Epekto Ng Paggamit Ng Online Class Sa Pag- Aaral Ng Piling Mag-Aaral Sa Unang Taon Sa Medyor Sa Filipino
- Stephanie S. Alba Bestlink College of the Philippines
- Jenalyn C. Arsaga Bestlink College of the Philippines
- Mark Angelo V. Bacatio Bestlink College of the Philippines
- Janeth Ross L. De Vera Bestlink College of the Philippines
- Mhica Shaine O. Mogarte Bestlink College of the Philippines
Ang online class ay umusbong nang magsimulang magkaroon ng pandemya sa ating bansa nga COVID-19 noong taong 2020. Naging daan ang online class upang maipagpatuloy ang pag aaral ng mga mag aaral kahit hindi pumupunta sa paaralan partikular na sa Bestlink College of the Philippines. Ito'y nangangailangan lamang ng mga kagamitan tulad ng kompyuter, o kahit anong gadyet at internet.
Naging malaking hamon ang panibagong paraan ng pag-aaral na ito sa ibat ibang larangan. Anuman ang husay ng mga guro sa kanilang pamamaraan at kagamitang panturo ay hindi pa rin magiging epektibo ang pagkatuto ng mga bata kung wala ang mga magulang na nagmomonitor sa kanilang mga anak sa bahay habang nasa online class ang bata. Mahalagang makipagugnayan ang mga guro sa mga magulang dahil ito ay isa sa mga estratehiya upang maging aktibo ang mga bata sa kanilang pagaaral. Sa pag-aaral na ito'y nalaman ng mga mananaliksik ang epekto ng paggamit ng online class sa mga piling mag-aaral mula sa unang taon sa kolehiyo sa BSED medyor sa Filipino, natukoy at nailahad sa pag-aral na ito kung angkop bang gamitin ang online class sa makabagong panahon bilang ganap na pantulong sa pagtuturo.
Ang isinagawang pananaliksik na ito ay patungkol sa epekto ng paggamit ng Online class sa piling mag-aaral mula sa unang taon sa kolehiyo sa Medyor sa Filipino. Ito ay ginamitan ng deskriptong metodolohiya, pinili ng mga mananaliksik. Ang descriptive survey research design na gumagamit ng talatanungan upang malikom ng mga datos. Ang mga mananaliksik ay naniniwala na ang disenyong ginamit ay ang angkop para sa paksa dahil mas mapapadali ang pangangalap ng mga datos na isinasagawa at naiintindihan ng mga mananaliksik sa pag-aaral na ito ang descriptive survey research design ay nababagay sa pagaaral na isinasagawa kahit limitado lamang ang kanilang respondente. Ito ay dahil hindi lamang sila nakadenpende sa mga sagot sa kanilang talatanungan kundi maaari rin silang magsagawa ng panayam at obserbasyon upang idagdag sa mga nakalap nilang datos at impormasyon. Ang mga mananaliksik ay nangalap ng datos sa paaaralan ng Bestlink College of the Philippines. Ang mga ito ay nagpasagot sa apatnapu (40) na mga mag-aaral sa unang taon ng BSED medyor sa Filipino Panuruang taon 2022-2023. Sila ang aming napiling respondente dahil nais naming malaman kung paano nakaka-apekto sa kanila, Bilang isang mag aaral ang pag gamit ng Online class Mula sa unang taon sa kolehiyo sa Medyor sa Filipino. Makikita sa bahaging ito ang frequency at bahagdan ng bawat respondente ayon sa kanilang edad na 35 o 87.5% ng mga estudayante na kabilang sa edad na 20 na taong gulang, kung saan ito ay mayroong pinakamataas na bahagdan. At mula sa ikalawa pinapakita na ang frequency at bahagdan ng respondente ayon sa kanilang mga kasarian 34 o 85% na babae ang pinakamataas na sumagot sa talatanungan at may pinaka mataas na ranggo. At mula sa ikatlo, Matatagpuan ang frequency at bahagdan ng mga respondente ayon sa kanilang mga 17 o 42.5% ang pinakamataas na nakuha sa paggugol ng oras sa Online class ng mga estudyante sa paggamit ng online learning mode. Dulot ng makabagong paraan ng pag-aaral ito ay nagpapakita sa bawat talahanayan ng weighted mean at bahagdan ng mga respondente ayon sa epektong dulot ng makabagong paraan ng pag-aaral 3.35 ang grand mean nito. At 3.625 ang mean at nakakuha ng pinaka mataas na ranggo. Sa pananaw sa Online class ito ay nagpapakita ng weighted mean ay nakakuha ng mataas na Mean na may 4.45 na lubos na nakaaapekto sa paggamit ng online learning mode, weighted mean at bahagdan ng mga respondente ayon sa ayon sa pananaw ng online class at sa bawat na nararamdaman ng mga estudyante sa iba't-ibang platform extensions for Google Classroom, Google Meet, and Zoom. Ipinapakita sa talahanayan ng weighted mean at bawat bahagdan ng bawat respondente na nakakuha na Mean 3.55 na kailangan pang linangin ang kanilang kaalaman sa paggamit ng online learning mode sa online class. Sa pagpapaunlad ng kaalaman at kahusayan sa pag-aaral ng asignaturang Filipino hinggil sa epekto nito. Ang talahanayan ay nagpapakita ng weighted mean at bahagdan ng mga respondente ayon sa mula sa unang aytem nito ay nakakuha ng may Mean na 4 ito ay madalas na nakaaapekto ayon sa pagpapaunlad ng kaalaman. Panghuli na posibleng rekomendasyon ang bawat talahanayan ay nagpapakita ng weighted mean at bawat bahagdan ng bawat respondente ayon sa mula sa unang aytem ito ay nakakuha ng mayroong mean na 3.475 na naaayon sa kanilang isinagot sa talatanungan.

How to Cite
- Endnote/Zotero/Mendeley (RIS)
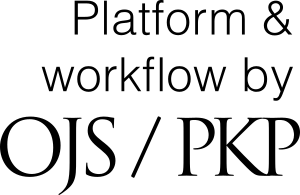
Information
- Author Services
Initiatives
You are accessing a machine-readable page. In order to be human-readable, please install an RSS reader.
All articles published by MDPI are made immediately available worldwide under an open access license. No special permission is required to reuse all or part of the article published by MDPI, including figures and tables. For articles published under an open access Creative Common CC BY license, any part of the article may be reused without permission provided that the original article is clearly cited. For more information, please refer to https://www.mdpi.com/openaccess .
Feature papers represent the most advanced research with significant potential for high impact in the field. A Feature Paper should be a substantial original Article that involves several techniques or approaches, provides an outlook for future research directions and describes possible research applications.
Feature papers are submitted upon individual invitation or recommendation by the scientific editors and must receive positive feedback from the reviewers.
Editor’s Choice articles are based on recommendations by the scientific editors of MDPI journals from around the world. Editors select a small number of articles recently published in the journal that they believe will be particularly interesting to readers, or important in the respective research area. The aim is to provide a snapshot of some of the most exciting work published in the various research areas of the journal.
Original Submission Date Received: .
- Active Journals
- Find a Journal
- Journal Proposal
- Proceedings Series
- For Authors
- For Reviewers
- For Editors
- For Librarians
- For Publishers
- For Societies
- For Conference Organizers
- Open Access Policy
- Institutional Open Access Program
- Special Issues Guidelines
- Editorial Process
- Research and Publication Ethics
- Article Processing Charges
- Testimonials
- Preprints.org
- SciProfiles
- Encyclopedia
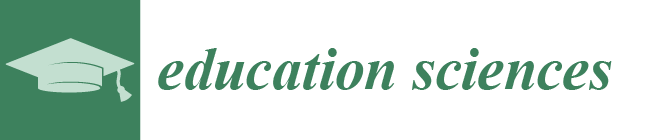
Article Menu

- Subscribe SciFeed
- Recommended Articles
- Google Scholar
- on Google Scholar
- Table of Contents
Find support for a specific problem in the support section of our website.
Please let us know what you think of our products and services.
Visit our dedicated information section to learn more about MDPI.
JSmol Viewer
Characteristics of filipino online learners: a survey of science education students’ engagement, self-regulation, and self-efficacy.
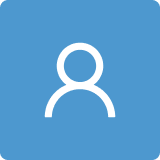
1. Introduction
1.1. frameworks used in the study, 1.2. student engagement, 1.3. self-regulated learning, 1.4. self-efficacy, 1.5. research gap.
- Gender preference;
- Internet access;
- Internet signal;
- Region of residence;
- Gadgets used in online learning;
- Most preferred or best time to study online;
- Online learning tools?
- Student engagement (OSE);
- Self-regulated online learning (SROL);
- Online learning self-efficacy (OLSE)?
2. Materials and Methods
2.1. research design, 2.2. research sampling and participants, 2.3. research instruments, 2.4. data gathering and ethics, 2.5. data analysis, 2.6. assumptions for manova, 2.7. process flow of this survey research, 3.1. demographic profile, 3.2. gadgets for online learning, 3.3. online learning tools, 3.4. online learning characteristics, 3.4.1. online student engagement, 3.4.2. self-regulated learning, 3.4.3. online learning self-efficacy, 3.4.4. effects of the demographic profile on ose, srol, and olse, 3.4.5. olse characteristics according to gender preference, 4. discussion, 5. conclusions, implications, and recommendations, author contributions, institutional review board statement, informed consent statement, data availability statement, acknowledgments, conflicts of interest.
- Pangeni, S.K. Open and distance learning: Cultural practices in Nepal. Eur. J. Open Distance E-Learn. 2016 , 19 , 32–45. [ Google Scholar ] [ CrossRef ]
- Hasan, N.; Khan, N.H. Online teaching-learning during Covid-19 pandemic: Students’ perspective. Online J. Distance Educ. E-Learn. 2020 , 8 , 202–213. Available online: http://tojdel.net/journals/tojdel/articles/v08i04/v08i04-03.pdf (accessed on 15 December 2020).
- Susila, H.R.; Qosim, A.; Rositasari, T. Students’ perception of online learning in COVID-19 pandemic: A preparation for developing a strategy for learning from home. Univers. J. Educ. Res. 2020 , 8 , 6042–6047. [ Google Scholar ] [ CrossRef ]
- Sutarto, S.; Sari, D.P.; Fathurrochman, I. Teacher strategies in online learning to increase students’ interest in learning during COVID-19 pandemic. J. Konseling Pendidik. 2020 , 8 , 129–137. [ Google Scholar ] [ CrossRef ] [ PubMed ]
- Rizun, M.; Strzelecki, A. Students’ acceptance of the COVID-19 impact on shifting higher education to distance learning in Poland. Int. J. Environ. Res. Public Health 2020 , 17 , 6468. [ Google Scholar ] [ CrossRef ]
- Rapanta, C.; Botturi, L.; Goodyear, P.; Guàrdia, L.; Koole, M. Online university teaching during and after the Covid-19 crisis: Refocusing teacher presence and learning activity. Postdigit. Sci. Educ. 2020 , 2 , 923–945. [ Google Scholar ] [ CrossRef ]
- Cabaleiro-Cerviño, G.; Vera, C. The Impact of Educational Technologies in Higher Education. GIST Educ. Learn. Res. J. 2020 , 20 , 155–169. Available online: https://files.eric.ed.gov/fulltext/EJ1262695.pdf (accessed on 21 September 2023). [ CrossRef ]
- Shahabadi, M.M.; Uplane, M. Synchronous and Asynchronous E-Learning Styles and Academic Performance of e-Learners. Procedia—Soc. Behav. Sci. 2015 , 176 , 129–138. [ Google Scholar ] [ CrossRef ]
- Ghavifekr, S.; Rosdy, W.A.W. Teaching and learning with technology: Effectiveness of ICT integration in schools. Int. J. Res. Educ. Sci. 2016 , 1 , 175–191. Available online: https://files.eric.ed.gov/fulltext/EJ1105224.pdf (accessed on 15 January 2021). [ CrossRef ]
- Ukata, P.F.; Onuekwa, F.A. Application of ICT towards minimizing traditional classroom challenges of teaching and learning during COVID-19 pandemic in Rivers State Tertiary Institutions. Int. J. Educ. Eval. 2020 , 6 , 24–39. Available online: https://www.iiardjournals.org/get/IJEE/VOL.%206%20NO.%205%202020/APPLICATION%20OF%20ICT%20TOWARDS.pdf (accessed on 20 September 2023).
- Sun, A.; Chen, X. Online education and its effective practice: A research review. J. Inf. Technol. Educ. Res. 2016 , 15 , 157–190. [ Google Scholar ] [ CrossRef ]
- Rodrigues, H.; Almeida, F.; Figueiredo, V.; Lopes, S.L. Tracking e-learning through published papers: A systematic review. Comput. Educ. 2019 , 136 , 87–98. [ Google Scholar ] [ CrossRef ]
- Stephen, J.S.; Rockinson-Szapkiw, A.J.; Dubay, C. Persistence model of non-traditional online learners: Self-efficacy, self-regulation, and self-direction. Am. J. Distance Educ. 2020 , 34 , 306–321. [ Google Scholar ] [ CrossRef ]
- Manco-Chavez, J.A.; Uribe-Hernandez, Y.C.; Buendia-Aparcana, R.; Vertiz-Osores, J.J.; Alcoser, S.D.I.; Rengifo-Lozano, R.A. Integration of ICTS and digital skills in times of the pandemic COVID-19. Int. J. High. Educ. 2020 , 9 , 11. [ Google Scholar ] [ CrossRef ]
- Rahiem, M.D.H. The emergency remote learning experience of university students in Indonesia amidst COVID-19 crisis. Int. J. Learn. Teach. Educ. Res. 2020 , 16 , 1–26. [ Google Scholar ] [ CrossRef ]
- Biwer, F.; Wiradhany, W.; Oude Egbrink, M.; Hospers, H.; Wasenitz, S.; Jansen, W.; De Bruin, A. Changes and adaptations: How university students self-regulate their online learning during the COVID-19 pandemic. Front. Psychol. 2021 , 12 , 642593. [ Google Scholar ] [ CrossRef ]
- Baticulon, R.E.; Alberto, N.R.I.; Baron, M.B.C.; Mabulay, R.E.C.; Rizada, L.G.T.; Sy, J.J.; Tiu, C.J.S.; Clarion, C.A. Barriers to online learning in the time of COVID-19: A national survey of medical students in the Philippines. medRxiv 2020 . [ Google Scholar ] [ CrossRef ] [ PubMed ]
- Juhary, J. Perceived usefulness and ease of use of the learning management system as a learning tool. Int. Educ. Stud. 2014 , 7 , 23. [ Google Scholar ] [ CrossRef ]
- Hensley, L.C.; Iaconelli, R.; Wolters, C.A. “This weird time we’re in”: How a sudden change to remote education impacted college students’ self-regulated learning. J. Res. Technol. Educ. 2022 , 54 (Suppl. S1), S203–S218. [ Google Scholar ] [ CrossRef ]
- Gambo, Y.; Shakir, M. Evaluating students’ experiences in self-regulated smart learning environment. Educ. Inf. Technol. 2023 , 28 , 547–580. [ Google Scholar ] [ CrossRef ]
- Gonzales, K.P. Rising from Covid-19: Private schools’ readiness and response amidst a global pandemic. Int. Multidiscip. Res. J. 2020 , 2 , 81–90. [ Google Scholar ] [ CrossRef ]
- Bruso, J.; Stefaniak, J.; Bol, L. An examination of personality traits as a predictor of the use of self-regulated learning strategies and considerations for online instruction. Educ. Technol. Res. Dev. 2020 , 68 , 2659–2683. [ Google Scholar ] [ CrossRef ]
- Abou-Khalil, V.; Helou, S.; Khalifé, E.; Chen, M.A.; Majumdar, R.; Ogata, H. Emergency online learning in low-resource settings: Effective student engagement strategies. Educ. Sci. 2021 , 11 , 24. [ Google Scholar ] [ CrossRef ]
- Churiyah, M.; Sholikhan, S.; Filianti, F.; Sakdiyyah, D.A. Indonesia education readiness conducting distance learning in Covid-19 pandemic situation. Int. J. Multicult. Multireligious Underst. 2020 , 7 , 491–507. [ Google Scholar ] [ CrossRef ]
- Kerr, M.S.; Rynearson, K.; Kerr, M.C. Student characteristics for online learning success. Internet High. Educ. 2006 , 9 , 91–105. [ Google Scholar ] [ CrossRef ]
- Soffer, T.; Cohen, A. Students’ engagement characteristics predict success and completion of online courses. J. Comp. Asstd. Learn. 2019 , 35 , 378–389. [ Google Scholar ] [ CrossRef ]
- Broadbent, J.; Poon, W.L. Self-regulated learning strategies and academic achievement in online higher education learning environments: A systematic review. Internet High. Educ. 2015 , 27 , 1–13. [ Google Scholar ] [ CrossRef ]
- Alqurashi, E. Self-Efficacy in online learning environments: A literature review. Contemp. Issues Educ. Res. 2016 , 9 , 45–52. [ Google Scholar ] [ CrossRef ]
- Czerkawski, B.C.; Lyman, E.W. An instructional design framework for fostering student engagement in online learning environments. TechTrends 2016 , 60 , 532–539. [ Google Scholar ] [ CrossRef ]
- Panadero, E.; Alonso-Tapia, J. How Do Students Self-Regulate? Review of Zimmerman’s Cyclical Model of Self-Regulated Learning. Ann. Psicol. 2014 , 30 , 450–462. [ Google Scholar ]
- Redmond, P.; Abawi, L.; Brown, A.; Henderson, R.; Heffernan, A. An online engagement framework for higher education. Online Learn. J. 2018 , 22 , 183–204. [ Google Scholar ] [ CrossRef ]
- Ryan, R.M.; Deci, E.L. Self-determination theory and the facilitation of intrinsic motivation, social development, and well-being. Am. Psych. 2000 , 55 , 68–78. [ Google Scholar ] [ CrossRef ] [ PubMed ]
- Ryan, R.M.; Deci, E.L. Intrinsic and extrinsic motivation from a self-determination theory perspective: Defnitions, theory, practices, and future directions. Cont. Educ. Psych. 2020 , 61 , 101860. [ Google Scholar ] [ CrossRef ]
- Reeve, J. A self-determination theory perspective on student engagement. In Handbook of Research on Student Engagement ; Springer: Boston, MA, USA, 2012; pp. 149–172. ISBN 978-1-4614-2017-0. [ Google Scholar ]
- Chiu, T.K.F. Applying the self-determination theory (SDT) to explain student engagement in online learning during the COVID-19 pandemic. J. Res. Technol. Educ. 2022 , 54 (Suppl. S1), S14–S30. [ Google Scholar ] [ CrossRef ]
- Gronchi, M. Self-determination, self-efficacy, and attribution in FL online learning: An exploratory survey with university students during the pandemic emergency. Studi Glottodidattica 2021 , 6 , 118–134. [ Google Scholar ] [ CrossRef ]
- Niemiec, C.P.; Lynch, M.F.; Vansteenkiste, M.; Bernstein, J.; Deci, E.L.; Ryan, R.M. The antecedents and consequences of autonomous self-regulation for college: A self-determination theory perspective on socialization. J. Adolesc. 2006 , 29 , 761–775. [ Google Scholar ] [ CrossRef ]
- Trowler, V. Student engagement literature review. High. Educ. Acad. 2010 , 11 , 1–15. Available online: https://pure.hud.ac.uk/en/publications/student-engagement-literature-review (accessed on 6 March 2021).
- Pittaway, S.M. Student and staff engagement: Developing an engagement framework in a faculty of education. Aust. J. Teach. Educ. 2012 , 37 , 37–45. [ Google Scholar ] [ CrossRef ]
- Meyer, K.A. Student engagement in online learning: What works and why. ASHE High. Educ. Rep. 2014 , 40 , 1–114. [ Google Scholar ] [ CrossRef ]
- Bolliger, D.U.; Martin, F. Instructor and student perceptions of online student engagement strategies. Distance Educ. 2018 , 39 , 568–583. [ Google Scholar ] [ CrossRef ]
- Muir, T.; Douglas, T.; Trimble, A. Facilitation strategies for enhancing the learning and engagement of online students. J. Univ. Teach. Learn. Pract. 2020 , 17 , 8. Available online: https://ro.uow.edu.au/jutlp/vol17/iss3/8 (accessed on 20 March 2021). [ CrossRef ]
- Aderibigbe, S.A. Online discussions as an intervention for strengthening students’ engagement in general education. J. Open Innov. Technol. Mark. Complex. 2020 , 6 , 98. [ Google Scholar ] [ CrossRef ]
- Malan, M. Student engagement in a fully online accounting module: An action research study. S. Afr. J. High. Educ. 2020 , 34 , 112–129. [ Google Scholar ] [ CrossRef ]
- Douglas, T.; James, A.; Earwaker, L.; Mather, C.; Murray, S. Online discussion boards: Improving practice and student engagement by harnessing facilitator perceptions. J. Univ. Teach. Learn. Pract. 2020 , 17 , 7. Available online: https://files.eric.ed.gov/fulltext/EJ1264456.pdf (accessed on 20 March 2021). [ CrossRef ]
- SOLAS, E.C.; Wilson, K. Lessons learned and strategies used while teaching core-curriculum science courses to english language learners at a Middle Eastern university. J. Turk. Sci. Educ. 2015 , 12 , 81–94. [ Google Scholar ]
- Delfino, A. Student engagement and academic performance of students of Partido State University. Asian J. Univ. Educ. 2019 , 15 , n1. Available online: https://files.eric.ed.gov/fulltext/EJ1222588.pdf (accessed on 25 September 2023).
- Rodgers, T. Student engagement in the e-learning process and the impact on their grades. Int. J. Cyber Soc. Educ. 2008 , 1 , 143–156, ATISR. Available online: https://www.learntechlib.org/p/209167/ (accessed on 22 September 2023).
- Barnard-Brak, L.; Paton, V.O.; Lan, W.Y. Profiles in self-regulated learning in the online learning environment. Int. Rev. Res. Open Distrib. Learn. 2010 , 11 , 61–80. [ Google Scholar ] [ CrossRef ]
- Cai, R.; Wang, Q.; Xu, J.; Zhou, L. Effectiveness of students’ self-regulated learning during the COVID-19 pandemic. Sci. Insigt. 2020 , 34 , 175–182. [ Google Scholar ] [ CrossRef ]
- Greene, J.A.; Plumley, R.D.; Urban, C.J.; Bernacki, M.L.; Gates, K.M.; Hogan, K.A.; Demetriou, C.; Panter, A.T. Modeling temporal self-regulatory processing in a higher education biology course. Learn. Instr. 2021 , 72 , 101201. [ Google Scholar ] [ CrossRef ]
- Azizah, U.; Nasrudin, H. Metacognitive skills and self-regulated learning in pre-service teachers: Role of metacognitive-based teaching materials. J. Turk. Sci. Educ. 2021 , 18 , 461–476. [ Google Scholar ] [ CrossRef ]
- Anderton, B. Using the online course to promote self-regulated learning strategies in pre-service teachers. J. Inter. Online Learn. 2006 , 5 , 156–177. Available online: https://www.ncolr.org/jiol/issues/pdf/5.2.3.pdf (accessed on 24 September 2023).
- Martín-del-Pozo, M.; Martín-Sánchez, I. Self-assessment and pre-Service teachers’ self-regulated learning in a school organisation course in hybrid learning. J. Inter. Media Educ. 2022 , 1 , 1–15. [ Google Scholar ] [ CrossRef ]
- Lewis, J.P.; Litchfield, B.C. Effects of self-regulated learning strategies on preservice teachers in an educational technology course. Education 2011 , 132 , 455–464. [ Google Scholar ]
- Kibirige, I.; Odora, R.J. Pre-service Teachers’ Use and Usefulness of Blackboard Learning Management Systems for Self-Regulated Learning. J. Educ. Technol. 2023 , 7 , 226–234. [ Google Scholar ] [ CrossRef ]
- Ejubovic, A.; Puška, A. Impact of self-regulated learning on academic performance and satisfaction of students in the online environment. Knowl. Manag. E-Learn. 2019 , 11 , 345–363. [ Google Scholar ] [ CrossRef ]
- Sletten, S.R. Investigating flipped learning: Student self-regulated learning, perceptions, and achievement in an introductory biology course. J. Sci. Educ. Technol. 2017 , 26 , 347–358. [ Google Scholar ] [ CrossRef ]
- Cho, M.-H.; Kim, B.J. Students’ self-regulation for interaction with others in online learning environments. Internet High. Educ. 2013 , 17 , 69–75. [ Google Scholar ] [ CrossRef ]
- Aşkar, P.; Umay, A. Perceived computer self-efficacy of the students in the elementary mathematics teaching programme. Hacet. Univ. J. Educ. 2001 , 21 , 1–8. [ Google Scholar ]
- Zimmerman, B.J. Self-efficacy and educational development. Self-Effic. Chang. Soc. 1995 , 1 , 202–231. [ Google Scholar ] [ CrossRef ]
- Elstad, E.; Christophersen, K.-A. Perceptions of digital competency among student teachers: Contributing to the development of student teachers’ instructional self-Efficacy in technology-rich classrooms. Educ. Sci. 2017 , 7 , 27. [ Google Scholar ] [ CrossRef ]
- Peechapol, C.; Na-Songkhla, J.; Sujiva, S.; Luangsodsai, A. An exploration of factors influencing self-efficacy in online learning: A systematic review. Int. J. Emer. Technol. Learn. 2018 , 13 , 64. [ Google Scholar ] [ CrossRef ]
- Lee, D.; Watson, S.L.; Watson, W.R. The relationships between self-Efficacy, task value, and self-regulated learning strategies in massive open online courses. Int. Rev. Res. Open Distrib. Learn. 2020 , 21 , 23–39. [ Google Scholar ] [ CrossRef ]
- Robledo, D.A.; Miguel, F.; Arizala-Pillar, G.; Errabo, D.D.; Cajimat, R.; Prudente, M.; Aguja, S. Students’ knowledge gains, self-efficacy, perceived level of engagement, and perceptions with regard to home-based biology experiments (HBEs). J. Turk. Sci. Educ. 2023 , 20 , 84–118. [ Google Scholar ] [ CrossRef ]
- Funa, A.; Gabay, R.; Deblois, E.; Lerios, L.; Jetomo, F. Exploring Filipino preservice teachers’ online self-regulated learning skills and strategies amid the COVID-19 pandemic. Soc. Sci. Hum. Open. 2023 , 7 , 100470. [ Google Scholar ] [ CrossRef ] [ PubMed ]
- Lord, H.G. Ex Post Facto Studies as a Research Method. Special Report No. 7320; 1973; ERIC. Available online: https://files.eric.ed.gov/fulltext/ED090962.pdf (accessed on 16 June 2023).
- Joaquin, J.J.B.; Biana, H.T.; Dacela, M.A. The Philippine higher education sector in the time of COVID-19. Front. Educ. 2020 , 5 , 208. [ Google Scholar ] [ CrossRef ]
- Etikan, I.; Musa, S.A.; Alkassim, R.S. Comparison of convenience sampling and purposive sampling. Am. J. Theor. Appl. Stat. 2016 , 5 , 1–4. [ Google Scholar ] [ CrossRef ]
- Statista. Perception on the effectiveness of current distance learning compared to face-to-face schooling in the Philippines as of April 2021. Educ. Sci. 2023 . Available online: https://www.statista.com/statistics/1262427/philippines-view-on-effectiveness-of-distance-learning-compared-to-face-to-face-schooling/ (accessed on 6 October 2023).
- Dixson, M.D. Measuring student engagement in the online course: The online student engagement scale (OSE). Online Learn. 2015 , 19 , n4. Available online: https://files.eric.ed.gov/fulltext/EJ1079585.pdf (accessed on 15 February 2021). [ CrossRef ]
- Jansen, R.S.; Van Leeuwen, A.; Janssen, J.; Kester, L.; Kalz, M. Validation of the self-regulated online learning questionnaire. J. Comput. High. Educ. 2017 , 29 , 6–27. [ Google Scholar ] [ CrossRef ]
- Zimmerman, W.A.; Kulikowich, J.M. Online learning self-efficacy in students with and without online learning experience. Am. J. Distance Educ. 2016 , 30 , 180–191. [ Google Scholar ] [ CrossRef ]
- Jin, W.; Prudente, M.S.; Aguja, S.E. Students’ experiences and perceptions on the use of mobile devices for learning. Adv. Sci. Lett. 2018 , 24 , 8507–8510. [ Google Scholar ] [ CrossRef ]
- Barbour, M.; Plough, C. Helping to make online learning less isolating. TechTrends 2009 , 53 , 57. Available online: https://www.academia.edu/download/79948695/Barbour2009.pdf (accessed on 14 June 2022).
- Buelow, J.R.; Barry, T.; Rich, L.E. Supporting learning engagement with online students. Online Learn. 2018 , 22 , 313–340. [ Google Scholar ] [ CrossRef ]
- Briones, M.R.B.; Errabo, D.D.R. Student’s voice of e-learning: Implication to online teaching practice. In Proceedings of the ICMET 2021: 2021 3rd International Conference on Modern Educational Technology (ICMET 2021), Jakarta, Indonesia, 21–23 May 2021; Association of Computing Machinery: New York, NY, USA, 2021. [ Google Scholar ] [ CrossRef ]
- Yavuzalp, N.; Bahcivan, E. The online learning self-efficacy scale: Its adaptation into Turkish and interpretation according to various variables. Turk. Online J. Distance Educ. 2020 , 21 , 31–44. [ Google Scholar ] [ CrossRef ]
- Beltran-Cruz, M.; Cruz, S.B.B. The use of internet-based social enhancing student’s learning experiences in biological sciences. High. Learn. Res. Com. 2013 , 3 , 68–80. [ Google Scholar ] [ CrossRef ]
- Alexiou, A.; Paraskeva, F. Enhancing self-regulated learning skills through the implementation of an e-portfolio tool. Procedia-Soc. Behav. Sci. 2010 , 2 , 3048–3054. [ Google Scholar ] [ CrossRef ]
- Dianati, S.; Iwashita, N.; Vasquez, C. Flipped classroom experiences: Comparing undergraduate and postgraduate perceptions of self-regulated learning. Issues Educ. Res. 2022 , 32 , 473–493. [ Google Scholar ]
- Tanti; Maison; Mukminin, A.; Syahria; Habibi, A.; Syamsurizal, S. Exploring the relationship between preservice science teachers’ beliefs and self-regulated strategies of studying physics: A structural equation model. J. Turk. Sci. Educ. 2018 , 15 , 79–92. [ Google Scholar ]
Click here to enlarge figure
Demographics | Number of Respondents | Percentage | |
---|---|---|---|
Age group | 18–22 years old | 350 | 94.09 |
23–26 years old | 21 | 5.65 | |
27 years old and above | 1 | 0.26 | |
Gender preference | Women | 157 | 42.20 |
Men | 186 | 49.19 | |
LGBTQ+ | 29 | 7.80 | |
Internet access | Via school access | 4 | 1.08 |
Via home broadband | 222 | 59.67 | |
Via mobile data | 146 | 39.25 | |
Internet signal | Poor (B/s) | 35 | 9.41 |
Good (KB/s) | 182 | 48.92 | |
Better (MB/s) | 147 | 39.52 | |
Best (GB/s) | 8 | 2.15 | |
Region of residence | National Capital Region (NCR) | 183 | 49.19 |
Region I—Ilocos Region | 2 | 0.54 | |
Region III—Central Luzon | 33 | 8.87 | |
Region IV-A—CALABARZON | 66 | 17.74 | |
Region V—Bicol Region | 8 | 2.15 | |
Region VIII—Eastern Visayas | 39 | 10.48 | |
Region IX—Zamboanga Peninsula | 1 | 0.27 | |
Region XIII—CARAGA | 38 | 10.22 | |
MIMAROPA | 2 | 0.54 | |
Most preferred time to study online | Morning | 91 | 24.46 |
Afternoon | 58 | 15.86 | |
Evening | 173 | 46.51 | |
Dawn | 49 | 13.17 |
Characteristics | (5) Very Characteristic of Me (%) | (4) Characteristic of Me (%) | (3) Moderately Characteristic of Me (%) | (2) Not Really Characteristic of Me (%) | (1) Not at All Characteristic of Me (%) | Mean | SD |
---|---|---|---|---|---|---|---|
1. Making sure to study on a regular basis | 20.70 | 43.55 | 27.96 | 7.26 | 0.54 | ||
2. Putting forth effort | 30.65 | 48.12 | 18.28 | 2.42 | 0.54 | ||
3. Keeping up with the readings | 14.78 | 35.48 | 36.83 | 10.75 | 2.15 | ||
4. Looking over class notes before going online to make sure I understand the material | 26.88 | 44.62 | 25.00 | 3.23 | 0.27 | ||
5. Being organized | 30.65 | 40.86 | 22.04 | 4.03 | 2.42 | ||
6. Taking good notes on readings, PowerPoints, or video lectures | 27.96 | 36.56 | 27.96 | 6.45 | 1.08 | ||
7. Listening/reading carefully | 32.53 | 47.31 | 17.74 | 2.15 | 0.27 | ||
8. Finding ways to make the course material relevant to my life | 28.23 | 41.67 | 23.39 | 5.38 | 1.34 | ||
9. Applying the course material to my life | 23.92 | 43.28 | 26.08 | 5.91 | 0.81 | ||
10. Finding ways to make the course material relevant to me | 25.54 | 46.77 | 21.77 | 4.84 | 1.08 | ||
11. Having a strong desire to learn the material | 28.76 | 45.16 | 22.58 | 2.69 | 0.81 | ||
12. Having fun when engaging in online chats, discussions, or email with the instructor or classmates | 25.81 | 39.52 | 23.92 | 8.33 | 2.42 | ||
13. Participating actively in small-group discussion forums | 20.70 | 41.94 | 27.15 | 7.80 | 2.42 | ||
14. Helping fellow students | 40.59 | 40.32 | 15.32 | 2.96 | 0.81 | ||
15. Attaining a good grade | 30.11 | 48.39 | 18.28 | 2.69 | 0.54 | ||
16. Achieving good results on tests/quizzes | 20.70 | 52.96 | 22.85 | 2.69 | 0.81 | ||
17. Engaging in conversations online (chats/discussions, email, etc.) | 25.54 | 41.40 | 24.19 | 6.72 | 2.15 | ||
18. Posting in the discussion forum regularly | 14.78 | 26.61 | 37.63 | 12.63 | 8.33 | ||
19. Getting to know other students in the class | 27.96 | 38.71 | 20.97 | 8.33 | 4.03 | ||
Characteristics | (5) Very True for Me (%) | (4) True for Me (%) | (3) Moderately True for Me (%) | (2) Not Really True for Me (%) | (1) Not at All True for Me (%) | Mean | SD |
---|---|---|---|---|---|---|---|
1. I think about what I really need to learn before I begin a task in this online course. | 26.34 | 45.16 | 24.73 | 3.76 | 0 | ||
2. I ask myself questions about what I will be studying before I begin learning in this online course. | 25.54 | 44.62 | 25.81 | 3.49 | 0.54 | ||
3. I set short-term (daily or weekly) goals as well as long-term goals (monthly or for the whole online course). | 23.12 | 42.47 | 25.27 | 6.99 | 2.15 | ||
4. I set goals to help me manage my studying time for this online course. | 35.84 | 37.63 | 19.35 | 5.91 | 1.61 | ||
5. I set specific goals before I begin a task in this online course. | 27.42 | 42.47 | 23.12 | 6.18 | 0.81 | ||
6. I think of alternative ways of solving a problem and choose the best one for this online course. | 30.38 | 49.19 | 18.55 | 1.88 | 0 | ||
7. I try to use strategies in this online course that have worked in the past. | 31.18 | 44.89 | 19.62 | 3.76 | 0.54 | ||
8. I have a specific purpose for each strategy I use in this online course. | 22.58 | 45.16 | 27.15 | 4.84 | 0.27 | ||
9. I am aware of what strategies I use when I study for this online course. | 20.97 | 47.58 | 26.34 | 4.03 | 1.08 | ||
10. Although we do not have to attend daily classes, I still try to distribute my studying time for this online course evenly throughout the days of the week. | 20.43 | 43.28 | 27.15 | 6.99 | 2.15 | ||
11. I periodically review to help me understand important relationships in this online course. | 17.74 | 45.70 | 27.42 | 8.06 | 1.08 | ||
12. I find myself pausing regularly to check my comprehension of this online course. | 22.31 | 44.89 | 26.34 | 5.38 | 1.08 | ||
13. I ask myself questions about how well I am performing while learning something in this online course. | 27.15 | 43.01 | 24.73 | 4.84 | 0.27 | ||
14. I think about what I have learned after I finish working on material in this online course. | 22.58 | 48.39 | 23.12 | 5.38 | 0.54 | ||
15. I ask myself how well I accomplished my goals once I have finished working on material in this online course. | 24.73 | 49.46 | 22.31 | 3.23 | 0.27 | ||
16. I change strategies when I do not make progress while learning in this online course. | 27.96 | 42.74 | 23.12 | 5.11 | 1. 08 | ||
17. I find myself analyzing the usefulness of strategies while I study for this online course. | 23.12 | 47.04 | 24.73 | 4.57 | 0.54 | ||
18. I ask myself if there were other ways to complete things after I finish learning material from this online course. | 24.19 | 47.85 | 23.12 | 3.76 | 1.08 | ||
19. I find it difficult to stick to a study schedule for this online course. | 30.65 | 38.17 | 22.04 | 7.80 | 1.34 | ||
20. I make sure I keep up with weekly readings and assignments for this online course. | 25.27 | 45.70 | 21.77 | 5.91 | 1.34 | ||
21. I often find that I do not spend very much time on this online course because of other activities. | 22.58 | 37.10 | 28.49 | 9.41 | 2.42 | ||
22. I choose a location where I can avoid an excessive amount of distraction when studying for this online course. | 39.78 | 32.80 | 19.89 | 6.18 | 1.34 | ||
23. I try to find a comfortable place to study for this online course. | 37.90 | 33.60 | 19.89 | 6.18 | 2.42 | ||
24. I know where I can study most efficiently for this online course. | 29.03 | 39.52 | 24.19 | 5.91 | 1.34 | ||
25. I have a regular place set aside for studying for this online course. | 27.96 | 41.94 | 20.43 | 7.53 | 2.15 | ||
26. I know what the instructor expects me to learn in this online course. | 20.16 | 44.35 | 30.11 | 4.84 | 0.54 | ||
27. When I am feeling bored when studying for this online course, I force myself to pay attention. | 22.04 | 36.83 | 28.69 | 9.41 | 4.03 | ||
28. When my mind begins to wander during a learning session for this online course, I make a special effort to keep concentrating. | 22.04 | 42.47 | 27.42 | 6.72 | 1.34 | ||
29. When I begin to lose interest for this online course, I push myself even further. | 23.92 | 35.48 | 29.30 | 8.87 | 2.42 | ||
30. I work hard to perform well in this online course even if I do not like the tasks I have to perform. | 29.57 | 43.28 | 21.24 | 4.84 | 1.08 | ||
31. Even when materials in this online course are dull and uninteresting, I manage to keep working until I finish. | 26.88 | 43.82 | 22.04 | 6.18 | 1.08 | ||
32. When I do not fully understand something, I ask other course members in this online course for ideas. | 33.87 | 38.44 | 21.24 | 4.57 | 1.88 | ||
33. I share my problems with my classmates in this course online so that we know what we are struggling with and how to solve our problems. | 31.99 | 32.53 | 22.04 | 8.87 | 4.57 | ||
34. I am persistent in receiving help from the instructor of this online course. | 18.01 | 35.22 | 31.99 | 8.06 | 6.72 | ||
35. When I am not sure about material in this online course, I check with other people. | 31.99 | 40.59 | 21.51 | 3.23 | 2.69 | ||
36. I communicate with my classmates to find out how I am performing in this online course. | 37.75 | 30.65 | 23.66 | 4.57 | 5.38 | ||
Characteristics | (4) Strongly Agree (%) | (3) Agree (%) | (2) Disagree (%) | (1) Strongly Disagree (%) | Mean | SD |
---|---|---|---|---|---|---|
1. I can navigate online course materials efficiently | 31.99 | 58.60 | 9.41 | 0 | ||
2. I can find the course syllabus online | 36.02 | 53.49 | 9.41 | 1.08 | ||
3. I can effectively communicate with my instructor via e-mail | 26.08 | 48.12 | 23.92 | 1.88 | ||
4. I can communicate effectively with technical support via e-mail or live online chat | 27.69 | 48.12 | 20.97 | 3.23 | ||
5. I can submit assignments using an online drop box | 45.70 | 41.94 | 10.75 | 1.61 | ||
6. I can overcome technical difficulties on my own | 37.90 | 50.00 | 11.02 | 1.08 | ||
7. I can navigate the online grade book | 26.34 | 55.11 | 16.13 | 2.42 | ||
8. I manage my time effectively | 33.33 | 43.82 | 17.74 | 5.11 | ||
9. I complete all assignments on time | 39.25 | 44.09 | 13.71 | 2.96 | ||
10. I am able learn how to use a new type of technology efficiently | 43.01 | 45.97 | 10.22 | 0.81 | ||
11. I can learn without being in the same room as the instructor | 26.34 | 52.69 | 18.82 | 2.15 | ||
12. I can learn without being in the same room as the other students | 27.42 | 51.34 | 18.28 | 2.96 | ||
13. I often search the internet to find the answer to a course-related question | 37.90 | 48.12 | 11.83 | 2.15 | ||
14. I often search the online course materials | 40.32 | 48.66 | 10.48 | 0.54 | ||
15. I often communicate using asynchronous technologies (discussion boards, e-mail, etc.) | 33.87 | 50.54 | 12.90 | 2.69 | ||
16. I meet deadlines with very few reminders | 30.11 | 54.03 | 13.17 | 2.69 | ||
17. I can complete a group project entirely online | 38.71 | 49.73 | 11.02 | 0.54 | ||
18. I use synchronous technology (such as Skype) to communicate with others | 47.04 | 44.89 | 7.80 | 0.27 | ||
19. I can focus on schoolwork when faced with distractions | 25.00 | 49.46 | 20.70 | 4.84 | ||
20. I often develop and follow a plan for completing all required work on time | 30.91 | 55.91 | 12.37 | 0.81 | ||
21. I use the library’s online resources efficiently | 20.97 | 42.20 | 24.19 | 12.63 | ||
22. When a problem arises, I promptly ask questions in the appropriate forum (e-mail, discussion board, etc.) | 35.48 | 44.35 | 17.20 | 2.96 | ||
Characteristics | Men | Women | LGBTQ+ | |||
---|---|---|---|---|---|---|
Mean | SD | Mean | SD | Mean | SD | |
1. I can navigate online course materials efficiently | 3.29 | 0.60 | 3.26 | 0.61 | 2.97 | 0.50 |
2. I can find the course syllabus online | 3.20 | 0.73 | 3.32 | 0.59 | 3.14 | 0.58 |
3. I can communicate effectively with my instructor via e-mail | 2.97 | 0.80 | 3.01 | 0.71 | 2.93 | 0.80 |
4. I can communicate effectively with technical support via e-mail or live online chat | 3.01 | 0.77 | 3.00 | 0.80 | 3.00 | 0.79 |
5. I can submit assignments using an online drop box | 3.26 | 0.76 | 3.36 | 0.68 | 3.41 | 0.73 |
6. I can overcome technical difficulties on my own | 3.28 | 0.68 | 3.24 | 0.69 | 3.07 | 0.70 |
7. I can navigate the online grade book | 3.03 | 0.74 | 3.10 | 0.67 | 3.00 | 0.89 |
8. I manage my time effectively | 3.08 | 0.86 | 3.04 | 0.84 | 2.97 | 0.78 |
9. I complete all assignments on time | 3.26 | 0.79 | 3.16 | 0.79 | 2.97 | 0.91 |
10. I can learn to use a new type of technology efficiently | 3.32 | 0.64 | 3.30 | 0.74 | 3.31 | 0.66 |
11. I can learn without being in the same room as the instructor | 3.10 | 0.72 | 2.98 | 0.71 | 3.90 | 0.90 |
12. I can learn without being in the same room as the other students | 3.09 | 0.74 | 2.99 | 0.75 | 3.90 | 0.90 |
13. I often search the internet to find the answer to a course-related question | 3.20 | 0.75 | 3.27 | 0.70 | 3.03 | 0.78 |
14. I often search for the online course materials | 3.26 | 0.69 | 3.33 | 0.63 | 3.24 | 0.74 |
15. I often communicate using asynchronous technologies (discussion boards, e-mail, etc.) | 3.18 | 0.74 | 3.20 | 0.69 | 2.79 | 0.94 |
16. I meet deadlines with very few reminders | 3.11 | 0.73 | 3.15 | 0.71 | 2.97 | 0.82 |
17. I can complete a group project entirely online | 3.31 | 0.67 | 3.21 | 0.66 | 3.28 | 0.70 |
18. I use synchronous technology to communicate with others (such as Skype) | 3.35 | 0.65 | 3.43 | 0.61 | 3.34 | 0.72 |
19. I can focus on schoolwork when faced with distractions | 3.06 | 0.77 | 2.85 | 0.83 | 2.72 | 0.84 |
20. I often develop and follow a plan for completing all required work on time | 3.17 | 0.65 | 3.20 | 0.67 | 2.97 | 0.78 |
21. I use the library’s online resources efficiently | 2.70 | 0.98 | 2.76 | 0.87 | 2.59 | 1.02 |
22. When a problem arises, I promptly ask questions in the appropriate forum (e-mail, discussion board, etc.) | 3.18 | 0.79 | 3.10 | 0.79 | 2.90 | 0.82 |
The statements, opinions and data contained in all publications are solely those of the individual author(s) and contributor(s) and not of MDPI and/or the editor(s). MDPI and/or the editor(s) disclaim responsibility for any injury to people or property resulting from any ideas, methods, instructions or products referred to in the content. |
Share and Cite
Briones, M.R.; Prudente, M.; Errabo, D.D. Characteristics of Filipino Online Learners: A Survey of Science Education Students’ Engagement, Self-Regulation, and Self-Efficacy. Educ. Sci. 2023 , 13 , 1131. https://doi.org/10.3390/educsci13111131
Briones MR, Prudente M, Errabo DD. Characteristics of Filipino Online Learners: A Survey of Science Education Students’ Engagement, Self-Regulation, and Self-Efficacy. Education Sciences . 2023; 13(11):1131. https://doi.org/10.3390/educsci13111131
Briones, Mary Rose, Maricar Prudente, and Denis Dyvee Errabo. 2023. "Characteristics of Filipino Online Learners: A Survey of Science Education Students’ Engagement, Self-Regulation, and Self-Efficacy" Education Sciences 13, no. 11: 1131. https://doi.org/10.3390/educsci13111131
Article Metrics
Article access statistics, further information, mdpi initiatives, follow mdpi.
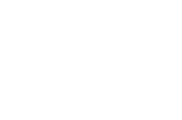
Subscribe to receive issue release notifications and newsletters from MDPI journals
- Title & authors
Ignacio, Aris E. "Online Classes and Learning in the Philippines During the Covid-19 Pandemic." International Journal on Integrated Education , vol. 4, no. 3, 2021, pp. 1-6, doi: 10.31149/ijie.v4i3.1301 .
Download citation file:
The COVID-19 pandemic brought great disruption to all aspects of life specifically on how classes were conducted both in an offline and online modes. The sudden shift to purely online method of teaching and learning was a result of the lockdowns that were imposed by the Philippine government. While some institutions have dealt with the situation by shutting down operations, others continued to deliver instructions and lessons using the Internet and different applications that support online learning. The continuation of classes online had caused several issues from students and teachers ranging from lack of technology to mental health matters. Finally, recommendations were asserted to mitigate the presented concerns and improve the delivery of the necessary quality education to the intended learners.
Table of contents

Exploring the Online Learning Experience of Filipino College Students During Covid-19 Pandemic
- Louie Giray College of Education, Polytechnic University of the Philipines, Taguig City, Philippines
- Daxjhed Gumalin College of Education, Polytechnic University of the Philipines, Taguig City, Philippines
- Jomarie Jacob College of Education, Polytechnic University of the Philipines, Taguig City, Philippines
- Karl Villacorta College of Education, Polytechnic University of the Philipines, Taguig City, Philippines
This study was endeavored to understand the online learning experience of Filipino college students enrolled in the academic year 2020-2021 during the COVID-19 pandemic. The data were obtained through an open-ended qualitative survey. The responses were analyzed and interpreted using thematic analysis. A total of 71 Filipino college students from state and local universities in the Philippines participated in this study. Four themes were classified from the collected data: (1) negative views toward online schooling, (2) positive views toward online schooling, (3) difficulties encountered in online schooling, and (4) motivation to continue studying. The results showed that although many Filipino college students find online learning amid the COVID-19 pandemic to be a positive experience such as it provides various conveniences, eliminates the necessity of public transportation amid the COVID-19 pandemic, among others, a more significant number of respondents believe otherwise. The majority of the respondents shared a general difficulty adjusting toward the new online learning setup because of problems related to technology and Internet connectivity, mental health, finances, and time and space management. A large portion of students also got their motivation to continue studying despite the pandemic from fear of being left behind, parental persuasion, and aspiration to help the family.
- EndNote - EndNote format (Macintosh & Windows)
- ProCite - RIS format (Macintosh & Windows)
- Reference Manager - RIS format (Windows only)

This work is licensed under a Creative Commons Attribution-ShareAlike 4.0 International License .
Authors who publish with this journal agree to the following terms: (1) Authors retain copyright and grant the journal right of first publication with the work simultaneously licensed under a Creative Commons Attribution-ShareAlike 4.0 International License (CC-BY-SA) that allows others to share the work with an acknowledgment of the work's authorship and initial publication in this journal; (2) Authors are able to enter into separate, additional contractual arrangements for the non-exclusive distribution of the journal's published version of the work (e.g., post it to an institutional repository or publish it in a book), with an acknowledgment of its initial publication in this journal; (3) Authors are permitted and encouraged to post their work online (e.g., in institutional repositories or on their website) prior to and during the submission process, as it can lead to productive exchanges, as well as earlier and greater citation of published work ( See The Effect of Open Access ).
Content Use Policy

Click through the PLOS taxonomy to find articles in your field.
For more information about PLOS Subject Areas, click here .
Loading metrics
Open Access
Study Protocol
Assessing the effect of the COVID-19 pandemic, shift to online learning, and social media use on the mental health of college students in the Philippines: A mixed-method study protocol
Roles Funding acquisition, Writing – original draft
Affiliation College of Medicine, University of the Philippines, Manila, Philippines
Roles Methodology, Supervision, Visualization, Writing – original draft, Writing – review & editing
Affiliations Department of Clinical Epidemiology, College of Medicine, University of the Philippines, Manila, Philippines, Institute of Clinical Epidemiology, National Institutes of Health, University of the Philippines, Manila, Philippines

Roles Methodology
Affiliation Department of Psychiatry, College of Medicine, University of the Philippines, Manila, Philippines
Roles Conceptualization, Funding acquisition, Project administration, Supervision, Writing – original draft, Writing – review & editing
* E-mail: [email protected]
- Leonard Thomas S. Lim,
- Zypher Jude G. Regencia,
- J. Rem C. Dela Cruz,
- Frances Dominique V. Ho,
- Marcela S. Rodolfo,
- Josefina Ly-Uson,
- Emmanuel S. Baja
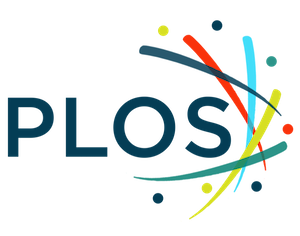
- Published: May 3, 2022
- https://doi.org/10.1371/journal.pone.0267555
- Peer Review
- Reader Comments
Introduction
The COVID-19 pandemic declared by the WHO has affected many countries rendering everyday lives halted. In the Philippines, the lockdown quarantine protocols have shifted the traditional college classes to online. The abrupt transition to online classes may bring psychological effects to college students due to continuous isolation and lack of interaction with fellow students and teachers. Our study aims to assess Filipino college students’ mental health status and to estimate the effect of the COVID-19 pandemic, the shift to online learning, and social media use on mental health. In addition, facilitators or stressors that modified the mental health status of the college students during the COVID-19 pandemic, quarantine, and subsequent shift to online learning will be investigated.
Methods and analysis
Mixed-method study design will be used, which will involve: (1) an online survey to 2,100 college students across the Philippines; and (2) randomly selected 20–40 key informant interviews (KIIs). Online self-administered questionnaire (SAQ) including Depression, Anxiety, and Stress Scale (DASS-21) and Brief-COPE will be used. Moreover, socio-demographic factors, social media usage, shift to online learning factors, family history of mental health and COVID-19, and other factors that could affect mental health will also be included in the SAQ. KIIs will explore factors affecting the student’s mental health, behaviors, coping mechanism, current stressors, and other emotional reactions to these stressors. Associations between mental health outcomes and possible risk factors will be estimated using generalized linear models, while a thematic approach will be made for the findings from the KIIs. Results of the study will then be triangulated and summarized.
Ethics and dissemination
Our study has been approved by the University of the Philippines Manila Research Ethics Board (UPMREB 2021-099-01). The results will be actively disseminated through conference presentations, peer-reviewed journals, social media, print and broadcast media, and various stakeholder activities.
Citation: Lim LTS, Regencia ZJG, Dela Cruz JRC, Ho FDV, Rodolfo MS, Ly-Uson J, et al. (2022) Assessing the effect of the COVID-19 pandemic, shift to online learning, and social media use on the mental health of college students in the Philippines: A mixed-method study protocol. PLoS ONE 17(5): e0267555. https://doi.org/10.1371/journal.pone.0267555
Editor: Elisa Panada, UNITED KINGDOM
Received: June 9, 2021; Accepted: April 11, 2022; Published: May 3, 2022
Copyright: © 2022 Lim et al. This is an open access article distributed under the terms of the Creative Commons Attribution License , which permits unrestricted use, distribution, and reproduction in any medium, provided the original author and source are credited.
Funding: This project is being supported by the American Red Cross through the Philippine Red Cross and Red Cross Youth. The funder will not have a role in the study design, data collection and analysis, decision to publish, or preparation of the manuscript.
Competing interests: The authors have declared that no competing interests exist.
The World Health Organization (WHO) declared the Coronavirus 2019 (COVID-19) outbreak as a global pandemic, and the Philippines is one of the 213 countries affected by the disease [ 1 ]. To reduce the virus’s transmission, the President imposed an enhanced community quarantine in Luzon, the country’s northern and most populous island, on March 16, 2020. This lockdown manifested as curfews, checkpoints, travel restrictions, and suspension of business and school activities [ 2 ]. However, as the virus is yet to be curbed, varying quarantine restrictions are implemented across the country. In addition, schools have shifted to online learning, despite financial and psychological concerns [ 3 ].
Previous outbreaks such as the swine flu crisis adversely influenced the well-being of affected populations, causing them to develop emotional problems and raising the importance of integrating mental health into medical preparedness for similar disasters [ 4 ]. In one study conducted on university students during the swine flu pandemic in 2009, 45% were worried about personally or a family member contracting swine flu, while 10.7% were panicking, feeling depressed, or emotionally disturbed. This study suggests that preventive measures to alleviate distress through health education and promotion are warranted [ 5 ].
During the COVID-19 pandemic, researchers worldwide have been churning out studies on its psychological effects on different populations [ 6 – 9 ]. The indirect effects of COVID-19, such as quarantine measures, the infection of family and friends, and the death of loved ones, could worsen the overall mental wellbeing of individuals [ 6 ]. Studies from 2020 to 2021 link the pandemic to emotional disturbances among those in quarantine, even going as far as giving vulnerable populations the inclination to commit suicide [ 7 , 8 ], persistent effect on mood and wellness [ 9 ], and depression and anxiety [ 10 ].
In the Philippines, a survey of 1,879 respondents measuring the psychological effects of COVID-19 during its early phase in 2020 was released. Results showed that one-fourth of respondents reported moderate-to-severe anxiety, while one-sixth reported moderate-to-severe depression [ 11 ]. In addition, other local studies in 2020 examined the mental health of frontline workers such as nurses and physicians—placing emphasis on the importance of psychological support in minimizing anxiety [ 12 , 13 ].
Since the first wave of the pandemic in 2020, risk factors that could affect specific populations’ psychological well-being have been studied [ 14 , 15 ]. A cohort study on 1,773 COVID-19 hospitalized patients in 2021 found that survivors were mainly troubled with fatigue, muscle weakness, sleep difficulties, and depression or anxiety [ 16 ]. Their results usually associate the crisis with fear, anxiety, depression, reduced sleep quality, and distress among the general population.
Moreover, the pandemic also exacerbated the condition of people with pre-existing psychiatric disorders, especially patients that live in high COVID-19 prevalence areas [ 17 ]. People suffering from mood and substance use disorders that have been infected with COVID-19 showed higher suicide risks [ 7 , 18 ]. Furthermore, a study in 2020 cited the following factors contributing to increased suicide risk: social isolation, fear of contagion, anxiety, uncertainty, chronic stress, and economic difficulties [ 19 ].
Globally, multiple studies have shown that mental health disorders among university student populations are prevalent [ 13 , 20 – 22 ]. In a 2007 survey of 2,843 undergraduate and graduate students at a large midwestern public university in the United States, the estimated prevalence of any depressive or anxiety disorder was 15.6% and 13.0% for undergraduate and graduate students, respectively [ 20 ]. Meanwhile, in a 2013 study of 506 students from 4 public universities in Malaysia, 27.5% and 9.7% had moderate and severe or extremely severe depression, respectively; 34% and 29% had moderate and severe or extremely severe anxiety, respectively [ 21 ]. In China, a 2016 meta-analysis aiming to establish the national prevalence of depression among university students analyzed 39 studies from 1995 to 2015; the meta-analysis found that the overall prevalence of depression was 23.8% across all studies that included 32,694 Chinese university students [ 23 ].
A college student’s mental status may be significantly affected by the successful fulfillment of a student’s role. A 2013 study found that acceptable teaching methods can enhance students’ satisfaction and academic performance, both linked to their mental health [ 24 ]. However, online learning poses multiple challenges to these methods [ 3 ]. Furthermore, a 2020 study found that students’ mental status is affected by their social support systems, which, in turn, may be jeopardized by the COVID-19 pandemic and the physical limitations it has imposed. Support accessible to a student through social ties to other individuals, groups, and the greater community is a form of social support; university students may draw social support from family, friends, classmates, teachers, and a significant other [ 25 , 26 ]. Among individuals undergoing social isolation and distancing during the COVID-19 pandemic in 2020, social support has been found to be inversely related to depression, anxiety, irritability, sleep quality, and loneliness, with higher levels of social support reducing the risk of depression and improving sleep quality [ 27 ]. Lastly, it has been shown in a 2020 study that social support builds resilience, a protective factor against depression, anxiety, and stress [ 28 ]. Therefore, given the protective effects of social support on psychological health, a supportive environment should be maintained in the classroom. Online learning must be perceived as an inclusive community and a safe space for peer-to-peer interactions [ 29 ]. This is echoed in another study in 2019 on depressed students who narrated their need to see themselves reflected on others [ 30 ]. Whether or not online learning currently implemented has successfully transitioned remains to be seen.
The effect of social media on students’ mental health has been a topic of interest even before the pandemic [ 31 , 32 ]. A systematic review published in 2020 found that social media use is responsible for aggravating mental health problems and that prominent risk factors for depression and anxiety include time spent, activity, and addiction to social media [ 31 ]. Another systematic review published in 2016 argues that the nature of online social networking use may be more important in influencing the symptoms of depression than the duration or frequency of the engagement—suggesting that social rumination and comparison are likely to be candidate mediators in the relationship between depression and social media [ 33 ]. However, their findings also suggest that the relationship between depression and online social networking is complex and necessitates further research to determine the impact of moderators and mediators that underly the positive and negative impact of online social networking on wellbeing [ 33 ].
Despite existing studies already painting a picture of the psychological effects of COVID-19 in the Philippines, to our knowledge, there are still no local studies contextualized to college students living in different regions of the country. Therefore, it is crucial to elicit the reasons and risk factors for depression, stress, and anxiety and determine the potential impact that online learning and social media use may have on the mental health of the said population. In turn, the findings would allow the creation of more context-specific and regionalized interventions that can promote mental wellness during the COVID-19 pandemic.
Materials and methods
The study’s general objective is to assess the mental health status of college students and determine the different factors that influenced them during the COVID-19 pandemic. Specifically, it aims:
- To describe the study population’s characteristics, categorized by their mental health status, which includes depression, anxiety, and stress.
- To determine the prevalence and risk factors of depression, anxiety, and stress among college students during the COVID-19 pandemic, quarantine, and subsequent shift to online learning.
- To estimate the effect of social media use on depression, anxiety, stress, and coping strategies towards stress among college students and examine whether participant characteristics modified these associations.
- To estimate the effect of online learning shift on depression, anxiety, stress, and coping strategies towards stress among college students and examine whether participant characteristics modified these associations.
- To determine the facilitators or stressors among college students that modified their mental health status during the COVID-19 pandemic, quarantine, and subsequent shift to online learning.
Study design
A mixed-method study design will be used to address the study’s objectives, which will include Key Informant Interviews (KIIs) and an online survey. During the quarantine period of the COVID-19 pandemic in the Philippines from April to November 2021, the study shall occur with the population amid community quarantine and an abrupt transition to online classes. Since this is the Philippines’ first study that will look at the prevalence of depression, anxiety, and stress among college students during the COVID-19 pandemic, quarantine, and subsequent shift to online learning, the online survey will be utilized for the quantitative part of the study design. For the qualitative component of the study design, KIIs will determine facilitators or stressors among college students that modified their mental health status during the quarantine period.
Study population
The Red Cross Youth (RCY), one of the Philippine Red Cross’s significant services, is a network of youth volunteers that spans the entire country, having active members in Luzon, Visayas, and Mindanao. The group is clustered into different age ranges, with the College Red Cross Youth (18–25 years old) being the study’s population of interest. The RCY has over 26,060 students spread across 20 chapters located all over the country’s three major island groups. The RCY is heterogeneously composed, with some members classified as college students and some as out-of-school youth. Given their nationwide scope, disseminating information from the national to the local level is already in place; this is done primarily through email, social media platforms, and text blasts. The research team will leverage these platforms to distribute the online survey questionnaire.
In addition, the online survey will also be open to non-members of the RCY. It will be disseminated through social media and engagements with different university administrators in the country. Stratified random sampling will be done for the KIIs. The KII participants will be equally coming from the country’s four (4) primary areas: 5–10 each from the national capital region (NCR), Luzon, Visayas, and Mindanao, including members and non-members of the RCY.
Inclusion and exclusion criteria
The inclusion criteria for the online survey will include those who are 18–25 years old, currently enrolled in a university, can provide consent for the study, and are proficient in English or Filipino. The exclusion criteria will consist of those enrolled in graduate-level programs (e.g., MD, JD, Master’s, Doctorate), out-of-school youth, and those whose current curricula involve going on duty (e.g., MDs, nursing students, allied medical professions, etc.). The inclusion criteria for the KIIs will include online survey participants who are 18–25 years old, can provide consent for the study, are proficient in English or Filipino, and have access to the internet.
Sample size
A continuity correction method developed by Fleiss et al. (2013) was used to calculate the sample size needed [ 34 ]. For a two-sided confidence level of 95%, with 80% power and the least extreme odds ratio to be detected at 1.4, the computed sample size was 1890. With an adjustment for an estimated response rate of 90%, the total sample size needed for the study was 2,100. To achieve saturation for the qualitative part of the study, 20 to 40 participants will be randomly sampled for the KIIs using the respondents who participated in the online survey [ 35 ].
Study procedure
Self-administered questionnaire..
The study will involve creating, testing, and distributing a self-administered questionnaire (SAQ). All eligible study participants will answer the SAQ on socio-demographic factors such as age, sex, gender, sexual orientation, residence, household income, socioeconomic status, smoking status, family history of mental health, and COVID-19 sickness of immediate family members or friends. The two validated survey tools, Depression, Anxiety, and Stress Scale (DASS-21) and Brief-COPE, will be used for the mental health outcome assessment [ 36 – 39 ]. The DASS-21 will measure the negative emotional states of depression, anxiety, and stress [ 40 ], while the Brief-COPE will measure the students’ coping strategies [ 41 ].
For the exposure assessment of the students to social media and shift to online learning, the total time spent on social media (TSSM) per day will be ascertained by querying the participants to provide an estimated time spent daily on social media during and after their online classes. In addition, students will be asked to report their use of the eight commonly used social media sites identified at the start of the study. These sites include Facebook, Twitter, Instagram, LinkedIn, Pinterest, TikTok, YouTube, and social messaging sites Viber/WhatsApp and Facebook Messenger with response choices coded as "(1) never," "(2) less often," "(3) every few weeks," "(4) a few times a week," and “(5) daily” [ 42 – 44 ]. Furthermore, a global frequency score will be calculated by adding the response scores from the eight social media sites. The global frequency score will be used as an additional exposure marker of students to social media [ 45 ]. The shift to online learning will be assessed using questions that will determine the participants’ satisfaction with online learning. This assessment is comprised of 8 items in which participants will be asked to respond on a 5-point Likert scale ranging from ‘strongly disagree’ to ‘strongly agree.’
The online survey will be virtually distributed in English using the Qualtrics XM™ platform. Informed consent detailing the purpose, risks, benefits, methods, psychological referrals, and other ethical considerations will be included before the participants are allowed to answer the survey. Before administering the online survey, the SAQ shall undergo pilot testing among twenty (20) college students not involved with the study. It aims to measure total test-taking time, respondent satisfaction, and understandability of questions. The survey shall be edited according to the pilot test participant’s responses. Moreover, according to the Philippines’ Data Privacy Act, all the answers will be accessible and used only for research purposes.
Key informant interviews.
The research team shall develop the KII concept note, focusing on the extraneous factors affecting the student’s mental health, behaviors, and coping mechanism. Some salient topics will include current stressors (e.g., personal, academic, social), emotional reactions to these stressors, and how they wish to receive support in response to these stressors. The KII will be facilitated by a certified psychologist/psychiatrist/social scientist and research assistants using various online video conferencing software such as Google Meet, Skype, or Zoom. All the KIIs will be recorded and transcribed for analysis. Furthermore, there will be a debriefing session post-KII to address the psychological needs of the participants. Fig 1 presents the diagrammatic flowchart of the study.
- PPT PowerPoint slide
- PNG larger image
- TIFF original image
https://doi.org/10.1371/journal.pone.0267555.g001
Data analyses
Quantitative data..
Descriptive statistics will be calculated, including the prevalence of mental health outcomes such as depression, anxiety, stress, and coping strategies. In addition, correlation coefficients will be estimated to assess the relations among the different mental health outcomes, covariates, and possible risk factors.
Several study characteristics as effect modifiers will also be assessed, including sex, gender, sexual orientation, family income, smoking status, family history of mental health, and Covid-19. We will include interaction terms between the dichotomized modifier variable and markers of social media use (total TSSM and global frequency score) and shift to online learning in the models. The significance of the interaction terms will be evaluated using the likelihood ratio test. All the regression analyses will be done in R ( http://www.r-project.org ). P values ≤ 0.05 will be considered statistically significant.
Qualitative data.
After transcribing the interviews, the data transcripts will be analyzed using NVivo 1.4.1 software [ 50 ] by three research team members independently using the inductive logic approach in thematic analysis: familiarizing with the data, generating initial codes, searching for themes, reviewing the themes, defining and naming the themes, and producing the report [ 51 ]. Data familiarization will consist of reading and re-reading the data while noting initial ideas. Additionally, coding interesting features of the data will follow systematically across the entire dataset while collating data relevant to each code. Moreover, the open coding of the data will be performed to describe the data into concepts and themes, which will be further categorized to identify distinct concepts and themes [ 52 ].
The three researchers will discuss the results of their thematic analyses. They will compare and contrast the three analyses in order to come up with a thematic map. The final thematic map of the analysis will be generated after checking if the identified themes work in relation to the extracts and the entire dataset. In addition, the selection of clear, persuasive extract examples that will connect the analysis to the research question and literature will be reviewed before producing a scholarly report of the analysis. Additionally, the themes and sub-themes generated will be assessed and discussed in relevance to the study’s objectives. Furthermore, the gathering and analyzing of the data will continue until saturation is reached. Finally, pseudonyms will be used to present quotes from qualitative data.
Data triangulation.
Data triangulation using the two different data sources will be conducted to examine the various aspects of the research and will be compared for convergence. This part of the analysis will require listing all the relevant topics or findings from each component of the study and considering where each method’s results converge, offer complementary information on the same issue, or appear to contradict each other. It is crucial to explicitly look for disagreements between findings from different data collection methods because exploration of any apparent inter-method discrepancy may lead to a better understanding of the research question [ 53 , 54 ].
Data management plan.
The Project Leader will be responsible for overall quality assurance, with research associates and assistants undertaking specific activities to ensure quality control. Quality will be assured through routine monitoring by the Project Leader and periodic cross-checks against the protocols by the research assistants. Transcribed KIIs and the online survey questionnaire will be used for recording data for each participant in the study. The project leader will be responsible for ensuring the accuracy, completeness, legibility, and timeliness of the data captured in all the forms. Data captured from the online survey or KIIs should be consistent, clarified, and corrected. Each participant will have complete source documentation of records. Study staff will prepare appropriate source documents and make them available to the Project Leader upon request for review. In addition, study staff will extract all data collected in the KII notes or survey forms. These data will be secured and kept in a place accessible to the Project Leader. Data entry and cleaning will be conducted, and final data cleaning, data freezing, and data analysis will be performed. Key informant interviews will always involve two researchers. Where appropriate, quality control for the qualitative data collection will be assured through refresher KII training during research design workshops. The Project Leader will check through each transcript for consistency with agreed standards. Where translations are undertaken, the quality will be assured by one other researcher fluent in that language checking against the original recording or notes.
Ethics approval.
The study shall abide by the Principles of the Declaration of Helsinki (2013). It will be conducted along with the Guidelines of the International Conference on Harmonization-Good Clinical Practice (ICH-GCP), E6 (R2), and other ICH-GCP 6 (as amended); National Ethical Guidelines for Health and Health-Related Research (NEGHHRR) of 2017. This protocol has been approved by the University of the Philippines Manila Research Ethics Board (UPMREB 2021-099-01 dated March 25, 2021).
The main concerns for ethics were consent, data privacy, and subject confidentiality. The risks, benefits, and conflicts of interest are discussed in this section from an ethical standpoint.
Recruitment.
The participants will be recruited to answer the online SAQ voluntarily. The recruitment of participants for the KIIs will be chosen through stratified random sampling using a list of those who answered the online SAQ; this will minimize the risk of sampling bias. In addition, none of the participants in the study will have prior contact or association with the researchers. Moreover, power dynamics will not be contacted to recruit respondents. The research objectives, methods, risks, benefits, voluntary participation, withdrawal, and respondents’ rights will be discussed with the respondents in the consent form before KII.
Informed consent will be signified by the potential respondent ticking a box in the online informed consent form and the voluntary participation of the potential respondent to the study after a thorough discussion of the research details. The participant’s consent is voluntary and may be recanted by the participant any time s/he chooses.
Data privacy.
All digital data will be stored in a cloud drive accessible only to the researchers. Subject confidentiality will be upheld through the assignment of control numbers and not requiring participants to divulge the name, address, and other identifying factors not necessary for analysis.
Compensation.
No monetary compensation will be given to the participants, but several tokens will be raffled to all the participants who answered the online survey and did the KIIs.
This research will pose risks to data privacy, as discussed and addressed above. In addition, there will be a risk of social exclusion should data leaks arise due to the stigma against mental health. This risk will be mitigated by properly executing the data collection and analysis plan, excluding personal details and tight data privacy measures. Moreover, there is a risk of psychological distress among the participants due to the sensitive information. This risk will be addressed by subjecting the SAQ and the KII guidelines to the project team’s psychiatrist’s approval, ensuring proper communication with the participants. The KII will also be facilitated by registered clinical psychologists/psychiatrists/social scientists to ensure the participants’ appropriate handling; there will be a briefing and debriefing of the participants before and after the KII proper.
Participation in this study will entail health education and a voluntary referral to a study-affiliated psychiatrist, discussed in previous sections. Moreover, this would contribute to modifications in targeted mental-health campaigns for the 18–25 age group. Summarized findings and recommendations will be channeled to stakeholders for their perusal.
Dissemination.
The results will be actively disseminated through conference presentations, peer-reviewed journals, social media, print and broadcast media, and various stakeholder activities.
This study protocol rationalizes the examination of the mental health of the college students in the Philippines during the COVID-19 pandemic as the traditional face-to-face classes transitioned to online and modular classes. The pandemic that started in March 2020 is now stretching for more than a year in which prolonged lockdown brings people to experience social isolation and disruption of everyday lifestyle. There is an urgent need to study the psychosocial aspects, particularly those populations that are vulnerable to mental health instability. In the Philippines, where community quarantine is still being imposed across the country, college students face several challenges amidst this pandemic. The pandemic continues to escalate, which may lead to fear and a spectrum of psychological consequences. Universities and colleges play an essential role in supporting college students in their academic, safety, and social needs. The courses of activities implemented by the different universities and colleges may significantly affect their mental well-being status. Our study is particularly interested in the effect of online classes on college students nationwide during the pandemic. The study will estimate this effect on their mental wellbeing since this abrupt transition can lead to depression, stress, or anxiety for some students due to insufficient time to adjust to the new learning environment. The role of social media is also an important exposure to some college students [ 55 , 56 ]. Social media exposure to COVID-19 may be considered a contributing factor to college students’ mental well-being, particularly their stress, depression, and anxiety [ 57 , 58 ]. Despite these known facts, little is known about the effect of transitioning to online learning and social media exposure on the mental health of college students during the COVID-19 pandemic in the Philippines. To our knowledge, this is the first study in the Philippines that will use a mixed-method study design to examine the mental health of college students in the entire country. The online survey is a powerful platform to employ our methods.
Additionally, our study will also utilize a qualitative assessment of the college students, which may give significant insights or findings of the experiences of the college students during these trying times that cannot be captured on our online survey. The thematic findings or narratives from the qualitative part of our study will be triangulated with the quantitative analysis for a more robust synthesis. The results will be used to draw conclusions about the mental health status among college students during the pandemic in the country, which will eventually be used to implement key interventions if deemed necessary. A cross-sectional study design for the online survey is one of our study’s limitations in which contrasts will be mainly between participants at a given point of time. In addition, bias arising from residual or unmeasured confounding factors cannot be ruled out.
The COVID-19 pandemic and its accompanying effects will persistently affect the mental wellbeing of college students. Mental health services must be delivered to combat mental instability. In addition, universities and colleges should create an environment that will foster mental health awareness among Filipino college students. The results of our study will tailor the possible coping strategies to meet the specific needs of college students nationwide, thereby promoting psychological resilience.
Introduction
Filipino students experiences in online learning: a meta-synthesis.
Authors: Faith Loraine Aying, Angelito Cabanilla Jr. , Joyce Canillo, Allanah Capangpangan, Angel Sheen Conocono
DOI Link: https://doi.org/10.22214/ijraset.2023.49511
Certificate: View Certificate
This study presented a meta-synthesis of Filipino students’ experiences in Online Learning. It utilized 15 out of 1090 studies from 2021 to 2022 that were screened through inclusion and exclusion criteria. The studies were organized using the Preferred Reporting Items for Systematic Reviews and Meta-Analysis (PRISMA) 2020 flow diagram and were quality-checked using the Critical Appraisal Skills Programme (CASP) checklist for systematic review. Clark and Braunes’ six-step thematic analysis was done and has emerged seven themes: mental and emotional barrier, social interaction barrier, lack of availability of gadgets and intermittent internet connections, lack of financial support, attention span and academic performances, and parental involvement in an online class. Students’ experiences in online learning emerged as the meta-theme of the study. Filipino students\' experiences in online learning opened up new avenues for new ways of learning but a lack of resources and training makes it difficult for them to learn. It is recommended that students should join a free online symposium that tackles various coping mechanisms amidst distance learning. Academic Institutions must provide adaptive learning systems and the Philippine Government must address the issues and concerns in distance learning.
I. INTRODUCTION
Education is widely recognized as a significant social necessity that serves as a foundation for a prosperous future and a key to individual freedom. Bhardwaj (2016) This is an essential factor in a person's life because having a successful future opens up many opportunities. For as long as we have been here, philosophers, the state, and those in positions of authority have emphasized its significance. All the progress present today, would not have been possible without education. Thus, making humans lifelong learners. However, the world took a step back when an infectious virus called the Coronavirus disease (COVID-19), which made its first appearance in Southeast Asia in February 2020, spread across the globe. Jobs and schools were immediately closed for two years. The economy and population both declined dramatically. The primary cause is a sharp decline in government investment. Many private-sector businesses have been forced to close or downsize their operations, resulting in job losses. Despite the rapid decline, people looked for ways to continue to prosper and fight the pandemic. As a response to the needs of the learners, institutions have implemented policies to continue learning.
Singapore has faced online learning positively. Although the country has almost 90% of its citizens have internet access, the Education Minister continued to emphasize the difficulties associated with the unexpected shift from face-to-face to virtual classes, noting that some households do not have internet connectivity. Luckily, their government has been providing them with the resources they need, such as devices and internet access to continue learning.
To ensure learning continuity, the Department of Education (DepEd) in the Philippines has used alternative learning modalities that involve technology and internet service. People had many opinions about the solution because it was clear that they lacked the resources to implement it. For example, through an online campaign based on faculty and student sentiments, student politicians from multiple colleges urged CHEd to authorize the withdrawal of online classes, stating that “while we understand the need for learning to continue, the different circumstances of students across universities are not ideal and conducive for such.” The pleaders contend that “access to the internet connection and learning devices continues to be a privilege up to this day, placing those with poor internet access at a disadvantage when it comes to online classes.” To give you a better picture, 45% of Filipino citizens (46 million) and 74% (34,500) of students in public schools do not have internet access (Jones, 2019). However, DepEd and CHED still pushed through with the solution and are used to this day. Though online learning is a great solution to the problems caused by COVID-19, there is not much to say about student learning, especially since it reduces experiential learning. This drastic change may or may not be beneficial to the student's learning needs. Many students do not have consistent internet access; others do not even have adequate electronic devices, let alone the necessary technical skills. As a result, the goal of this meta-synthesis is to establish the effectiveness and experiences of online learning among Filipino students.
A. Research Objective
This systematic review aims to know the experiences of Filipino students in online learning using a meta-synthesis method. Additionally, we would like to identify all the possible challenges that Filipino students encounter while having online learning.
II. METHODOLOGY
A. Research Design
In the study, meta-synthesis was applied in order to synthesize data on students' online learning experiences in the Philippines. In connection with this paper, online learning, experiences, and challenges of the Filipino students will be reviewed by utilizing qualitative data. By doing it, relevant data will be drawn upon to help build a stronger foundation relating to online learning while addressing the concerns of the students.
B. Search Strategy
Key terms found in the Publish or Perish Software database via Google Scholar are online learning, students, and the Philippines and there were nine hundred eighty-two related studies found. Concurrently, in the Scopus database the keywords used are still online learning, students, and the Philippines, and found one hundred eight related studies. From 2021 to 2022, all studies pertinent to online learning for Filipino students were retrieved and examined. The Critical Appraisal Skills Programme (CASP) Systematic Review checklist was used to screen remaining studies. These words were specifically chosen to collect information from Google Scholar and Scopus in order to compile variables required for the meta-synthesis. The retrieved data was organized using a PRISMA 2020 flow diagram.
C. Selection/Inclusion Criteria
All reported studies met the set of criteria: articles must involve online learning in the Philippines, be focused on students' online learning experiences, be 2021-2022 studies, and meet the Critical Appraisal Skills Programme (CASP) checklist.
D. Data Analysis
Thematic data analysis was used in identifying essential or recurrent themes. The findings were organized thematically. Thematic analysis used Clarke and Braune (2017) protocol to analyze the selected data. Thematic analysis, according to Caulfied, is a method for detecting, evaluating, and incorporating various themes throughout qualitative data.Six phases are involved in thematic analysis: familiarization, developing initial ideas, searching for articles, evaluating topics, specifying and labeling portions, finally creating a result.
III. RESULTS AND DISCUSSION The data is perceived in accordance with the study's purpose based on a meta-synthesis of the selected research. The meta-theme was defined as students' online learning experiences. As a result of the meta-theme, seven themes emerged, namely, mental and emotional barrier, social interaction barrier, lack of availability of gadgets and intermittent internet connection, communication barrier, lack of financial support, attention span and academic performance, parental involvement in online class
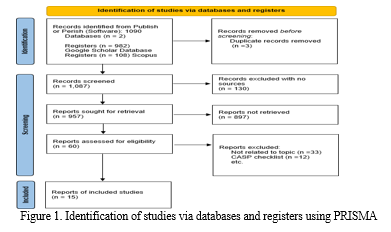
In research paper selection, there were three (3) stages using the PRISMA Flow Diagram. Identification, Screening, and Included are the three stages. During the identification stage, 982 research were published in Google Scholar and 108 studies were published in the Scopus database, totaling One Thousand Ninety (1090) studies on the initial screening utilizing the Publish or Perish software. Due to duplication of studies in Google Scholar and Scopus, three (3) research were deleted, leaving 1,087 studies in the identification stage. The screening stage had three sub-stages. One hundred thirty (130) studies were eliminated from the first phase due to a lack of sources, resulting in one thousand eighty-seven (1, 087) papers. In the second stage, eight hundred thirty-four (834) studies have been eliminated because the citation did not fulfill the researcher's specifications, thirty (30) studies were eliminated because there was no URL, eighteen (18) studies were eliminated because they could not be accessed, and fifteen (15) studies were eliminated because the authors were unknown, resulting in sixty (60) studies.Thirty-three (33) studies were omitted from the third substage because they were unrelated to the topic, and twelve (12) studies were also removed since they did not meet the requirements using the CASP checklists, which resulted in fifteen (15) studies on the screening stage's final substage.
In the meta-synthesis, 15 studies were considered which are focusing on students' online learning experiences were used to determine Filipino students' online learning experiences. In table 1, it shows the studies focusing on the experiences of students in online learning included in the qualitative meta-synthesis. It also shows the authors, year, setting of the study, the publication type, the design of the study and the students’ experiences in online learning based on each study.
Table 1. Studies focusing on students’ experiences in online learning as part of the qualitative meta-synthesis
No. | Authors | Year | Setting | Publication Type | Design | Students’ Experiences in Online Learning |
S1 | JS Barrot, II Llenares, LS Del Rosario | 2021 | Philippines | Article | Mixed | |
S2 | AJ Dianito, J Espinosa, J Duran | 2021 | Philippines | Published | Quali | |
S3 | R Alibudbu d | 2021 | Philippines | Article | Quali | |
S4 | RM Oducado, H Estoque | 2021 | Philippines | Article | Mixed | |
S5 | JN Guillaspe r, RMF Oducado, GP Soriano | 2021 | Philippines | Article | Quanti |
|
S6 | JV Cleofas | 2021 | Philippines | Article | Mixed | |
S7 | DJ Lemay, P Bazelais, T Doleck | 2021 | Philippines | Article | Quanti | |
S8 | JMR Asio, E Gadia, E | 2021 | Philippines | Article | Quanti | |
| Abarintos , D Paguio… |
|
|
|
| |
S9 | J Santos, LF De Jesus, RR Sealmoy … | 2021 | Philippines | Article | Mixed | |
S10 | GD Anzaldo | 2021 | Philippines | Article | Quali | |
S11 | E Rotas, M Cahapay | 2021 | Philippines | Article | Quali | |
S12 | AD Talosa, BS Javier, EL Dirain | 2021 | Philippines | Article | Quali | |
S13 | M Aristeido u, S Cross | 2021 | Philippines | Article | Quanti | |
S14 | RE Baticulon , JJ Sy, NRI Alberto, MBC Baron | 2021 | Philippines | Article | Mixed | communication between teachers and students |
S15 | A Funa, F Talaue | 2021 | Philippines | Article | Quanti |
There are 15 studies counted in the final set; these include (14) published articles and (1) book. The data was gathered and analyzed using qualitative (5), quantitative (5), and mixed methods (5). One meta-theme and seven themes emerged from the fifteen (15) publications.
A. Theme 1: Mental and Emotional Barrier
According to Fawaz and Samaha (2020), college students are more likely to suffer from depression and stress as a result of psychological challenges, learning conditions, future uncertainties, afraid of infectious disease, reports about an insufficiency of safety equipment, tiredness caused by lockdown, disappointments, as well as an absence of freedom, and fears caused by rumors and false information in the media. College students who took part in studies done during the COVID-19 lockout reported a negative impact on their mental wellbeing and feelings.
In general, the COVID-19 outbreak has increased students' stress, anxiety, and depression (Aslan et al., 2020). Emotionally negative reactions such as rage, confusion, anguish, agony, disgust, fear, or unease are among those associated with it. It is also associated with significant emotional trauma, delayed stress disorder, and signs of depression among students. (Brooks et al., 2020) Notwithstanding the earlier studies concentrating on negative emotions According to (Aslan et al., 2020), future research on students' psychological health must include positive emotions to determine the extent to which these reduce as a result of the pandemic situation. Positive emotions have been shown to aid in the preservation and improvement of human mental health; thus, measuring them throughout a public health emergency is crucial (Yamaguchi et al., 2020). Encouraging social interaction and connection will benefit students' mental health both during and after the epidemic, according to (Holen et al., 2018).
B. Theme 2: Social Interaction Barrier
Academic social interactions frequently enhance students' sense of belonging., and if they don't feel like they belong, they may be less motivated to complete their academic work (Yeager et al. 2013). According to recent research, students are generally isolated during the pandemic and lack of in-person social interactions can significantly increase students' stress levels (Dumitrache et al. 2021; Son et al). It is commonly assumed that an apparent lack of academic social relationships in an online learning environment exacerbates students' learning and self-regulation challenges. This is because the absence of continuous engagement with students and superiors in an online learning can be a source of stress for students and affect their academic performance (Chaturvedi et al. 2021; Yeager et al. 2013). Students who have fewer educational conversations portray their lives being more impacted as a result of the epidemic, which is consistent with other recent studies indicating the problems of social detachment on students' lifestyles and well-being (e.g., C. Liu et al. 2021; Lukács 2021). Similarly, Rodriguez-Besteiro et al. (2021) state that due to academic delays and financial difficulties, college students are among the most communities affected by the global epidemic. Furthermore, during the pandemic, significantly reduced social connections may be associated with a generally poorer adjustment to this unpleasant condition, particularly among younger students, who have greater difficulties coping with pandemic-induced stress, according to Babicka-Wirkus et al. (2021).
C. Theme 3: The Lack of Availability of gadgets and Intermittent Internet Connection
According to Fabito et al. (2020), reliable internet connection was one of the obstacles faced by students in online learning. Similarly, Casillano (2019) stated that there is only a few portion of students who have full access to the internet making it hard for some to access the platform for electronic learning. Furthermore, according to (Cleofas & Rocha, 2021), students who have limited internet access and do not own laptops or personal computers are at a much greater disadvantage. However, according to Jin and Sabio (2018), using mobile devices for online learning is likely to be an advantage as this can facilitate learning. Furthermore, according to (Estira, 2020), the more device types a student owns, the higher the level of learning preparedness.A survey discovered that students are equipped for online classes: However, computer and rental internet cafes still exist but are costly. (Yra et al., 2020). Furthermore, a survey revealed that students were ready for online lessons; yet, the cost of computer and internet rentals in cafes exists. Another survey found that smartphones are popular among students and have become one of the apex tools for educational institutions to use (Al Tameemy, 2017). However, in the local environment, some kids still do not have access to these devices. This becomes a burden or a struggle for individuals, especially when it comes to learning (Cleofas & Rocha, 2021; Yra et al., 2020).
D. Theme 4: Communication Barrier
The utilization of technology-based data and communication to transmit content in a variety of ways facilitates online learning. Whether this is with visual and auditory components in order to make learning attentive and appealing (Rusu et al 2021), communication only occurs via phone, video calls, or teleconference (Zoom meeting) during online learning, according to Anggraini et al., (2022). However, the abrupt change in communication style has forced pupils to face issues in lowering their communication and social connection with their professors and friends. (Aboagye et al., 2020) Furthermore, online learning assists students in creating virtual communities in which they participate in collaborative learning through communication. (Virca et al., 2021). According to one study, assertive students who are enrolled in online learning or courses can have a detrimental effect on some other pupils' skills and opportunity to engage and interact since assertive pupils may be uninformed of their very own interpersonal communication, tends to result in pretty accurate in classroom discussion by other students.(McClsaac and Tug, 2002)
As a result, feedback improves educational communication by increasing student and teacher satisfaction. Electronic learning, on the other hand, should offer a wide range of technological services that enable students to actively express themselves as well as communicate in a collaborative context (Popa et al., 2021).
E. Theme 5: Lack of Financial Support
COVID-19 increased several students' financial challenges, negatively impacting their online learning experience. The principal reasons for these financial consequences is that students can afford to pay for their online course and also as a consequence since their parents do not have jobs. Also, it is really difficult for students to pay for the high cost of having internet connectivity. In an attempt to counteract the development of COVID-19, academic facilities shifted from face-to-face interactions to mostly online learning modalities, exacerbating these consequences (Malolos et al., 2021).Whereas all learners could be effected, children from lower socioeconomic situations are more likely to experience emotional discomfort due to a lack of monetary capacity to acquire necessary technology and internet connection.Given these factors, a digital divide caused by socioeconomic inequities can lead to mental health disparities among students during the epidemic (Cleofas and Rocha, 2021). Adequate recognition is essential to guarantee adequate assistance and security for all individuals with disabilities (Smith-Khan et al., 2015). During the epidemic, the majority of participants encountered social and economical challenges. Some of them stated that they had difficulty obtaining support from their peer groups (Barrot et al., 2021). In addition, students have financial difficulties as a result of flexible learning because the internet is expensive in the Philippines and gadgets are pricey. As a result, it is suggested that they improve technological access for educational delivery. (Aban et al., 2022)
F. Theme 6: Attention Span and Academic Performance
Multitasking is one of the benefits of technology improvements; as a result, students can easily access information at one’s convenience (B. B. K. King, 2020; May, 2020). According to (Paul & Jefferson, 2019), flexibility and time efficiency have become viable as a result of these demands and opportunities.
According to (Cicekci & Sadik, 2019), despite the possibilities afforded by technology, students still find it difficult to keep their concentration on the content presented to them. According to the May (2020) study, a shorter attention span and a lower level of focus have a stronger impact on online learning.
However, according to (B. B. K. King, 2020), various factors impact student outcomes in online classrooms, and having access to the internet anywhere at the moment jeopardizes a student's focus and concentration. The overflow of information exhausts the student and forces them to redirect their attention elsewhere (May, 2020). Learners are required to be able to multitask since classes are videotaped and distributed, planned activities are offered, and texts are delivered by the university or given instructor (B. B. K. King, 2020).
According to (Deng & Wu, 2018), Frequent feedback from students improves the efficacy of online learning. Students are called out at random during online sessions to be evaluated by the teacher to ascertain whether their attention is focused towards the learning experience or if they have been disturbed by environmental factors, or if there is live video feed, the teacher notices the pupils' facial expressions and body language. direction.
Furthermore, (Cicekci & Sadik, 2019) said that a student's attention span is an important component in distance learning; if the student is constantly chastised by the instructor for not listening nor listening attentively, the discussion will be disrupted and the time allotted would be wasted.
G. Theme 7: Parent Involvement in Online Class
According to (Song et al., 2020), during the COVID-19 epidemic, most countries restricted school operations and shifted the instructional procedure to a virtual system for learning at their respective family residences.
Parent's involvement in a child's learning is one of the consequences in children's educational process due to a sudden shift of old fashion instruction to a virtual instruction without having to modify the curriculum or the educational philosophy. (Knopik & Oszwa, 2019). In certain situations, parents were required to commit additional time to their children's education(Carpenter & Dunn, 2021)particularly to kids with exceptional needs ( Brandenburg et al., 2020), Parent participation in children's education, according to (Szumski & Karwowski, 2017), may be characterized broadly as purposeful attempts to help them in the education process while taking into consideration their intellectual, affective, interpersonal, and character growth.
H. Meta-theme: Students’ Experiences in Online Learning
Online learning is an educational environment utilized on the Web as well as other digital equipment and resources to facilitate online and offline teaching processes as well as educational program administration (Usher & Barak, 2020; Huang, 2019).
According to Copeland et al. (2021), the worldwide epidemic harmed students' emotional and behavioral functioning, particularly focus and projecting difficulties (i.e., emotion and wellbeing conduct) caused by solitude, financial implications, and difficulties.
Furthermore, according to Yazon and Callo, all learners require equity in terms of the availability of technology that supports student learning; however, the digital gap is widening due to students' limited opportunities in economic hardship, even those in rural areas.
Students\' experiences with online learning in the Philippines opened up new avenues for new ways of learning. However, a lack of resources and training makes it difficult for students to learn. As a result, it is recommended that students should join a free online symposium that tackles various coping mechanisms amidst distance learning. Academic Institutions must provide adaptable learning systems and Government institutions must address the issues and concerns in distance learning.
[1] Aban, E. P., & Nicart, E. B. B. (2022). Online Learning readiness of secondary school teachers towards ... - AJHSSR. Online Learning Readiness of Secondary School Teachers towards Flexible and Blended Learning: Basis for Educational Strategy. Retrieved 18AD, from https://www.ajhssr.com/wp-content/uploads/2022/04/C226042432.pdf [2] Anggraini, S., & Dewi , S. K. (2022). Effect of brain gym in increasing the learning concentration of ... - UPM. Effect of Brain Gym in Increasing the Learning Concentration of 6th Grade on Online Learning during the Covid-19 Pandemic. Retrieved October 17, 2022, from 2022022317245617_0939.pdf (upm.edu.my) [3] Asio, J., Gadia, E., Abarintos, E., et al. Internet Connection and Learning Device Availability of College Students: Basis for Institutionalizing Flexible Learning in the New Normal. Studies in Humanities and Education. 2021 Volume 2, Issue 1: 56 – 69 https://files.eric.ed.gov/fulltext/ED613639.pdf [4] Balan, A. K., Montemayor, T. A., (2020). The Influence of Online Learning towards the Attention Span and Motivation of College Students. School of Social Sciences and Education. Retrieved November 2020, https://www.researchgate.net/profile/Thomas-Montemayor/publication/348916010_The_Influenc e_of_Online_Learning_towards_the_Attention_Span_and_Motivation_of_College_Students/link s/6178c8ae0be8ec17a9354f68/The-Influence-of-Online-Learning-towards-the-Attention-Span-an d-Motivation-of-College-Students.pdf [5] Baltà-Salvador, R., Olmedo-Torre, N., Peña, M. et al. Academic and emotional effects of online learning during the COVID-19 pandemic on engineering students. Educ Inf Technol 26, 7407–7434 (2021). https://doi.org/10.1007/s10639-021-10593-1 [6] Barrot, J.S., Llenares, I.I. & del Rosario, L.S. Students’ online learning challenges during the pandemic and how they cope with them: The case of the Philippines. Educ Inf Technol 26, 7321–7338 (2021). https://doi.org/10.1007/s10639-021-10589-x [7] Bernasor, C. C. T., Banto, A. J. A., Lacaran, L. D. M., Lusdoc, J. E., Conway, G. D., Regalado, L. J. D., & Ouano, J. A. (2022). Teacher-Student Relationship and Students’ Well-being in Online Learning during the COVID-19 Pandemic: Does Basic Psychological Needs Satisfaction Matter? Microsoft Word-pp_21-36_Bernasor et al.docx (researchgate.net) [8] Chih-Hsiung Tu & Marina McIsaac (2002) The Relationship of Social Presence and Interaction in Online Classes, American Journal of Distance Education The Relationship ofSocial Presence and Interaction in Online Classes (tandfonline.com) [9] MelissaE. Mendoza, Timothy F. Brewer, Matthew S. Smith, Michael Ashley Stein, S. Jody Heymann, Lessons from United States school district policies and approaches to special education during the COVID-19 pandemic, International Journal of Inclusive Education, 10.1080/13603116.2022.2056643, (1-20), (2022). https://onlinelibrary.wiley.com/doi/full/10.1111/ejed.12474 [10] Pavin Ivanec T. The Lack of Academic Social Interactions and Students’ Learning Difficulties during COVID-19 Faculty Lockdowns in Croatia: The Mediating Role of the Perceived Sense of Life Disruption Caused by the Pandemic and the Adjustment to Online Studying. Social Sciences. 2022; 11(2):42. https://doi.org/10.3390/socsci11020042 [11] Pelucio, L., Simões, P., Dourado, M.C.N. et al. Depression and anxiety among online learning students during the COVID-19 pandemic: a cross-sectional survey in Rio de Janeiro, Brazil. BMC Psychol 10, 192 (2022). https://doi.org/10.1186/s40359-022-00897-3 [12] Rusu,M.,Virca,I. & Popa,I.(2021).Efficiency of Communication in Perceiving Online Learning. International conference KNOWLEDGE-BASED ORGANIZATION,27(2) 187-192. https://doi.org/10.2478/kbo-2021-0071 STUDIES REFERENCES [1] Acquah, E. O., & Katz, H. T. (2020). Digital game-based L2 learning outcomes for primary through high-school students: A systematic literature review. Computers & Education, 143, 103667. [2] Agormedah, E. K., Henaku, E. A., Ayite, D. M. K., & Ansah, E. A. (2020). Online learning in higher education during COVID-19 pandemic: A case of Ghana. Journal of Educational Technology and Online Learning, 3(3), 183-210. [3] Alam, N. S. K. M. (2021). Struggling with Digital Pandemic: Students’ Narratives about Adapting to Online Learning at Home during the COVID-19 Outbreak. [4] Anwar, I. S. K., Omar, N., Isa, N. S. M., & Shamsudin, S. M. (2020). STUDENTS ACCEPTANCE AND READINESS TOWARDS DISTANCE LEARNING DURING COVID-19 PANDEMIC. International Journal of Education and Pedagogy, 2(4), 39-50. [5] Barrot, J. S., Llenares, I. I., & Del Rosario, L. S. (2021). Students’ online learning challenges during the pandemic and how they cope with them: The case of the Philippines. Education and Information Technologies, 26(6), 7321-7338. [6] Baticulon, R. E., Sy, J. J., Alberto, N. R. I., Baron, M. B. C., Mabulay, R. E. C., Rizada, L. G. T., ... & Reyes, J. C. B. (2021). Barriers to online learning in the time of COVID-19: A national survey of medical students in the Philippines. Medical science educator, 31(2), 615-626. [7] Choi, J. J., Robb, C. A., Mifli, M., & Zainuddin, Z. (2021). University students’ perception of online class delivery methods during the COVID-19 pandemic: A focus on hospitality education in Korea and Malaysia. Journal of Hospitality, Leisure, Sport & Tourism Education, 29, 100336. [8] Duc-Long, L., Thien-Vu, G., & Dieu-Khuon, H. (2021). The Impact of the COVID-19 Pandemic on Online Learning in Higher Education: A Vietnamese Case. European Journal of Educational Research, 10(4), 1683-1695. [9] Gocotano, T. E., Jerodiaz, M. A. L., Banggay, J. C. P., Nasibog, H. B. R., & Go, M. B. (2021). Higher Education Students’ Challenges on Flexible Online Learning Implementation in the Rural Areas: A Philippine Case. International Journal of Learning, Teaching and Educational Research, 20(7). [10] Huang, L., & Zhang, T. (2022). Perceived social support, psychological capital, and subjective well-being among college students in the context of online learning during the COVID-19 pandemic. The Asia-Pacific Education Researcher, 31(5), 563-574. [11] Jiang, N., Yan-Li, S., Pamanee, K., & Sriyanto, J. (2021). Depression, anxiety, and stress during the COVID-19 pandemic: Comparison among higher education students in four countries in the Asia-Pacific Region. Journal of Population and Social Studies [JPSS], 29, 370-383. [12] Karnalim, O., & Wijanto, M. C. (2021, July). Transitioning to online learning for Indonesian high school students: Challenges and possible solutions. In 2021 International Conference on Advanced Learning Technologies (ICALT) (pp.428-430). IEEE. [13] Pasaribu, T. A., & Dewi, N. (2021). Indonesian EFL Students\' Voices on Online Learning during COVID-19 through Appraisal Analysis. LEARN Journal: Language Education and Acquisition Research Network, 14(1), 399-426. [14] Taghizadeh, S. K., Rahman, S. A., Nikbin, D., Alam, M. M. D., Alexa, L., Ling Suan, C., & Taghizadeh, S. (2022). Factors influencing students’ continuance usage intention with online learning during the pandemic: a cross-country analysis. Behaviour & Information Technology, 41(9), 1998-2017. [15] Thomas, D. (2020). Thailand University students e-learning behavior during the global pandemic. Hum. Behav. Dev. Soc, 21(4), 57-65.
Copyright © 2023 Faith Loraine Aying, Angelito Cabanilla Jr. , Joyce Canillo, Allanah Capangpangan, Angel Sheen Conocono. This is an open access article distributed under the Creative Commons Attribution License , which permits unrestricted use, distribution, and reproduction in any medium, provided the original work is properly cited.
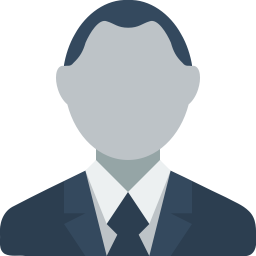
Download Paper
Authors : Faith Loraine Aying
Paper Id : IJRASET49511
Publish Date : 2023-03-12
ISSN : 2321-9653
Publisher Name : IJRASET
DOI Link : Click Here

- Open access
- Published: 14 July 2022
The impact of extreme weather on student online learning participation
- Ezekiel Adriel D. Lagmay 1 &
- Maria Mercedes T. Rodrigo ORCID: orcid.org/0000-0001-7881-7756 1
Research and Practice in Technology Enhanced Learning volume 17 , Article number: 26 ( 2022 ) Cite this article
22k Accesses
5 Citations
13 Altmetric
Metrics details
In March 2020, the COVID-19 pandemic forced over 1 billion learners to shift from face-to-face instruction to online learning. Seven months after it began, this transition became even more challenging for Filipino online learners. Eight typhoons struck the Philippines from October to November 2020. Two of these typhoons caused widespread flooding, utilities interruptions, property destruction, and loss of life. We examine how these severe weather conditions affected online learning participation of Filipino students pursuing their undergraduate and graduate studies. We used CausalImpact analysis to explore September 2020 to January 2021 data collected from the Moodle Learning Management System data of one university in the Philippines. We found that overall student online participation was significantly negatively affected by typhoons. However, the effect on participation in Assignments and Quizzes was not significant. These findings suggested that students continued to participate in activities that have a direct bearing on their final grades, rather than activities that had no impact on their course outcomes.
Context of the study
The shift to online learning because of COVID-19 offered us a unique opportunity to quantify the impact of extreme weather on the online learning participation of Filipino students. In prior years, the majority of education in the Philippines, as in most countries, took place in person. While some institutions made use of Learning Management Systems (LMSs), most instruction was face to face. LMSs were repositories for materials, submission sites, or test platforms, but were typically not used to replace class time. The onset of the pandemic forced 1 billion students (UNESCO, 2021 ), including Filipinos, to shift to an online mode. The struggle to teach and learn online worsened when eight typhoons entered the Philippine Area of Responsibility (PAR) from October 11 to November 12, 2020 (Lalu, 2020 ). Two of them, Typhoons Goni and Vamco, were particularly destructive, causing widespread destruction, utilities disruptions, and loss of life. The migration of all instruction to digital platforms thus enabled us to capture a greater variety of instructional activities, data that were previously unavailable, and to use this data to study the effects of these typhoons on student learning behaviors.
Effects of extreme weather on academic achievement
The immediate effects of extreme weather events such as severe typhoons and heat waves include property destruction, crop failure, and human casualties. On November 8, 2013, for example, Typhoon Haiyan made landfall in the Philippines. A Category 5 storm, it was one of the most powerful typhoons of all time. It displaced 4.1 million people, killed 6,000, damaged 1.1 million homes, and destroyed 33 million coconut trees, a major cash crop (World Vision, 2021 ). In total, Typhoon Haiyan caused damages estimated at US$5.8 billion. Typhoon Goni made landfall in the Philippines on October 27, 2020, seven months into the COVID-19 pandemic. Like Haiyan, Goni was a Category 5 storm, the strongest of 2020, with maximum sustained winds of 255 km per hour. It left 25 dead and damaged over 280,000 houses. Damage to crops, livestock, fisheries, and agriculture was estimated at P5 billion, while damage to infrastructure such as roads and bridges was estimated at P12.8 billion (International Federation of Red Cross & Red Crescent Societies, 2020a ). Typhoon Vamco made landfall in the Philippines on November 11, 2020. Vamco was weaker than Goni, with maximum sustained winds at 155 km per hour (International Federation of Red Cross & Red Crescent Societies, 2020b ). However, Vamco brought historically high levels of flooding in parts of the country—the worst in 45 years. The storm killed 101 people and left over P20 billion in damages to livelihoods and infrastructure.
The longer-term consequences of these events are far-reaching and complex. In the developing world specifically, limited savings among less wealthy households and the lack of social supports such as access to credit and insurance make it difficult for poorer families to recover from shocks caused by extreme weather (Groppo & Kraehnert, 2017 ; Marchetta et al., 2018 ). Parents are forced to shift their investments from their children’s schooling, e.g., uniforms, books, transportation, tuition (Joshi, 2019 ), instead directing their resources to recovery from the economic consequences of the typhoon’s damage (Deuchert & Felfe, 2015 ). Post-typhoon enrollment decreases. Parents spend less time on their children’s learning and care (Joshi, 2019 ). Children spend less time in school and more time helping at home. Teens and young adults who are transitioning from school to work are particularly vulnerable to these shocks. They are likely to drop out of school and join the workforce in order to mitigate the impact of extreme weather. Poor young women in particular are susceptible to being pushed into the labor market (Marchetta et al., 2018 ).
These necessary choices cause an immediate gap in learning that grows over time. When Typhoon Mike hit Cebu in 1990, the children whose houses suffered typhoon damage lagged 0.13 years behind in school. The lag grew to 0.27 years in 1998, 0.52 years in 2002, and 0.67 years in 2005. By the time children are 22 years old, the gap in educational attainment is approximated at one year (Deuchert & Felfe, 2015 ).
The work of Bernabe et al. ( 2021 ) agrees. They found that storms have a disruptive impact on education. In areas severely affected by winds, children are 9% more likely to accumulate an educational delay and 6.5% less likely to complete secondary education. Individuals severely affected by storms between the ages of 23 and 33 are less likely to complete higher education, reducing their ability to obtain regular salaried jobs.
One might ask: Is it not possible for these children and young adults to return to school to make up for these gaps? Cunha and Heckman ( 2007 ) argue that different stages of childhood are more receptive to certain types of inputs than others. Secondary language learning, for example, is best before 12. They also find that public training programs for adults that try to bridge learning gaps from childhood do not produce substantial gains for most of their participants and tend to be more costly than remediation provided at earlier ages.
In summary, the physical and economic damage wrought by extreme weather events has an adverse impact on educational achievement. The education of young children who come from economically disadvantaged homes receives less financial support and parental attention, resulting in an achievement gap that increases with time. Adolescents and young adults, on the other hand, are sometimes forced to discontinue their studies and to enter the workforce to help mitigate the effects of the event. Resuming studies after an interruption is challenging because oftentimes an optimal window for learning has passed and attempts at remediation are costly and generally produce fewer gains.
Research questions
For this study, we ask two main research questions:
RQ1: To what extent was student participation affected by Typhoons Goni and Vamco?
RQ2: Was student participation able to return to pre-typhoon levels, or did the typhoons dampen participation for the rest of the post-typhoon period? If participation did return to pre-typhoon levels, how long did it take for participation to recover?
Time series analysis in education
We use CausalImpact analysis (Brodersen et al., 2015 ) to analyze the ways in which student participation in an online learning environment was affected by Typhoons Goni and Vamco. CausalImpact is a type of causal inference analysis method for time series data.
Time series analysis methods
Causal inference refers to a family of analysis methods that enable researchers to draw conclusions about the effect of a causal variable or treatment on some outcome or phenomenon of interest (Hill & Stuart, 2015 ). These methods have the same general approach: They take time series data prior to an interruption or intervention, create a model from this data, use the model to predict counterfactual post-intervention trends, and then compare the counterfactual against the actual data to check for differences. They differ in terms of their underlying modeling approach. Examples of these methods are as follows (Kuromiya et al., 2020 ; Moraffah et al., 2021 ):
CausalImpact—It is developed to evaluate the impact of a market intervention using difference-in-difference to infer the causality from observational data. Under the hood, “…it builds a Bayesian structural time series model based on multiple comparable control groups (or markets) and uses the model to project (or forecast) a series of baseline values for the time period after the event.” (Brodersen et al., 2015 ; Nishida, 2017 )
Interrupted Time Series (ITS) Model—Uses segmented regression model with dummy variables representing the period of the intervention for evaluating the effectiveness of population-level interventions. It is simple in terms of interpreting the results. (Bernal et al., 2017 )
Prophet—A type of generalized additive model consisting of trend, seasonality, and holidays. There is no need to interpolate missing values since the model handles time series analysis as a curve fitting problem and can predict future values at a very high accuracy. (Taylor & Letham, 2018 )
CausalTransfer—An improvement to CausalImpact which estimates treatment effects from experiments spanning multiple time points by using a state-space model. The main issue with CausalImpact is that it “treats every time point as a separate experiment and does not pool information over time”; hence, one is “only able to observe the outcomes under the treatment for one time series and under the control for the treatment for another one, but not the potential outcome under control for the former and under treatment for the latter.” CausalTransfer “combines regression to adjust for confounding with time series modelling to learn the effect of the treatment and how it evolves over time” and does not assume that data is stationary. (Li & Bühlmann, 2020 )
Several methods based on neural networks and deep learning have been introduced in recent years (Moraffah et al., 2021 ):
Recurrent Marginal Structural Network (R-MSN)—A sequence-to-sequence recurrent neural network (RNN)-based architecture for forecasting responses to a series of planned treatments. In contrast to other marginal structural models (MSMs) which model “the potential outcomes associated with each possible treatment trajectory with the Inverse Probability of Treatment Weighted (IPTW),” which in turn is “dependent on a correct specification of the conditional probability of treatment assignment,” R-MSN directly learns “time-dependent treatment responses from observational data, based on the marginal structural modeling framework.” (Lim et al., 2018 )
Time Series Deconfounder—This method “uses a novel recurrent neural network architecture with multitask output to build a factor model over time and infer latent variables that render the assigned treatments conditionally independent” prior to performing causal inference with the aforementioned latent variables being used in place of the multi-cause unobserved confounders. To further ensure that the factor model is able to estimate the distribution of the assigned causes, “a validation set of subjects were considered in order to compare the similarity of the two test statistics.” This overcomes the problem of having to ensure that all the confounders are observed, which may lead to biased results otherwise. (Bica et al., 2020 )
Deep Sequential Weighting—It is used for estimating individual treatment effects with time-varying confounders by using a deep recurrent weighting neural network for inferring the hidden confounders using a combination of the current treatment assignments and historical information. The learned representations of hidden confounders combined with current observed data are then utilized for obtaining potential outcome and treatment predictions. For re-weighting the population, the time-varying inverse probabilities of treatment are computed. (Liu et al., 2020 )
For their own study, Kuromiya et al. ( 2020 ) first considered ITS and Prophet as possible approaches. They found that ITS had weak predictive power and limited flexibility. Prophet was better than ITS at predicting future values. In determining the impact of an event, though, Prophet was more difficult to interpret. They therefore decided to use a method called CausalImpact instead. As this was the study that we emulated, we used CausalImpact as well. We were not able to consider using CausalTransfer nor any of the neural network/deep learning methods.
Prior Studies using CausalImpact analysis
CausalImpact is a specific type of causal inference that enables researchers to estimate the impact of an intervention such as an ad campaign on an outcome variable such as additional clicks (Brodersen, 2014 ; Brodersen, et al., 2015 ). Given time series data, we first identify predictor variables, the outcome variable, and the pre- and post-intervention time segments. CausalImpact uses the pre-intervention data to model the relationship between the predictor variables and the outcome variable. It then uses the model to estimate the post-intervention counterfactual. The impact of the intervention is the difference between the counterfactual and the observed post-intervention data. While many algorithms may be used to model the counterfactual, CausalImpact made use of Bayesian structural time series models, explained in detail in (Brodersen, et al., 2015 ). The CausalImpact R package (Brodersen, 2014 ; Brodersen, et al., 2015 ) is publicly available at http://google.github.io/CausalImpact/CausalImpact.html .
CausalImpact was created within a commercial context and was intended for use on marketing data and clickstream traffic (Brodersen, 2014 ). Since its release in 2014, the method has also been used to model the effects of product modularity on bus manufacturing (Piran et al., 2017 ), US cyber policies on cyberattacks (Kumar et al., 2016 ), Arab uprisings and tourism (Perles-Ribes et al., 2018 ), and the performance of app store releases (Martin, 2016 ).
In 2020, Kuromiya and colleagues applied CausalImpact to estimate the effects of school closures on student use of the LMS Moodle and the electronic book reader BookRoll (Kuromiya et al., 2020 ). They performed this analysis for all courses in aggregate and for one specific English course. In their analysis, they found that student traffic in Moodle and BookRoll increased significantly during the COVID-19 pandemic. For all courses in aggregate, Moodle traffic increased by 163%, while BookRoll traffic increased by 77%. With the English course, Moodle traffic increased by 2227%, while BookRoll traffic increased by 875%. Note that Kuromiya and colleagues use the term “intervention” to refer to school closures rather than a new teaching strategy. They therefore expanded the definition of “intervention” to include external events that may affect a system, rather than deliberate actions from researchers, educators, or other persons that are intended to influence how the system behaves. In this study, we use this expanded definition of “intervention” to refer to the typhoons that affected online learning.
In 2021, Lagmay and Rodrigo began the analysis of Typhoons Goni and Vamco’s effects on student participation in online classes and published initial results at an international conference (Lagmay & Rodrigo, 2021 ). While this paper drew inspiration from Kuromiya et al. ( 2020 ), it differed in its choice of predictor variables. Lagmay and Rodrigo ( 2021 ) made use of teacher and non-editing teacher activity to predict student activity. In contrast, Kuromiya et al. ( 2020 ; personal communications, 26 January 2021) used number of logs per day as both the input variable and the outcome variable.
Lagmay and Rodrigo ( 2021 ) analyzed Moodle activity from September 9, 2020, to January 9, 2021. The pre-intervention period was defined as the pre-typhoon period from September 9, 2020, to October 28, 2020. The intervention period were the days disrupted by the typhoon, October 29 to November 13. Finally, the post-intervention period was November 14 to December 23, the period after the typhoon to just before the Christmas break. The paper found a statistically significant decrease in all LMS activity but a non-statistically significant difference in activities related to assessment. The paper we present here expands the Lagmay and Rodrigo ( 2021 ) paper by experimenting with the time periods.
While much educational research makes use of causal inference in general, as of the time of this writing, the works of Kuromiya et al. ( 2020 ) and Lagmay and Rodrigo ( 2021 ) were the only applications of CausalImpact on educational data that our survey of the literature could find.
The dataset was composed of a time series of log data from the Moodle of a privately owned university in Metro Manila, Philippines. Prior to the study, the researchers conferred with the University Data Protection Office and the University Counsel to determine whether we needed to seek informed consent from faculty and students to access their Moodle data. Since the data that we received were anonymized and because we did not have the ability to re-identify the same, there was no need to seek informed consent from the Moodle users (J. Jacob, personal communication, 25 September 2020; P. Sison-Arroyo, personal communication, 25 September 2020). Furthermore, the University Research Ethics Office determined that our research protocol was considered exempt from institutional ethics review because it was research conducted in educational settings involving normal educational practices, and that the information was processed such that participants could not be identified (L. Alampay, personal communication, 11 October 2020).
We collected data from 11,736 students, 925 teachers, and 38 non-editing teachers beginning September 9, 2020, and ending on January 9, 2021. The students were undergraduate and graduate students. Undergraduate students were from 18 to 22 years old, while graduate students were 23 and older. Students generally came from middle- to upper-class families. Teachers had at least a bachelor’s degree in the subject area that they were teaching. Most had master’s degrees or higher. Both students and teachers were a mix of males and females, though the exact distribution was not included in the Moodle data.
This time period of data collection represented two distinct academic terms: the first quarter (September 9 to October 24) and second quarter (October 28 to January 9). The dataset contained a total of 2,641,461 logs from 12,699 users. Each transaction was composed of the complete set of the following columns available from Moodle:
Time—timestamp of the of the action, up to the minute.
User ID—numerical identifier (ID) of the user performing the action.
Affected user—numerical identifier of the user affected by the action; When Teacher T sends a notification to Student S, the User ID would be that of Teacher T whereas the Affected user would be Student S.
Event context—teacher-given name of the module or activity within which the action took place, e.g., “Classroom Exercise 1 Module 1.”
Component—one of 43 Moodle-defined categories under which various events take place, e.g., Quiz.
Event name—one of 244 Moodle-defined names for actions that can be performed by the user, e.g., Quiz attempt viewed.
Description—narrative description of the action performed by the user, e.g., The user with id '1603' has viewed the attempt with id '20202' belonging to the user with id “1603” for the quiz with course module id “18804.”
Origin—The method used to access Moodle (examples: web, cli (Client), etc.).
IP address—If Moodle is accessed via the web, this gives the originating IP address (this was anonymized or deleted to ensure data privacy concerns).
The users of Moodle fell into three categories: teachers , non-editing teachers (e.g., a teaching assistant; non-editing teachers may view and grade work but may not edit or delete course content), and students . Because the logs did not include the user category, the university’s systems administrators provided the researchers with each user’s type.
We used transaction log volume, i.e., counts, as the indicator of participation. A transaction is defined as any interaction with Moodle. Each time a student performs an action such as accessing course materials or answering a quiz within Moodle, that action is logged as a transaction. The more the student works within Moodle, the more transaction Moodle logs for that student. While we were interested in broad types of transactions such as quizzes, we did not examine the actual content of course activities and resources. We did not read lectures, discussion postings, exams, quizzes, etc. To answer our research questions, an examination of transaction categories and volumes was sufficient.
Data preprocessing
The raw data consisted of 3 files of User IDs and User Types (each file representing a user type), and one transaction log file for each of the 123 days of the academic term under study. To preprocess the data, we first merged the list of User IDs and User Types with the transaction logs. We eliminated identifying features such as IP addresses, user full names, and ID numbers. We also had to parse and separate the Time column into separate Date and Time features. The log file was then aggregated according to the Date, User Type, and Component, and the rows that fall under each category were counted. All preprocessed files were then appended to a single file of transactions.
The second phase of the data preprocessing procedure, just prior to the CausalImpact analysis, was to normalize the data (See Table 1 ). We first aggregated the data frame according to User Type and Component columns (305 for 2020-10-10 and 1133 for 2020-12-23). We took the maximum possible Total for each group across all dates (6146). Then, the items in the Total column were divided by their respective maximum possible value according to the User Type and Component, normalizing the data between 0 and 1 for each User Type and Component (0.05 and 0.18).
We then decided to model three of the top ten most frequently occurring components overall: System, Quiz, and Assignment which, together, represented over 88% of all transactions (See Table 2 ). System refers to all actions related to communication and course management. Quizzes in Moodle are activities that are completed online and are often automatically graded. Assignment in Moodle is usually file uploads of work completed outside of the LMS.
CausalImpact analysis
We performed a CausalImpact analysis for four outcome variables: overall student LMS activity, the System component, the Assignment component, and the Quiz component. In this section, we discuss the analysis in three sections: predictor variable selection, time period definition, and CausalImpact results.
Predictor variable selection
We opted to use teacher and non-editing teacher transactions as predictor variables. Our theoretical grounding for this choice is the teacher expectancy effect (TEE), also known as the Pygmalion Effect. The Pygmalion Effect stems from research on how interpersonal expectations shape reality (Szumski & Karwowski, 2019 ). It is a form of self-fulfilling prophecy, asserting that teacher expectations have an impact on students’ academic progress. Through verbal and non-verbal behaviors, teachers signal their expectations to students about how the students will (not should) behave or how they will succeed or fail academically (Niari et al., 2016 ). Students then enact the behaviors or achievement levels that meet teachers’ expectations. Pygmalion effects have been observed at the individual and class level for both achievement outcomes and self-concept (see Friedrich et al., 2015 ; Szumski & Karwowski, 2019 ). These effects have been shown to persist over time (see Szumski & Karwowski, 2019 ). On this basis, we speculate that what teachers signal as their expectations for the online classes will serve as cues to the student about what they will deliver in order to pass the course.
Since teacher and non-editing teacher transactions were categorized under various components, it was necessary to determine which of these components were most predictive. We used Dynamic Time Warping (DTW) to arrive at a parsimonious set of predictor variables. As explained in (Larsen, 2021 ), the usual approach to finding the relationship between a predictor and a response variable in time series data is to use the Euclidean distance. However, this penalizes instances where the relationships between data have shifted. DTW finds the distance along the warping curve, as opposed to the raw data, to arrive at the best alignment between two time series. We used the MarketMatching R implementation of the DTW algorithm (Larsen, 2021 ). It should be noted, however, that MarketMatching will only work on predictor variables with a complete set of values and with a variance or standard deviation not equal to 0. To guarantee this, we trimmed the dataset to the top 10 most frequently used components across users. The result of this algorithm was a set of predictor variables with the closest relationship with the response variable (See Table 3 ).
Time period definition
The definitions of the pre- and post-intervention periods required some consideration. As mentioned in Sect. 2 , we collected data from the first quarter (September 9 to October 24) and second quarter (October 28 to January 9) of the academic year. The start of the second quarter was immediately disrupted by Typhoons Goni and Vamco. This led the university to suspend second quarter classes from November 16–21. The university mandated asynchronous-only classes from November 23–28 and resumed synchronous classes, if teachers chose to hold them, from November 29 onward (Vilches, 2020 ). Furthermore, the second quarter included a Christmas break from December 24 to January 3. To factor in the possible impacts of the class suspension and the Christmas break, we decided to run CausalImpact on four different time periods (See Fig. 1 ). The pre-intervention period was from September 9 to October 28, the days before the typhoons entered the Philippine Area of Responsibility (PAR). We included October 25 to 27, the period in-between the quarters, because it was during this time that teachers began contacting students to send them links to the online classroom where they would meet on the first meeting day. The intervention period was the period in which the two typhoons struck. We considered two possible endings to this period: November 13, the day Typhoon Vamco left the PAR, and November 21, the last day of the post-typhoon break. The post-intervention period followed and, like the intervention period, had two possible end dates: December 23, before the Christmas break, and January 9. Hence, we created four time periods:
Intervention period that does not include the post-typhoon break; post-intervention period that includes the Christmas break (NB-WC)
Intervention period that does not include the post-typhoon break; post-intervention period that does not include the Christmas break (NB-NC)
Intervention period that includes the post-typhoon break; post-intervention period that includes the Christmas break (WB-WC)
Intervention period that includes the post-typhoon break; post-intervention period that does not include the Christmas break (WB-NC)
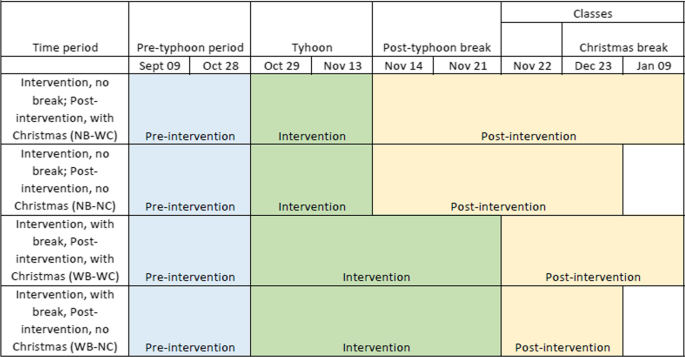
Time period definitions
Note that we were working with the same dataset reported in Lagmay and Rodrigo ( 2021 ). In this current paper, though, the end date of the intervention period and the start date of the post-intervention periods in time periods WB-WC and WB-NC are different. The dates in Fig. 1 are consistent with the university memo regarding the post-typhoon period (Vilches, 2020 ). These same time period definitions in Lagmay and Rodrigo ( 2021 ) were off by 2 days.
CausalImpact results
Tables 4 and 5 show the results of the analysis for each of the time periods.
All LMS Activity
During time period NB-WC, all LMS activity decreased significantly ( p = 0.02). The response variable had an average value of 0.17. The counterfactual prediction was 0.21. The typhoons therefore had an estimated effect of − 0.041 with a 95% confidence interval of [− 0.077, − 0.0063]. When the data points during the intervention period are summed, the response variable had an overall value of 9.61. The counterfactual prediction was 11.98 with a 95% confidence interval of [9.97, 14.10]. This means that overall student participation decreased by − 20% with a 95% confidence interval of [− 37%, − 3%].
Figure 2 a shows the CausalImpact graph of all LMS activity for time period NB-WC. Each unit on the x -axis represents one day in the time period. The topmost graph labeled “original” shows a solid line representing the actual observed data, i.e., the number of transactions per day. The broken line represents the prediction. The light blue band represents the confidence interval of the prediction. The middle graph labeled “pointwise” shows the difference between the predicted number of transactions and the actual number of transactions per day. If the predicted number of transactions for day 1 was 100 and the actual number of transactions was 80, the pointwise difference was 20. Finally, the cumulative graph at the bottom shows the accumulated difference between the predicted number of transactions and the actual number of transactions. If the pointwise difference on day 2 was 10, the accumulated difference of days 1 and 2 is 30. If the pointwise difference on day 3 was 12, the accumulated difference of days 1, 2, and 3 is 42. The gap in the pointwise and cumulative graphs is the intervention period. There is no accumulated difference during the pre-intervention period. The differences are accumulated post-intervention. Note that the cumulative graph shows a downward trend during the post-intervention period and that there was indeed a slump in the week or so following the typhoons.

CausalImpact graphs for all LMS activity
During time period NB-NC, all LMS activity also decreased significantly ( p = 0.01). Student participation had an average value of 0.18. The counterfactual prediction was 0.23. The typhoons therefore had an estimated effect of − 0.045 with a 95% interval of [− 0.082, − 0.010]. When the data points during the intervention period are summed, the response variable had an overall value of 7.41. The counterfactual prediction was 9.25 with a 95% confidence interval of [7.83, 10.77]. Like time period NB-WC, student participation decreased by − 20% with a 95% confidence interval of [− 36%, − 5%]. Figure 2 b shows the CausalImpact graph for time period NB-NC.
The results for all LMS activity during time periods WB-WC and WB-NC were insignificant. Time period WB-WC yielded a p value of 0.044, while time period WB-NC yielded a p value of 0.033. However, in both cases, the signs of the 95% CI fluctuated, which means that even if the p value implies significance, the results cannot be meaningfully interpreted. Since time periods WB-WC and WB-NC included the class suspension, it is possible that the definition of the intervention period was too long and the effect of the typhoons had already worn off. Figure 2 c, d shows a visualization of this scenario. We trim off the slump that follows immediately after the typhoons. Although the cumulative graph still follows a decreasing trajectory, the difference between the predicted and actual data is no longer significant. Note that the graph shape does not change, regardless of time period. What changes is the size of the intervention period from the end of October to around the middle of November and the length of the graph’s tail.
During time periods NB-WC (Fig. 3 a) and WB-WC (Fig. 3 c), System activity decreased, but not significantly. Although the p value of time period NB-WC was 0.03 and student participation showed a decrease of − 26%, the 95% interval of this percentage was [− 52%, + 1%]. The p value of time period WB-WC was 0.04 and the response variable showed a decrease of − 25% with a 95% interval of [− 52%, + 5%]. These fluctuations of the sign during the post-periods of the two time periods meant that the effect is not significant and cannot be meaningfully interpreted (Coqueret & Guida, 2020 ).
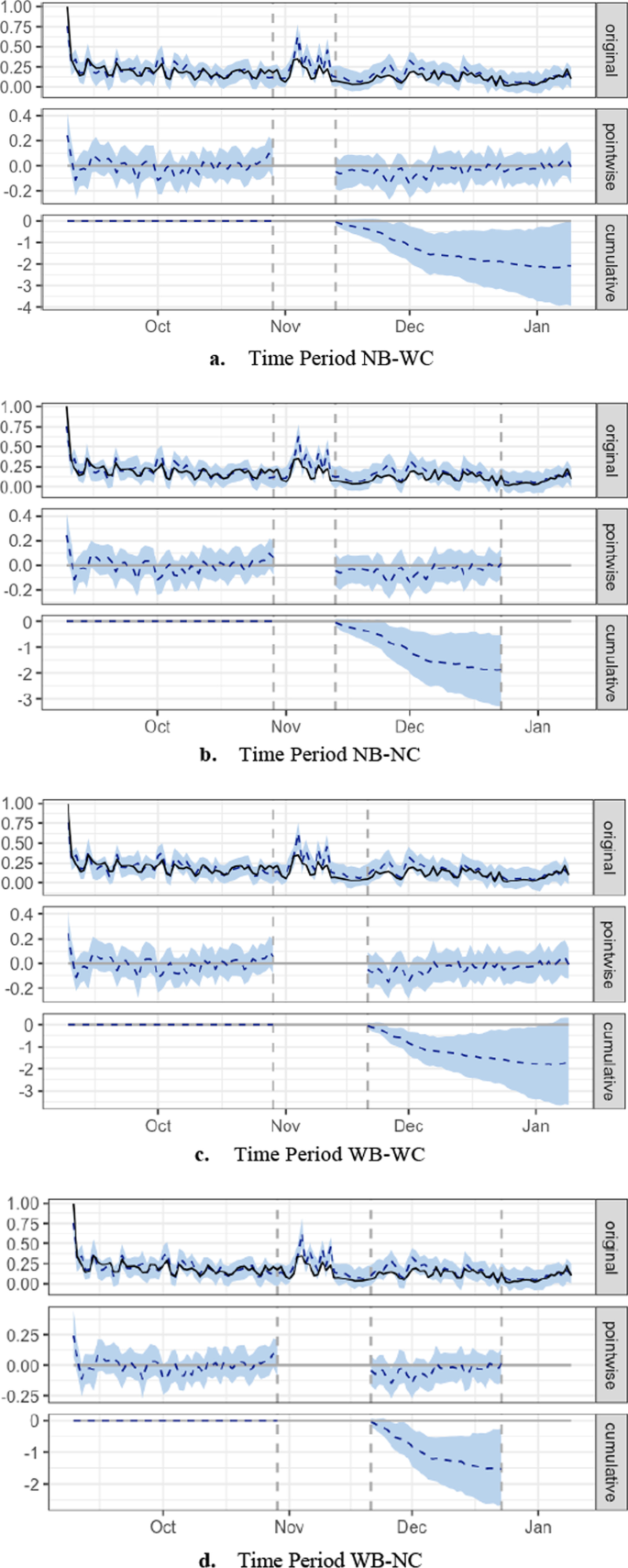
CausalImpact graphs for System component
System activity during period NB-NC (Fig. 3 b) significantly decreased ( p = 0.01). Student participation averaged 0.11 as opposed to a counterfactual prediction of 0.15 with a 95% interval of [0.12, 0.19]. The effect of the typhoons is estimated at − 0.046 with a 95% interval of [− 0.080, − 0.013]. The sum of student participation data points during the post-intervention period was 4.43 in contrast to a predicted 6.30 with a 95% interval of [4.96, 7.71].
The results of time period WB-NC (Fig. 3 d) were also statistically significant ( p = 0.01). Student participation averaged 0.12 as opposed to the predicted 0.17 with a 95% interval of [0.13, 0.20]. The effect of the typhoons was therefore estimated at − 0.046 with a 95% interval of [− 0.081, − 0.0072]. The sum of the student participation data points was 4.04 in contrast to a predicted 5.57 with a 95% interval of [4.28, 6.70].

Assignments
The effects of the typhoons on student behavior on Assignments were not significant across any of the time periods. p values were 0.14, 0.348, and 0.195 for time periods NB-WC, WB-WC, and WB-NC, respectively. Although time period NB-NC had a p value of 0.04, student participation’s sign fluctuated. It showed a decrease of − 18% with a 95% interval of [− 37%, + 2%]. This meant that the result could not be meaningfully interpreted.
The effects of the typhoons on student behavior on Quizzes were not statistically significant for any of the four time periods. p values were 0.29, 0.39, 0.45, and 0.14 for time periods NB-WC, NB-NC, WB-WC, and WB-NC, respectively.
The purpose of this paper was to determine (1) the extent to which extreme weather affected student participation during online classes and (2) whether and at what point they were able to return to pre-typhoon levels of participation. It extends the earlier work by Lagmay and Rodrigo ( 2021 ) in several ways: The earlier work only included one time period definition, which we labelled in this paper as NB-NC, while this paper experiments with four different time period definitions. Furthermore, Lagmay and Rodrigo ( 2021 ) limited the discussion of the findings to the significance of the decrease, the standard deviation, and the confidence interval. This paper also discusses the absolute and relative effects which were not discussed in the prior paper. Despite these differences in scope, the findings were consistent: Student participation decreased as a whole but those certain components of participation remained at pre-typhoon levels. These findings need to be unpacked for greater nuance.
While student participation as a whole decreased, we found that the significance of the decrease varied, first depending on the definition of the intervention period and second depending on component. When the post-intervention time period excluded the Christmas break (time periods NB-NC and WB-NC), post-typhoon participation as measured in System component significantly decreased, while when the intervention time period excluded the additional week of post-Vamco class suspensions (time periods NB-WC and NB-NC), all LMS Logs significantly decreased.
What was most interesting was that participation in the Assignments and Quizzes components was not significantly different from their predicted behavior, regardless of time period. Because we did not examine the details of actual learning design, course activities, or relative weights of assessments, these findings suggest that students continued to comply with academic assessments as assignments and quizzes make measurable contributions to their grades. System behavior, on the other hand, refers to actions such as checking the course for announcements. These activities are generally not graded. This implies that students were able to continue complying with academic requirements despite the setbacks brought on by the typhoons.
The findings from this study are consistent with findings from prior work on the negative effects of interruptions on academic outcomes. Short-term, small-scale interruptions from social media use, family and friends, sleepiness, and computer malfunctions can derail concentration and throw learning off-course (Zhang et al., 2022 ; Zureick et al., 2018 ). Hence, students who experience these interruptions tend to have lower assessment scores than peers who do not. Larger-scale interruptions such as extreme weather and other natural disasters have adverse long-term effects on educational outcomes, especially among marginalized groups (Bernabe et al., 2021 ; Groppo & Kraehnert, 2017 ; Marchetta et al., 2018 ). It is therefore unsurprising that overall student participation dropped following Typhoons Goni and Vamco.
That students were able to continue engaging with Assignments and Quizzes calls for further reflection. How did students still have the capacity to work on assessments when it seemed most logical, under the circumstances, for them to deprioritize their studies in general? The work of Lai et al. ( 2019 ) offers some insight in this regard. They found two trajectories of school recovery after a disaster: low-interrupted and high-stable. The low-interrupted trajectory referred to school performance levels that dropped following a disaster, while the high-stable trajectory referred to relatively unchanged performance levels. Schools that had higher levels of attendance in general were more likely to have high-stable trajectories, while schools with a high percentage of economically disadvantaged students were more likely to have low-interrupted trajectories. Sustained engagement with assessments despite the typhoons implies that the university examined in this study had a high-stable trajectory and that its students, by and large, were not economically disadvantaged.
There are solutions available to mitigate the effects of inclement weather. Herrera-Almanza and Cas ( 2017 ) studied the long-term academic outcomes of Filipino public school students whose schools were built as part of the Typhoon-Resistant School Building Program of the Philippine government and the Government of Japan. The project made use of Japanese pre-fabrication construction methods and materials to build more structures that were less prone to storm damage, increasing post-typhoon access to schools. The researchers found that students from these beneficiary schools accumulated more years of schooling and were more likely to complete secondary school. Programs such as this illustrate ways in which policy makers can increase the resilience of economically disadvantaged communities.
Limitations
The generalizability of these findings is subject to at least five limitations. Firstly, CausalImpact analysis requires that the predictor variables should not be affected by the same intervention as the response variable (Brodersen & Hauser, 2014 –2017). In this case, it was the likely case that the teachers and non-editing teachers were affected by the typhoons, just as their students were. To this point, we offer two counterarguments: First, we used DTW to find the teacher and non-editing teacher features that were most predictive of student behaviors. The algorithm eliminated the features with no predictive power, leaving only those that could give us a reasonable estimate of student behavior. Multicollinearity was not an issue of concern because CausalImpact’s underlying model “uses spike and slab priors to combat collinearity” (K. Larsen, personal communications, June 29, 2021). The methodology is provided in (Larsen, 2016 ).
Second, we return to our theoretical framework regarding the Pygmalion Effect (Szumski & Karwowski, 2019 ). Teacher expectations have been shown to affect student behavior, achievement, and self-concept. Since teachers continued to provide learning materials and assessments after the typhoons and throughout the second quarter, this may have signaled to the students that they were still expected to fulfill their academic obligations.
Our second limitation has to do with the population from which the data were taken. Prior research cited in the “ Effects of extreme weather on academic achievement ” section showed that extreme weather has detrimental, long-term effects on student achievement, and yet these students seemed to have flourished despite these two typhoons. One possible explanation for this is that the students in this sample were among the best in the country. They generally came from well-to-do socioeconomic backgrounds, and their families had the economic stability to withstand the typhoon’s shocks. Their resilience may not be indicative of the resilience of the Philippines or any developing country as a whole. It may, at best, serve as validation of prior findings that the impact of extreme weather varies along socioeconomic lines. Those who are more financially able will survive, possibly flourish. While it would have been revelatory to perform this analysis on data from an LMS used by less economically fortunate people, such data were not available.
Third, the university had two LMSs working in parallel, Moodle and Canvas. We were only able to capture Moodle data for this study, and the classes using the Moodle server were generally the Computer Science and Management Information Systems classes. The students were therefore technology-savvy and adept at online modes of communication. Students from other courses might have encountered greater challenges.
Fourth, the data captured here represent LMS participation but not other important outcomes such as assessment results, the quality of the educational experience, or the mental health consequences of online learning coupled with severe weather. While students and faculty evidently powered through their requirements, it would be best to triangulate these results with findings and observations from other constituency checks, for a more complete reading of our community.
Finally, as mentioned in the “ Time series analysis methods ” section, we were not able to consider using CausalTransfer, a more updated version of CausalImpact, nor any of the neural network or deep learning approaches. Future studies may consider experimenting with these other approaches to determine if they yield better results.
Despite these limitations, this paper contributes to technology-enhanced education research and practice. For education researchers, this paper adds to the literature by applying CausalImpact analysis on LMS data to determine the effects of severe weather on students. It contributes to what is quantitatively known about how Philippine students cope with online learning. In the context of severe weather, quantitative research on this subject is still scarce.
This paper serves also as a possible model for researchers who wish to determine the effects of an intervention on a system. They can consider the use of CausalImpact as a possible approach if they have sufficient pre-intervention data for CausalImpact to draw an accurate model, a clearly defined intervention period, and sufficient post-intervention data to serve as a comparison. Future researchers should also be careful with their choice of predictor variables as the behavior of predictor variables should not be affected by the intervention.
For education practitioners, this paper provides evidence that schools and their students can be resilient, and that academic continuity is possible even in the face of difficult circumstances. However, evidence of resilience for some students should not be interpreted as resilience for all. Markers of resilience such as hope and confidence must be grounded in reality (Mahdiani & Ungar, 2021 ). Resilience should not be used as an excuse for social inequalities and should not shift the responsibility to survive and thrive on people who may lack the power or resources to do so. As extreme weather events that are characteristic to the Philippines, policy makers have to invest in typhoon-resistant infrastructure (Herrera-Almanza & Cas, 2017 ) and practitioners will have to provide marginalized students with more support in order to achieve desired educational outcomes.
Availability of data and materials
The dataset(s) supporting the conclusions of this article are available in the RPTEL_CausalImpact_Lagmay_Rodrigo repository, https://github.com/KielLagmay/RPTEL_CausalImpact_Lagmay_Rodrigo .
Project name: RPTEL_CausalImpact_Lagmay_Rodrigo.
Project home page: https://github.com/KielLagmay/RPTEL_CausalImpact_Lagmay_Rodrigo .
Archived version: N/A.
Operating system(s): Windows 10 or later (with PowerShell), Windows 8.1 or later (with CMD), macOS 10.13 High Sierra or later (with BASH), macOS 10.14 Mojave or later (with ZSH), or Ubuntu 20.04 or later (with BASH) .
Programming language: R, Python, Jupyter Notebook, Shell, PowerShell, and Batch.
Other requirements: Anaconda with Python 3.6 or higher + Pyro5, pandas, import_ipynb, netifaces, and dateutil; R with CausalImpact, MarketMatching, dplyr, ggplot2, zoo, tidyr, reshape2, mctest, ppcor, and fsMTS libraries.
License: GPL-3.0.
Any restrictions to use by non-academics: For data privacy reasons, please send a request to [email protected] for the actual log files, user type files, and aggregated files.
Bernabe, A., Diop, B., Pelli, M., & Tschopp, J. (2021). Childhood exposure to storms and long-term educational attainments in India . Available at SSRN 3806538.
Bernal, J. L., Cummins, S., & Gasparrini, A. (2017). Interrupted time series regression for the evaluation of public health interventions: A tutorial. International Journal of Epidemiology, 46 (1), 348–355.
Google Scholar
Bica, I., Alaa, A. M., & van der Schaar, M. (2020). Time series deconfounder: Estimating treatment effects over time in the presence of hidden confounders. In Proceedings of the 37th international conference on machine learning (pp. 884–895). PLMR 119.
Brodersen, K. (2014). CausalImpact: A new open-source package for estimating causal effects in time series. Accessed from the Google Open Source Blog: https://opensource.googleblog.com/2014/09/causalimpact-new-open-source-package.html .
Brodersen, K. H., Gallusser, F., Koehler, J., Remy, N., & Scott, S. L. (2015). Inferring causal impact using Bayesian structural time-series models. Annals of Applied Statistics, 9 (1), 247–274.
Article Google Scholar
Brodersen & Hauser. (2014–2017). CausalImpact. Accessed from Google: http://google.github.io/CausalImpact/CausalImpact.html .
Coqueret, G., & Guida, T. (2020). Machine Learning for Factor Investing: R Version . CRC Press.
Book Google Scholar
Cunha, F., & Heckman, J. (2007). The technology of skill formation. American Economic Review, 97 (2), 31–47.
Deuchert, E., & Felfe, C. (2015). The tempest: Short-and long-term consequences of a natural disaster for children’s development. European Economic Review, 80 , 280–294.
Friedrich, A., Flunger, B., Nagengast, B., Jonkmann, K., & Trautwein, U. (2015). Pygmalion effects in the classroom: Teacher expectancy effects on students’ math achievement. Contemporary Educational Psychology, 41 , 1–12.
Groppo, V., & Kraehnert, K. (2017). The impact of extreme weather events on education. Journal of Population Economics, 30 (2), 433–472.
Herrera-Almanza, C., & Cas, A. G. (2017). Resilience to shocks during adolescence and later human capital outcomes: Evidence from natural disasters in the Philippines. Accessed online: https://econpapers.repec.org/scripts/redir.pf?u=https%3A%2F%2Fageconsearch.umn.edu%2Frecord%2F259129%2Ffiles%2FAbstracts_17_05_24_22_55_44_77__66_31_137_29_0.pdf;h=repec:ags:aaea17:259129 .
Hill, J., & Stuart, E. (2015). Causal inference: Overview. International Encyclopedia of the Social & Behavioral Sciences: Second Edition , 255–260.
International Federation of Red Cross and Red Crescent Societies. (2020a). Operation update report Philippines: Floods and typhoons 2020a (Typhoon Goni). Accessed from the ReliefWeb website: https://reliefweb.int/sites/reliefweb.int/files/resources/MDRPH041eu1_goni.pdf .
International Federation of Red Cross and Red Crescent Societies. (2020b). Operation Update Report Philippines: Floods and Typhoons 2020b (Typhoon Vamco). Accessed from the ReliefWeb website: https://reliefweb.int/sites/reliefweb.int/files/resources/Philippines%20-%20Floods%20and%20Typhoons%2020b20%20%28Typhoon%20Goni%29%20Operation%20Update%20Report%20n%C2%B0%204%2C%20DREF%20Operation%20n%C2%B0%20MDRPH041.pdf .
Joshi, K. (2019). The impact of drought on human capital in rural India. Environment and Development Economics, 24 (4), 413–436.
Kumar, S., Benigni, M., & Carley, K. M. (2016). The impact of US cyber policies on cyber-attacks trend. In 2016 IEEE conference on intelligence and security informatics (ISI) (pp. 181–186). IEEE.
Kuromiya, H., Majumdar, R., Kondo, T., Nakanishi, T., Takii, K., & Ogata, H. (2020). Impact of school closure during COVID-19 emergency: A time series analysis of learning logs. In 28th international conference on computers in education conference proceedings (Vol. 1, pp. 272–277). Asia-Pacific Society for Computers in Education (APSCE).
Lagmay, E. A., & Rodrigo, M. M. T. (2021). Quantifying the Impact of Severe Weather Conditions on Online Learning During the COVID-19 Pandemic. In 22nd International Conference on Artificial Intelligence in Education (Vol. 2, pp. 229–233). Cham: Springer.
Lai, B. S., Esnard, A. M., Wyczalkowski, C., Savage, R., & Shah, H. (2019). Trajectories of school recovery after a natural disaster: Risk and protective factors. Risk, Hazards & Crisis in Public Policy, 10 (1), 32–51.
Lalu, G. P. (2020). Student group wants academic freeze until floods clear, internet fixed. Accessed from the Inquirer.net website: https://newsinfo.inquirer.net/1361470/student-group-wants-academic-freeze-until-floods-clear-internet-is-fixed .
Larsen, K. (2016). Sorry, ARIMA, but I’m Going Bayesian. Accessed from the Multithreaded website: https://multithreaded.stitchfix.com/blog/2016/04/21/forget-arima/ .
Larsen, K. (2021). MarketMatching Package Vignette. Accessed from the Cran.r-Project website: https://cran.r-project.org/web/packages/MarketMatching/vignettes/MarketMatching-Vignette.html .
Li, S. & Bühlmann, P. (2020). Estimating heterogeneous treatment effects in nonstationary time series with state-space models. In Seminar for statistics . ETH Zürich.
Lim, B., Alaa, A., & van der Schaar, M. (2018). Forecasting treatment responses over time using recurrent marginal structural networks. In 32nd conference on neural information processing systems (pp. 7494–7504). Montréal, Canada.
Liu, R., Yin, C., & Zhang, P. (2020). estimating individual treatment effects with time-varying confounders. In 2020 IEEE international conference on data mining (pp. 382–391). ICCE.
Mahdiani, H., & Ungar, M. (2021). The dark side of resilience. Adversity and Resilience Science, 2 (3), 147–155.
Marchetta, F., Sahn, D. E., & Tiberti, L. (2018). School or work? The role of weather shocks in Madagascar. Études et Documents , 3 , CERDI.
Martin, W. (2016). Causal impact for app store analysis. In Proceedings of the 38th international conference on software engineering companion (pp. 659–661).
Moraffah, R., Sheth, P., Karami, M., Bhattacharya, A., Wang, Q., Tahir, A., Raglin, A., & Liu, H. (2021). Causal inference for time series analysis: Problems, methods and evaluation. Knowledge and Information Systems, 63 , 3041–3085.
Niari, M., Manousou, E., & Lionarakis, A. (2016). The Pygmalion effect in distance learning: A case study at the Hellenic Open University. European Journal of Open, Distance and E-Learning, 19 (1), 36–52.
Nishida, K. (2017). An Introduction to causal impact analysis. Accessed from the Exploratory.io website: https://blog.exploratory.io/an-introduction-to-causal-impact-analysis-a57bce54078e .
Perles-Ribes, J. F., Ramón-Rodríguez, A. B., Moreno-Izquierdo, L., & Torregrosa Martí, M. T. (2018). Winners and losers in the Arab uprisings: A Mediterranean tourism perspective. Current Issues in Tourism, 21 (16), 1810–1829.
Piran, F. A. S., Lacerda, D. P., Camargo, L. F. R., Viero, C. F., Teixeira, R., & Dresch, A. (2017). Product modularity and its effects on the production process: An analysis in a bus manufacturer. The International Journal of Advanced Manufacturing Technology, 88 (5–8), 2331–2343.
Szumski, G., & Karwowski, M. (2019). Exploring the Pygmalion effect: The role of teacher expectations, academic self-concept, and class context in students’ math achievement. Contemporary Educational Psychology, 59 , 101787.
Taylor, S. J., & Letham, B. (2018). Forecasting at scale. The American Statistician, 72 (1), 37–45.
UNESCO. (2021). Philippines: Education and Literacy. Accessed from the UNESCO website: http://uis.unesco.org/en/country/ph .
Vilches, M. L. (2020). Additional Measures in Dealing with Typhoon Aftermath. Accessed from the Ateneo website: https://drive.google.com/file/d/1MiJjcGRMOvwmm9WCK3PoyYlsvKEJavjM/view .
World Vision. (2021). 2013 Typhoon Haiyan: Facts, FAQs, and how to help. Accessed from the World Vision website: https://www.worldvision.org/disaster-relief-news-stories/2013-typhoon-haiyan-facts .
Zhang, C. J., Walser, E., Mierzwa, A., & Ott, M. (2022). Learning interrupted: Educational impact of interruptions on surgical residents. Journal of Surgical Education, 79 , 875–884.
Zureick, A. H., Burk-Rafel, J., Purkiss, J. A., & Hortsch, M. (2018). The interrupted learner: How distractions during live and video lectures influence learning outcomes. Anatomical Sciences Education, 11 (4), 366–376.
Download references
Acknowledgements
We thank Hiroyuki Kuromiya and Hiroaki Ogata of Kyoto University for their advice. Finally, we thank the Ateneo Laboratory for the Learning Sciences for its constant support.
Funding for this project was provided by Ateneo Research Institute for Science and Engineering (ARISE) of Ateneo de Manila University through the grant entitled: Analysis of Student and Faculty Behavior within Canvas and Moodle.
Author information
Authors and affiliations.
Ateneo de Manila University, Quezon City, Metro Manila, Philippines
Ezekiel Adriel D. Lagmay & Maria Mercedes T. Rodrigo
You can also search for this author in PubMed Google Scholar
Contributions
EADL was primarily responsible for the quantitative analysis of the data and contributed to the writing of the paper. MMTR conceptualized the study, scaffolded the analysis of the data, and contributed to the writing of the paper. All authors read and approved the final manuscript.
Corresponding author
Correspondence to Maria Mercedes T. Rodrigo .
Ethics declarations
Competing interests.
We have no competing interests to declare.
Additional information
Publisher's note.
Springer Nature remains neutral with regard to jurisdictional claims in published maps and institutional affiliations.
Rights and permissions
Open Access This article is licensed under a Creative Commons Attribution 4.0 International License, which permits use, sharing, adaptation, distribution and reproduction in any medium or format, as long as you give appropriate credit to the original author(s) and the source, provide a link to the Creative Commons licence, and indicate if changes were made. The images or other third party material in this article are included in the article's Creative Commons licence, unless indicated otherwise in a credit line to the material. If material is not included in the article's Creative Commons licence and your intended use is not permitted by statutory regulation or exceeds the permitted use, you will need to obtain permission directly from the copyright holder. To view a copy of this licence, visit http://creativecommons.org/licenses/by/4.0/ .
Reprints and permissions
About this article
Cite this article.
Lagmay, E.A.D., Rodrigo, M.M.T. The impact of extreme weather on student online learning participation. RPTEL 17 , 26 (2022). https://doi.org/10.1186/s41039-022-00201-2
Download citation
Received : 30 July 2021
Accepted : 04 July 2022
Published : 14 July 2022
DOI : https://doi.org/10.1186/s41039-022-00201-2
Share this article
Anyone you share the following link with will be able to read this content:
Sorry, a shareable link is not currently available for this article.
Provided by the Springer Nature SharedIt content-sharing initiative
- CausalImpact
- Learning Management System
- Philippines
Advertisement
The effects of online education on academic success: A meta-analysis study
- Published: 06 September 2021
- Volume 27 , pages 429–450, ( 2022 )
Cite this article
- Hakan Ulum ORCID: orcid.org/0000-0002-1398-6935 1
85k Accesses
32 Citations
4 Altmetric
Explore all metrics
The purpose of this study is to analyze the effect of online education, which has been extensively used on student achievement since the beginning of the pandemic. In line with this purpose, a meta-analysis of the related studies focusing on the effect of online education on students’ academic achievement in several countries between the years 2010 and 2021 was carried out. Furthermore, this study will provide a source to assist future studies with comparing the effect of online education on academic achievement before and after the pandemic. This meta-analysis study consists of 27 studies in total. The meta-analysis involves the studies conducted in the USA, Taiwan, Turkey, China, Philippines, Ireland, and Georgia. The studies included in the meta-analysis are experimental studies, and the total sample size is 1772. In the study, the funnel plot, Duval and Tweedie’s Trip and Fill Analysis, Orwin’s Safe N Analysis, and Egger’s Regression Test were utilized to determine the publication bias, which has been found to be quite low. Besides, Hedge’s g statistic was employed to measure the effect size for the difference between the means performed in accordance with the random effects model. The results of the study show that the effect size of online education on academic achievement is on a medium level. The heterogeneity test results of the meta-analysis study display that the effect size does not differ in terms of class level, country, online education approaches, and lecture moderators.
Explore related subjects
- Artificial Intelligence
- Digital Education and Educational Technology
Avoid common mistakes on your manuscript.
1 Introduction
Information and communication technologies have become a powerful force in transforming the educational settings around the world. The pandemic has been an important factor in transferring traditional physical classrooms settings through adopting information and communication technologies and has also accelerated the transformation. The literature supports that learning environments connected to information and communication technologies highly satisfy students. Therefore, we need to keep interest in technology-based learning environments. Clearly, technology has had a huge impact on young people's online lives. This digital revolution can synergize the educational ambitions and interests of digitally addicted students. In essence, COVID-19 has provided us with an opportunity to embrace online learning as education systems have to keep up with the rapid emergence of new technologies.
Information and communication technologies that have an effect on all spheres of life are also actively included in the education field. With the recent developments, using technology in education has become inevitable due to personal and social reasons (Usta, 2011a ). Online education may be given as an example of using information and communication technologies as a consequence of the technological developments. Also, it is crystal clear that online learning is a popular way of obtaining instruction (Demiralay et al., 2016 ; Pillay et al., 2007 ), which is defined by Horton ( 2000 ) as a way of education that is performed through a web browser or an online application without requiring an extra software or a learning source. Furthermore, online learning is described as a way of utilizing the internet to obtain the related learning sources during the learning process, to interact with the content, the teacher, and other learners, as well as to get support throughout the learning process (Ally, 2004 ). Online learning has such benefits as learning independently at any time and place (Vrasidas & MsIsaac, 2000 ), granting facility (Poole, 2000 ), flexibility (Chizmar & Walbert, 1999 ), self-regulation skills (Usta, 2011b ), learning with collaboration, and opportunity to plan self-learning process.
Even though online education practices have not been comprehensive as it is now, internet and computers have been used in education as alternative learning tools in correlation with the advances in technology. The first distance education attempt in the world was initiated by the ‘Steno Courses’ announcement published in Boston newspaper in 1728. Furthermore, in the nineteenth century, Sweden University started the “Correspondence Composition Courses” for women, and University Correspondence College was afterwards founded for the correspondence courses in 1843 (Arat & Bakan, 2011 ). Recently, distance education has been performed through computers, assisted by the facilities of the internet technologies, and soon, it has evolved into a mobile education practice that is emanating from progress in the speed of internet connection, and the development of mobile devices.
With the emergence of pandemic (Covid-19), face to face education has almost been put to a halt, and online education has gained significant importance. The Microsoft management team declared to have 750 users involved in the online education activities on the 10 th March, just before the pandemic; however, on March 24, they informed that the number of users increased significantly, reaching the number of 138,698 users (OECD, 2020 ). This event supports the view that it is better to commonly use online education rather than using it as a traditional alternative educational tool when students do not have the opportunity to have a face to face education (Geostat, 2019 ). The period of Covid-19 pandemic has emerged as a sudden state of having limited opportunities. Face to face education has stopped in this period for a long time. The global spread of Covid-19 affected more than 850 million students all around the world, and it caused the suspension of face to face education. Different countries have proposed several solutions in order to maintain the education process during the pandemic. Schools have had to change their curriculum, and many countries supported the online education practices soon after the pandemic. In other words, traditional education gave its way to online education practices. At least 96 countries have been motivated to access online libraries, TV broadcasts, instructions, sources, video lectures, and online channels (UNESCO, 2020 ). In such a painful period, educational institutions went through online education practices by the help of huge companies such as Microsoft, Google, Zoom, Skype, FaceTime, and Slack. Thus, online education has been discussed in the education agenda more intensively than ever before.
Although online education approaches were not used as comprehensively as it has been used recently, it was utilized as an alternative learning approach in education for a long time in parallel with the development of technology, internet and computers. The academic achievement of the students is often aimed to be promoted by employing online education approaches. In this regard, academicians in various countries have conducted many studies on the evaluation of online education approaches and published the related results. However, the accumulation of scientific data on online education approaches creates difficulties in keeping, organizing and synthesizing the findings. In this research area, studies are being conducted at an increasing rate making it difficult for scientists to be aware of all the research outside of their expertise. Another problem encountered in the related study area is that online education studies are repetitive. Studies often utilize slightly different methods, measures, and/or examples to avoid duplication. This erroneous approach makes it difficult to distinguish between significant differences in the related results. In other words, if there are significant differences in the results of the studies, it may be difficult to express what variety explains the differences in these results. One obvious solution to these problems is to systematically review the results of various studies and uncover the sources. One method of performing such systematic syntheses is the application of meta-analysis which is a methodological and statistical approach to draw conclusions from the literature. At this point, how effective online education applications are in increasing the academic success is an important detail. Has online education, which is likely to be encountered frequently in the continuing pandemic period, been successful in the last ten years? If successful, how much was the impact? Did different variables have an impact on this effect? Academics across the globe have carried out studies on the evaluation of online education platforms and publishing the related results (Chiao et al., 2018 ). It is quite important to evaluate the results of the studies that have been published up until now, and that will be published in the future. Has the online education been successful? If it has been, how big is the impact? Do the different variables affect this impact? What should we consider in the next coming online education practices? These questions have all motivated us to carry out this study. We have conducted a comprehensive meta-analysis study that tries to provide a discussion platform on how to develop efficient online programs for educators and policy makers by reviewing the related studies on online education, presenting the effect size, and revealing the effect of diverse variables on the general impact.
There have been many critical discussions and comprehensive studies on the differences between online and face to face learning; however, the focus of this paper is different in the sense that it clarifies the magnitude of the effect of online education and teaching process, and it represents what factors should be controlled to help increase the effect size. Indeed, the purpose here is to provide conscious decisions in the implementation of the online education process.
The general impact of online education on the academic achievement will be discovered in the study. Therefore, this will provide an opportunity to get a general overview of the online education which has been practiced and discussed intensively in the pandemic period. Moreover, the general impact of online education on academic achievement will be analyzed, considering different variables. In other words, the current study will allow to totally evaluate the study results from the related literature, and to analyze the results considering several cultures, lectures, and class levels. Considering all the related points, this study seeks to answer the following research questions:
What is the effect size of online education on academic achievement?
How do the effect sizes of online education on academic achievement change according to the moderator variable of the country?
How do the effect sizes of online education on academic achievement change according to the moderator variable of the class level?
How do the effect sizes of online education on academic achievement change according to the moderator variable of the lecture?
How do the effect sizes of online education on academic achievement change according to the moderator variable of the online education approaches?
This study aims at determining the effect size of online education, which has been highly used since the beginning of the pandemic, on students’ academic achievement in different courses by using a meta-analysis method. Meta-analysis is a synthesis method that enables gathering of several study results accurately and efficiently, and getting the total results in the end (Tsagris & Fragkos, 2018 ).
2.1 Selecting and coding the data (studies)
The required literature for the meta-analysis study was reviewed in July, 2020, and the follow-up review was conducted in September, 2020. The purpose of the follow-up review was to include the studies which were published in the conduction period of this study, and which met the related inclusion criteria. However, no study was encountered to be included in the follow-up review.
In order to access the studies in the meta-analysis, the databases of Web of Science, ERIC, and SCOPUS were reviewed by utilizing the keywords ‘online learning and online education’. Not every database has a search engine that grants access to the studies by writing the keywords, and this obstacle was considered to be an important problem to be overcome. Therefore, a platform that has a special design was utilized by the researcher. With this purpose, through the open access system of Cukurova University Library, detailed reviews were practiced using EBSCO Information Services (EBSCO) that allow reviewing the whole collection of research through a sole searching box. Since the fundamental variables of this study are online education and online learning, the literature was systematically reviewed in the related databases (Web of Science, ERIC, and SCOPUS) by referring to the keywords. Within this scope, 225 articles were accessed, and the studies were included in the coding key list formed by the researcher. The name of the researchers, the year, the database (Web of Science, ERIC, and SCOPUS), the sample group and size, the lectures that the academic achievement was tested in, the country that the study was conducted in, and the class levels were all included in this coding key.
The following criteria were identified to include 225 research studies which were coded based on the theoretical basis of the meta-analysis study: (1) The studies should be published in the refereed journals between the years 2020 and 2021, (2) The studies should be experimental studies that try to determine the effect of online education and online learning on academic achievement, (3) The values of the stated variables or the required statistics to calculate these values should be stated in the results of the studies, and (4) The sample group of the study should be at a primary education level. These criteria were also used as the exclusion criteria in the sense that the studies that do not meet the required criteria were not included in the present study.
After the inclusion criteria were determined, a systematic review process was conducted, following the year criterion of the study by means of EBSCO. Within this scope, 290,365 studies that analyze the effect of online education and online learning on academic achievement were accordingly accessed. The database (Web of Science, ERIC, and SCOPUS) was also used as a filter by analyzing the inclusion criteria. Hence, the number of the studies that were analyzed was 58,616. Afterwards, the keyword ‘primary education’ was used as the filter and the number of studies included in the study decreased to 3152. Lastly, the literature was reviewed by using the keyword ‘academic achievement’ and 225 studies were accessed. All the information of 225 articles was included in the coding key.
It is necessary for the coders to review the related studies accurately and control the validity, safety, and accuracy of the studies (Stewart & Kamins, 2001 ). Within this scope, the studies that were determined based on the variables used in this study were first reviewed by three researchers from primary education field, then the accessed studies were combined and processed in the coding key by the researcher. All these studies that were processed in the coding key were analyzed in accordance with the inclusion criteria by all the researchers in the meetings, and it was decided that 27 studies met the inclusion criteria (Atici & Polat, 2010 ; Carreon, 2018 ; Ceylan & Elitok Kesici, 2017 ; Chae & Shin, 2016 ; Chiang et al. 2014 ; Ercan, 2014 ; Ercan et al., 2016 ; Gwo-Jen et al., 2018 ; Hayes & Stewart, 2016 ; Hwang et al., 2012 ; Kert et al., 2017 ; Lai & Chen, 2010 ; Lai et al., 2015 ; Meyers et al., 2015 ; Ravenel et al., 2014 ; Sung et al., 2016 ; Wang & Chen, 2013 ; Yu, 2019 ; Yu & Chen, 2014 ; Yu & Pan, 2014 ; Yu et al., 2010 ; Zhong et al., 2017 ). The data from the studies meeting the inclusion criteria were independently processed in the second coding key by three researchers, and consensus meetings were arranged for further discussion. After the meetings, researchers came to an agreement that the data were coded accurately and precisely. Having identified the effect sizes and heterogeneity of the study, moderator variables that will show the differences between the effect sizes were determined. The data related to the determined moderator variables were added to the coding key by three researchers, and a new consensus meeting was arranged. After the meeting, researchers came to an agreement that moderator variables were coded accurately and precisely.
2.2 Study group
27 studies are included in the meta-analysis. The total sample size of the studies that are included in the analysis is 1772. The characteristics of the studies included are given in Table 1 .
2.3 Publication bias
Publication bias is the low capability of published studies on a research subject to represent all completed studies on the same subject (Card, 2011 ; Littell et al., 2008 ). Similarly, publication bias is the state of having a relationship between the probability of the publication of a study on a subject, and the effect size and significance that it produces. Within this scope, publication bias may occur when the researchers do not want to publish the study as a result of failing to obtain the expected results, or not being approved by the scientific journals, and consequently not being included in the study synthesis (Makowski et al., 2019 ). The high possibility of publication bias in a meta-analysis study negatively affects (Pecoraro, 2018 ) the accuracy of the combined effect size, causing the average effect size to be reported differently than it should be (Borenstein et al., 2009 ). For this reason, the possibility of publication bias in the included studies was tested before determining the effect sizes of the relationships between the stated variables. The possibility of publication bias of this meta-analysis study was analyzed by using the funnel plot, Orwin’s Safe N Analysis, Duval and Tweedie’s Trip and Fill Analysis, and Egger’s Regression Test.
2.4 Selecting the model
After determining the probability of publication bias of this meta-analysis study, the statistical model used to calculate the effect sizes was selected. The main approaches used in the effect size calculations according to the differentiation level of inter-study variance are fixed and random effects models (Pigott, 2012 ). Fixed effects model refers to the homogeneity of the characteristics of combined studies apart from the sample sizes, while random effects model refers to the parameter diversity between the studies (Cumming, 2012 ). While calculating the average effect size in the random effects model (Deeks et al., 2008 ) that is based on the assumption that effect predictions of different studies are only the result of a similar distribution, it is necessary to consider several situations such as the effect size apart from the sample error of combined studies, characteristics of the participants, duration, scope, and pattern of the study (Littell et al., 2008 ). While deciding the model in the meta-analysis study, the assumptions on the sample characteristics of the studies included in the analysis and the inferences that the researcher aims to make should be taken into consideration. The fact that the sample characteristics of the studies conducted in the field of social sciences are affected by various parameters shows that using random effects model is more appropriate in this sense. Besides, it is stated that the inferences made with the random effects model are beyond the studies included in the meta-analysis (Field, 2003 ; Field & Gillett, 2010 ). Therefore, using random effects model also contributes to the generalization of research data. The specified criteria for the statistical model selection show that according to the nature of the meta-analysis study, the model should be selected just before the analysis (Borenstein et al., 2007 ; Littell et al., 2008 ). Within this framework, it was decided to make use of the random effects model, considering that the students who are the samples of the studies included in the meta-analysis are from different countries and cultures, the sample characteristics of the studies differ, and the patterns and scopes of the studies vary as well.
2.5 Heterogeneity
Meta-analysis facilitates analyzing the research subject with different parameters by showing the level of diversity between the included studies. Within this frame, whether there is a heterogeneous distribution between the studies included in the study or not has been evaluated in the present study. The heterogeneity of the studies combined in this meta-analysis study has been determined through Q and I 2 tests. Q test evaluates the random distribution probability of the differences between the observed results (Deeks et al., 2008 ). Q value exceeding 2 value calculated according to the degree of freedom and significance, indicates the heterogeneity of the combined effect sizes (Card, 2011 ). I 2 test, which is the complementary of the Q test, shows the heterogeneity amount of the effect sizes (Cleophas & Zwinderman, 2017 ). I 2 value being higher than 75% is explained as high level of heterogeneity.
In case of encountering heterogeneity in the studies included in the meta-analysis, the reasons of heterogeneity can be analyzed by referring to the study characteristics. The study characteristics which may be related to the heterogeneity between the included studies can be interpreted through subgroup analysis or meta-regression analysis (Deeks et al., 2008 ). While determining the moderator variables, the sufficiency of the number of variables, the relationship between the moderators, and the condition to explain the differences between the results of the studies have all been considered in the present study. Within this scope, it was predicted in this meta-analysis study that the heterogeneity can be explained with the country, class level, and lecture moderator variables of the study in terms of the effect of online education, which has been highly used since the beginning of the pandemic, and it has an impact on the students’ academic achievement in different lectures. Some subgroups were evaluated and categorized together, considering that the number of effect sizes of the sub-dimensions of the specified variables is not sufficient to perform moderator analysis (e.g. the countries where the studies were conducted).
2.6 Interpreting the effect sizes
Effect size is a factor that shows how much the independent variable affects the dependent variable positively or negatively in each included study in the meta-analysis (Dinçer, 2014 ). While interpreting the effect sizes obtained from the meta-analysis, the classifications of Cohen et al. ( 2007 ) have been utilized. The case of differentiating the specified relationships of the situation of the country, class level, and school subject variables of the study has been identified through the Q test, degree of freedom, and p significance value Fig. 1 and 2 .
3 Findings and results
The purpose of this study is to determine the effect size of online education on academic achievement. Before determining the effect sizes in the study, the probability of publication bias of this meta-analysis study was analyzed by using the funnel plot, Orwin’s Safe N Analysis, Duval and Tweedie’s Trip and Fill Analysis, and Egger’s Regression Test.
When the funnel plots are examined, it is seen that the studies included in the analysis are distributed symmetrically on both sides of the combined effect size axis, and they are generally collected in the middle and lower sections. The probability of publication bias is low according to the plots. However, since the results of the funnel scatter plots may cause subjective interpretations, they have been supported by additional analyses (Littell et al., 2008 ). Therefore, in order to provide an extra proof for the probability of publication bias, it has been analyzed through Orwin’s Safe N Analysis, Duval and Tweedie’s Trip and Fill Analysis, and Egger’s Regression Test (Table 2 ).
Table 2 consists of the results of the rates of publication bias probability before counting the effect size of online education on academic achievement. According to the table, Orwin Safe N analysis results show that it is not necessary to add new studies to the meta-analysis in order for Hedges g to reach a value outside the range of ± 0.01. The Duval and Tweedie test shows that excluding the studies that negatively affect the symmetry of the funnel scatter plots for each meta-analysis or adding their exact symmetrical equivalents does not significantly differentiate the calculated effect size. The insignificance of the Egger tests results reveals that there is no publication bias in the meta-analysis study. The results of the analysis indicate the high internal validity of the effect sizes and the adequacy of representing the studies conducted on the relevant subject.
In this study, it was aimed to determine the effect size of online education on academic achievement after testing the publication bias. In line with the first purpose of the study, the forest graph regarding the effect size of online education on academic achievement is shown in Fig. 3 , and the statistics regarding the effect size are given in Table 3 .
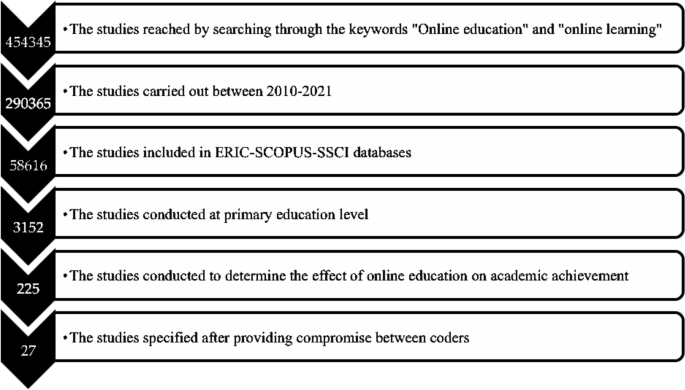
The flow chart of the scanning and selection process of the studies
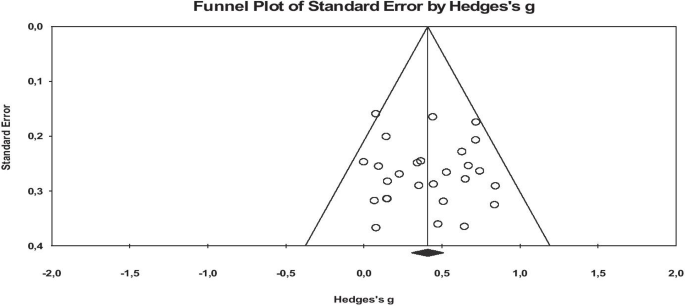
Funnel plot graphics representing the effect size of the effects of online education on academic success
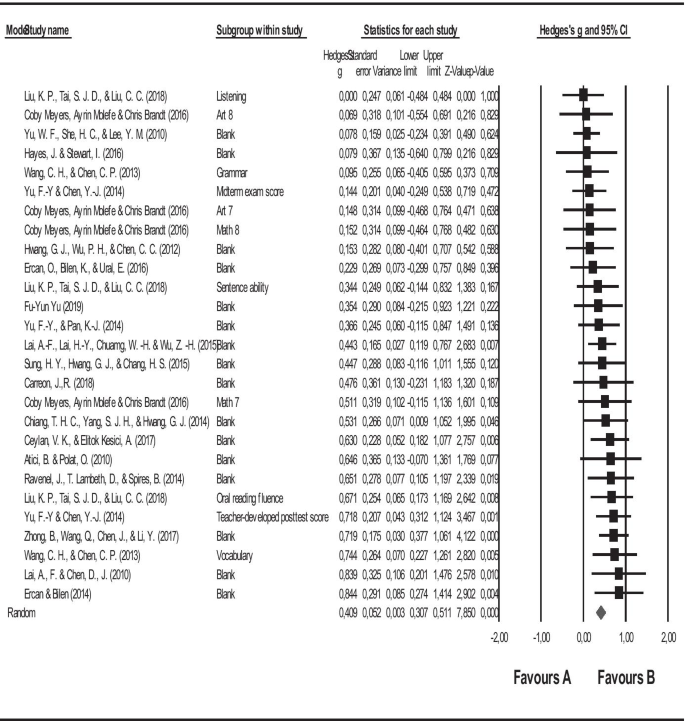
Forest graph related to the effect size of online education on academic success
The square symbols in the forest graph in Fig. 3 represent the effect sizes, while the horizontal lines show the intervals in 95% confidence of the effect sizes, and the diamond symbol shows the overall effect size. When the forest graph is analyzed, it is seen that the lower and upper limits of the combined effect sizes are generally close to each other, and the study loads are similar. This similarity in terms of study loads indicates the similarity of the contribution of the combined studies to the overall effect size.
Figure 3 clearly represents that the study of Liu and others (Liu et al., 2018 ) has the lowest, and the study of Ercan and Bilen ( 2014 ) has the highest effect sizes. The forest graph shows that all the combined studies and the overall effect are positive. Furthermore, it is simply understood from the forest graph in Fig. 3 and the effect size statistics in Table 3 that the results of the meta-analysis study conducted with 27 studies and analyzing the effect of online education on academic achievement illustrate that this relationship is on average level (= 0.409).
After the analysis of the effect size in the study, whether the studies included in the analysis are distributed heterogeneously or not has also been analyzed. The heterogeneity of the combined studies was determined through the Q and I 2 tests. As a result of the heterogeneity test, Q statistical value was calculated as 29.576. With 26 degrees of freedom at 95% significance level in the chi-square table, the critical value is accepted as 38.885. The Q statistical value (29.576) counted in this study is lower than the critical value of 38.885. The I 2 value, which is the complementary of the Q statistics, is 12.100%. This value indicates that the accurate heterogeneity or the total variability that can be attributed to variability between the studies is 12%. Besides, p value is higher than (0.285) p = 0.05. All these values [Q (26) = 29.579, p = 0.285; I2 = 12.100] indicate that there is a homogeneous distribution between the effect sizes, and fixed effects model should be used to interpret these effect sizes. However, some researchers argue that even if the heterogeneity is low, it should be evaluated based on the random effects model (Borenstein et al., 2007 ). Therefore, this study gives information about both models. The heterogeneity of the combined studies has been attempted to be explained with the characteristics of the studies included in the analysis. In this context, the final purpose of the study is to determine the effect of the country, academic level, and year variables on the findings. Accordingly, the statistics regarding the comparison of the stated relations according to the countries where the studies were conducted are given in Table 4 .
As seen in Table 4 , the effect of online education on academic achievement does not differ significantly according to the countries where the studies were conducted in. Q test results indicate the heterogeneity of the relationships between the variables in terms of countries where the studies were conducted in. According to the table, the effect of online education on academic achievement was reported as the highest in other countries, and the lowest in the US. The statistics regarding the comparison of the stated relations according to the class levels are given in Table 5 .
As seen in Table 5 , the effect of online education on academic achievement does not differ according to the class level. However, the effect of online education on academic achievement is the highest in the 4 th class. The statistics regarding the comparison of the stated relations according to the class levels are given in Table 6 .
As seen in Table 6 , the effect of online education on academic achievement does not differ according to the school subjects included in the studies. However, the effect of online education on academic achievement is the highest in ICT subject.
The obtained effect size in the study was formed as a result of the findings attained from primary studies conducted in 7 different countries. In addition, these studies are the ones on different approaches to online education (online learning environments, social networks, blended learning, etc.). In this respect, the results may raise some questions about the validity and generalizability of the results of the study. However, the moderator analyzes, whether for the country variable or for the approaches covered by online education, did not create significant differences in terms of the effect sizes. If significant differences were to occur in terms of effect sizes, we could say that the comparisons we will make by comparing countries under the umbrella of online education would raise doubts in terms of generalizability. Moreover, no study has been found in the literature that is not based on a special approach or does not contain a specific technique conducted under the name of online education alone. For instance, one of the commonly used definitions is blended education which is defined as an educational model in which online education is combined with traditional education method (Colis & Moonen, 2001 ). Similarly, Rasmussen ( 2003 ) defines blended learning as “a distance education method that combines technology (high technology such as television, internet, or low technology such as voice e-mail, conferences) with traditional education and training.” Further, Kerres and Witt (2003) define blended learning as “combining face-to-face learning with technology-assisted learning.” As it is clearly observed, online education, which has a wider scope, includes many approaches.
As seen in Table 7 , the effect of online education on academic achievement does not differ according to online education approaches included in the studies. However, the effect of online education on academic achievement is the highest in Web Based Problem Solving Approach.
4 Conclusions and discussion
Considering the developments during the pandemics, it is thought that the diversity in online education applications as an interdisciplinary pragmatist field will increase, and the learning content and processes will be enriched with the integration of new technologies into online education processes. Another prediction is that more flexible and accessible learning opportunities will be created in online education processes, and in this way, lifelong learning processes will be strengthened. As a result, it is predicted that in the near future, online education and even digital learning with a newer name will turn into the main ground of education instead of being an alternative or having a support function in face-to-face learning. The lessons learned from the early period online learning experience, which was passed with rapid adaptation due to the Covid19 epidemic, will serve to develop this method all over the world, and in the near future, online learning will become the main learning structure through increasing its functionality with the contribution of new technologies and systems. If we look at it from this point of view, there is a necessity to strengthen online education.
In this study, the effect of online learning on academic achievement is at a moderate level. To increase this effect, the implementation of online learning requires support from teachers to prepare learning materials, to design learning appropriately, and to utilize various digital-based media such as websites, software technology and various other tools to support the effectiveness of online learning (Rolisca & Achadiyah, 2014 ). According to research conducted by Rahayu et al. ( 2017 ), it has been proven that the use of various types of software increases the effectiveness and quality of online learning. Implementation of online learning can affect students' ability to adapt to technological developments in that it makes students use various learning resources on the internet to access various types of information, and enables them to get used to performing inquiry learning and active learning (Hart et al., 2019 ; Prestiadi et al., 2019 ). In addition, there may be many reasons for the low level of effect in this study. The moderator variables examined in this study could be a guide in increasing the level of practical effect. However, the effect size did not differ significantly for all moderator variables. Different moderator analyzes can be evaluated in order to increase the level of impact of online education on academic success. If confounding variables that significantly change the effect level are detected, it can be spoken more precisely in order to increase this level. In addition to the technical and financial problems, the level of impact will increase if a few other difficulties are eliminated such as students, lack of interaction with the instructor, response time, and lack of traditional classroom socialization.
In addition, COVID-19 pandemic related social distancing has posed extreme difficulties for all stakeholders to get online as they have to work in time constraints and resource constraints. Adopting the online learning environment is not just a technical issue, it is a pedagogical and instructive challenge as well. Therefore, extensive preparation of teaching materials, curriculum, and assessment is vital in online education. Technology is the delivery tool and requires close cross-collaboration between teaching, content and technology teams (CoSN, 2020 ).
Online education applications have been used for many years. However, it has come to the fore more during the pandemic process. This result of necessity has brought with it the discussion of using online education instead of traditional education methods in the future. However, with this research, it has been revealed that online education applications are moderately effective. The use of online education instead of face-to-face education applications can only be possible with an increase in the level of success. This may have been possible with the experience and knowledge gained during the pandemic process. Therefore, the meta-analysis of experimental studies conducted in the coming years will guide us. In this context, experimental studies using online education applications should be analyzed well. It would be useful to identify variables that can change the level of impacts with different moderators. Moderator analyzes are valuable in meta-analysis studies (for example, the role of moderators in Karl Pearson's typhoid vaccine studies). In this context, each analysis study sheds light on future studies. In meta-analyses to be made about online education, it would be beneficial to go beyond the moderators determined in this study. Thus, the contribution of similar studies to the field will increase more.
The purpose of this study is to determine the effect of online education on academic achievement. In line with this purpose, the studies that analyze the effect of online education approaches on academic achievement have been included in the meta-analysis. The total sample size of the studies included in the meta-analysis is 1772. While the studies included in the meta-analysis were conducted in the US, Taiwan, Turkey, China, Philippines, Ireland, and Georgia, the studies carried out in Europe could not be reached. The reason may be attributed to that there may be more use of quantitative research methods from a positivist perspective in the countries with an American academic tradition. As a result of the study, it was found out that the effect size of online education on academic achievement (g = 0.409) was moderate. In the studies included in the present research, we found that online education approaches were more effective than traditional ones. However, contrary to the present study, the analysis of comparisons between online and traditional education in some studies shows that face-to-face traditional learning is still considered effective compared to online learning (Ahmad et al., 2016 ; Hamdani & Priatna, 2020 ; Wei & Chou, 2020 ). Online education has advantages and disadvantages. The advantages of online learning compared to face-to-face learning in the classroom is the flexibility of learning time in online learning, the learning time does not include a single program, and it can be shaped according to circumstances (Lai et al., 2019 ). The next advantage is the ease of collecting assignments for students, as these can be done without having to talk to the teacher. Despite this, online education has several weaknesses, such as students having difficulty in understanding the material, teachers' inability to control students, and students’ still having difficulty interacting with teachers in case of internet network cuts (Swan, 2007 ). According to Astuti et al ( 2019 ), face-to-face education method is still considered better by students than e-learning because it is easier to understand the material and easier to interact with teachers. The results of the study illustrated that the effect size (g = 0.409) of online education on academic achievement is of medium level. Therefore, the results of the moderator analysis showed that the effect of online education on academic achievement does not differ in terms of country, lecture, class level, and online education approaches variables. After analyzing the literature, several meta-analyses on online education were published (Bernard et al., 2004 ; Machtmes & Asher, 2000 ; Zhao et al., 2005 ). Typically, these meta-analyzes also include the studies of older generation technologies such as audio, video, or satellite transmission. One of the most comprehensive studies on online education was conducted by Bernard et al. ( 2004 ). In this study, 699 independent effect sizes of 232 studies published from 1985 to 2001 were analyzed, and face-to-face education was compared to online education, with respect to success criteria and attitudes of various learners from young children to adults. In this meta-analysis, an overall effect size close to zero was found for the students' achievement (g + = 0.01).
In another meta-analysis study carried out by Zhao et al. ( 2005 ), 98 effect sizes were examined, including 51 studies on online education conducted between 1996 and 2002. According to the study of Bernard et al. ( 2004 ), this meta-analysis focuses on the activities done in online education lectures. As a result of the research, an overall effect size close to zero was found for online education utilizing more than one generation technology for students at different levels. However, the salient point of the meta-analysis study of Zhao et al. is that it takes the average of different types of results used in a study to calculate an overall effect size. This practice is problematic because the factors that develop one type of learner outcome (e.g. learner rehabilitation), particularly course characteristics and practices, may be quite different from those that develop another type of outcome (e.g. learner's achievement), and it may even cause damage to the latter outcome. While mixing the studies with different types of results, this implementation may obscure the relationship between practices and learning.
Some meta-analytical studies have focused on the effectiveness of the new generation distance learning courses accessed through the internet for specific student populations. For instance, Sitzmann and others (Sitzmann et al., 2006 ) reviewed 96 studies published from 1996 to 2005, comparing web-based education of job-related knowledge or skills with face-to-face one. The researchers found that web-based education in general was slightly more effective than face-to-face education, but it is insufficient in terms of applicability ("knowing how to apply"). In addition, Sitzmann et al. ( 2006 ) revealed that Internet-based education has a positive effect on theoretical knowledge in quasi-experimental studies; however, it positively affects face-to-face education in experimental studies performed by random assignment. This moderator analysis emphasizes the need to pay attention to the factors of designs of the studies included in the meta-analysis. The designs of the studies included in this meta-analysis study were ignored. This can be presented as a suggestion to the new studies that will be conducted.
Another meta-analysis study was conducted by Cavanaugh et al. ( 2004 ), in which they focused on online education. In this study on internet-based distance education programs for students under 12 years of age, the researchers combined 116 results from 14 studies published between 1999 and 2004 to calculate an overall effect that was not statistically different from zero. The moderator analysis carried out in this study showed that there was no significant factor affecting the students' success. This meta-analysis used multiple results of the same study, ignoring the fact that different results of the same student would not be independent from each other.
In conclusion, some meta-analytical studies analyzed the consequences of online education for a wide range of students (Bernard et al., 2004 ; Zhao et al., 2005 ), and the effect sizes were generally low in these studies. Furthermore, none of the large-scale meta-analyzes considered the moderators, database quality standards or class levels in the selection of the studies, while some of them just referred to the country and lecture moderators. Advances in internet-based learning tools, the pandemic process, and increasing popularity in different learning contexts have required a precise meta-analysis of students' learning outcomes through online learning. Previous meta-analysis studies were typically based on the studies, involving narrow range of confounding variables. In the present study, common but significant moderators such as class level and lectures during the pandemic process were discussed. For instance, the problems have been experienced especially in terms of eligibility of class levels in online education platforms during the pandemic process. It was found that there is a need to study and make suggestions on whether online education can meet the needs of teachers and students.
Besides, the main forms of online education in the past were to watch the open lectures of famous universities and educational videos of institutions. In addition, online education is mainly a classroom-based teaching implemented by teachers in their own schools during the pandemic period, which is an extension of the original school education. This meta-analysis study will stand as a source to compare the effect size of the online education forms of the past decade with what is done today, and what will be done in the future.
Lastly, the heterogeneity test results of the meta-analysis study display that the effect size does not differ in terms of class level, country, online education approaches, and lecture moderators.
*Studies included in meta-analysis
Ahmad, S., Sumardi, K., & Purnawan, P. (2016). Komparasi Peningkatan Hasil Belajar Antara Pembelajaran Menggunakan Sistem Pembelajaran Online Terpadu Dengan Pembelajaran Klasikal Pada Mata Kuliah Pneumatik Dan Hidrolik. Journal of Mechanical Engineering Education, 2 (2), 286–292.
Article Google Scholar
Ally, M. (2004). Foundations of educational theory for online learning. Theory and Practice of Online Learning, 2 , 15–44. Retrieved on the 11th of September, 2020 from https://eddl.tru.ca/wp-content/uploads/2018/12/01_Anderson_2008-Theory_and_Practice_of_Online_Learning.pdf
Arat, T., & Bakan, Ö. (2011). Uzaktan eğitim ve uygulamaları. Selçuk Üniversitesi Sosyal Bilimler Meslek Yüksek Okulu Dergisi , 14 (1–2), 363–374. https://doi.org/10.29249/selcuksbmyd.540741
Astuti, C. C., Sari, H. M. K., & Azizah, N. L. (2019). Perbandingan Efektifitas Proses Pembelajaran Menggunakan Metode E-Learning dan Konvensional. Proceedings of the ICECRS, 2 (1), 35–40.
*Atici, B., & Polat, O. C. (2010). Influence of the online learning environments and tools on the student achievement and opinions. Educational Research and Reviews, 5 (8), 455–464. Retrieved on the 11th of October, 2020 from https://academicjournals.org/journal/ERR/article-full-text-pdf/4C8DD044180.pdf
Bernard, R. M., Abrami, P. C., Lou, Y., Borokhovski, E., Wade, A., Wozney, L., et al. (2004). How does distance education compare with classroom instruction? A meta- analysis of the empirical literature. Review of Educational Research, 3 (74), 379–439. https://doi.org/10.3102/00346543074003379
Borenstein, M., Hedges, L. V., Higgins, J. P. T., & Rothstein, H. R. (2009). Introduction to meta-analysis . Wiley.
Book Google Scholar
Borenstein, M., Hedges, L., & Rothstein, H. (2007). Meta-analysis: Fixed effect vs. random effects . UK: Wiley.
Card, N. A. (2011). Applied meta-analysis for social science research: Methodology in the social sciences . Guilford.
Google Scholar
*Carreon, J. R. (2018 ). Facebook as integrated blended learning tool in technology and livelihood education exploratory. Retrieved on the 1st of October, 2020 from https://files.eric.ed.gov/fulltext/EJ1197714.pdf
Cavanaugh, C., Gillan, K. J., Kromrey, J., Hess, M., & Blomeyer, R. (2004). The effects of distance education on K-12 student outcomes: A meta-analysis. Learning Point Associates/North Central Regional Educational Laboratory (NCREL) . Retrieved on the 11th of September, 2020 from https://files.eric.ed.gov/fulltext/ED489533.pdf
*Ceylan, V. K., & Elitok Kesici, A. (2017). Effect of blended learning to academic achievement. Journal of Human Sciences, 14 (1), 308. https://doi.org/10.14687/jhs.v14i1.4141
*Chae, S. E., & Shin, J. H. (2016). Tutoring styles that encourage learner satisfaction, academic engagement, and achievement in an online environment. Interactive Learning Environments, 24(6), 1371–1385. https://doi.org/10.1080/10494820.2015.1009472
*Chiang, T. H. C., Yang, S. J. H., & Hwang, G. J. (2014). An augmented reality-based mobile learning system to improve students’ learning achievements and motivations in natural science inquiry activities. Educational Technology and Society, 17 (4), 352–365. Retrieved on the 11th of September, 2020 from https://www.researchgate.net/profile/Gwo_Jen_Hwang/publication/287529242_An_Augmented_Reality-based_Mobile_Learning_System_to_Improve_Students'_Learning_Achievements_and_Motivations_in_Natural_Science_Inquiry_Activities/links/57198c4808ae30c3f9f2c4ac.pdf
Chiao, H. M., Chen, Y. L., & Huang, W. H. (2018). Examining the usability of an online virtual tour-guiding platform for cultural tourism education. Journal of Hospitality, Leisure, Sport & Tourism Education, 23 (29–38), 1. https://doi.org/10.1016/j.jhlste.2018.05.002
Chizmar, J. F., & Walbert, M. S. (1999). Web-based learning environments guided by principles of good teaching practice. Journal of Economic Education, 30 (3), 248–264. https://doi.org/10.2307/1183061
Cleophas, T. J., & Zwinderman, A. H. (2017). Modern meta-analysis: Review and update of methodologies . Switzerland: Springer. https://doi.org/10.1007/978-3-319-55895-0
Cohen, L., Manion, L., & Morrison, K. (2007). Observation. Research Methods in Education, 6 , 396–412. Retrieved on the 11th of September, 2020 from https://www.researchgate.net/profile/Nabil_Ashraf2/post/How_to_get_surface_potential_Vs_Voltage_curve_from_CV_and_GV_measurements_of_MOS_capacitor/attachment/5ac6033cb53d2f63c3c405b4/AS%3A612011817844736%401522926396219/download/Very+important_C-V+characterization+Lehigh+University+thesis.pdf
Colis, B., & Moonen, J. (2001). Flexible Learning in a Digital World: Experiences and Expectations. Open & Distance Learning Series . Stylus Publishing.
CoSN. (2020). COVID-19 Response: Preparing to Take School Online. CoSN. (2020). COVID-19 Response: Preparing to Take School Online. Retrieved on the 3rd of September, 2021 from https://www.cosn.org/sites/default/files/COVID-19%20Member%20Exclusive_0.pdf
Cumming, G. (2012). Understanding new statistics: Effect sizes, confidence intervals, and meta-analysis. New York, USA: Routledge. https://doi.org/10.4324/9780203807002
Deeks, J. J., Higgins, J. P. T., & Altman, D. G. (2008). Analysing data and undertaking meta-analyses . In J. P. T. Higgins & S. Green (Eds.), Cochrane handbook for systematic reviews of interventions (pp. 243–296). Sussex: John Wiley & Sons. https://doi.org/10.1002/9780470712184.ch9
Demiralay, R., Bayır, E. A., & Gelibolu, M. F. (2016). Öğrencilerin bireysel yenilikçilik özellikleri ile çevrimiçi öğrenmeye hazır bulunuşlukları ilişkisinin incelenmesi. Eğitim ve Öğretim Araştırmaları Dergisi, 5 (1), 161–168. https://doi.org/10.23891/efdyyu.2017.10
Dinçer, S. (2014). Eğitim bilimlerinde uygulamalı meta-analiz. Pegem Atıf İndeksi, 2014(1), 1–133. https://doi.org/10.14527/pegem.001
*Durak, G., Cankaya, S., Yunkul, E., & Ozturk, G. (2017). The effects of a social learning network on students’ performances and attitudes. European Journal of Education Studies, 3 (3), 312–333. 10.5281/zenodo.292951
*Ercan, O. (2014). Effect of web assisted education supported by six thinking hats on students’ academic achievement in science and technology classes . European Journal of Educational Research, 3 (1), 9–23. https://doi.org/10.12973/eu-jer.3.1.9
Ercan, O., & Bilen, K. (2014). Effect of web assisted education supported by six thinking hats on students’ academic achievement in science and technology classes. European Journal of Educational Research, 3 (1), 9–23.
*Ercan, O., Bilen, K., & Ural, E. (2016). “Earth, sun and moon”: Computer assisted instruction in secondary school science - Achievement and attitudes. Issues in Educational Research, 26 (2), 206–224. https://doi.org/10.12973/eu-jer.3.1.9
Field, A. P. (2003). The problems in using fixed-effects models of meta-analysis on real-world data. Understanding Statistics, 2 (2), 105–124. https://doi.org/10.1207/s15328031us0202_02
Field, A. P., & Gillett, R. (2010). How to do a meta-analysis. British Journal of Mathematical and Statistical Psychology, 63 (3), 665–694. https://doi.org/10.1348/00071010x502733
Geostat. (2019). ‘Share of households with internet access’, National statistics office of Georgia . Retrieved on the 2nd September 2020 from https://www.geostat.ge/en/modules/categories/106/information-and-communication-technologies-usage-in-households
*Gwo-Jen, H., Nien-Ting, T., & Xiao-Ming, W. (2018). Creating interactive e-books through learning by design: The impacts of guided peer-feedback on students’ learning achievements and project outcomes in science courses. Journal of Educational Technology & Society., 21 (1), 25–36. Retrieved on the 2nd of October, 2020 https://ae-uploads.uoregon.edu/ISTE/ISTE2019/PROGRAM_SESSION_MODEL/HANDOUTS/112172923/CreatingInteractiveeBooksthroughLearningbyDesignArticle2018.pdf
Hamdani, A. R., & Priatna, A. (2020). Efektifitas implementasi pembelajaran daring (full online) dimasa pandemi Covid-19 pada jenjang Sekolah Dasar di Kabupaten Subang. Didaktik: Jurnal Ilmiah PGSD STKIP Subang, 6 (1), 1–9.
Hart, C. M., Berger, D., Jacob, B., Loeb, S., & Hill, M. (2019). Online learning, offline outcomes: Online course taking and high school student performance. Aera Open, 5(1).
*Hayes, J., & Stewart, I. (2016). Comparing the effects of derived relational training and computer coding on intellectual potential in school-age children. The British Journal of Educational Psychology, 86 (3), 397–411. https://doi.org/10.1111/bjep.12114
Horton, W. K. (2000). Designing web-based training: How to teach anyone anything anywhere anytime (Vol. 1). Wiley Publishing.
*Hwang, G. J., Wu, P. H., & Chen, C. C. (2012). An online game approach for improving students’ learning performance in web-based problem-solving activities. Computers and Education, 59 (4), 1246–1256. https://doi.org/10.1016/j.compedu.2012.05.009
*Kert, S. B., Köşkeroğlu Büyükimdat, M., Uzun, A., & Çayiroğlu, B. (2017). Comparing active game-playing scores and academic performances of elementary school students. Education 3–13, 45 (5), 532–542. https://doi.org/10.1080/03004279.2016.1140800
*Lai, A. F., & Chen, D. J. (2010). Web-based two-tier diagnostic test and remedial learning experiment. International Journal of Distance Education Technologies, 8 (1), 31–53. https://doi.org/10.4018/jdet.2010010103
*Lai, A. F., Lai, H. Y., Chuang W. H., & Wu, Z.H. (2015). Developing a mobile learning management system for outdoors nature science activities based on 5e learning cycle. Proceedings of the International Conference on e-Learning, ICEL. Proceedings of the International Association for Development of the Information Society (IADIS) International Conference on e-Learning (Las Palmas de Gran Canaria, Spain, July 21–24, 2015). Retrieved on the 14th November 2020 from https://files.eric.ed.gov/fulltext/ED562095.pdf
Lai, C. H., Lin, H. W., Lin, R. M., & Tho, P. D. (2019). Effect of peer interaction among online learning community on learning engagement and achievement. International Journal of Distance Education Technologies (IJDET), 17 (1), 66–77.
Littell, J. H., Corcoran, J., & Pillai, V. (2008). Systematic reviews and meta-analysis . Oxford University.
*Liu, K. P., Tai, S. J. D., & Liu, C. C. (2018). Enhancing language learning through creation: the effect of digital storytelling on student learning motivation and performance in a school English course. Educational Technology Research and Development, 66 (4), 913–935. https://doi.org/10.1007/s11423-018-9592-z
Machtmes, K., & Asher, J. W. (2000). A meta-analysis of the effectiveness of telecourses in distance education. American Journal of Distance Education, 14 (1), 27–46. https://doi.org/10.1080/08923640009527043
Makowski, D., Piraux, F., & Brun, F. (2019). From experimental network to meta-analysis: Methods and applications with R for agronomic and environmental sciences. Dordrecht: Springer. https://doi.org/10.1007/978-94-024_1696-1
* Meyers, C., Molefe, A., & Brandt, C. (2015). The Impact of the" Enhancing Missouri's Instructional Networked Teaching Strategies"(eMINTS) Program on Student Achievement, 21st-Century Skills, and Academic Engagement--Second-Year Results . Society for Research on Educational Effectiveness. Retrieved on the 14 th November, 2020 from https://files.eric.ed.gov/fulltext/ED562508.pdf
OECD. (2020). ‘A framework to guide an education response to the COVID-19 Pandemic of 2020 ’. https://doi.org/10.26524/royal.37.6
Pecoraro, V. (2018). Appraising evidence . In G. Biondi-Zoccai (Ed.), Diagnostic meta-analysis: A useful tool for clinical decision-making (pp. 99–114). Cham, Switzerland: Springer. https://doi.org/10.1007/978-3-319-78966-8_9
Pigott, T. (2012). Advances in meta-analysis . Springer.
Pillay, H. , Irving, K., & Tones, M. (2007). Validation of the diagnostic tool for assessing Tertiary students’ readiness for online learning. Higher Education Research & Development, 26 (2), 217–234. https://doi.org/10.1080/07294360701310821
Prestiadi, D., Zulkarnain, W., & Sumarsono, R. B. (2019). Visionary leadership in total quality management: efforts to improve the quality of education in the industrial revolution 4.0. In the 4th International Conference on Education and Management (COEMA 2019). Atlantis Press
Poole, D. M. (2000). Student participation in a discussion-oriented online course: a case study. Journal of Research on Computing in Education, 33 (2), 162–177. https://doi.org/10.1080/08886504.2000.10782307
Rahayu, F. S., Budiyanto, D., & Palyama, D. (2017). Analisis penerimaan e-learning menggunakan technology acceptance model (Tam)(Studi Kasus: Universitas Atma Jaya Yogyakarta). Jurnal Terapan Teknologi Informasi, 1 (2), 87–98.
Rasmussen, R. C. (2003). The quantity and quality of human interaction in a synchronous blended learning environment . Brigham Young University Press.
*Ravenel, J., T. Lambeth, D., & Spires, B. (2014). Effects of computer-based programs on mathematical achievement scores for fourth-grade students. i-manager’s Journal on School Educational Technology, 10 (1), 8–21. https://doi.org/10.26634/jsch.10.1.2830
Rolisca, R. U. C., & Achadiyah, B. N. (2014). Pengembangan media evaluasi pembelajaran dalam bentuk online berbasis e-learning menggunakan software wondershare quiz creator dalam mata pelajaran akuntansi SMA Brawijaya Smart School (BSS). Jurnal Pendidikan Akuntansi Indonesia, 12(2).
Sitzmann, T., Kraiger, K., Stewart, D., & Wisher, R. (2006). The comparative effective- ness of Web-based and classroom instruction: A meta-analysis . Personnel Psychology, 59 (3), 623–664. https://doi.org/10.1111/j.1744-6570.2006.00049.x
Stewart, D. W., & Kamins, M. A. (2001). Developing a coding scheme and coding study reports. In M. W. Lipsey & D. B. Wilson (Eds.), Practical metaanalysis: Applied social research methods series (Vol. 49, pp. 73–90). Sage.
Swan, K. (2007). Research on online learning. Journal of Asynchronous Learning Networks, 11 (1), 55–59.
*Sung, H. Y., Hwang, G. J., & Chang, Y. C. (2016). Development of a mobile learning system based on a collaborative problem-posing strategy. Interactive Learning Environments, 24 (3), 456–471. https://doi.org/10.1080/10494820.2013.867889
Tsagris, M., & Fragkos, K. C. (2018). Meta-analyses of clinical trials versus diagnostic test accuracy studies. In G. Biondi-Zoccai (Ed.), Diagnostic meta-analysis: A useful tool for clinical decision-making (pp. 31–42). Cham, Switzerland: Springer. https://doi.org/10.1007/978-3-319-78966-8_4
UNESCO. (2020, Match 13). COVID-19 educational disruption and response. Retrieved on the 14 th November 2020 from https://en.unesco.org/themes/education-emergencies/ coronavirus-school-closures
Usta, E. (2011a). The effect of web-based learning environments on attitudes of students regarding computer and internet. Procedia-Social and Behavioral Sciences, 28 (262–269), 1. https://doi.org/10.1016/j.sbspro.2011.11.051
Usta, E. (2011b). The examination of online self-regulated learning skills in web-based learning environments in terms of different variables. Turkish Online Journal of Educational Technology-TOJET, 10 (3), 278–286. Retrieved on the 14th November 2020 from https://files.eric.ed.gov/fulltext/EJ944994.pdf
Vrasidas, C. & MsIsaac, M. S. (2000). Principles of pedagogy and evaluation for web-based learning. Educational Media International, 37 (2), 105–111. https://doi.org/10.1080/095239800410405
*Wang, C. H., & Chen, C. P. (2013). Effects of facebook tutoring on learning english as a second language. Proceedings of the International Conference e-Learning 2013, (2009), 135–142. Retrieved on the 15th November 2020 from https://files.eric.ed.gov/fulltext/ED562299.pdf
Wei, H. C., & Chou, C. (2020). Online learning performance and satisfaction: Do perceptions and readiness matter? Distance Education, 41 (1), 48–69.
*Yu, F. Y. (2019). The learning potential of online student-constructed tests with citing peer-generated questions. Interactive Learning Environments, 27 (2), 226–241. https://doi.org/10.1080/10494820.2018.1458040
*Yu, F. Y., & Chen, Y. J. (2014). Effects of student-generated questions as the source of online drill-and-practice activities on learning . British Journal of Educational Technology, 45 (2), 316–329. https://doi.org/10.1111/bjet.12036
*Yu, F. Y., & Pan, K. J. (2014). The effects of student question-generation with online prompts on learning. Educational Technology and Society, 17 (3), 267–279. Retrieved on the 15th November 2020 from http://citeseerx.ist.psu.edu/viewdoc/download?doi=10.1.1.565.643&rep=rep1&type=pdf
*Yu, W. F., She, H. C., & Lee, Y. M. (2010). The effects of web-based/non-web-based problem-solving instruction and high/low achievement on students’ problem-solving ability and biology achievement. Innovations in Education and Teaching International, 47 (2), 187–199. https://doi.org/10.1080/14703291003718927
Zhao, Y., Lei, J., Yan, B, Lai, C., & Tan, S. (2005). A practical analysis of research on the effectiveness of distance education. Teachers College Record, 107 (8). https://doi.org/10.1111/j.1467-9620.2005.00544.x
*Zhong, B., Wang, Q., Chen, J., & Li, Y. (2017). Investigating the period of switching roles in pair programming in a primary school. Educational Technology and Society, 20 (3), 220–233. Retrieved on the 15th November 2020 from https://repository.nie.edu.sg/bitstream/10497/18946/1/ETS-20-3-220.pdf
Download references
Author information
Authors and affiliations.
Primary Education, Ministry of Turkish National Education, Mersin, Turkey
You can also search for this author in PubMed Google Scholar
Corresponding author
Correspondence to Hakan Ulum .
Additional information
Publisher's note.
Springer Nature remains neutral with regard to jurisdictional claims in published maps and institutional affiliations.
Rights and permissions
Reprints and permissions
About this article
Ulum, H. The effects of online education on academic success: A meta-analysis study. Educ Inf Technol 27 , 429–450 (2022). https://doi.org/10.1007/s10639-021-10740-8
Download citation
Received : 06 December 2020
Accepted : 30 August 2021
Published : 06 September 2021
Issue Date : January 2022
DOI : https://doi.org/10.1007/s10639-021-10740-8
Share this article
Anyone you share the following link with will be able to read this content:
Sorry, a shareable link is not currently available for this article.
Provided by the Springer Nature SharedIt content-sharing initiative
- Online education
- Student achievement
- Academic success
- Meta-analysis
- Find a journal
- Publish with us
- Track your research

An official website of the United States government
The .gov means it’s official. Federal government websites often end in .gov or .mil. Before sharing sensitive information, make sure you’re on a federal government site.
The site is secure. The https:// ensures that you are connecting to the official website and that any information you provide is encrypted and transmitted securely.
- Publications
- Account settings
The PMC website is updating on October 15, 2024. Learn More or Try it out now .
- Advanced Search
- Journal List
- Springer Nature - PMC COVID-19 Collection

Students’ online learning challenges during the pandemic and how they cope with them: The case of the Philippines
Jessie s. barrot.
College of Education, Arts and Sciences, National University, Manila, Philippines
Ian I. Llenares
Leo s. del rosario, associated data.
The datasets used and/or analysed during the current study are available from the corresponding author on reasonable request.
Recently, the education system has faced an unprecedented health crisis that has shaken up its foundation. Given today’s uncertainties, it is vital to gain a nuanced understanding of students’ online learning experience in times of the COVID-19 pandemic. Although many studies have investigated this area, limited information is available regarding the challenges and the specific strategies that students employ to overcome them. Thus, this study attempts to fill in the void. Using a mixed-methods approach, the findings revealed that the online learning challenges of college students varied in terms of type and extent. Their greatest challenge was linked to their learning environment at home, while their least challenge was technological literacy and competency. The findings further revealed that the COVID-19 pandemic had the greatest impact on the quality of the learning experience and students’ mental health. In terms of strategies employed by students, the most frequently used were resource management and utilization, help-seeking, technical aptitude enhancement, time management, and learning environment control. Implications for classroom practice, policy-making, and future research are discussed.
Introduction
Since the 1990s, the world has seen significant changes in the landscape of education as a result of the ever-expanding influence of technology. One such development is the adoption of online learning across different learning contexts, whether formal or informal, academic and non-academic, and residential or remotely. We began to witness schools, teachers, and students increasingly adopt e-learning technologies that allow teachers to deliver instruction interactively, share resources seamlessly, and facilitate student collaboration and interaction (Elaish et al., 2019 ; Garcia et al., 2018 ). Although the efficacy of online learning has long been acknowledged by the education community (Barrot, 2020 , 2021 ; Cavanaugh et al., 2009 ; Kebritchi et al., 2017 ; Tallent-Runnels et al., 2006 ; Wallace, 2003 ), evidence on the challenges in its implementation continues to build up (e.g., Boelens et al., 2017 ; Rasheed et al., 2020 ).
Recently, the education system has faced an unprecedented health crisis (i.e., COVID-19 pandemic) that has shaken up its foundation. Thus, various governments across the globe have launched a crisis response to mitigate the adverse impact of the pandemic on education. This response includes, but is not limited to, curriculum revisions, provision for technological resources and infrastructure, shifts in the academic calendar, and policies on instructional delivery and assessment. Inevitably, these developments compelled educational institutions to migrate to full online learning until face-to-face instruction is allowed. The current circumstance is unique as it could aggravate the challenges experienced during online learning due to restrictions in movement and health protocols (Gonzales et al., 2020 ; Kapasia et al., 2020 ). Given today’s uncertainties, it is vital to gain a nuanced understanding of students’ online learning experience in times of the COVID-19 pandemic. To date, many studies have investigated this area with a focus on students’ mental health (Copeland et al., 2021 ; Fawaz et al., 2021 ), home learning (Suryaman et al., 2020 ), self-regulation (Carter et al., 2020 ), virtual learning environment (Almaiah et al., 2020 ; Hew et al., 2020 ; Tang et al., 2020 ), and students’ overall learning experience (e.g., Adarkwah, 2021 ; Day et al., 2021 ; Khalil et al., 2020 ; Singh et al., 2020 ). There are two key differences that set the current study apart from the previous studies. First, it sheds light on the direct impact of the pandemic on the challenges that students experience in an online learning space. Second, the current study explores students’ coping strategies in this new learning setup. Addressing these areas would shed light on the extent of challenges that students experience in a full online learning space, particularly within the context of the pandemic. Meanwhile, our nuanced understanding of the strategies that students use to overcome their challenges would provide relevant information to school administrators and teachers to better support the online learning needs of students. This information would also be critical in revisiting the typology of strategies in an online learning environment.
Literature review
Education and the covid-19 pandemic.
In December 2019, an outbreak of a novel coronavirus, known as COVID-19, occurred in China and has spread rapidly across the globe within a few months. COVID-19 is an infectious disease caused by a new strain of coronavirus that attacks the respiratory system (World Health Organization, 2020 ). As of January 2021, COVID-19 has infected 94 million people and has caused 2 million deaths in 191 countries and territories (John Hopkins University, 2021 ). This pandemic has created a massive disruption of the educational systems, affecting over 1.5 billion students. It has forced the government to cancel national examinations and the schools to temporarily close, cease face-to-face instruction, and strictly observe physical distancing. These events have sparked the digital transformation of higher education and challenged its ability to respond promptly and effectively. Schools adopted relevant technologies, prepared learning and staff resources, set systems and infrastructure, established new teaching protocols, and adjusted their curricula. However, the transition was smooth for some schools but rough for others, particularly those from developing countries with limited infrastructure (Pham & Nguyen, 2020 ; Simbulan, 2020 ).
Inevitably, schools and other learning spaces were forced to migrate to full online learning as the world continues the battle to control the vicious spread of the virus. Online learning refers to a learning environment that uses the Internet and other technological devices and tools for synchronous and asynchronous instructional delivery and management of academic programs (Usher & Barak, 2020 ; Huang, 2019 ). Synchronous online learning involves real-time interactions between the teacher and the students, while asynchronous online learning occurs without a strict schedule for different students (Singh & Thurman, 2019 ). Within the context of the COVID-19 pandemic, online learning has taken the status of interim remote teaching that serves as a response to an exigency. However, the migration to a new learning space has faced several major concerns relating to policy, pedagogy, logistics, socioeconomic factors, technology, and psychosocial factors (Donitsa-Schmidt & Ramot, 2020 ; Khalil et al., 2020 ; Varea & González-Calvo, 2020 ). With reference to policies, government education agencies and schools scrambled to create fool-proof policies on governance structure, teacher management, and student management. Teachers, who were used to conventional teaching delivery, were also obliged to embrace technology despite their lack of technological literacy. To address this problem, online learning webinars and peer support systems were launched. On the part of the students, dropout rates increased due to economic, psychological, and academic reasons. Academically, although it is virtually possible for students to learn anything online, learning may perhaps be less than optimal, especially in courses that require face-to-face contact and direct interactions (Franchi, 2020 ).
Related studies
Recently, there has been an explosion of studies relating to the new normal in education. While many focused on national policies, professional development, and curriculum, others zeroed in on the specific learning experience of students during the pandemic. Among these are Copeland et al. ( 2021 ) and Fawaz et al. ( 2021 ) who examined the impact of COVID-19 on college students’ mental health and their coping mechanisms. Copeland et al. ( 2021 ) reported that the pandemic adversely affected students’ behavioral and emotional functioning, particularly attention and externalizing problems (i.e., mood and wellness behavior), which were caused by isolation, economic/health effects, and uncertainties. In Fawaz et al.’s ( 2021 ) study, students raised their concerns on learning and evaluation methods, overwhelming task load, technical difficulties, and confinement. To cope with these problems, students actively dealt with the situation by seeking help from their teachers and relatives and engaging in recreational activities. These active-oriented coping mechanisms of students were aligned with Carter et al.’s ( 2020 ), who explored students’ self-regulation strategies.
In another study, Tang et al. ( 2020 ) examined the efficacy of different online teaching modes among engineering students. Using a questionnaire, the results revealed that students were dissatisfied with online learning in general, particularly in the aspect of communication and question-and-answer modes. Nonetheless, the combined model of online teaching with flipped classrooms improved students’ attention, academic performance, and course evaluation. A parallel study was undertaken by Hew et al. ( 2020 ), who transformed conventional flipped classrooms into fully online flipped classes through a cloud-based video conferencing app. Their findings suggested that these two types of learning environments were equally effective. They also offered ways on how to effectively adopt videoconferencing-assisted online flipped classrooms. Unlike the two studies, Suryaman et al. ( 2020 ) looked into how learning occurred at home during the pandemic. Their findings showed that students faced many obstacles in a home learning environment, such as lack of mastery of technology, high Internet cost, and limited interaction/socialization between and among students. In a related study, Kapasia et al. ( 2020 ) investigated how lockdown impacts students’ learning performance. Their findings revealed that the lockdown made significant disruptions in students’ learning experience. The students also reported some challenges that they faced during their online classes. These include anxiety, depression, poor Internet service, and unfavorable home learning environment, which were aggravated when students are marginalized and from remote areas. Contrary to Kapasia et al.’s ( 2020 ) findings, Gonzales et al. ( 2020 ) found that confinement of students during the pandemic had significant positive effects on their performance. They attributed these results to students’ continuous use of learning strategies which, in turn, improved their learning efficiency.
Finally, there are those that focused on students’ overall online learning experience during the COVID-19 pandemic. One such study was that of Singh et al. ( 2020 ), who examined students’ experience during the COVID-19 pandemic using a quantitative descriptive approach. Their findings indicated that students appreciated the use of online learning during the pandemic. However, half of them believed that the traditional classroom setting was more effective than the online learning platform. Methodologically, the researchers acknowledge that the quantitative nature of their study restricts a deeper interpretation of the findings. Unlike the above study, Khalil et al. ( 2020 ) qualitatively explored the efficacy of synchronized online learning in a medical school in Saudi Arabia. The results indicated that students generally perceive synchronous online learning positively, particularly in terms of time management and efficacy. However, they also reported technical (internet connectivity and poor utility of tools), methodological (content delivery), and behavioral (individual personality) challenges. Their findings also highlighted the failure of the online learning environment to address the needs of courses that require hands-on practice despite efforts to adopt virtual laboratories. In a parallel study, Adarkwah ( 2021 ) examined students’ online learning experience during the pandemic using a narrative inquiry approach. The findings indicated that Ghanaian students considered online learning as ineffective due to several challenges that they encountered. Among these were lack of social interaction among students, poor communication, lack of ICT resources, and poor learning outcomes. More recently, Day et al. ( 2021 ) examined the immediate impact of COVID-19 on students’ learning experience. Evidence from six institutions across three countries revealed some positive experiences and pre-existing inequities. Among the reported challenges are lack of appropriate devices, poor learning space at home, stress among students, and lack of fieldwork and access to laboratories.
Although there are few studies that report the online learning challenges that higher education students experience during the pandemic, limited information is available regarding the specific strategies that they use to overcome them. It is in this context that the current study was undertaken. This mixed-methods study investigates students’ online learning experience in higher education. Specifically, the following research questions are addressed: (1) What is the extent of challenges that students experience in an online learning environment? (2) How did the COVID-19 pandemic impact the online learning challenges that students experience? (3) What strategies did students use to overcome the challenges?
Conceptual framework
The typology of challenges examined in this study is largely based on Rasheed et al.’s ( 2020 ) review of students’ experience in an online learning environment. These challenges are grouped into five general clusters, namely self-regulation (SRC), technological literacy and competency (TLCC), student isolation (SIC), technological sufficiency (TSC), and technological complexity (TCC) challenges (Rasheed et al., 2020 , p. 5). SRC refers to a set of behavior by which students exercise control over their emotions, actions, and thoughts to achieve learning objectives. TLCC relates to a set of challenges about students’ ability to effectively use technology for learning purposes. SIC relates to the emotional discomfort that students experience as a result of being lonely and secluded from their peers. TSC refers to a set of challenges that students experience when accessing available online technologies for learning. Finally, there is TCC which involves challenges that students experience when exposed to complex and over-sufficient technologies for online learning.
To extend Rasheed et al. ( 2020 ) categories and to cover other potential challenges during online classes, two more clusters were added, namely learning resource challenges (LRC) and learning environment challenges (LEC) (Buehler, 2004 ; Recker et al., 2004 ; Seplaki et al., 2014 ; Xue et al., 2020 ). LRC refers to a set of challenges that students face relating to their use of library resources and instructional materials, whereas LEC is a set of challenges that students experience related to the condition of their learning space that shapes their learning experiences, beliefs, and attitudes. Since learning environment at home and learning resources available to students has been reported to significantly impact the quality of learning and their achievement of learning outcomes (Drane et al., 2020 ; Suryaman et al., 2020 ), the inclusion of LRC and LEC would allow us to capture other important challenges that students experience during the pandemic, particularly those from developing regions. This comprehensive list would provide us a clearer and detailed picture of students’ experiences when engaged in online learning in an emergency. Given the restrictions in mobility at macro and micro levels during the pandemic, it is also expected that such conditions would aggravate these challenges. Therefore, this paper intends to understand these challenges from students’ perspectives since they are the ones that are ultimately impacted when the issue is about the learning experience. We also seek to explore areas that provide inconclusive findings, thereby setting the path for future research.
Material and methods
The present study adopted a descriptive, mixed-methods approach to address the research questions. This approach allowed the researchers to collect complex data about students’ experience in an online learning environment and to clearly understand the phenomena from their perspective.
Participants
This study involved 200 (66 male and 134 female) students from a private higher education institution in the Philippines. These participants were Psychology, Physical Education, and Sports Management majors whose ages ranged from 17 to 25 ( x ̅ = 19.81; SD = 1.80). The students have been engaged in online learning for at least two terms in both synchronous and asynchronous modes. The students belonged to low- and middle-income groups but were equipped with the basic online learning equipment (e.g., computer, headset, speakers) and computer skills necessary for their participation in online classes. Table Table1 1 shows the primary and secondary platforms that students used during their online classes. The primary platforms are those that are formally adopted by teachers and students in a structured academic context, whereas the secondary platforms are those that are informally and spontaneously used by students and teachers for informal learning and to supplement instructional delivery. Note that almost all students identified MS Teams as their primary platform because it is the official learning management system of the university.
Participants’ Online Learning Platforms
Learning Platforms | Classification | |||
---|---|---|---|---|
Primary | Supplementary | |||
Blackboard | - | - | 1 | 0.50 |
Canvas | - | - | 1 | 0.50 |
Edmodo | - | - | 1 | 0.50 |
9 | 4.50 | 170 | 85.00 | |
Google Classroom | 5 | 2.50 | 15 | 7.50 |
Moodle | - | - | 7 | 3.50 |
MS Teams | 184 | 92.00 | - | - |
Schoology | 1 | 0.50 | - | - |
- | - | - | - | |
Zoom | 1 | 0.50 | 5 | 2.50 |
200 | 100.00 | 200 | 100.00 |
Informed consent was sought from the participants prior to their involvement. Before students signed the informed consent form, they were oriented about the objectives of the study and the extent of their involvement. They were also briefed about the confidentiality of information, their anonymity, and their right to refuse to participate in the investigation. Finally, the participants were informed that they would incur no additional cost from their participation.
Instrument and data collection
The data were collected using a retrospective self-report questionnaire and a focused group discussion (FGD). A self-report questionnaire was considered appropriate because the indicators relate to affective responses and attitude (Araujo et al., 2017 ; Barrot, 2016 ; Spector, 1994 ). Although the participants may tell more than what they know or do in a self-report survey (Matsumoto, 1994 ), this challenge was addressed by explaining to them in detail each of the indicators and using methodological triangulation through FGD. The questionnaire was divided into four sections: (1) participant’s personal information section, (2) the background information on the online learning environment, (3) the rating scale section for the online learning challenges, (4) the open-ended section. The personal information section asked about the students’ personal information (name, school, course, age, and sex), while the background information section explored the online learning mode and platforms (primary and secondary) used in class, and students’ length of engagement in online classes. The rating scale section contained 37 items that relate to SRC (6 items), TLCC (10 items), SIC (4 items), TSC (6 items), TCC (3 items), LRC (4 items), and LEC (4 items). The Likert scale uses six scores (i.e., 5– to a very great extent , 4– to a great extent , 3– to a moderate extent , 2– to some extent , 1– to a small extent , and 0 –not at all/negligible ) assigned to each of the 37 items. Finally, the open-ended questions asked about other challenges that students experienced, the impact of the pandemic on the intensity or extent of the challenges they experienced, and the strategies that the participants employed to overcome the eight different types of challenges during online learning. Two experienced educators and researchers reviewed the questionnaire for clarity, accuracy, and content and face validity. The piloting of the instrument revealed that the tool had good internal consistency (Cronbach’s α = 0.96).
The FGD protocol contains two major sections: the participants’ background information and the main questions. The background information section asked about the students’ names, age, courses being taken, online learning mode used in class. The items in the main questions section covered questions relating to the students’ overall attitude toward online learning during the pandemic, the reasons for the scores they assigned to each of the challenges they experienced, the impact of the pandemic on students’ challenges, and the strategies they employed to address the challenges. The same experts identified above validated the FGD protocol.
Both the questionnaire and the FGD were conducted online via Google survey and MS Teams, respectively. It took approximately 20 min to complete the questionnaire, while the FGD lasted for about 90 min. Students were allowed to ask for clarification and additional explanations relating to the questionnaire content, FGD, and procedure. Online surveys and interview were used because of the ongoing lockdown in the city. For the purpose of triangulation, 20 (10 from Psychology and 10 from Physical Education and Sports Management) randomly selected students were invited to participate in the FGD. Two separate FGDs were scheduled for each group and were facilitated by researcher 2 and researcher 3, respectively. The interviewers ensured that the participants were comfortable and open to talk freely during the FGD to avoid social desirability biases (Bergen & Labonté, 2020 ). These were done by informing the participants that there are no wrong responses and that their identity and responses would be handled with the utmost confidentiality. With the permission of the participants, the FGD was recorded to ensure that all relevant information was accurately captured for transcription and analysis.
Data analysis
To address the research questions, we used both quantitative and qualitative analyses. For the quantitative analysis, we entered all the data into an excel spreadsheet. Then, we computed the mean scores ( M ) and standard deviations ( SD ) to determine the level of challenges experienced by students during online learning. The mean score for each descriptor was interpreted using the following scheme: 4.18 to 5.00 ( to a very great extent ), 3.34 to 4.17 ( to a great extent ), 2.51 to 3.33 ( to a moderate extent ), 1.68 to 2.50 ( to some extent ), 0.84 to 1.67 ( to a small extent ), and 0 to 0.83 ( not at all/negligible ). The equal interval was adopted because it produces more reliable and valid information than other types of scales (Cicchetti et al., 2006 ).
For the qualitative data, we analyzed the students’ responses in the open-ended questions and the transcribed FGD using the predetermined categories in the conceptual framework. Specifically, we used multilevel coding in classifying the codes from the transcripts (Birks & Mills, 2011 ). To do this, we identified the relevant codes from the responses of the participants and categorized these codes based on the similarities or relatedness of their properties and dimensions. Then, we performed a constant comparative and progressive analysis of cases to allow the initially identified subcategories to emerge and take shape. To ensure the reliability of the analysis, two coders independently analyzed the qualitative data. Both coders familiarize themselves with the purpose, research questions, research method, and codes and coding scheme of the study. They also had a calibration session and discussed ways on how they could consistently analyze the qualitative data. Percent of agreement between the two coders was 86 percent. Any disagreements in the analysis were discussed by the coders until an agreement was achieved.
This study investigated students’ online learning experience in higher education within the context of the pandemic. Specifically, we identified the extent of challenges that students experienced, how the COVID-19 pandemic impacted their online learning experience, and the strategies that they used to confront these challenges.
The extent of students’ online learning challenges
Table Table2 2 presents the mean scores and SD for the extent of challenges that students’ experienced during online learning. Overall, the students experienced the identified challenges to a moderate extent ( x ̅ = 2.62, SD = 1.03) with scores ranging from x ̅ = 1.72 ( to some extent ) to x ̅ = 3.58 ( to a great extent ). More specifically, the greatest challenge that students experienced was related to the learning environment ( x ̅ = 3.49, SD = 1.27), particularly on distractions at home, limitations in completing the requirements for certain subjects, and difficulties in selecting the learning areas and study schedule. It is, however, found that the least challenge was on technological literacy and competency ( x ̅ = 2.10, SD = 1.13), particularly on knowledge and training in the use of technology, technological intimidation, and resistance to learning technologies. Other areas that students experienced the least challenge are Internet access under TSC and procrastination under SRC. Nonetheless, nearly half of the students’ responses per indicator rated the challenges they experienced as moderate (14 of the 37 indicators), particularly in TCC ( x ̅ = 2.51, SD = 1.31), SIC ( x ̅ = 2.77, SD = 1.34), and LRC ( x ̅ = 2.93, SD = 1.31).
The Extent of Students’ Challenges during the Interim Online Learning
CHALLENGES | ||
---|---|---|
Self-regulation challenges (SRC) | 2.37 | 1.16 |
1. I delay tasks related to my studies so that they are either not fully completed by their deadline or had to be rushed to be completed. | 1.84 | 1.47 |
2. I fail to get appropriate help during online classes. | 2.04 | 1.44 |
3. I lack the ability to control my own thoughts, emotions, and actions during online classes. | 2.51 | 1.65 |
4. I have limited preparation before an online class. | 2.68 | 1.54 |
5. I have poor time management skills during online classes. | 2.50 | 1.53 |
6. I fail to properly use online peer learning strategies (i.e., learning from one another to better facilitate learning such as peer tutoring, group discussion, and peer feedback). | 2.34 | 1.50 |
Technological literacy and competency challenges (TLCC) | 2.10 | 1.13 |
7. I lack competence and proficiency in using various interfaces or systems that allow me to control a computer or another embedded system for studying. | 2.05 | 1.39 |
8. I resist learning technology. | 1.89 | 1.46 |
9. I am distracted by an overly complex technology. | 2.44 | 1.43 |
10. I have difficulties in learning a new technology. | 2.06 | 1.50 |
11. I lack the ability to effectively use technology to facilitate learning. | 2.08 | 1.51 |
12. I lack knowledge and training in the use of technology. | 1.76 | 1.43 |
13. I am intimidated by the technologies used for learning. | 1.89 | 1.44 |
14. I resist and/or am confused when getting appropriate help during online classes. | 2.19 | 1.52 |
15. I have poor understanding of directions and expectations during online learning. | 2.16 | 1.56 |
16. I perceive technology as a barrier to getting help from others during online classes. | 2.47 | 1.43 |
Student isolation challenges (SIC) | 2.77 | 1.34 |
17. I feel emotionally disconnected or isolated during online classes. | 2.71 | 1.58 |
18. I feel disinterested during online class. | 2.54 | 1.53 |
19. I feel unease and uncomfortable in using video projection, microphones, and speakers. | 2.90 | 1.57 |
20. I feel uncomfortable being the center of attention during online classes. | 2.93 | 1.67 |
Technological sufficiency challenges (TSC) | 2.31 | 1.29 |
21. I have an insufficient access to learning technology. | 2.27 | 1.52 |
22. I experience inequalities with regard to to and use of technologies during online classes because of my socioeconomic, physical, and psychological condition. | 2.34 | 1.68 |
23. I have an outdated technology. | 2.04 | 1.62 |
24. I do not have Internet access during online classes. | 1.72 | 1.65 |
25. I have low bandwidth and slow processing speeds. | 2.66 | 1.62 |
26. I experience technical difficulties in completing my assignments. | 2.84 | 1.54 |
Technological complexity challenges (TCC) | 2.51 | 1.31 |
27. I am distracted by the complexity of the technology during online classes. | 2.34 | 1.46 |
28. I experience difficulties in using complex technology. | 2.33 | 1.51 |
29. I experience difficulties when using longer videos for learning. | 2.87 | 1.48 |
Learning resource challenges (LRC) | 2.93 | 1.31 |
30. I have an insufficient access to library resources. | 2.86 | 1.72 |
31. I have an insufficient access to laboratory equipment and materials. | 3.16 | 1.71 |
32. I have limited access to textbooks, worksheets, and other instructional materials. | 2.63 | 1.57 |
33. I experience financial challenges when accessing learning resources and technology. | 3.07 | 1.57 |
Learning environment challenges (LEC) | 3.49 | 1.27 |
34. I experience online distractions such as social media during online classes. | 3.20 | 1.58 |
35. I experience distractions at home as a learning environment. | 3.55 | 1.54 |
36. I have difficulties in selecting the best time and area for learning at home. | 3.40 | 1.58 |
37. Home set-up limits the completion of certain requirements for my subject (e.g., laboratory and physical activities). | 3.58 | 1.52 |
AVERAGE | 2.62 | 1.03 |
Out of 200 students, 181 responded to the question about other challenges that they experienced. Most of their responses were already covered by the seven predetermined categories, except for 18 responses related to physical discomfort ( N = 5) and financial challenges ( N = 13). For instance, S108 commented that “when it comes to eyes and head, my eyes and head get ache if the session of class was 3 h straight in front of my gadget.” In the same vein, S194 reported that “the long exposure to gadgets especially laptop, resulting in body pain & headaches.” With reference to physical financial challenges, S66 noted that “not all the time I have money to load”, while S121 claimed that “I don't know until when are we going to afford budgeting our money instead of buying essentials.”
Impact of the pandemic on students’ online learning challenges
Another objective of this study was to identify how COVID-19 influenced the online learning challenges that students experienced. As shown in Table Table3, 3 , most of the students’ responses were related to teaching and learning quality ( N = 86) and anxiety and other mental health issues ( N = 52). Regarding the adverse impact on teaching and learning quality, most of the comments relate to the lack of preparation for the transition to online platforms (e.g., S23, S64), limited infrastructure (e.g., S13, S65, S99, S117), and poor Internet service (e.g., S3, S9, S17, S41, S65, S99). For the anxiety and mental health issues, most students reported that the anxiety, boredom, sadness, and isolation they experienced had adversely impacted the way they learn (e.g., S11, S130), completing their tasks/activities (e.g., S56, S156), and their motivation to continue studying (e.g., S122, S192). The data also reveal that COVID-19 aggravated the financial difficulties experienced by some students ( N = 16), consequently affecting their online learning experience. This financial impact mainly revolved around the lack of funding for their online classes as a result of their parents’ unemployment and the high cost of Internet data (e.g., S18, S113, S167). Meanwhile, few concerns were raised in relation to COVID-19’s impact on mobility ( N = 7) and face-to-face interactions ( N = 7). For instance, some commented that the lack of face-to-face interaction with her classmates had a detrimental effect on her learning (S46) and socialization skills (S36), while others reported that restrictions in mobility limited their learning experience (S78, S110). Very few comments were related to no effect ( N = 4) and positive effect ( N = 2). The above findings suggest the pandemic had additive adverse effects on students’ online learning experience.
Summary of students’ responses on the impact of COVID-19 on their online learning experience
Areas | Sample Responses | |
---|---|---|
Reduces the quality of learning experience | 86 | (S13) (S65) (S118) |
Causes anxiety and other mental health issues | 52 | (S11) (S56) (S192) |
Aggravates financial problems | 16 | (S18) (S167) |
Limits interaction | 7 | (S36) (S46) |
Restricts mobility | 7 | (S78) (S110) |
No effect | 4 | (S100) (S168) |
Positive effect | 2 | (S35) (S112) |
Students’ strategies to overcome challenges in an online learning environment
The third objective of this study is to identify the strategies that students employed to overcome the different online learning challenges they experienced. Table Table4 4 presents that the most commonly used strategies used by students were resource management and utilization ( N = 181), help-seeking ( N = 155), technical aptitude enhancement ( N = 122), time management ( N = 98), and learning environment control ( N = 73). Not surprisingly, the top two strategies were also the most consistently used across different challenges. However, looking closely at each of the seven challenges, the frequency of using a particular strategy varies. For TSC and LRC, the most frequently used strategy was resource management and utilization ( N = 52, N = 89, respectively), whereas technical aptitude enhancement was the students’ most preferred strategy to address TLCC ( N = 77) and TCC ( N = 38). In the case of SRC, SIC, and LEC, the most frequently employed strategies were time management ( N = 71), psychological support ( N = 53), and learning environment control ( N = 60). In terms of consistency, help-seeking appears to be the most consistent across the different challenges in an online learning environment. Table Table4 4 further reveals that strategies used by students within a specific type of challenge vary.
Students’ Strategies to Overcome Online Learning Challenges
Strategies | SRC | TLCC | SIC | TSC | TCC | LRC | LEC | Total |
---|---|---|---|---|---|---|---|---|
Adaptation | 7 | 1 | 11 | 4 | 10 | 10 | 17 | 60 |
Cognitive aptitude enhancement | 2 | 3 | 0 | 0 | 2 | 4 | 2 | 13 |
Concentration and focus | 13 | 2 | 7 | 0 | 4 | 5 | 12 | 43 |
Focus and concentration | 0 | 3 | 0 | 0 | 0 | 0 | 0 | 3 |
Goal-setting | 8 | 0 | 0 | 2 | 2 | 0 | 1 | 13 |
Help-seeking | 13 | 42 | 2 | 36 | 16 | 28 | 18 | 155 |
Learning environment control | 1 | 3 | 0 | 6 | 3 | 0 | 60 | 73 |
Motivation | 2 | 0 | 4 | 0 | 5 | 1 | 0 | 12 |
Optimism | 4 | 5 | 9 | 15 | 9 | 2 | 3 | 47 |
Peer learning | 3 | 2 | 6 | 0 | 1 | 0 | 0 | 12 |
Psychosocial support | 3 | 0 | 53 | 1 | 0 | 0 | 0 | 57 |
Reflection | 6 | 0 | 0 | 0 | 0 | 0 | 0 | 6 |
Relaxation and recreation | 16 | 1 | 13 | 0 | 7 | 0 | 0 | 37 |
Resource management & utilization | 3 | 11 | 0 | 52 | 20 | 89 | 6 | 181 |
Self-belief | 0 | 1 | 11 | 0 | 1 | 0 | 1 | 14 |
Self-discipline | 12 | 3 | 3 | 6 | 3 | 1 | 4 | 32 |
Self-study | 6 | 0 | 0 | 0 | 0 | 1 | 0 | 7 |
Technical aptitude enhancement | 0 | 77 | 0 | 7 | 38 | 0 | 0 | 122 |
Thought control | 6 | 0 | 2 | 0 | 1 | 1 | 3 | 13 |
Time management | 71 | 3 | 2 | 10 | 4 | 3 | 5 | 98 |
Transcendental strategies | 2 | 0 | 0 | 0 | 0 | 0 | 0 | 2 |
Discussion and conclusions
The current study explores the challenges that students experienced in an online learning environment and how the pandemic impacted their online learning experience. The findings revealed that the online learning challenges of students varied in terms of type and extent. Their greatest challenge was linked to their learning environment at home, while their least challenge was technological literacy and competency. Based on the students’ responses, their challenges were also found to be aggravated by the pandemic, especially in terms of quality of learning experience, mental health, finances, interaction, and mobility. With reference to previous studies (i.e., Adarkwah, 2021 ; Copeland et al., 2021 ; Day et al., 2021 ; Fawaz et al., 2021 ; Kapasia et al., 2020 ; Khalil et al., 2020 ; Singh et al., 2020 ), the current study has complemented their findings on the pedagogical, logistical, socioeconomic, technological, and psychosocial online learning challenges that students experience within the context of the COVID-19 pandemic. Further, this study extended previous studies and our understanding of students’ online learning experience by identifying both the presence and extent of online learning challenges and by shedding light on the specific strategies they employed to overcome them.
Overall findings indicate that the extent of challenges and strategies varied from one student to another. Hence, they should be viewed as a consequence of interaction several many factors. Students’ responses suggest that their online learning challenges and strategies were mediated by the resources available to them, their interaction with their teachers and peers, and the school’s existing policies and guidelines for online learning. In the context of the pandemic, the imposed lockdowns and students’ socioeconomic condition aggravated the challenges that students experience.
While most studies revealed that technology use and competency were the most common challenges that students face during the online classes (see Rasheed et al., 2020 ), the case is a bit different in developing countries in times of pandemic. As the findings have shown, the learning environment is the greatest challenge that students needed to hurdle, particularly distractions at home (e.g., noise) and limitations in learning space and facilities. This data suggests that online learning challenges during the pandemic somehow vary from the typical challenges that students experience in a pre-pandemic online learning environment. One possible explanation for this result is that restriction in mobility may have aggravated this challenge since they could not go to the school or other learning spaces beyond the vicinity of their respective houses. As shown in the data, the imposition of lockdown restricted students’ learning experience (e.g., internship and laboratory experiments), limited their interaction with peers and teachers, caused depression, stress, and anxiety among students, and depleted the financial resources of those who belong to lower-income group. All of these adversely impacted students’ learning experience. This finding complemented earlier reports on the adverse impact of lockdown on students’ learning experience and the challenges posed by the home learning environment (e.g., Day et al., 2021 ; Kapasia et al., 2020 ). Nonetheless, further studies are required to validate the impact of restrictions on mobility on students’ online learning experience. The second reason that may explain the findings relates to students’ socioeconomic profile. Consistent with the findings of Adarkwah ( 2021 ) and Day et al. ( 2021 ), the current study reveals that the pandemic somehow exposed the many inequities in the educational systems within and across countries. In the case of a developing country, families from lower socioeconomic strata (as in the case of the students in this study) have limited learning space at home, access to quality Internet service, and online learning resources. This is the reason the learning environment and learning resources recorded the highest level of challenges. The socioeconomic profile of the students (i.e., low and middle-income group) is the same reason financial problems frequently surfaced from their responses. These students frequently linked the lack of financial resources to their access to the Internet, educational materials, and equipment necessary for online learning. Therefore, caution should be made when interpreting and extending the findings of this study to other contexts, particularly those from higher socioeconomic strata.
Among all the different online learning challenges, the students experienced the least challenge on technological literacy and competency. This is not surprising considering a plethora of research confirming Gen Z students’ (born since 1996) high technological and digital literacy (Barrot, 2018 ; Ng, 2012 ; Roblek et al., 2019 ). Regarding the impact of COVID-19 on students’ online learning experience, the findings reveal that teaching and learning quality and students’ mental health were the most affected. The anxiety that students experienced does not only come from the threats of COVID-19 itself but also from social and physical restrictions, unfamiliarity with new learning platforms, technical issues, and concerns about financial resources. These findings are consistent with that of Copeland et al. ( 2021 ) and Fawaz et al. ( 2021 ), who reported the adverse effects of the pandemic on students’ mental and emotional well-being. This data highlights the need to provide serious attention to the mediating effects of mental health, restrictions in mobility, and preparedness in delivering online learning.
Nonetheless, students employed a variety of strategies to overcome the challenges they faced during online learning. For instance, to address the home learning environment problems, students talked to their family (e.g., S12, S24), transferred to a quieter place (e.g., S7, S 26), studied at late night where all family members are sleeping already (e.g., S51), and consulted with their classmates and teachers (e.g., S3, S9, S156, S193). To overcome the challenges in learning resources, students used the Internet (e.g., S20, S27, S54, S91), joined Facebook groups that share free resources (e.g., S5), asked help from family members (e.g., S16), used resources available at home (e.g., S32), and consulted with the teachers (e.g., S124). The varying strategies of students confirmed earlier reports on the active orientation that students take when faced with academic- and non-academic-related issues in an online learning space (see Fawaz et al., 2021 ). The specific strategies that each student adopted may have been shaped by different factors surrounding him/her, such as available resources, student personality, family structure, relationship with peers and teacher, and aptitude. To expand this study, researchers may further investigate this area and explore how and why different factors shape their use of certain strategies.
Several implications can be drawn from the findings of this study. First, this study highlighted the importance of emergency response capability and readiness of higher education institutions in case another crisis strikes again. Critical areas that need utmost attention include (but not limited to) national and institutional policies, protocol and guidelines, technological infrastructure and resources, instructional delivery, staff development, potential inequalities, and collaboration among key stakeholders (i.e., parents, students, teachers, school leaders, industry, government education agencies, and community). Second, the findings have expanded our understanding of the different challenges that students might confront when we abruptly shift to full online learning, particularly those from countries with limited resources, poor Internet infrastructure, and poor home learning environment. Schools with a similar learning context could use the findings of this study in developing and enhancing their respective learning continuity plans to mitigate the adverse impact of the pandemic. This study would also provide students relevant information needed to reflect on the possible strategies that they may employ to overcome the challenges. These are critical information necessary for effective policymaking, decision-making, and future implementation of online learning. Third, teachers may find the results useful in providing proper interventions to address the reported challenges, particularly in the most critical areas. Finally, the findings provided us a nuanced understanding of the interdependence of learning tools, learners, and learning outcomes within an online learning environment; thus, giving us a multiperspective of hows and whys of a successful migration to full online learning.
Some limitations in this study need to be acknowledged and addressed in future studies. One limitation of this study is that it exclusively focused on students’ perspectives. Future studies may widen the sample by including all other actors taking part in the teaching–learning process. Researchers may go deeper by investigating teachers’ views and experience to have a complete view of the situation and how different elements interact between them or affect the others. Future studies may also identify some teacher-related factors that could influence students’ online learning experience. In the case of students, their age, sex, and degree programs may be examined in relation to the specific challenges and strategies they experience. Although the study involved a relatively large sample size, the participants were limited to college students from a Philippine university. To increase the robustness of the findings, future studies may expand the learning context to K-12 and several higher education institutions from different geographical regions. As a final note, this pandemic has undoubtedly reshaped and pushed the education system to its limits. However, this unprecedented event is the same thing that will make the education system stronger and survive future threats.
Authors’ contributions
Jessie Barrot led the planning, prepared the instrument, wrote the report, and processed and analyzed data. Ian Llenares participated in the planning, fielded the instrument, processed and analyzed data, reviewed the instrument, and contributed to report writing. Leo del Rosario participated in the planning, fielded the instrument, processed and analyzed data, reviewed the instrument, and contributed to report writing.
No funding was received in the conduct of this study.
Availability of data and materials
Declarations.
The study has undergone appropriate ethics protocol.
Informed consent was sought from the participants.
Authors consented the publication. Participants consented to publication as long as confidentiality is observed.
Publisher’s note
Springer Nature remains neutral with regard to jurisdictional claims in published maps and institutional affiliations.
- Adarkwah MA. “I’m not against online teaching, but what about us?”: ICT in Ghana post Covid-19. Education and Information Technologies. 2021; 26 (2):1665–1685. doi: 10.1007/s10639-020-10331-z. [ PMC free article ] [ PubMed ] [ CrossRef ] [ Google Scholar ]
- Almaiah MA, Al-Khasawneh A, Althunibat A. Exploring the critical challenges and factors influencing the E-learning system usage during COVID-19 pandemic. Education and Information Technologies. 2020; 25 :5261–5280. doi: 10.1007/s10639-020-10219-y. [ PMC free article ] [ PubMed ] [ CrossRef ] [ Google Scholar ]
- Araujo T, Wonneberger A, Neijens P, de Vreese C. How much time do you spend online? Understanding and improving the accuracy of self-reported measures of Internet use. Communication Methods and Measures. 2017; 11 (3):173–190. doi: 10.1080/19312458.2017.1317337. [ CrossRef ] [ Google Scholar ]
- Barrot, J. S. (2016). Using Facebook-based e-portfolio in ESL writing classrooms: Impact and challenges. Language, Culture and Curriculum, 29 (3), 286–301.
- Barrot, J. S. (2018). Facebook as a learning environment for language teaching and learning: A critical analysis of the literature from 2010 to 2017. Journal of Computer Assisted Learning, 34 (6), 863–875.
- Barrot, J. S. (2020). Scientific mapping of social media in education: A decade of exponential growth. Journal of Educational Computing Research . 10.1177/0735633120972010.
- Barrot, J. S. (2021). Social media as a language learning environment: A systematic review of the literature (2008–2019). Computer Assisted Language Learning . 10.1080/09588221.2021.1883673.
- Bergen N, Labonté R. “Everything is perfect, and we have no problems”: Detecting and limiting social desirability bias in qualitative research. Qualitative Health Research. 2020; 30 (5):783–792. doi: 10.1177/1049732319889354. [ PubMed ] [ CrossRef ] [ Google Scholar ]
- Birks, M., & Mills, J. (2011). Grounded theory: A practical guide . Sage.
- Boelens R, De Wever B, Voet M. Four key challenges to the design of blended learning: A systematic literature review. Educational Research Review. 2017; 22 :1–18. doi: 10.1016/j.edurev.2017.06.001. [ CrossRef ] [ Google Scholar ]
- Buehler MA. Where is the library in course management software? Journal of Library Administration. 2004; 41 (1–2):75–84. doi: 10.1300/J111v41n01_07. [ CrossRef ] [ Google Scholar ]
- Carter RA, Jr, Rice M, Yang S, Jackson HA. Self-regulated learning in online learning environments: Strategies for remote learning. Information and Learning Sciences. 2020; 121 (5/6):321–329. doi: 10.1108/ILS-04-2020-0114. [ CrossRef ] [ Google Scholar ]
- Cavanaugh CS, Barbour MK, Clark T. Research and practice in K-12 online learning: A review of open access literature. The International Review of Research in Open and Distributed Learning. 2009; 10 (1):1–22. doi: 10.19173/irrodl.v10i1.607. [ CrossRef ] [ Google Scholar ]
- Cicchetti D, Bronen R, Spencer S, Haut S, Berg A, Oliver P, Tyrer P. Rating scales, scales of measurement, issues of reliability: Resolving some critical issues for clinicians and researchers. The Journal of Nervous and Mental Disease. 2006; 194 (8):557–564. doi: 10.1097/01.nmd.0000230392.83607.c5. [ PubMed ] [ CrossRef ] [ Google Scholar ]
- Copeland WE, McGinnis E, Bai Y, Adams Z, Nardone H, Devadanam V, Hudziak JJ. Impact of COVID-19 pandemic on college student mental health and wellness. Journal of the American Academy of Child & Adolescent Psychiatry. 2021; 60 (1):134–141. doi: 10.1016/j.jaac.2020.08.466. [ PMC free article ] [ PubMed ] [ CrossRef ] [ Google Scholar ]
- Day T, Chang ICC, Chung CKL, Doolittle WE, Housel J, McDaniel PN. The immediate impact of COVID-19 on postsecondary teaching and learning. The Professional Geographer. 2021; 73 (1):1–13. doi: 10.1080/00330124.2020.1823864. [ CrossRef ] [ Google Scholar ]
- Donitsa-Schmidt S, Ramot R. Opportunities and challenges: Teacher education in Israel in the Covid-19 pandemic. Journal of Education for Teaching. 2020; 46 (4):586–595. doi: 10.1080/02607476.2020.1799708. [ CrossRef ] [ Google Scholar ]
- Drane, C., Vernon, L., & O’Shea, S. (2020). The impact of ‘learning at home’on the educational outcomes of vulnerable children in Australia during the COVID-19 pandemic. Literature Review Prepared by the National Centre for Student Equity in Higher Education. Curtin University, Australia.
- Elaish M, Shuib L, Ghani N, Yadegaridehkordi E. Mobile English language learning (MELL): A literature review. Educational Review. 2019; 71 (2):257–276. doi: 10.1080/00131911.2017.1382445. [ CrossRef ] [ Google Scholar ]
- Fawaz, M., Al Nakhal, M., & Itani, M. (2021). COVID-19 quarantine stressors and management among Lebanese students: A qualitative study. Current Psychology , 1–8. [ PMC free article ] [ PubMed ]
- Franchi T. The impact of the Covid-19 pandemic on current anatomy education and future careers: A student’s perspective. Anatomical Sciences Education. 2020; 13 (3):312–315. doi: 10.1002/ase.1966. [ PMC free article ] [ PubMed ] [ CrossRef ] [ Google Scholar ]
- Garcia R, Falkner K, Vivian R. Systematic literature review: Self-regulated learning strategies using e-learning tools for computer science. Computers & Education. 2018; 123 :150–163. doi: 10.1016/j.compedu.2018.05.006. [ CrossRef ] [ Google Scholar ]
- Gonzalez T, De La Rubia MA, Hincz KP, Comas-Lopez M, Subirats L, Fort S, Sacha GM. Influence of COVID-19 confinement on students’ performance in higher education. PLoS ONE. 2020; 15 (10):e0239490. doi: 10.1371/journal.pone.0239490. [ PMC free article ] [ PubMed ] [ CrossRef ] [ Google Scholar ]
- Hew KF, Jia C, Gonda DE, Bai S. Transitioning to the “new normal” of learning in unpredictable times: Pedagogical practices and learning performance in fully online flipped classrooms. International Journal of Educational Technology in Higher Education. 2020; 17 (1):1–22. doi: 10.1186/s41239-020-00234-x. [ PMC free article ] [ PubMed ] [ CrossRef ] [ Google Scholar ]
- Huang Q. Comparing teacher’s roles of F2F learning and online learning in a blended English course. Computer Assisted Language Learning. 2019; 32 (3):190–209. doi: 10.1080/09588221.2018.1540434. [ CrossRef ] [ Google Scholar ]
- John Hopkins University. (2021). Global map . https://coronavirus.jhu.edu/
- Kapasia N, Paul P, Roy A, Saha J, Zaveri A, Mallick R, Chouhan P. Impact of lockdown on learning status of undergraduate and postgraduate students during COVID-19 pandemic in West Bengal. India. Children and Youth Services Review. 2020; 116 :105194. doi: 10.1016/j.childyouth.2020.105194. [ PMC free article ] [ PubMed ] [ CrossRef ] [ Google Scholar ]
- Kebritchi M, Lipschuetz A, Santiague L. Issues and challenges for teaching successful online courses in higher education: A literature review. Journal of Educational Technology Systems. 2017; 46 (1):4–29. doi: 10.1177/0047239516661713. [ CrossRef ] [ Google Scholar ]
- Khalil R, Mansour AE, Fadda WA, Almisnid K, Aldamegh M, Al-Nafeesah A, Al-Wutayd O. The sudden transition to synchronized online learning during the COVID-19 pandemic in Saudi Arabia: A qualitative study exploring medical students’ perspectives. BMC Medical Education. 2020; 20 (1):1–10. doi: 10.1186/s12909-020-02208-z. [ PMC free article ] [ PubMed ] [ CrossRef ] [ Google Scholar ]
- Matsumoto K. Introspection, verbal reports and second language learning strategy research. Canadian Modern Language Review. 1994; 50 (2):363–386. doi: 10.3138/cmlr.50.2.363. [ CrossRef ] [ Google Scholar ]
- Ng W. Can we teach digital natives digital literacy? Computers & Education. 2012; 59 (3):1065–1078. doi: 10.1016/j.compedu.2012.04.016. [ CrossRef ] [ Google Scholar ]
- Pham T, Nguyen H. COVID-19: Challenges and opportunities for Vietnamese higher education. Higher Education in Southeast Asia and beyond. 2020; 8 :22–24. [ Google Scholar ]
- Rasheed RA, Kamsin A, Abdullah NA. Challenges in the online component of blended learning: A systematic review. Computers & Education. 2020; 144 :103701. doi: 10.1016/j.compedu.2019.103701. [ CrossRef ] [ Google Scholar ]
- Recker MM, Dorward J, Nelson LM. Discovery and use of online learning resources: Case study findings. Educational Technology & Society. 2004; 7 (2):93–104. [ Google Scholar ]
- Roblek V, Mesko M, Dimovski V, Peterlin J. Smart technologies as social innovation and complex social issues of the Z generation. Kybernetes. 2019; 48 (1):91–107. doi: 10.1108/K-09-2017-0356. [ CrossRef ] [ Google Scholar ]
- Seplaki CL, Agree EM, Weiss CO, Szanton SL, Bandeen-Roche K, Fried LP. Assistive devices in context: Cross-sectional association between challenges in the home environment and use of assistive devices for mobility. The Gerontologist. 2014; 54 (4):651–660. doi: 10.1093/geront/gnt030. [ PMC free article ] [ PubMed ] [ CrossRef ] [ Google Scholar ]
- Simbulan N. COVID-19 and its impact on higher education in the Philippines. Higher Education in Southeast Asia and beyond. 2020; 8 :15–18. [ Google Scholar ]
- Singh K, Srivastav S, Bhardwaj A, Dixit A, Misra S. Medical education during the COVID-19 pandemic: a single institution experience. Indian Pediatrics. 2020; 57 (7):678–679. doi: 10.1007/s13312-020-1899-2. [ PMC free article ] [ PubMed ] [ CrossRef ] [ Google Scholar ]
- Singh V, Thurman A. How many ways can we define online learning? A systematic literature review of definitions of online learning (1988–2018) American Journal of Distance Education. 2019; 33 (4):289–306. doi: 10.1080/08923647.2019.1663082. [ CrossRef ] [ Google Scholar ]
- Spector P. Using self-report questionnaires in OB research: A comment on the use of a controversial method. Journal of Organizational Behavior. 1994; 15 (5):385–392. doi: 10.1002/job.4030150503. [ CrossRef ] [ Google Scholar ]
- Suryaman M, Cahyono Y, Muliansyah D, Bustani O, Suryani P, Fahlevi M, Munthe AP. COVID-19 pandemic and home online learning system: Does it affect the quality of pharmacy school learning? Systematic Reviews in Pharmacy. 2020; 11 :524–530. [ Google Scholar ]
- Tallent-Runnels MK, Thomas JA, Lan WY, Cooper S, Ahern TC, Shaw SM, Liu X. Teaching courses online: A review of the research. Review of Educational Research. 2006; 76 (1):93–135. doi: 10.3102/00346543076001093. [ CrossRef ] [ Google Scholar ]
- Tang, T., Abuhmaid, A. M., Olaimat, M., Oudat, D. M., Aldhaeebi, M., & Bamanger, E. (2020). Efficiency of flipped classroom with online-based teaching under COVID-19. Interactive Learning Environments , 1–12.
- Usher M, Barak M. Team diversity as a predictor of innovation in team projects of face-to-face and online learners. Computers & Education. 2020; 144 :103702. doi: 10.1016/j.compedu.2019.103702. [ CrossRef ] [ Google Scholar ]
- Varea, V., & González-Calvo, G. (2020). Touchless classes and absent bodies: Teaching physical education in times of Covid-19. Sport, Education and Society , 1–15.
- Wallace RM. Online learning in higher education: A review of research on interactions among teachers and students. Education, Communication & Information. 2003; 3 (2):241–280. doi: 10.1080/14636310303143. [ CrossRef ] [ Google Scholar ]
- World Health Organization (2020). Coronavirus . https://www.who.int/health-topics/coronavirus#tab=tab_1
- Xue, E., Li, J., Li, T., & Shang, W. (2020). China’s education response to COVID-19: A perspective of policy analysis. Educational Philosophy and Theory , 1–13.

COMMENTS
[email protected], [email protected]. Cebu Normal University. Abstract. This study presented a meta-synthesis of Filipino students' experiences in Online. Learning. It utilized 15 out ...
The finding of the research revealed that online learning via Microsoft Teams is categorized as something new for the students but this interaction and learning environment motivated students in ...
The DepEd launched different distance learning modalities to ensure the continuation of teaching-learning process and also it was the department responds to the situation brought by the pandemic. This research was to assess the used of modular distance learning and online distance learning in the subject Filipino 10.
Academia.edu is a platform for academics to share research papers. ... Ang Asignaturang Filipino ay kabilang sa K to 12 Curriculum na idinisenyo ng mga eskperto sa Kagawaran ng Edukasyon upang mapalawak ang kaalaman ng bawat Filipino patungkol sa sariling wika at ang mga kagamitan nito. ... Layunin ng pananaliksik ang makabuo ng mga lunsarang ...
This paper presents a Teacher-Friendly Class Observation Post-Conference Framework anchored on Carl Roger's Self Theory which focuses on a person-centered approach, thus having self as the core ...
Lozada, et al., Mamaya na, Maaga pa Naman: Ang Implikasyon ng "Filipino Time" … _____ 107 Asia Pacific Journal of Management and Sustainable Development Volume 10, No 3, September 2022 Simula noong Akademikong Taong 2020- 2021, ipinatupad na sa Pilipinas ang distance learning para hindi tumigil o huminto ang pag-aaral sa kabila ng
Ang online class ay umusbong nang magsimulang magkaroon ng pandemya sa ating bansa nga COVID-19 noong taong 2020. Naging daan ang online class upang maipagpatuloy ang pag aaral ng mga mag aaral kahit hindi pumupunta sa paaralan partikular na sa Bestlink College of the Philippines. Ito'y nangangailangan lamang ng mga kagamitan tulad ng kompyuter, o kahit anong gadyet at internet. Naging ...
Online education allows learners to develop knowledge and skills flexibly and conveniently—an observation made among students whose characteristics involve student engagement, self-regulation, and self-efficacy. However, studies characterizing Filipino online learners seem to be lacking. This study aimed to characterize science education tertiary students in the Philippines concerning their ...
Academia.edu is a platform for academics to share research papers. Filipino Students' Experiences in Online Learning: A Meta-Synthesis (PDF) Filipino Students' Experiences in Online Learning: A Meta-Synthesis | ALLANAH CAPANGPANGAN - Academia.edu
However, this poses challenges for many colleges, especially state universities in the Philippines, due to online learning being a stark contrast to 228} JIP-The International Journal of Social Sciences Exploring the Online Learning Experience of Filipino College Students Louie Giray et.al. traditional learning environments; and its abrupt ...
Abstract. The COVID-19 pandemic brought great disruption to all aspects of life specifically on how classes were conducted both in an offline and online modes. The sudden shift to purely online method of teaching and learning was a result of the lockdowns that were imposed by the Philippine government. While some institutions have dealt with ...
A lecture on the challenges faced by Philippines public school teachers in blended/online learning and teaching as they embraced the new normal caused by COVID-19 pandemic on 11 July 2020.
This study was endeavored to understand the online learning experience of Filipino college students enrolled in the academic year 2020-2021 during the COVID-19 pandemic. The data were obtained through an open-ended qualitative survey. The responses were analyzed and interpreted using thematic analysis. A total of 71 Filipino college students from state and local universities in the Philippines ...
Additionally, we would like to identify all the possible challenges that Filipino students encounter while having online learning. Methodology Research Design: In the study, meta-synthesis was applied in order to synthesize data on students' online learning experiences in the Philippines. In connection with this paper, online learning ...
Considering that the COVID-19 crisis restricts physical encounters, Higher Education institutions enforced online learning for the academic year 2020- 2021. The practice of speaking and listening skills in the current setting is crucial due to the absence of social presence and limited interactions, even more so when students’ perceptions and attitudes towards online learning are ...
Introduction The COVID-19 pandemic declared by the WHO has affected many countries rendering everyday lives halted. In the Philippines, the lockdown quarantine protocols have shifted the traditional college classes to online. The abrupt transition to online classes may bring psychological effects to college students due to continuous isolation and lack of interaction with fellow students and ...
A. Research Design. In the study, meta-synthesis was applied in order to synthesize data on students' online learning experiences in the Philippines. In connection with this paper, online learning, experiences, and challenges of the Filipino students will be reviewed by utilizing qualitative data. By doing it, relevant data will be drawn upon ...
In March 2020, the COVID-19 pandemic forced over 1 billion learners to shift from face-to-face instruction to online learning. Seven months after it began, this transition became even more challenging for Filipino online learners. Eight typhoons struck the Philippines from October to November 2020. Two of these typhoons caused widespread flooding, utilities interruptions, property destruction ...
The purpose of this study is to analyze the effect of online education, which has been extensively used on student achievement since the beginning of the pandemic. In line with this purpose, a meta-analysis of the related studies focusing on the effect of online education on students' academic achievement in several countries between the years 2010 and 2021 was carried out. Furthermore, this ...
The students have been engaged in online learning for at least two terms in both synchronous and asynchronous modes. The students belonged to low- and middle-income groups but were equipped with the basic online learning equipment (e.g., computer, headset, speakers) and computer skills necessary for their participation in online classes.
Abstract. The COVID-19 pandemic brought great disruption to all aspects of life specifically on how. classes were conducted both in an offline and online modes. The sudden shift to purely online ...
This paper draws on data from a project that investigated teachers' experiences during their professional learning with an online learning platform called inclusionED. Developed by Autism CRC, inclusionED provides access to evidence-based and research-informed teaching practices and tools to support diverse learners in inclusive classrooms ...
The authors in ref. proposed the multi-snapshot sparse Bayesian learning (SBL) target direction of arrive (DOA) estimation algorithm that achieves super resolution under the condition of Gaussian noise background. The cross-spectral density matrix in the algorithm can be obtained through adaptive learning from the observed data.
In connection with this paper, online learning, experiences, and challenges of the Filipino students will be reviewed by utilizing qualitative data. By doing it, relevant data will be drawn upon to help build a stronger foundation relating to online learning while addressing the concerns of the students.