy as a hotel performance-enhancing tool. The rapidly evolving market demands, galvanised by the rise of online booking platforms, has underlined the need for a real time, flexible forecasting tool to avoid missing out important demands trends and providing inconsistent forecast. Inaccurate OR forecast results in poor pricing strategy, which negatively impacts the financial performance of the hotel.
With the rise in adoption of Artificial Intelligence technologies, several machine learning (ML) techniques have been applied to improve the efficiency of OR forecasting. Most of the studies in this field follows the long-stranding quantitative approach where the prediction model is built on from training data on past/realised figures of several OR deterministic factors [ 3 ]. Although generating good forecast accuracy, they are still classic ML statistical models, requiring domain expert for feature identification to reduce the complexity of the data [ 4 ]. Another noticeable gap in ML approaches is the few inputs features they consider for prediction – feature engineering causing loss of granularity of data. While being a subdiscipline of ML, Deep learning (DL) differentiates itself with its hidden layer architecture, allowing it to learn high-level features in an incremental way [ 4 ]. The need for experts doing the tedious task of feature extraction is bypassed. The neural network concept of deep learning permits processing huge amounts of data and the integration of hyper-parameters - multiple features input [ 5 ].
The systematic literature review (SLR) provides the answer to a number of research questions through the thorough review of current literature using rigourous protocols for selection and assessment while minimizing bias. Throughout the academic realm, adopting a systematic approach to build up knowledge through literature is considered essential by many authors. Academic writers use techniques such as evidence-based software engineering [ 6 ] and information systems research [ 7 ] to ease their way through the vast volume of published papers while doing their research.
Hence this SLR attemps to answer three research questions related to forecasting occupation rate for resort hotels which are as follows.
What are the factors which mostly impact the occupancy rate of resort hotels?
Which deep learning algorithm gives best results in forecasting the hotel occupancy rate using the identified key factors?
How efficient is the deep learning algorithms?
The review is restricted to DL algorithms and to the best of the author's knowledge, at the time the systematic literature review was done, there was no similar reviews which discussed specifically hotel occupancy forecasting using deep learning algorithms. Section 2 presents the snowballing methodology [ 8 ] used. In Section 3 , the extraction and synthesis of data is discussed. The 50 selected papers are presented as well as their distribution over the year, and their catagorisation in terms of publication type, and variable dimensions discussed. Section 4 summarises the findings based on the research questions and section 5 further discusses the variables for prediction, the deep learning algorithms as well as their accuracy.
2. Methodology
For the requirement of this study, the snowballing systematic literature review (SLR) method [ 8 ] was preferred over a database search-based review. Snowballing technique, although rarely mentioned in the methodology sections [ 9 ], is one of the most commonly used approach by expert researchers to conduct their systematic reviews. In fact, snowballing accounts for nearly 51% of references identified in a systematic review [ 10 ] – a statistic attributed to its ability to stream down the number of studies to be assessed whilst still providing a good identification of relevant papers [ 11 ]. Snowballing can be summarised as an approach which uses the 1) lists of papers being referred in the paper to and 2) citations to the paper to systematically discover additional papers. The initial step of snowballing method is the identification of a start set of relevant papers from which backward and forward snowballing is iteratively conducted. During backward snowballing the list of a papers that have been referred to in the paper are analysed and meaningful papers are selected. Those referenced papers, despite relevant have manifestly been published at an earlier stage and to make sure that recent publications are not missed out, forward snowballing is an essential step. It implies identifying pertinent papers by searching through a list, which cited the initially considered paper. Fig. 1 depicts those different steps of the snowballing SLR carried out for this study.
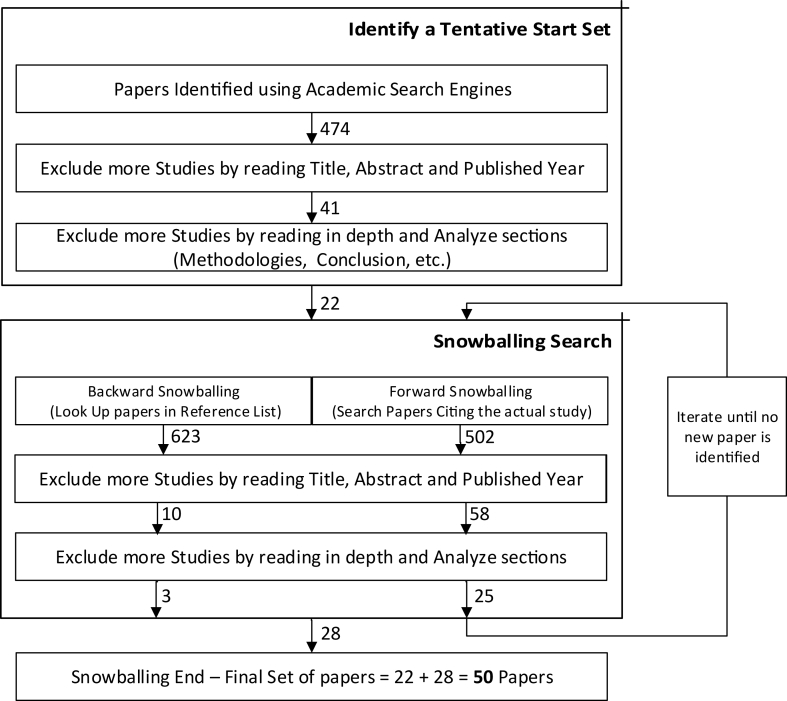
Paper selection process based on Wohlin's snowballing method [ 9 ].
The principle advantages of using snowballing over a database approach is that in addition to the fact the it is comparable to searching within multiple databases, its focus on cited or referenced papers, offers considerable reduction of noise. It is common practice that new studies cite articles from previous relevant works or from a SLR study in a specific area. Having an approach that centres on this aspect is not only convenient to avoid superfluous papers but enables the expansion of the existing literature review with new criteria. Moreover, the interdisciplinary characteristics of deep learning research area makes snowballing an ideal method for literature review since there no restriction to specific databases for searches, avoiding the challenging task for precise search string construction. The iterative search technique of snowballing allows the approximation of the search string but still caters for the discarding of irrelevant papers along the process. The studies were analysed by two researchers to ensure there is no bias in including as well as excluding any of those studies.
The subsections which follows, detail the different stages of the SLR.
2.1. Setting the research questions
The first step of the review was to define the research questions (RQ). The three RQs are.
RQ1 What are the factors which mostly impact the occupancy rate of resort hotels? Although algorithm such as deep learning does not require feature extraction, data set for the actual hotel occupancy prediction is mostly obtained from the hotel's property management system. As such, any external data that hypothetically has some influence on the occupancy rate needs to be identified to expose more features for the algorithm to learn from. Therefore, those forecast result, determining factors (determinants) should be studied and analysed in context of island resort hotels, before being used as input variables for the model. Grouping more variables, even though this might consequently decrease after feature engineering, allows the algorithm to extract insights from broader contextual frame.
RQ2 Which deep learning algorithm gives best results in forecasting the hotel occupancy rate using the identified key factors? Lara-Benítez et al. [ 12 ] recently reviewed several DL algorithms for forecasting and compared their accuracy. Despite demonstrating the ability of DL to have acceptable prediction result, the study only based itself on univariate principle of time series model, ignoring other features – hyper-parameters, which might have produced comparable outcomes. A more hotel specific DL approach is the work of Chang et al. [ 13 ], proposing the use of customer online reviews, extracting the sentiments and producing a prediction model. This study shows the possibility of integrating the new market behavioural features – in voluminous amount, to occupancy prediction model. Such capabilities make the DL algorithm an interesting area of study to investigate the possibilities to build accurate models for the multi factors OR prediction.
RQ3 How efficient is the algorithm derived in RQ2 as compared to existing algorithms? The final objective of this research is to identify which algorithm best predict the occupancy rate accurately and address any underlying issues related to forecasting. Different DL algorithms have been studied and tweaked in several researches so as to continuously improve the accuracy of the forecasting results. This vast canopy of DL techniques, on the other hand, creates a new conundrum, which is the choice of the most appropriate one within the actual context. As such it is essential that an evaluation metric is identified to compare the performance of the identified DL algorithm in RQ2 against existing benchmark algorithms.
2.2. Identification of the start set
A starting list of papers was retrieved by the conventional SLR search method where online databases or academic search engines (ASE) are queried with accurately formulated search strings. Academic search engines are commonly used to search for studies, as they prevent the risk of bias towards specific publishers [ 9 ]. However, these searchers often yield large number of papers – most of them were too broad and hence not useful. The search string “(“tourism demand” or “hotel occupancy rate”) and “deep learning” and forecast” returned 23,800 results. As such, well-known academic databases were opted to conduct the searches using a search string, derived from the RQs. Following the recommendations of Kitchenham et al. [ 14 ] the search strings were built by exploding the RQs according the PICOC criteria and using Boolean operators to link the individual terms. Table 1 illustrates the breakdown of the RQs using PICOC and using the “OR” operator to link synonyms and “AND” to concatenate the search terms. The results of searches from each academic database were scrutinising and an initial set of 200 papers was handpicked. This involved (1) browsing through the title of the paper and reading the abstract to see whether the paper is related to the set RQ (2) perusing the entire content of the selected papers. From this list, further selection criteria are applied as defined by the following inclusion and exclusion rules.
PICOC criteria used to search papers for the start set.
2.2.1. Inclusion an exclusion criteria
The last five years (2017–2022) is considered as the period of DL research explosion, owing to the advances in computational capabilities of ML-adapted hardware like GPU and TPU – TPUv2 released in 2017 and the extensive adoption of open-source ML tools such as Tensor-Flow and PyTorch. As such this SLR considers only papers with emphasizes on technology developed during this period. The criteria list is in Table 2 .
List of inclusion and exclusion criteria used to select the papers.
2.3. Start set
At the end of the selection process, 22 papers were included in our start set. The list of papers is found in Table 3 .
List of papers in the in initial start set.
2.4. Backward and forward snowballing
Backward snowballing was done on the references of each paper in the start set. The process, as illustrated in Fig. 1 , starts by the reviewing the title and the reference context of each referenced paper, which can be inferred through the analysis of the text surrounding the reference in the paper. This is key to determine the relevance of the study. Furthermore, in cases of generalised titles, the abstract and keywords were evaluated and the criteria defined in Table 2 were applied after a thorough read of the papers. After this initial filtering, the relevant paper was selected and this process was repeated on all newly detected papers, until no new papers are found. In our case, three backward snowballing iterations were required, resulting in the review of 623 referenced papers, from which 10 papers were relevant out of which only three papers were selected as they were not duplicates.
Forward snowballing on the papers was done by going through the citation lists. Google Scholar was used to identify each paper's citations. The title, abstract, keywords and reference context of each study were reviewed and the selected papers were included in the start set. The process was reiterated until no new papers were revealed. During the three forward snowballing iterations, 502 papers were reviewed, of which, 58 studies were identified and only 25 papers were considered after filtering through the inclusion/exclusion criteria.
2.5. Final papers set codification
In all 28 relevant papers were identified and added to the start set, making a final pool of 50 papers to conduct the review. Once downloaded for in-depth analysis, these papers are codified using their identification process as suffix followed by an ordered rank of iteration – e.g. F3_01 is the first paper found from the third iteration of forward snowballing. Codification facilitates the recognition of any overlapping of papers and allows the quick retrieval/referencing of the papers from the final set, when carrying out the review. The final set of the codified papers is listed in Table 3 , Table 4 .
Papers identified and selected during forward and backward snowballing.
3. Data extraction and synthesis
Extracting relevant information from the identified set of eligible studies is a major step in the SLR process. The grouping and summarisation of those key data is crucial for the synthesis of the studies which when organised following some pre-established guidelines, facilitates the drawing informed conclusions. In this SLR, based on the research questions, a data extraction form was created. The data extracted are listed in Table 5 .
Data Extraction form.
For the qualitative and quantitative analysis of the studies, this review considered a combination of the constant comparison method (CCM) and summarisation of the extracted data for the data synthesis approach. CCM, which is the core component of grounded theory [ 64 ], is the method of arranging pieces of raw data into groups based on their attributes and organising those group in a structured way to drive conclusions. CCM focuses on the idenfication of similarities and differences between extracted data, and this property has encouraged the intensive use CCM for qualitative analysis [ 65 ].
3.1. Yearly distribution
The distribution of the primary studies from 2017 – the start of DL era, until 2022 in Fig. 2 . The number of published papers in this domain follows a constant increase per year - approximately doubling each year, with the largest number of publications in 2021 (23 studies). The seven published studies in the early months of 2022 shows the rising trend of DL oriented papers in the tourism demand prediction field.
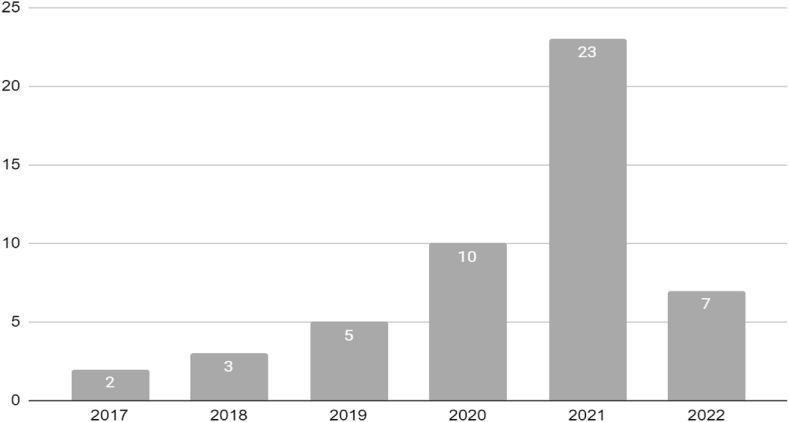
Distribution of the 50 selected studies across the last 5 years.
3.2. Publication type and database
Most of the publications identified in this review originate from journal articles published from a several domains such as Hospitality, Electronics & Communications, Information Technology, etc. Fig. 3 shows the ratio of the two types of publications domination this SLR, where only 13 studies (26%) were from conference papers. Popular databases were consulted for the identifications of those studies and the bulk of them were obtained from Science Direct (14 studies) and IEEE Xplore (12 studies), followed by MDPI with eight studies. Two studies were obtained from each of Institute of Physics, Springer Link and SSRN databases, whereas each of ACM Digital Library, CORE, Wiley Online Library, Hindawi, Taylor & Francis, HRCAK revealed one study.
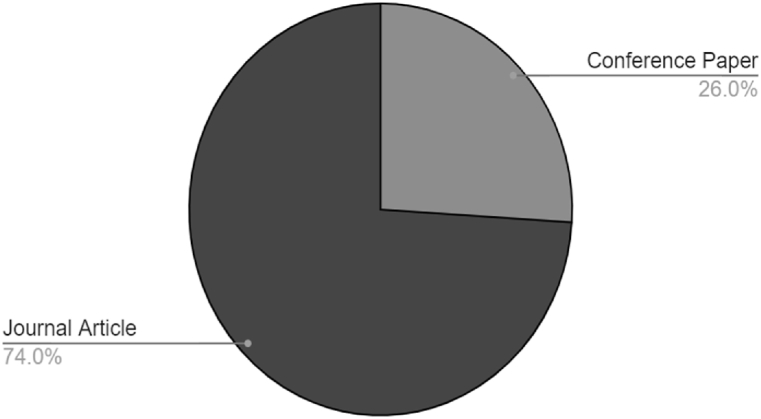
Papers analysed per publication type.
3.3. Factors/variables dimensions
The 50 selected studies were primarily categorised based on the number of input variables that are used for training DL models. As listed in Table 6 , the majority of the papers rely on a multivariate dataset to feed the DL algorithms. Although the others are based on a single feature, the input dimension of some - especially those based on time series methods, is consequently increased when adding a defined time lag. The wide use of multivariate dimensions in those DL-oriented studies, demonstrate the suitability of DL approaches to thrive with not only large volumes of data but also high dimensions and complexity (Mahapatra, 2019 [ 4 ]).
Variable Dimension Category of Selected studies.
4. Findings
The results of the review as well as the answers related to the research questions are presented in this section.
4.1. Types of variables
This section answers RQ1 . An indepth analysis of the papers showed that the selection of input variables was mainly determined by the availability of the data. Forecasting algorithms commonly use time series approaches and as such, they often rely of the lag order of historical data to derive predictions. Past statistical data is in many cases the only input of the forecasting model (12 studies) but are also used with additional input variables with the goal to increase the prediction efficiency. In this SLR, those factors are classified into different input type groups as per their use in the analysed papers. This distribution is summarised in Table 7 .
Input variables type.
4.1.1. Historical data
In the forecasting and prediction studies, historical data refers to the values of target/prediction variable at different point in time. Tourism demand refers to the number of tourists visiting a region, country or a hospitality establishment but depending on the context, this can be viewed and calculated differently. For instance, in papers SD_01 [ 16 ], SD_06 [ 21 ], SD_12 [ 18 ] daily volume of people visiting attractions as a demand variable is used whereas in SD_18 [ 32 ], SD_20 [ 34 ], B3_01 [ 62 ], F1_12 [ 48 ], F1_14 [ 50 ] the monthly tourist arrivals is considered. Whether it is hotel passenger flow discussed in F1_02 [ 38 ] or daily arrivals in a city discussed in F1_16 [ 3 ], these historical data points, used in time series techniques, are generated by the continuous recording of the values over the same source over a time interval, to interpret the temporal pattern. From all the papers covered in this SLR, the historical data is the one of the most used input variables for the DL algorithms (34 studies).
4.1.2. Causal econometric variable
The causal econometric variable input type englobes all the economic factors affect the value of the targeted variable (directly or indirectly). In the tourism demand forecasting domain, these variables include factors such as the destination country GDP, the current exchange rate, public expenditure or even oil prices as shown in Table 8 . Depending on the availability of the data and algorithm used, some papers make use of only the causal econometric variable to forecast tourism demand (8 studies) whereas in some cases, they are combined other variables types such as historical data (5 studies). In F3_01 [ 63 ] it is seen that the adding the price index as variable, which is the best available rate of a hotel, increases the forecasting performance OR prediction model. Table 7 lists all the causal econometric variables identified in this review.
Causal econometrics variables.
4.1.3. Extrinsic variables
Non-economic external factors that correlates to the tourism demands are grouped under this category and are mostly used as supplementary variables to increase the precision of the forecast. This variable type groups external determinants such as seasonal component (SD_13 [ 28 ]), weather/climate data (SD_11 [ 26 ]), events in the hotel area (SD_09 [ 24 ]), website reviews (F2_01 [ 58 ]) and sentiment index analysis (F2_02 [ 59 ]). Kim et al. (2021) also applied external factors such as political index, disease situations such as COVID in addition to the historical data to build the forecasting mode in paper SD_13 [ 28 ]. The 10 studies that includes external variables, shows that they are only used as add-ons features with other variable type in attempts to improve the forecast accuracy. As depicted in Table 9 , depending on the forecasting conditions/location, several extrinsic variables exist in literature, with some common among those reviewed in this tourism demand SL.
External/extrinsic variables.
4.1.4. Search intensity index (SII) data
SII can be classified as part of the external variable types but with the rising trend of SII in recent studies, it was necessary to single out papers that emphasise on the volume of related search words of a specific area, as an element for prediction. With the easy access to network-based information search platforms such as google, Bing or Baidu, massive data are generated by consumers, looking to make informed decisions as discussed in SD_07 [ 22 ]. It was seen in paper F1_09 [ 45 ], that in the travel industry, these data directly reflect the objective and intents of potential tourists and hence a highly indicative feature of tourism demand (F1_03 [ 39 ]). For instance, in SD_11 [ 26 ], the authors demonstrated the increase in their model's performance by adding search engine data to the historical data - showing SII's importance in tourism forecasting. In paper SD_13 [ 28 ] keywords representing the attractions of Korean culture, was gathered from google trend and Baidu, to be used as an external factor together with other determinants. Those keywords, although varying from studies/tourist destinations, can be categorised based on commonly searched criteria of a person while to planning his travel or stay. In papers F1_03 [ 39 ] and F1_19 [ 54 ] forecasting frameworks have been built for predicting tourism influx, by including search words as variables, categorised into tourism planning criteria such as Dining, Lodging, Shopping, Transportation, Tours, and Recreation. Likewise, 20 studies in this review uses tourism specific search words, related to the location of the hotel, resort, city or country to come up with as SII metric, an input to the forecasting model. Whether used as the only input (7 studies) or associated with other variable types (13 studies), SII represents more than one third of the total studies in this SLR. Table 10 summarises the various keywords/category used by those papers as a valuable external factor.
Search index intensity - tourism category.
4.1.5. Geographic data
The country of origin of the travelling tourists is an important determinant of the destination choice, especially for resort hotels where access is mostly through air. Likewise, the destination's location, region or area, together with external factors such as air travel prices highly contributes to the tourism demand outcome. Based on this variable, Li et al. in paper SD_03 [ 18 ] and L. Huang et al. in paper SD_08 [ 23 ], proposed a novel deep learning forecasting method using the special temporal effect generated by multi-destination travels, sharing common regional resources. Following the same principle, out of the 50 papers in the SLR, five studies used geographic data as additional variable for tourism demand forecasting. In paper F1_02 [ 38 ], the authors make use of the geographic location of different hotels as a determining factor to predict each one's tourism demand. In paper F1_01 [ 37 ], arrivals from the three origin countries were considered as input variable and additionally, as in paper SD_22 [ 36 ], tourists from cities considered as main market source were also selected.

4.2. Deep learning algorithms
This section presents the findings for RQ2 which is about the deep learning algorithm developed for room occupancy predication and their performance. Several deep learning algorithms were employed for the tourism demand forecast across the 50 selected studies with many having more than one technique – sometimes used in a hybrid model, combined or compared for best performance. These algorithms are classified in three distinctive models comprising of four Deep Neural Networks (DNN) – variants or enhancements, 3 Recurrent Neural Network (RNN) a-one Stack AutoEncoder as summarised in Table 11 . It shows that RNN is the most popular algorithm, being used in 30 studies (60%). Long short-term memory (LSTM) is the most utilized model (24 studies). DNN makes up to 26% of the studies (13 papers) whereas the hybrid models, combining those mentioned algorithms, is used in other six papers.
Deep learning algorithms.
In the following subsections, the different DL algorithms identified in this review are introduced, including the methodologies of the hybrid models.
4.2.1. Deep neural network
An Artificial Neural Network (ANN) which forms the basis of a deep neural network (DNN), consist of multiple neuron nodes, stacked into layers - input and output, connected by hidden layers. Data is taken by the neuron, and simple mathematical operations are performed. The result, determined by an activation function, is passed to neurons in the next layer until the output node is reached. DNN is based on the same principle and is defined as a stack of multi-neural – multilayer perceptron. In the following subsections, four DNNs identified are discussed.
4.2.1.1. Feed-forward neural network (FF-DNN)
The feed-forward networks is one of the most common type of DNN. It is defined as an ANN with a one-way, cycle less flow of information between the nodes. Data always moves in one direction and never backwards, although it might flow through a hidden layer [ 66 ]. FF-DNN operates by having the series of input entering the layer, multiplied by initial weights and added together to feed the network with the initial weighted input values. A specific threshold value is predefined and any weighted value above that threshold will have an output of 1 whereas the opposite will result in a value of −1.
Machine learning is achieved in FFN model through a delta rule property whereby the algorithm compares the value of each output node against the actual one. The neural network then adjusts its weights through training and learning process - known as gradient descent, to produce more accurate output values. The training algorithm in a multi-layered architecture FF-DNN is defined as back-propagation (BP). In a multi-layered perceptron, the weights of each hidden layer in the network is backward, sequentially adjusted with respect to the margin of error of the output value in the final layer.
In this review, 9 papers were identified using FF-DNN. Paper F1_14 [ 50 ] presents an enhanced BP algorithm such as particle swarm optimization to increase the forecasting accuracy. Paper B2_01 [ 57 ] considers a back propagation deep neural network to forecast China's domestic tourism by the following two variables 1) time to travel 2) the number of visitors. The novelty of the proposed model is the standardization of raw irregular data series, by the use of grey model algorithm, before running those an input through the several hidden layers of the DNN. In SD_15 [ 13 ], in combination with a pre-feature selection algorithm, a deep learning model, with 10 hidden layers was used to process 69 economic causal variables for the forecasting of visitors in Taiwan. The model in SD_16 [ 30 ] consisting of 31 hidden neurons, looked into the non-linear relationship and high fluctuation in seasonal tourism data. The monthly tourist arrivals in Indonesia is thus predicted. In SD_10 [ 25 ], the authors introduced a neural networks-based model to handle large Vector Auto-Regressions (VARs) - algorithm selected to predict occupancy rate of hotels. The neural network handles the curse of dimensionality challenge when processing nonlinear compressed VARS as input for the forecasting model. In SD_17 [ 31 ] a tourism-package sales forecasting model is proposed while in SD_20 [ 34 ] and F1_03 [ 39 ] the authors predict the tourism arrivals in Vietnam and Macau respectively, by using past data as input to a feed forward neural network. In SD_19 [ 33 ], Huang et al. compare several algorithms built on FF-DNN and found that variable weight combination prediction model based on Gradient Boosted Decision Tree (GBDT), produced the best performance. The authors in F1_14 [ 50 ] stated that the performance of the BPNN in predicting the monthly tourism demand was improved using chaotic particle swarm optimization (ICPSO). ICPSO was used to initialize the model with the optimum connection weights and thresholds.
4.2.1.2. Convolution neural network (CNN)
CNN is another prominent variant of DNN proposed by LeCun et al. [ 67 ] which although is a feed forward neural network works differently in terms of neuron activation. In CNN, multiple convolution operations are executed first before a neuron is activated for feature extraction or classification. Besides convolution, CNN employs several stages of operations such pooling and nonlinearity activation. It shares weights between local connection and apply filter elements to extract feature from the complex original data to build complete, dense feature vectors. This capability of CNN has seen its rise in use for high complexity feature detection such as image processing and computer vision.
This feature extraction ability of CNN has prompted its solicitation in several tourism forecasting studies, especially in time series dataset. In this review, four studies have been selected which applied CNN as a prediction algorithm and 3 of those are only used in hybrid models – associated with other models. CNN has had a low use in forecasting due to its low performance on one dimensional (1-d) data such as tourism demand. However, recently, with the key technique of transforming 1-d data to 2-d image data, researchers can now reap the full potential of CNN, giving rise to a new horizon of extracting embedded features from complex time-series dataset to boost forecasting accuracy [ 26 ]. SD_13 [ 28 ] uses CNN uniquely as a forecasting model. The authors extracted spatiotemporal patterns from time-series by using a 1-d convolutional neural network. A multi-head attention model was added to the model due and this resulted to better results than common time series techniques. The attention mechanism derived the correlations between the input variables.
4.2.1.3. DNN enhanced
Out of the 13 studies categorised under DNN in this review, 3 of them employs the basic DNN methods but with additional enhancement techniques which have been reported to increase the accuracy and performance. In F1_12 [ 48 ], the authors use the Fourier series technique. The residuals of the deep learning algorithm were modified to enhance the precision of the forecast output. Other improvement tools such as the Ensemble discussed in paper F1_19 [ 54 ] and Decomposition discussed in paper SD_04 [ 19 ] reduce the processing load by running DLs on sub-datasets and combining individuals forecast outputs to a final, more precise prediction.
4.2.1.4. Recurrent neural network (RNN)
Recurrent Neural Network (RNN) is another type of deep learning model where the prediction algorithm is based on sequential, time stamp data for the output prediction. Similar to other deep learning models such as Feed Forward Network, RNN has to be trained, learn through backpropagation and weight adjustments. RNN differentiates from the other DL models with a “memory” feature where current input and subsequently the output, is influenced by the prior inputs for each learning cycle. The final output in RNN is therefore dependent on all the prior elements with the sequence of inputs. Although RNN is highly regarded as a major tool for time series prediction, in this review, no studies rely of RNN base algorithm for tourism demand forecasts due to a key flaw. The drawback of this simple RNN architecture is the exponential growing or decaying of the gradient vector - learning function, with long data sequences [ 68 ]. Hence, classic RNN might sometimes omit long distance correlation in a sequence, hindering the performance and accuracy of the predictions. To address this issue, variants of the RNN have been developed.
4.2.1.5. Long Short-term memory (LSTM)
Long Short-term Memory Network (LSTM) proposed by Hochreiter et al. [ 69 ], as compared to RNN, has an additional separate memory cell, enabling the management its content, i.e., updating or exposing its content depending on the necessity. The input gate, the forget gate, and the output gate - components that distinguish LSTM from classic RNN. These gates control the erasure, update and exposure of the internal memory state allows LSTM models to analyze previous data and decisions, making new informed decisions from that. This characteristic of LSTMs makes the algorithm suitable to make informed predictions when being fed with time series data and find trends in the data to predict future trends - traits appropriate for tourist demand prediction. Such is the popularity of LSTM in forecasting, that 64% of the studies employs the algorithm, whether in a unique, hybrid or enhanced model.
Most of LSTM models in this study follow the basic algorithm to capture the nonlinear relationship in the time series dataset of tourism demands using at times only the past historical data to predict the future demand. In papers F1_06 [ 42 ], F1_09 [ 45 ] and F1_15 [ 51 ], the models were used to forecast the future demands based on sequential online search data and past tourism arrivals. The variables (univariate/multivariate) may be of various types such as econometrical in seen is paper F1_13 [ 49 ] or geographical in paper F1_20 [ 55 ] but the principle of finding patterns in timestamp data remains the same.
Enhancement to the popular LSTM models consist primarily of optimizing the quality, quantity of the input variables to boost performance. For instance, in paper F1_05 [ 41 ] , the random forest algorithm was used to reduce the number of search key words – focusing only on those highly correlated to tourism, thus reducing computing time. Papers SD_09 [ 24 ] and F2_01 [ 58 ] present how K-means clustering was used to group similar attributes together so as to decrease the number of features fed to the LSTM. Same grouping of similar features to a singular variable is also explored in paper SD_04 [ 19 ]. The authors of paper SD_02 [ 17 ] integrate an attention mechanism in the LSTM structure which filters out the most influential factors and the appropriate lag order, by assigning attention scores to them. Only the best variables are selected to feed in the LSTM for prediction. Paper F1_17 [ 52 ] focusses on the optimization of the initial parameter setup using Adam optimization method while paper F2_04 [ 61 ] combined the LSTM forecast output with that of the traditional autoregressive model as an ensemble for an improved prediction performance.
4.2.1.6. Bidirectional Long Short-term memory (BiLSTM)
BiLSTM is an enhancement of the LSTM algorithm since it allows the information to be processed in a sequential way. Architecturally, the only difference is the addition of a backward LSTM layers, enabling the output layer to processed simultaneous forward and backward input [ 70 ]. The training of data in a bidirectional way, i.e. processing twice the same input, hence improving the performance of LSTM model to. 3 papers were identified on using the BiLSTM algorithm for the forecasting of tourism demand.
Authors of paper SD_05 [ 20 ] improved the forecasting performance of the BiLSTM model by optimizing the hyper-parameters using Bayesian optimization. In the study, several benchmark models were compared (LSTM, Support Vector Machine) to BiLSTM. Papers F1_17 [ 52 ] and F1_11 [ 47 ], proposed the optimization of the BiLSTM model by decomposing the data into trend, seasonality, and residual before using them as input variables while the former used the Adam Optimization algorithm with adaptive learning rate to boost the initial parameters setup.
4.2.1.7. Gate recurrent unit (GRU)
Another variant RNN model identified in this review is the Gated Recurrent Unit (GRU) network which is basically a simplified LSTM model. GRU as proposed by Cho et al. [ 70 ], uses only with two gates but still carries the key function of adaptively formulating the dependencies of each data cycle at different time scales. The structural difference with GRU is the update gate, which replaced the functionalities of the input and forget gate of the LSTM. The update gate eliminates the cell state feature and uses hidden state to pass on information. Despite having a less complicated structure, GRU still delivers high performance for time series forecasting requiring “memory” to process past data. 3 papers were identified in this study using GRU to predict tourism demand.
In paper SD_18 [ 32 ], the authors used plain GRU model to compare the performance of the model developed to forecast Morocco's tourist demand against an LSTM model. Authors of papers F1_17 [ 52 ] and F2_03 [ 60 ] used improvement techniques over GRU to increase the forecasting accuracy. Zhang et al. used a variation mode decomposition method to break the historical data into different frequency modes before to be used as different time series variables.
4.2.1.8. Stacked auto encoder
Stacked auto-encoder (SAE) network is another type of fully connected DNN [ 71 ] and is used in one study in this tourism demand forecasting techniques review. An auto-encoder is a single-hidden layered feed forward network where the output contributes to the reconstruction of the input. It fits an identity function between the input and the output layer vectors. The change from the input to the hidden layer is non linear.
SAE, is a combined structure of several auto-encoders - deep encoders, where the hidden layer output on the previous auto-encoder is the input for the next auto-encoder. This architecture allows the SAE to hierarchically learn key representations of complex, dense dataset and eliminates irrelevant features. SAE is used in paper SD_07 [ 22 ] for automatic feature extraction and then used an RNN algorithm to predict the tourism demand.
4.2.1.9. Hybrid models
Hybrid models refers to those forecasting techniques identified in this review, where multiple DL algorithms are sequentially combined to improve the generalised performance. This is achieved by exploiting the strong characteristics of the individual algorithms and splitting the forecasting tasks into different operations, best fitted to those algorithms. Out of the 6 studies using hybrid models, half of those use the combination of CNN and LSTM for prediction. CNN is best suited for feature extraction, allowing the LSTM for emphasis only on its strong attribute – forecasting tourism demand.
CNN-LSTM is used in papers SD_01 [ 16 ], SD_03 [ 18 ], SD_06 [ 21 ] and F1_10 [ 37 ] to build the prediction model where the features extraction is handled by the CNN, allowing the LSTM algorithm learn the temporal dependence of the sequence of the extracted features. In F1_16 [ 3 ] and SD_06 [ 21 ] the SARIMA algorithm is used to capture linear features and processes the seasonality and trends, before running the consequent residual through LSTM/CNN-LSTM algorithm for the final forecast values. Optimization techniques are used in papers B1_01 [ 56 ]. F1_10 [ 46 ] where the former uses Principal Component Analysis (PCA) to decrease the larger dataset's dimensions and de-correlate input data, while the latter optimises the number of neurons to be used by the CNN-LSTM model, by a generic algorithm. In paper F2_04 [ 61 ], the hybrid GM-LSTM model was used. The overall trend of the tourism demand was captured by the first order grey model. The LSTM explained the nonlinear residual of the tourists flow fluctuation.
4.3. Performance metrics and evaluation of the identified DL algorithms
In the quest of finding the best forecasting algorithm for tourism demand, it is essential that the performance evaluation be based on some common/standard criteria among the studies is this review. Measuring the error margin of the prediction is the most common way in determining the performance of a model and multiple error criteria, found in literature, has been adopted in the reviewed papers. Fig. 4 summarises the performances measures identified and their usage distribution and Table 12 gives a brief description of each measure.
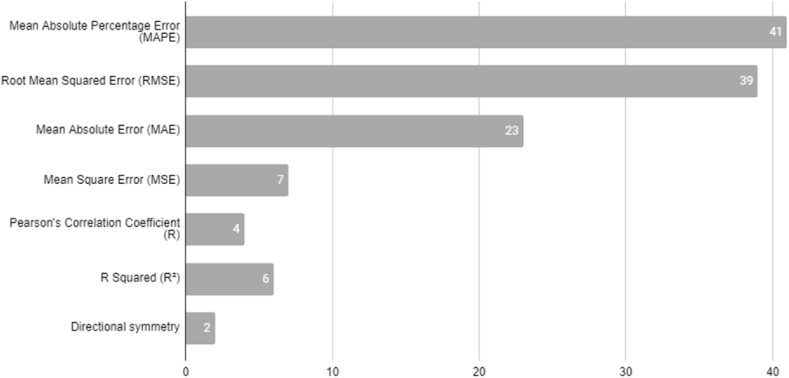
Distribution of the identified studies per Performance Measures.
78% of the publications have evaluated the performances against benchmarked DL models, validating the results against different proven measures. The most popular performance metrics, found in this review are the Mean Absolute Percentage Error (MAPE) and Root Mean Square Error (RMSE), followed by the Mean Absolute Error (MAE).
5. Discussion
5.1. variables for prediction.
With the advent of online booking, review and destination search, accurate tourism demand prediction is nowadays bigger than a statistical dilemma and relying solely on historical data to observe trend is almost irrelevant as revealed in this review, whereby 75% of the studies considers other factors for improved forecast. Single variable models rely on the concept of extracting temporal features [ 3 , 18 , 26 , 42 ] but tourism demands have been reported in various studies to be impacted by several factors such as SII Data [ 28 ], geography and many other causal econometric Variable - countries GDP, Oil prices [ 31 ], hotel reviews [ 37 ].Therefore, to continue building up on the performance of tourism forecasting algorithms, recent studies have demonstrated the importance of incorporating multiple determinants in their proposed models.
Tourist demand forecasting has been a classical time series statistical algorithm and as such, past tourist arrival data with a defined lag order is the base variable for any proposed model. Other from this popular time stamped historical data (35 papers), the next major variable that has a high weightage of tourism is the search intensity index ( SII ). More than a third (19) of the studies in the review has adopted SII data for forecasting and more than a half (11) of these are from the last 2 years.
The rise in researches regarding impact of data from search engines comes again with the explosion of online bookings/hotel reviews, fast changing the marketing demand of the hospitality industry. Quick access to hotel information – location, features, offers and critics, from search engines has made those platforms such as Google and Baidu, essential for tourists in planning their vacation. Reaping the best of that information has been such a success that SII has been acknowledged in several studies as a potential indicator of tourists preferred destination. SII has been so popular is recent tourism demand forecasting studies, that some researchers have considered SII as sole variable for demand prediction [ 17 , 23 , 42 , 44 , 45 ], skipping the hurdle of feature engineering external factors. The convincing performance results of SII based models, has encouraged to further improve the principle by using terminal variable i.e. the device on which searches were made - adding the element of portability vs user-friendliness to search queries [ 44 ] .
SII data do pose some constraints for its adoption in a forecasting model and the main barrier is the choice of the key words as input data, requiring feature engineering and domain expertise to select the deterministic keys from thousands of search queries. In addition, for time series forecasting, the lag/lead relationships between the data are potential prediction determinants but only a few studies, mostly based Pearson correlation coefficients or the Granger causality test has venture into lag-order selection methodology. Despite those barriers, the advent of deep learning techniques such as CNN [ 21 ] - for efficient feature extraction and LSTM [ 46 ] - for learning long-term dependencies, there is an increasing number of studies having SII as root component for tourism forecasting.
As reviewed in this study, there are various other extrinsic forecasting determinants such as seasonal effects political index or climate variation [ 18 , 28 ], which unlike econometrics variables have direct effect on tourism demands. Considering all of those features in literature, is not ideal as some of these data may not be available and result into a sparse dataset. Moreover, depending on the context e.g. island tropical resort, some of those features may not be valid or bear high correlation coefficient value with others. For instance, the tropical temperature of in tropical islands might have the four-season impact like European countries. Econometrics factors like oil prices is considered a major determinant of tourism especially for island resorts where most of tourist arrivals are through air travel. On the other hand, its high correlation with other factors such air ticket price might require its omission in the forecasting algorithm if those other features are considered. GDP or CPI are metrics of tourists’ countries of origin and can is considered if prediction is segmented by market [ 13 , 20 , 30 ].
Finally, selecting a set of determinants from those found in literature, the domain, context and availability of the data should be considered. Validation of those variables through domain experts – revenue managers, marketing managers, hoteliers is essential to pin out repetitive/correlated features or even uncover new features unique to the resort's context.
5.2. Deep learning models
There has been significant improvement of the performance and accuracy of the classical forecasting techniques using DL models. Previous researches on tourism demand forecasting focussed mainly on linear models. These models relied mostly economonic variables as well as stable time series data. Actual tourism prediction bears nonlinear characteristics with many impacting factors and with traditional machine learning, methods it is very difficult to effective simulate the demand trend. Classical methods were not able to deal with increasing data from online search, reviews and so on, due to the inability to automatically extract the features.
Early deep learning architecture such as the FF-DNN – discussed earlier, has helped in addressing the setbacks of classical methods by its layers of hidden neutrals handling the non-linearity of the data. Chang et al. [ 13 ] showed that deep neural network facilitates the extraction of key features as compared to the classical methods which require domain experts and supervised feature engineering. The inbuilt feature engineering capability of multi-layer deep neural networks the ability to handle more than two non-linear processes simultaneously has encouraged further researches in DNN for tourism forecasting. As seen in this review, CNN, a variant of the DNN, can formulate the relationship between timestamped data in a time series analysis. In search on increasing the accuracy of FF-DNN, several enhancement techniques were pointed out this review such as decomposition and ensemble but with basic DNN main disadvantage remains – its inefficiency of handling long-term dependencies.
5.2.1. RNN vs FF-DNN
RNN and specially its improved derivatives - LSTM, BiLSTM and GRU has been very popular in recent time-series analysis studies, owing to its key “memory” feature. In addition to the advantages that its DNN architecture offers, the ability of LSTM to use sequence of data recurrently as input enabled the extraction of contextual information embedded in the time-series structure of most tourism forecasting dataset. Such has been the performance of RNN, LSTM that many of the recent studies has adopted these algorithms for tourism forecasting. As demonstrated in Fig. 5 , LSTM is not only the most utilized model in this review but also the most adopted since the last 2 years.
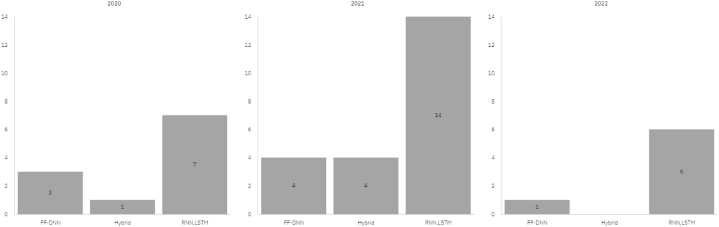
No. Studies by algorithms by year.
Recurrent-based networks has been providing excellent performance in tourism demand forecasting but the constant need to increase the accuracy has revealed some of their limitations. Although RNNs’ key property is the extraction of temporal features, they are structured to handle these timestamped dependencies for single variables while tourism demand prediction is affected by several factors. Therefore, recurrent models are limited when it comes to interpreting the correlation among the different external variables which may have embedded contextual value influencing the predicted value. Furthermore, the autoregressive structure of RNN and LSTM, where the predicted value of each sequence is used back as input, causes a decrease in long-term accuracy with nonlinear trend such as tourism forecasting. As a matter of fact, long-term forecasting in the hospitality industry is an essential information to help stakeholders plan and organize resources on monthly or even yearly basis. From a hotel operations practical point of view, recurrent models might just not suffice.
5.2.2. Hybrid/enhanced models
Attempts to improve on an already good performing tourism demand forecasting model, takes the shape of mostly hybrid models where different techniques are merged to reap the benefits of each. As found in this review, one popular combination is the CNN-LSTM [ 21 , 26 , 46 ] model. The techniques involve the encoding of the time series dataset into images, utilizing the strength of CNN at image processing to extract more embedded features and then passing those features to the LSTM networks. The LSTM whose key design is the processing of sequential data, has now a larger pool of features to exploit and learn temporal dependences between them. This combination of the two deep learning algorithms brings the best out of both in mining hidden features in tourism demand time series, yielding better forecasts.
Based on same principle of reducing the burden of feature extraction, other enhancement techniques were employed such as empirical mode decomposition [ 53 ], PCA [ 18 ] and Seasonal Autoregressive Integrated Moving Average (SARIMA) [ 3 ] - all of which are purposed to feed in the optimised set of features to the final prediction model (LSTM/BPNN). Most the hybrid models in the review emphasizes primarily features engineering - even Stacked Auto Encoders (SAE) reduces high dimensions dataset to only high-level predictors but one critical barrier which even well sought LSTM is ineffective to tackle is the long-term prediction necessary in tourism industry (Kim et al.,2021).
Out of all the reviewed studies, one particular research paper addressed this long-term tourism demand prediction requirement without compromising on the variable-wise correlation. Instead of using LSTM for time series forecast, which is considered as the usual trend, Kim et al. [ 28 ] developed a hybrid model. The model emulates the processing of sequential data through 1-D temporal CNNs per variable, connected to an attention module. The 1-D CNN is well suited for the extraction of temporal features with irregular intervals or patterns, which is an attempt to solve the issue of long-term forecasting. The attention module linked to the CNNs learns the correlations between the determinants and the prediction of the touristic demands is output by processing the temporal features and attention content.
The performance result of the Multi-head Attention CNN [ 28 ] as compared to the benchmark CNN-LSTM model shows such an improvement – accurate even with COVID period that should considered as a new avenue for forecasting demand in the tourism domain. It is yet to be validated under other context and as suggested by the author, future studies would require the validation of the model in other countries using relative determinants. Developing a tourism demand forecasting tool for a resort island hotel with this model would be at the same time, a purposeful continuation of this novel approach of prediction.
5.3. Accuracy of deep learning models
In an attempt to have a common ground for performance comparision of the different deep learning models identified in this SLR, the MAPE metrics of each study were recorded since it indicates the accuracy in relative terms. The MAPE measure of the identified studies is tabulated in Table 13 (where available) and categorised by the DL algorithms, showing the extent to which the predicted value differs from the context-specific actual value. One very interesting observation is that tourism demand prediction with deep learning has an accuracy percentage above 75%, even outperforming the one study (Wu et al.,2021) which included the conventional technique (SARIMA) in its hybrid model.
MAPE performance result of identified DL algorithms.
Although only the DLs involved in the tourism demand are being compared, it is not very accurate to conclude the performance based on the MAPE results only since the context, complexity and the type of predicted values varies significantly among those studies. For instance, predicting the next-day tourism arrival in a specific city [ 54 ] involves a completely different set of variables, patterns than forecasting the hotel's monthly occupancy rate for the next 6 months. MAPE is a good landing mark for a consolidated performance evaluation but for an accurate conclusion, it is essential that the individual comparison grid of each study against other benchmark algorithms be consulted.
In the DNN DL category, the FF-DNN algorithm has performance range of 1.59%–11.21% demonstrating the complexity of tourism demand, influenced by the various contextual aspects such as geography and definition of demand. This observation is further justified in Ref. [ 25 ] whereby the different location of hotels bears different range of MAPE values, despite using same set of external variables. The best performance is achieved based on back propagation model using accurate influential factors, regularised by a grey model. It is observed that DNN accuracy increases with the addition of determining factors –a univariate model of historical values has an error score of 9.2% whereas using a large set of econometrical yields a score of 1.6%.
Attempts to enhance the FF-DNN models are observed to have little improvement with only Sun et al. [ 54 ] achieving an average score of 0.8% by using adding SII data in an ensemble of models. Decomposition technique of Zhang et al. [ 37 ] or the adoption of Fourier series as proposed by Shabri et al. [ 48 ] could not better the error range of 10% and this can be attributed the nature of DNN prone to overfitting – mainly due limited data volume and explanatory variables in tourism domain [ 37 ]. Moreover, tourism demand is mostly a time-series analysis dilemma and as identified in this SLR, capturing the hidden patterns in sequential data has helped in addressing the barriers in DNN. CNN is one of the best approaches in DNN category to extract features (temporal) from timestamped data. Even with a performance of 25.67% [ 49 ] is one of the very promising recent study, suited for long-term prediction using time-series data.
LSTM, as identified in this SLR, has been very popular lately in the tourism demand prediction owing to its ability to handle sequential data and describe the nonlinear relationship between large set of variables. With the exception of papers SD_09 [ 24 ] and SD_21 [ 35 ] that consider only hotel specific features/attributes as determinants, all the RNN models have a MAPE score within the 10% range with the BiLSTM model in Ref. [ 20 ] having the best overall score of 0.71% error. The performance of the RNN models increases significantly with the addition/tuning of input variables-especially external factors such as SII, with BiLSTM being the standout performer of the RNN variants. The difference in the error score in RNN is also dependant of the type and term (long or short) of the tourism demand being predicted.
The error score of the hybrid models in the SLR demonstrate that separating tasks to best utilise the strength of each algorithm, do bring about improvements to forecasting performance but the underlying barriers remains with the final prediction algorithm. Although the PCA in Ref. [ 27 ] optimises the input variables quality, there is only a slight amelioration of as compared to the BPNN models, with overfitting issues still prevailing. The low performance of 42.6% error rate of the model in paper F1_16 [ 3 ] reveals the outdated use of conventional models (SARIMA) for tourism demand forecast – even when being used with LSTM. On the other hand, the attempt to solve the one inefficient aspect of LSTM, which is the long-term prediction, take the shape of a hybrid CNN-LSTM model (with variants). Also, using convolution can extract not only the relationship between each variable and the observation to be predicted but also the relationship between those variables in the time series can be determined. This allows the capturing of deeper hidden sequential features for a clearer long-term prediction. Depending on the desired forecast term, CNN-LSTM is a key area of further enhancement study, especially in tourism demand prediction.
6. Conclusion
Through the intensive literature-review carried out, it is observed that the hospitality industry in a sector of growing research works for demand forecasting, especially with DL techniques gaining popularity with time-series predictions. With majority of papers (70%) acknowledging better performances with multivariate approach and the suitability of DL to thrive with high dimensions/complexity, has encouraged DL-oriented studies for long term demand forecasting. Several papers utilise different sets of influential variables as input for the DL models, demonstrating the contextual weightage of those external factors on the forecasting models. The DL algorithms reviewed have their own stand out characteristics but in the demand prediction area, recurrent neural networks – LSTM, GRU tops the performance charts with their hybrid models (including CNN), even enhancing the output.
This SRL has provided an overview on the use of deep learning models for forecasting occupation rate. Contributing in this research realm, the next step is to experiment with the best performing DL algorithms using the appropriate demand influencing variables idenfied in this review. From the findings it has been deduced that a multivariate approach for tourism demand forecasting is required. As future work seven demand-affecting external variables will be selected and validated for their suitability in our context by the experts in the field.
Author contribution statement
All authors listed have significantly contributed to the development and the writing of this article.
Declaration of competing interest
The authors declare that they have no known competing financial interests or personal relationships that could have appeared to influence the work reported in this paper.
- 1. Nandkeolyar M. 2016. Manoj Nandkeolyar, 2016 - Key Influencing Factors of ADR, Occupancy Rate and RevPAR. https://www.rosegoldhospitality.com/blog/key-influencing-factors-of-adr-occupancy-rate-and-revpar.html [ Google Scholar ]
- 2. Rosenthal Victoria. Mauritius reports another strong year of hotel performance. 2018. https://www.hotelmanagement.net/record-tourism-growth-mauritius-drives-strong-hotel-performance
- 3. Wu D.C.W., Ji L., He K., Tso K.F.G. Forecasting tourist daily arrivals with A hybrid sarima–lstm approach. J. Hospit. Tourism Res. 2021;45(1):52–67. doi: 10.1177/1096348020934046. [ DOI ] [ Google Scholar ]
- 4. Mahapatra S. Why deep learning over traditional machine learning? Medium. 2019. https://towardsdatascience.com/why-deep-learning-is-needed-over-traditional-machine-learning-1b6a99177063
- 5. Shehhi M.A., Karathanasopoulos A. Forecasting hotel room prices in selected GCC cities using deep learning. J. Hospit. Tourism Manag. 2020;42:40–50. [ Google Scholar ]
- 6. Kitchenham B.A., Dyba T., Jorgensen M. Proceedings of the 26th International Conference on Software Engineering. IEEE Computer Society (ICSE ’04); USA: 2004. Evidence-based software engineering; pp. 273–281. [ Google Scholar ]
- 7. Webster J., Watson R.T. Analyzing the past to prepare for the future: writing a literature review. MIS Q. 2002;26(2) (xiii–xxiii) [ Google Scholar ]
- 8. Wohlin C. Proceedings of the 18th International Conference on Evaluation and Assessment in Software Engineering. Association for Computing Machinery (EASE ’14); New York, NY, USA: 2014. Guidelines for snowballing in systematic literature studies and a replication in software engineering; pp. 1–10. [ DOI ] [ Google Scholar ]
- 9. Vassar M., Atakpo P., Kash M.J. Manual search approaches used by systematic reviewers in dermatology. J. Med. Libr. Assoc.: JMLA. 2016;104(4):302–304. doi: 10.3163/1536-5050.104.4.009. [ DOI ] [ PMC free article ] [ PubMed ] [ Google Scholar ]
- 10. Greenhalgh T., Peacock R. Effectiveness and efficiency of search methods in systematic reviews of complex evidence: audit of primary sources. BMJ (Clinical research ed.) 2005;331(7524):1064–1065. doi: 10.1136/bmj.38636.593461.68. [ DOI ] [ PMC free article ] [ PubMed ] [ Google Scholar ]
- 11. Felizardo K., Mendes E., Kalinowski M., Souza E., Vijaykumar N. Using forward snowballing to update systematic. Reviews in Software Engineering. 2016 doi: 10.1145/2961111.2962630. [ DOI ] [ Google Scholar ]
- 12. Lara-Benítez P., Carranza-García M., Riquelme J. An experimental review on deep learning architectures for time series forecasting. Int. J. Neural Syst. 2020;31 doi: 10.1142/S0129065721300011. [ DOI ] [ PubMed ] [ Google Scholar ]
- 13. Chang Y.W., Tsai C.Y. Proceedings - 31st IEEE International Conference on Advanced Information Networking and Applications Workshops. WAINA; 2017. Apply deep learning neural network to forecast number of tourists; pp. 259–264. [ DOI ] [ Google Scholar ]
- 14. Kitchenham B., Charters S. 2007. Guidelines for Performing Systematic Literature Reviews in Software Engineering. [ Google Scholar ]
- 15. Dean J. 2020 IEEE International Solid- State Circuits Conference - (ISSCC). 2020 IEEE International Solid- State Circuits Conference - (ISSCC) IEEE; San Francisco, CA, USA: 2020. 1.1 the deep learning revolution and its implications for computer architecture and chip design; pp. 8–14. [ DOI ] [ Google Scholar ]
- 16. Bi J.W., Li H., Fan Z.P. Tourism demand forecasting with time series imaging: a deep learning model. Ann. Tourism Res. 2021;90 [ Google Scholar ]
- 17. Law R., Au N. A neural network model to forecast Japanese demand for travel to Hong Kong. Tourism Manag. 1999;20(1):89–97. [ Google Scholar ]
- 18. Li C., Zheng W., Ge P. Tourism demand forecasting with spatiotemporal features. Ann. Tourism Res. 2022 doi: 10.1016/j.annals.2022.103384. [ DOI ] [ Google Scholar ]
- 19. Zhang Y., Li G., Muskat B., Law R., Yang Y. Group pooling for deep tourism demand forecasting. Ann. Tourism Res. 2020;82 doi: 10.1016/j.annals.2020.102899. [ DOI ] [ Google Scholar ]
- 20. Kulshrestha A., Krishnaswamy V., Sharma M. Bayesian BILSTM approach for tourism demand forecasting. Ann. Tourism Res. 2020;83 doi: 10.1016/j.annals.2020.102925. [ DOI ] [ Google Scholar ]
- 21. He K., Ji L., Wu C., Tso K. Using SARIMA–CNN–LSTM approach to forecast daily tourism demand. J. Hospit. Tourism Manag. 2021 doi: 10.1016/j.jhtm.2021.08.022. [ DOI ] [ Google Scholar ]
- 22. Lv S.X., Peng L., Wang L. Stacked autoencoder with echo-state regression for tourism demand forecasting using search query data. Appl. Soft Comput. 2018;73:119–133. [ Google Scholar ]
- 23. Huang L., Zheng W. Novel deep learning approach for forecasting daily hotel demand with agglomeration effect. International Journal of Hospitality. 2021 doi: 10.1016/j.ijhm.2021.103038. [ DOI ] [ Google Scholar ]
- 24. Kaya K., Yılmaz Y., Yaslan Y., Öğüdücü Ş.G., Çıngı F. Demand forecasting model using hotel clustering findings for hospitality industry. Inf. Process. Manag. 2022;59(1) doi: 10.1016/j.ipm.2021.102816. [ DOI ] [ Google Scholar ]
- 25. Assaf A.G., Tsionas M.G. Forecasting occupancy rate with Bayesian compression methods. Ann. Tourism Res. 2019;75:439–449. [ Google Scholar ]
- 26. Bi J.W., Liu Y., Li H. Daily tourism volume forecasting for tourist attractions. Ann. Tourism Res. 2020;83 doi: 10.1016/j.annals.2020.102923. [ DOI ] [ Google Scholar ]
- 27. Li Y., Cao H. Prediction for tourism flow based on LSTM neural network. Procedia Comput. Sci. 2018;129:277–283. [ Google Scholar ]
- 28. Kim D.K., Shyn S., Kim D., Jang S.… . SSCI); Orlando, FL, USA: 2021. ‘A Daily Tourism Demand Prediction Framework Based on Multi-Head Attention CNN: the Case of the Foreign Entrant in South Korea’, 2021 IEEE Symposium Series on Computational Intelligence; pp. 1–10. [ DOI ] [ Google Scholar ]
- 29. Iha T., Kawamitsu I., Ohshiro A., Nakamura M. ‘An LSTM-based multivariate time series predicting method for number of restaurant customers in tourism resorts’, in 2021 36th international technical Conference on circuits/systems, Computers and Communications (ITC- CSCC). 2021 36th international technical Conference on circuits/systems. Comput. Commun. 2021:1–4. doi: 10.1109/ITC-CSCC52171.2021.9501432. [ DOI ] [ Google Scholar ]
- 30. Alamsyah A., Ayastri Friscintia P.B. 2019. ‘Artificial Neural Network for Indonesian Tourism Demand Forecasting’, in 2019 7th International Conference on Information and Communication Technology (ICoICT). 2019 7th International Conference on Information and Communication Technology (ICoICT) pp. 1–7. [ DOI ] [ Google Scholar ]
- 31. Ramos-Carrasco R., Galvez-Diaz S., Nuñez-Ponce V., Alvarez-Merino J. 2019 IEEE Sciences and Humanities International Research Conference (SHIRCON). 2019. IEEE Sciences and Humanities International Research Conference (SHIRCON); 2019. Artificial neural networks to estimate the forecast of tourism demand in Peru; pp. 1–4. [ DOI ] [ Google Scholar ]
- 32. Laaroussi H., Guerouate F. 2020. ‘Deep Learning Framework for Forecasting Tourism Demand’, 020 IEEE International Conference on Technology Management, Operations and Decisions (ICTMOD), Marrakech, Morocco; pp. 1–4. [ DOI ] [ Google Scholar ]
- 33. Huang Y., Huang W., Yan S., Wang H., Yan J. 2021 13th International Conference on Intelligent Human-Machine Systems and Cybernetics (IHMSC). 2021 13th International Conference on Intelligent Human-Machine Systems and Cybernetics. IHMSC); 2021. The Research on the forecast of tourism demand based on Baidu search index–Taking Beijing as an example; pp. 91–94. [ DOI ] [ Google Scholar ]
- 34. Nguyen L.Q., Fernandes P.O., Teixeira J.P. Analyzing and forecasting tourism demand in Vietnam with artificial neural networks. Forecasting. 2022;4(1):36–50. doi: 10.3390/forecast4010003. [ DOI ] [ Google Scholar ]
- 35. Chang Y.-M., Chen C.-H., Lai J.-P., Lin Y.-L., Pai P.-F. Forecasting hotel room occupancy using long short-term memory networks with sentiment analysis and scores of customer online reviews. Appl. Sci. 2021;11(21) doi: 10.3390/app112110291. [ DOI ] [ Google Scholar ]
- 36. Dong Y., Xiao L. 2022 3rd Asia Service Sciences and Software. 2022. A combination model based deep long term model for tourism demand forecasting. [ DOI ] [ Google Scholar ]
- 37. Zhang C., Jiang F., Wang S., Sun S. A new decomposition ensemble approach for tourism demand forecasting: evidence from major source countries in Asia-Pacific region. Int. J. Tourism Res. 2021;23(5):832–845. doi: 10.1002/jtr.2445. [ DOI ] [ Google Scholar ]
- 38. Wang T. An intelligent passenger flow prediction method for pricing strategy and hotel operations. Complexity. 2021;2021 doi: 10.1155/2021/5520223. [ DOI ] [ Google Scholar ]
- 39. Hu M., Song H. Data source combination for tourism demand forecasting. Tourism Econ. 2020;26(7):1248–1265. doi: 10.1177/1354816619872592. [ DOI ] [ Google Scholar ]
- 40. Ouassou E. houssin, Taya H. Forecasting regional tourism demand in Morocco from traditional and AI-based methods to ensemble modeling. Forecasting. 2022;4(2):420–437. doi: 10.3390/forecast4020024. [ DOI ] [ Google Scholar ]
- 41. Peng L., Wang L., Ai X., Zeng Y. Cognitive Computation [Preprint]; 2021. Forecasting Tourist Arrivals via Random Forest and Long Short-Term Memory. Query date: 2022-06-18 00:07:21) [ DOI ] [ Google Scholar ]
- 42. Kaewmanee P., Muangprathub J., Sae-Jie W. 2021. ‘Forecasting Tourist Arrivals with Keyword Search Using Time Series’, in 2021 18th International Conference on Electrical Engineering/Electronics, Computer, Telecommunications and Information Technology (ECTI-CON). 2021 18th International Conference on Electrical Engineering/Electronics, Computer, Telecommunications and Information Technology (ECTI-CON) pp. 171–174. [ DOI ] [ Google Scholar ]
- 43. Gricar S., Baldigara T., Šugar V. Sustainable determinants that affect tourist arrival forecasting. Sustainability. 2021;13(17):9659. doi: 10.3390/su13179659. [ DOI ] [ Google Scholar ]
- 44. Yang Y., Sun S. Tourism demand forecasting and tourists' search behavior: evidence from segmented Baidu search volume. Data Science and Management. 2021 doi: 10.1016/j.dsm.2021.10.002. [ DOI ] [ Google Scholar ]
- 45. Zhang Y., Li G., Muskat B., Law R., Yang Y. Group pooling for deep tourism demand forecasting. Ann. Tourism Res. 2020;82 doi: 10.1016/j.annals.2020.102899. [ DOI ] [ Google Scholar ]
- 46. Lu W., et al. A method based on ga-cnn-lstm for daily tourist flow prediction at scenic spots. Entropy. 2020;22(3):261. doi: 10.3390/e22030261. [ DOI ] [ PMC free article ] [ PubMed ] [ Google Scholar ]
- 47. Adil M., et al. Attention-based STL-BiLSTM network to forecast tourist arrival. Processes. 2021 doi: 10.3390/pr9101759. [ DOI ] [ Google Scholar ]
- 48. Shabri A., Samsudin R., Yusoff Y. Combining deep neural network and fourier series for tourist arrivals forecasting. OP Conf. Ser.: Mater. Sci. Eng. 2020;864 doi: 10.1088/1757-899X/864/1/012094. [ DOI ] [ Google Scholar ]
- 49. Anisa M.P., Irawan H., Widiyanesti S. Forecasting demand factors of tourist arrivals in Indonesia's tourism industry using recurrent neural network. IOP Conf. Ser. Mater. Sci. Eng. 2021;1077(1) doi: 10.1088/1757-899X/1077/1/012035. [ DOI ] [ Google Scholar ]
- 50. Wang L., Wu B., Zhu Q., Zeng Y. Forecasting monthly tourism demand using enhanced backpropagation neural network. Neural Process. Lett. 2020 doi: 10.1007/s11063-020-10363-z. [ DOI ] [ Google Scholar ]
- 51. Zhang B., Pu Y., Wang Y., Li J. Forecasting hotel accommodation demand based on lstm model incorporating internet search index. Sustainability. 2019;11(17):4708. [ Google Scholar ]
- 52. Hsieh S. Tourism demand forecasting based on an LSTM network and its variants. Algorithms. 2021;14(8):243. doi: 10.3390/a14080243. [ DOI ] [ Google Scholar ]
- 53. Zhang Y., Li G., Muskat B., Law R. Tourism demand forecasting: a decomposed deep learning approach. J. Trav. Res. 2020;60(5):981–997. [ Google Scholar ]
- 54. Sun S., Li Y., Guo J., Wang S. Tourism demand forecasting: an ensemble deep learning approach. Tourism Econ. 2021 doi: 10.1177/13548166211025160. [ DOI ] [ Google Scholar ]
- 55. Wang J., Ge P., Liu Z. 2021 IEEE 2nd International Conference on Pattern Recognition and Machine Learning (PRML). 2021 IEEE 2nd International Conference on Pattern Recognition and Machine Learning. PRML); 2021. Using denoised LSTM network for tourist arrivals prediction; pp. 176–182. [ DOI ] [ Google Scholar ]
- 56. Li S., Chen T., Wang L., Ming C. Effective tourist volume forecasting supported by PCA and improved BPNN using Baidu index. Tourism Manag. 2018;68:116–126. [ Google Scholar ]
- 57. Ma X. ‘Tourism demand forecasting based on grey model and BP neural network’. Complexity. 2021;2021 doi: 10.1155/2021/5528383. [ DOI ] [ Google Scholar ]
- 58. Tianyang W. A K-means group division and LSTM based method for hotel demand forecasting. Tech. Gaz. 2021 https://hrcak.srce.hr/260859 [ Google Scholar ]
- 59. Chen Y., Hu T. 2020. Exploring Dual-Element Sustainable Tourism on Hainan Island Based on Deep Learning: Early Warning and Forecasting. https://papers.ssrn.com/sol3/papers.cfm?abstract_id=4091314 SSRN 4091314. [ Google Scholar ]
- 60. Zhang C., Tian Y.-X. Social Science Research Network; Rochester, NY: 2022. Forecast Daily Tourist Volumes during the Epidemic Period Using Covid-19 Data, Search Engine Data and Weather Data. SSRN Scholarly Paper 4102837. [ DOI ] [ PMC free article ] [ PubMed ] [ Google Scholar ]
- 61. Zheng S. 2020. Research on GM-LSTM Hybrid Model for Tourism Prediction Based on One Belt and One Road. https://core.ac.uk/download/pdf/344921448.pdf [ Google Scholar ]
- 62. Saivijayalakshmi J., Ayyanathan N. ICCICA); 2021. ‘Comparative Performance Analysis of Deep Learning Technique with Statistical Models on Forecasting the Foreign Tourists Arrival Pattern to India’, in 2021 International Conference on Computational Intelligence and Computing Applications (ICCICA). 2021 International Conference on Computational Intelligence and Computing Applications; pp. 1–3. [ DOI ] [ Google Scholar ]
- 63. Guizzardi A., Pons F.M.E., Angelini G., Ranieri E. Big data from dynamic pricing: a smart approach to tourism demand forecasting. Int. J. Forecast. 2021;37(3):1049–1060. doi: 10.1016/j.ijforecast.2020.11.006. [ DOI ] [ Google Scholar ]
- 64. Glaser B., Strauss A. 1967. The discovery of grounded theory: strategies for qualitative research aldine de gruyter. [ DOI ] [ Google Scholar ]
- 65. Harding J. SAGE; 2018. Qualitative Data Analysis: from Start to Finish. [ Google Scholar ]
- 66. Gupta V. 2017. ‘Understanding Feedforward Neural Networks | LearnOpenCV’, 9 October. https://learnopencv.com/understanding-feedforward-neural-networks [ Google Scholar ]
- 67. LeCun Y., Bottou L., Bengio Y., Haffner P. Gradient-based learning applied to document recognition. Proc. IEEE. 1998;86(11):2278–2324. [ Google Scholar ]
- 68. Hochreiter S., Younger A., Conwell P. 2001. Learning to Learn Using Gradient Descent; pp. 87–94. [ DOI ] [ Google Scholar ]
- 69. Hochreiter S., Schmidhuber J. Long short-term memory. Neural Comput. 1997;9(8):1735–1780. doi: 10.1162/neco.1997.9.8.1735. [ DOI ] [ PubMed ] [ Google Scholar ]
- 70. Yildirim O. A novel wavelet sequence based on deep bidirectional LSTM network model for ECG signal classification. Comput. Biol. Med. 2018;96:189–202. doi: 10.1016/j.compbiomed.2018.03.016. [ DOI ] [ PubMed ] [ Google Scholar ]
- 71. Bengio Y., Lamblin P., Popovici D., Larochelle H. Proceedings of the 19th International Conference on Neural Information Processing Systems. MIT Press (NIPS’06); Cambridge, MA, USA: 2006. Greedy layer-wise training of deep networks; pp. 153–160. [ Google Scholar ]
- 72. Mishra A. 2020. Metrics to Evaluate Your Machine Learning Algorithm, Medium. https://towardsdatascience.com/metrics-to-evaluate-your-machine-learning-algorithm- f10ba6e38234 [ Google Scholar ]
- 73. Goyal S. 2021. ‘Evaluation Metrics for Regression Models’, Analytics Vidhya, 16 August. https://medium.com/analytics-vidhya/evaluation-metrics-for-regression-models-c91c65d73af [ Google Scholar ]
- 74. Stephanie Mean Absolute percentage error (MAPE), statistics how to. 2022. https://www.statisticshowto.com/mean-absolute-percentage-error-mape
- 75. Kumar A. Mean squared error or R-squared - which one to use? Data Analytics. 2022 https://vitalflux.com/mean-square-error-r-squared-which-one-to-use/ [ Google Scholar ]
- View on publisher site
- PDF (1.4 MB)
- Collections
Similar articles
Cited by other articles, links to ncbi databases.
- Download .nbib .nbib
- Format: AMA APA MLA NLM
Add to Collections

IMAGES
VIDEO
COMMENTS
Chapter 2 REVIEW OF RELATED LITERATURE AND STUDIES This chapter presents the review of related literature and studies particularly on the Proposed Computerized Hotel Management System of ... update the list of the facilities available in the resort. The proposed system will also enable the administrator to generate reports about the information ...
This paper reviews a content analysis of integrated resort papers published in the hospitality and tourism literature over the past 26 years (1991-2017). Based on a detailed examination of the contents, a database consisting of information on (1) study expansion, (2) study locations, (3) major subject domains, and (4) research methodology was ...
Online Reservation with Billing System for Villa Silvina Resort (Zapanta, B., Belardo, R., Flores, F., Sobrevilla, G., 2009) The "Online Reservation with Billing System for Villa Silvina Resort" done by B. Zapanta, Belardo, Flores and Sobrevilla is a system that is mostly focused on making reservations for the resort and the rooms in their ...
This study addresses the situation by cataloguing and analysing existing literature in the destination resort field. Literature is examined from three perspectives: functional classification ...
A literature review is considered the most appropriate and valid approach as it paves the way for researchers to structure a research field while forming the backbone of any piece of research ...
The resort industry is a distinctive and dynamic segment of the hospitality industry, producing a unique environment where managers must deal with a wide range of unique issues. Prior research into the area of resort management has been limited in scope with limited investigation into the underlying reasoning behind management practices.
This paper reviews a content analysis of integrated resort papers published in the hospitality and tourism literature over the past 26 years (1991-2017). Based on a detailed examination of the contents, a database consisting of information on (1) study expansion, (2) study locations, (3) major subject domains, and (4) research methodology was ...
Literature review A tourism destination, resort in this instance, is a defined geographical area in which visitors spend at least one night, and comprises tourism products such as accommodation, transportation, infrastructure, facilities and services (Keyser, 2009). Resorts located at the
The above-mentioned journals have routinely been used for systematic literature reviews (e.g., Guzzo et al., 2019; Honag et al., 2023; Kumar et al., 2023). Based on the systematic literature review process guidelines provided by Peričić and Tanveer (2019), the following set of inclusion/exclusion criteria were chosen: •
The systematic literature review (SLR) provides the answer to a number of research questions through the thorough review of current literature using rigourous protocols for selection and assessment while minimizing bias. ... data set for the actual hotel occupancy prediction is mostly obtained from the hotel's property management system. As ...